Introduction
Reliability Growth Analysis (RGA) is a vital tool in product development. It focuses on improving product reliability through structured analysis. By systematically identifying and addressing weaknesses, RGA enhances overall product performance. Advanced statistical models play a crucial role in this process, allowing for accurate assessments and predictions.
If you’re interested in diving deeper into the world of statistical models, consider checking out The Crow-AMSAA Model Book. It’s a great read for anyone looking to enhance their understanding of reliability growth!
Summary and Overview
Reliability Growth Analysis (RGA) evaluates product reliability throughout development. It tracks changes over time, identifying failure patterns and improvements. The Crow-AMSAA model, along with other statistical tools, helps quantify reliability growth. RGA utilizes two main data types: developmental data, gathered during testing, and fielded data, collected from products in real-world use. Implementing RGA benefits organizations by improving product reliability, reducing costs, and enhancing customer satisfaction. This strategic approach supports better decision-making and fosters continuous improvement.
For those looking to broaden their statistical knowledge, Statistical Analysis with R for Dummies is a fantastic resource. It simplifies complex concepts, making them accessible to everyone!
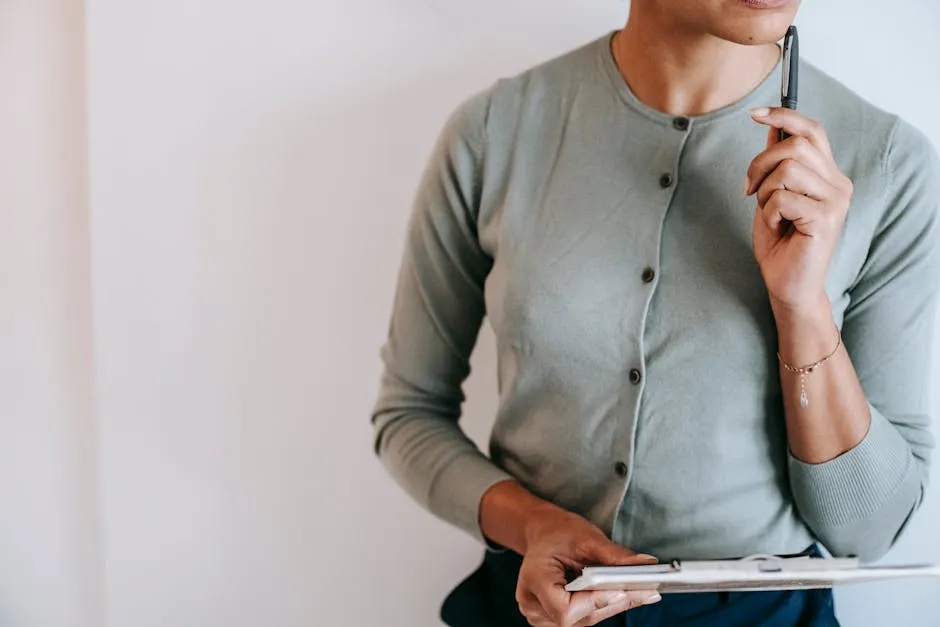
Understanding Reliability Growth Analysis
What is Reliability Growth Analysis?
Reliability Growth Analysis (RGA) is a method used to evaluate and enhance the reliability of products during development. Its primary purpose is to identify and rectify weaknesses in design or manufacturing processes. By systematically analyzing failure data, RGA aims to predict a product’s reliability as it matures.
RGA is particularly significant in product reliability as it helps organizations reduce failures and improve performance. It provides insights into failure patterns, which enables teams to implement corrective actions effectively. This proactive approach is crucial in ensuring that products meet reliability goals before they enter the market.
RGA is applied at various stages of product development. Initially, during the prototype phase, it helps identify design flaws. As testing progresses, RGA continues to track reliability improvements through iterative cycles of testing and refinement. This ensures that by the time the product reaches customers, it is both reliable and robust.
Speaking of reliability, if you’re looking for a comprehensive guide, consider Reliability Engineering and Risk Analysis: A Practical Guide. It’s packed with valuable insights for enhancing product reliability.
The Importance of RGA in Product Development
RGA plays a vital role in continuous improvement. It allows teams to monitor reliability trends and make data-driven decisions. By analyzing failure data and customer feedback, organizations can identify areas for enhancement. This iterative process fosters a culture of quality and reliability.
Customer satisfaction is greatly impacted by RGA. When products are more reliable, customers experience fewer issues, leading to increased trust and loyalty. Moreover, RGA contributes to operational efficiency. It reduces costs associated with warranty claims and repairs, ultimately improving the bottom line.
If you’re interested in learning more about the intersection of data and business, I recommend Data Science for Business: What You Need to Know about Data Mining and Data-Analytic Thinking. It’s a must-read for anyone looking to leverage data in their decision-making processes!
In today’s competitive market, organizations that prioritize RGA gain a significant advantage. By investing in reliability growth, they not only enhance product quality but also strengthen their brand reputation. This holistic approach to product development is essential for long-term success.
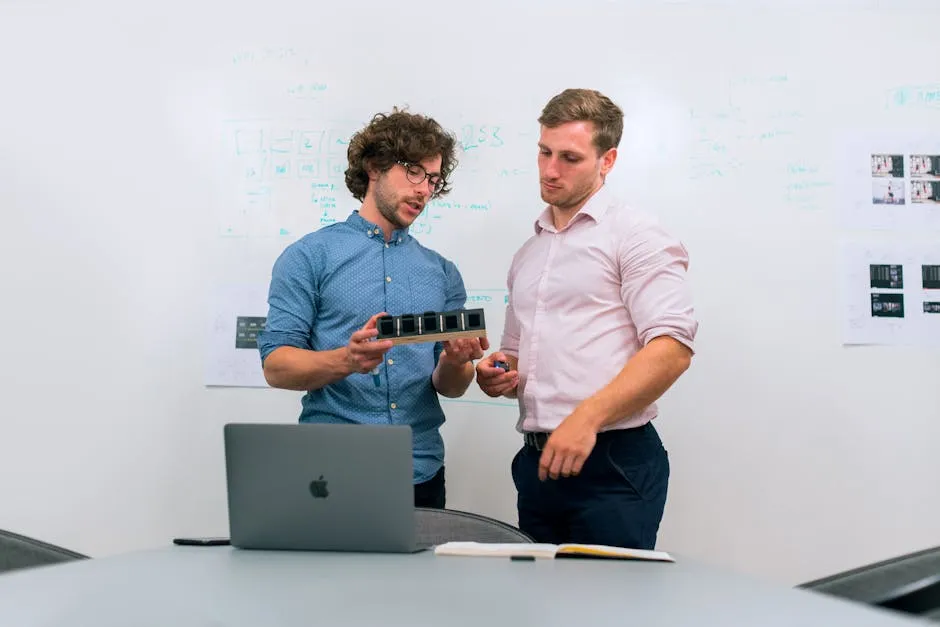
Key Statistical Models in RGA
The Crow-AMSAA Model
The Crow-AMSAA model is essential in Reliability Growth Analysis (RGA). This statistical model effectively tracks reliability improvements over time. It analyzes failure data, revealing how corrective actions impact reliability. The model utilizes the Non-Homogeneous Poisson Process (NHPP), which allows the failure rate to change based on improvements made during development or operational phases.
In NHPP, the failure rate isn’t constant. Instead, it varies over time, indicating whether reliability is increasing or decreasing. This characteristic makes NHPP particularly valuable for organizations aiming to enhance product performance as they can adapt strategies based on real-time data. By applying the Crow-AMSAA model, teams can pinpoint reliability trends and make informed decisions for future enhancements.
If you’re keen on mastering statistical methods, consider picking up Practical Reliability Engineering. It’s a practical guide that provides hands-on techniques to improve reliability.
Other Models Used in RGA
In addition to the Crow-AMSAA model, several alternative models are commonly used in RGA. These include the Gompertz, Modified Gompertz, and Weibull models. Each has unique characteristics suited for different scenarios.
The Gompertz model is beneficial for systems experiencing an increasing failure rate, while the Modified Gompertz offers flexibility for various failure behaviors. The Weibull model is versatile and can handle various data types, making it a reliable choice in many cases. Selecting the appropriate model depends on your specific data type and analysis needs. Understanding the context and characteristics of each model ensures effective reliability analysis, helping organizations make data-driven decisions for product improvements.
For a more in-depth understanding of statistical analysis techniques, you might find Weibull Analysis for Reliability very useful. It offers practical insights into using Weibull analysis effectively across various applications!
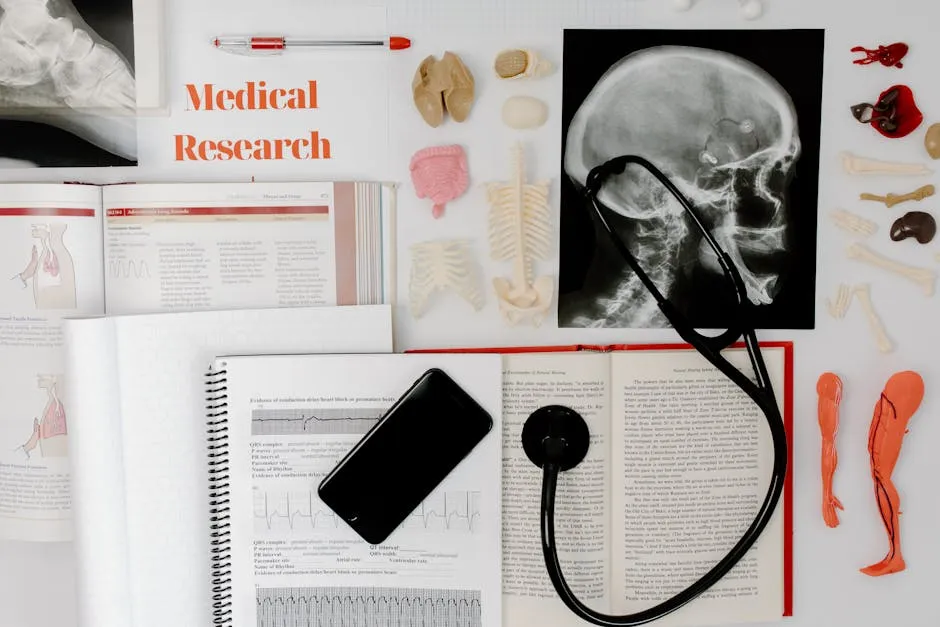
For a deeper understanding of statistical models, you can explore generative ai models are statistical models true or false.
Data Types and Their Role in RGA
In Reliability Growth Analysis (RGA), understanding the types of data collected is crucial. There are two primary categories: developmental data and fielded data.
Developmental data is gathered during controlled testing phases. This type involves analyzing prototypes and early versions. It helps identify design flaws before full-scale production. Collecting this data often uses structured test environments, ensuring reliability metrics are accurate.
On the other hand, fielded data is collected from products in real-world conditions. This data comes from systems already in use, providing insights into how products perform under typical customer interactions. Fielded data can reveal failure patterns that developmental testing might miss.
The implications of these data types on reliability analysis are significant. Developmental data is beneficial for early-stage improvements, while fielded data informs ongoing reliability management. Both types must be analyzed to create a comprehensive view of a product’s performance over time, allowing for informed decision-making.
To further your understanding of data types, you might want to explore Introduction to Reliability Engineering. It’s a great foundational text for understanding the principles behind reliability engineering.
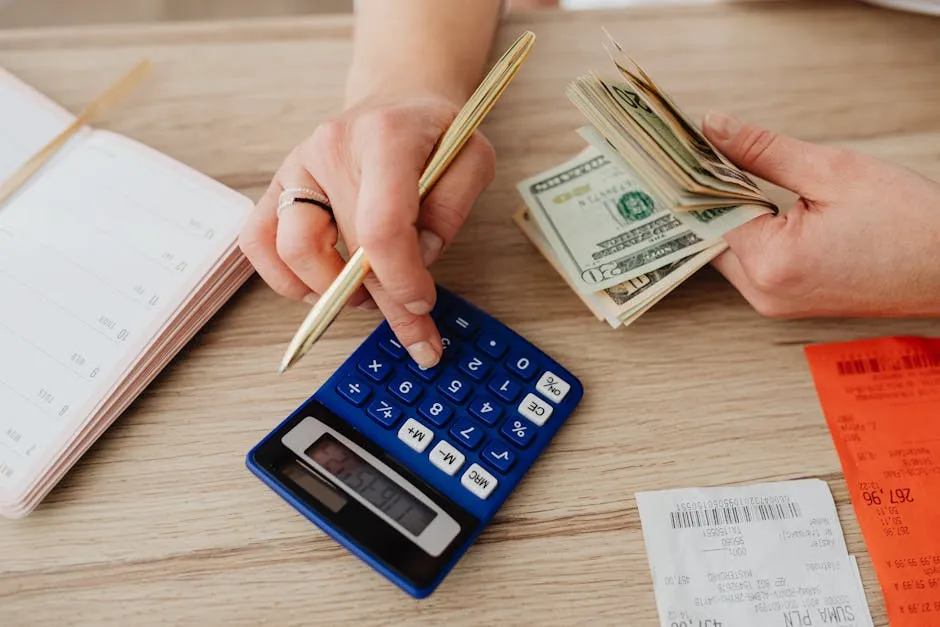
To learn more about various data analysis techniques, check out data analysis techniques for economics and statistics students.
Data Analysis Techniques in RGA
Several data analysis techniques play a vital role in RGA. Common methods include statistical modeling, trend analysis, and graphical representation of reliability data. Each technique serves to elucidate reliability growth patterns effectively.
The quality and integrity of data are paramount in achieving reliable results. Poor quality data can lead to misleading conclusions. Therefore, rigorous data collection and validation processes are essential. Ensure that data is accurate and representative of real-world conditions for effective analysis.
Common pitfalls in data analysis include overlooking data outliers or biases in the dataset. These can skew results and hinder accurate reliability projections. By focusing on data quality and employing diverse analysis techniques, organizations can enhance their RGA efforts and improve overall product reliability.
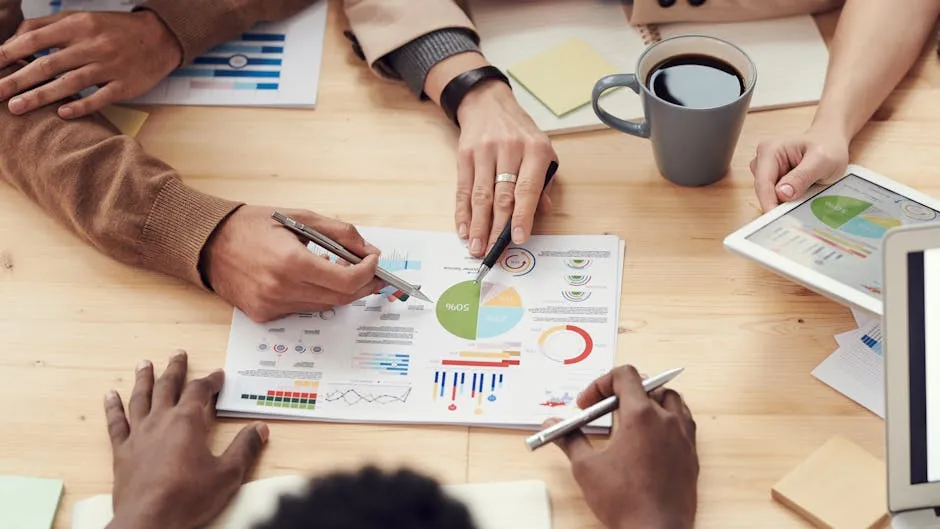
Conducting Reliability Growth Analysis
Steps for Implementing RGA
Conducting a Reliability Growth Analysis (RGA) involves several key steps to ensure success. First, define your objectives clearly. What reliability metrics do you aim to improve? Setting specific goals will guide your analysis effectively.
Next, design a comprehensive test plan. Choose the right environment, duration, and sample size for your tests. Ensure you include clear failure criteria to track issues accurately. This preparation sets the stage for reliable data collection.
Once the plan is in place, execute the tests and gather data. Maintain strict protocols to ensure data accuracy. Document any anomalies during testing, as they can provide valuable insights later.
After collecting data, analyze it using appropriate statistical methods. Select a model, like the Crow-AMSAA, that fits your data type. Estimate parameters and validate the model with independent data if possible. This step is crucial for understanding reliability trends.
Finally, identify and implement corrective actions based on your analysis. Prioritize failure modes that significantly impact reliability. Monitor the effectiveness of these actions and adjust your model accordingly. This iterative process fosters continual improvement.
Best practices include engaging cross-functional teams and maintaining open communication throughout the analysis. Common challenges often arise from data quality issues or inadequate testing environments. Addressing these proactively ensures a smoother RGA process.
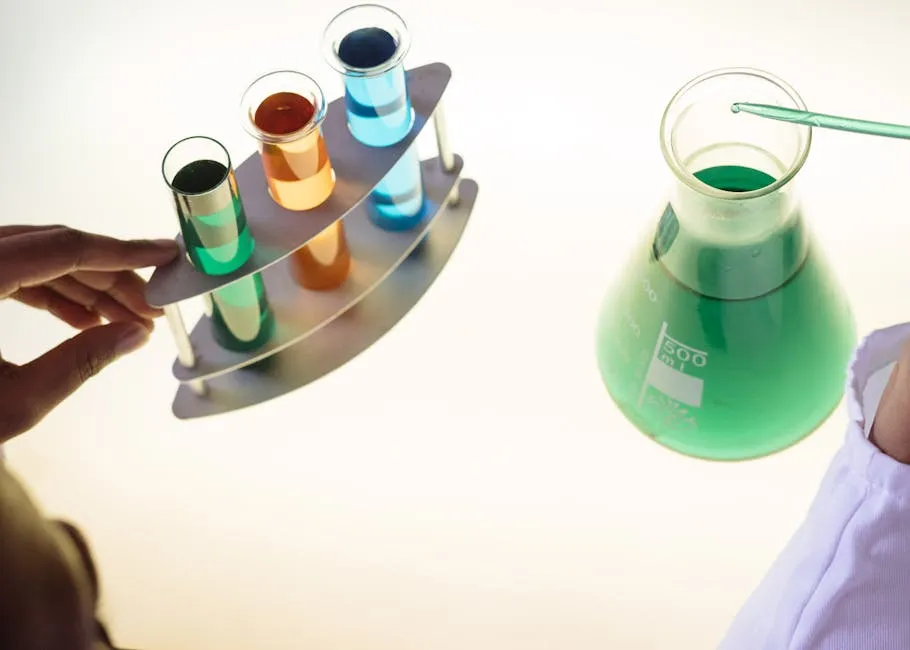
Analyzing and Interpreting RGA Results
Interpreting RGA results is essential for understanding reliability improvements. Start by examining key metrics, such as Mean Time Between Failures (MTBF) and failure intensity. MTBF indicates how often failures occur, while failure intensity shows the rate of failures over time.
Visualizing these results is vital. Use plots to present data clearly. For example, cumulative failure plots illustrate how many failures have occurred over time, while instantaneous MTBF plots show reliability trends at specific intervals. These visuals help stakeholders grasp reliability performance quickly.
When interpreting results, look for patterns. Are improvements consistent? Is there a reduction in failure rates after corrective actions? Identifying these trends can guide future decisions and help forecast reliability.
Visualization not only aids interpretation but also enhances communication. Engaging presentations of reliability data can foster discussions and encourage collaborative efforts in improving product reliability.
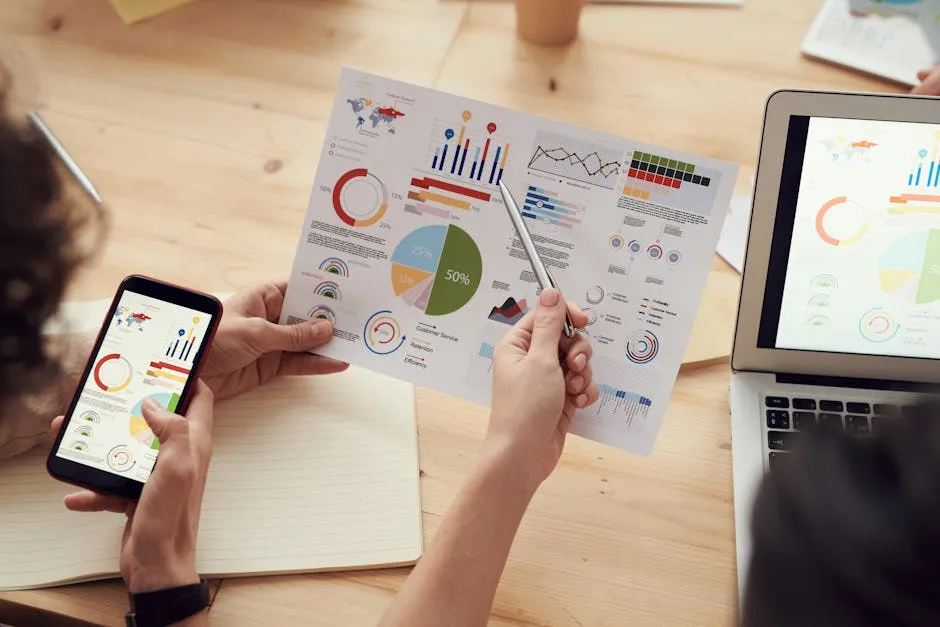
Reliability Growth Projections
Importance of Projections in RGA
Projections play a critical role in Reliability Growth Analysis (RGA). They help forecast reliability and inform future improvement plans. By analyzing past performance, organizations can predict how reliability may evolve as changes are implemented.
Growth potential is a key concept in RGA projections. It assesses how much reliability can be improved based on historical data. This assessment allows teams to set realistic goals and expectations for product reliability.
Effective projections support strategic decision-making. By understanding potential reliability trajectories, organizations can allocate resources wisely. They can prioritize areas needing improvement and adjust testing strategies to meet reliability targets.
Ultimately, these projections help organizations enhance overall product reliability while managing risks associated with product failures.
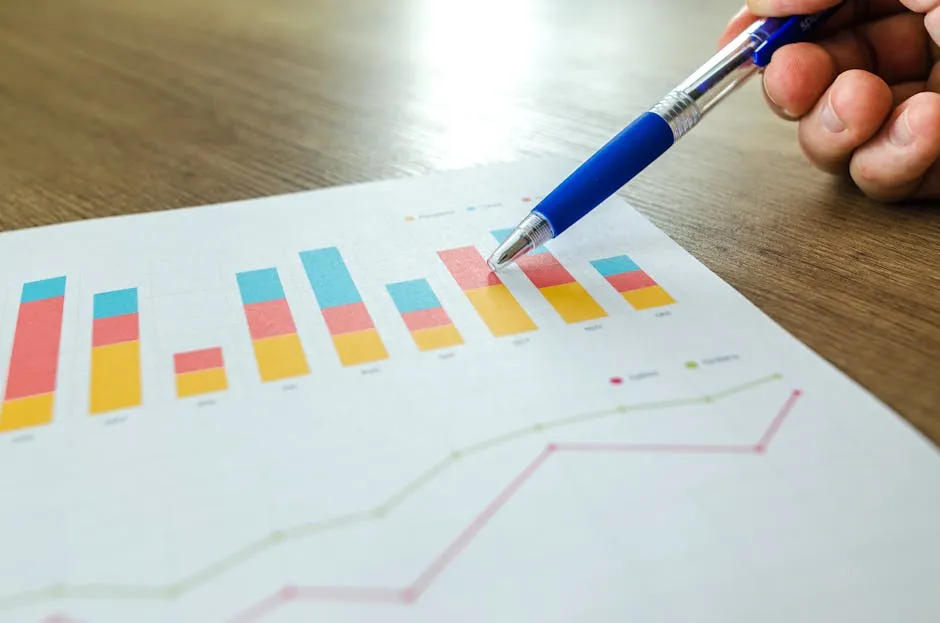
Tools and Software for RGA
Several software tools are available for conducting Reliability Growth Analysis (RGA). Popular options include ReliaSoft and Weibull++. These platforms facilitate data analysis and visualization, making the RGA process more efficient.
Key features of these tools include data entry spreadsheets that support various data types. They also offer advanced modeling capabilities, such as the Crow-AMSAA model, for analyzing failure data effectively.
Additionally, these tools provide graphical outputs, helping users visualize reliability trends clearly. Users can generate plots that show cumulative failures or MTBF over time, enhancing the understanding of reliability improvements.
Using software for RGA streamlines analysis and reporting. Automated reporting features allow teams to share insights quickly with stakeholders. This can promote collaborative efforts to enhance product reliability and inform future development strategies.

Conclusion
Reliability Growth Analysis is essential for improving product reliability. It helps organizations identify weaknesses and implement corrective actions effectively. By adopting RGA, companies can enhance their reliability management strategies, leading to increased customer satisfaction and better operational performance. Embracing RGA not only strengthens product quality but also fosters a culture of continuous improvement. Organizations should consider integrating RGA into their processes for optimal results.
For those curious about diving even deeper into data science, I suggest Data Science for Dummies. It’s a great starting point for anyone looking to build a solid foundation in data science!
Please let us know what you think about our content by leaving a comment down below!
Thank you for reading till here 🙂
All images from Pexels