Introduction
Statistical analysis plays a crucial role in research and data interpretation. It provides the framework to draw meaningful conclusions from collected data. However, statistical errors frequently occur, affecting research outcomes. These mistakes can lead to misguided conclusions, influencing decisions and policies. This article aims to educate you on common statistical analysis errors and how to avoid them effectively.
To deepen your understanding of statistical concepts, consider diving into “The Art of Statistics: Learning from Data” by David Spiegelhalter. This book provides a solid foundation for anyone looking to make sense of data in a humorous and engaging way.
Summary and Overview
Statistical errors are discrepancies that occur during data analysis. They can undermine the reliability of research findings. Understanding these errors is key to improving research quality. There are two primary types of statistical errors: sampling errors and non-sampling errors. Sampling errors arise from using a sample instead of the entire population. Non-sampling errors, on the other hand, stem from data collection processes and analysis methods.
Awareness of these errors is essential for researchers. Proper statistical practices can significantly enhance the accuracy of research results. By identifying and mitigating common mistakes, you can achieve more reliable outcomes in your studies. For those who want to delve deeper into statistical methods, “Statistics for Data Science” by James D. Miller is a great resource.
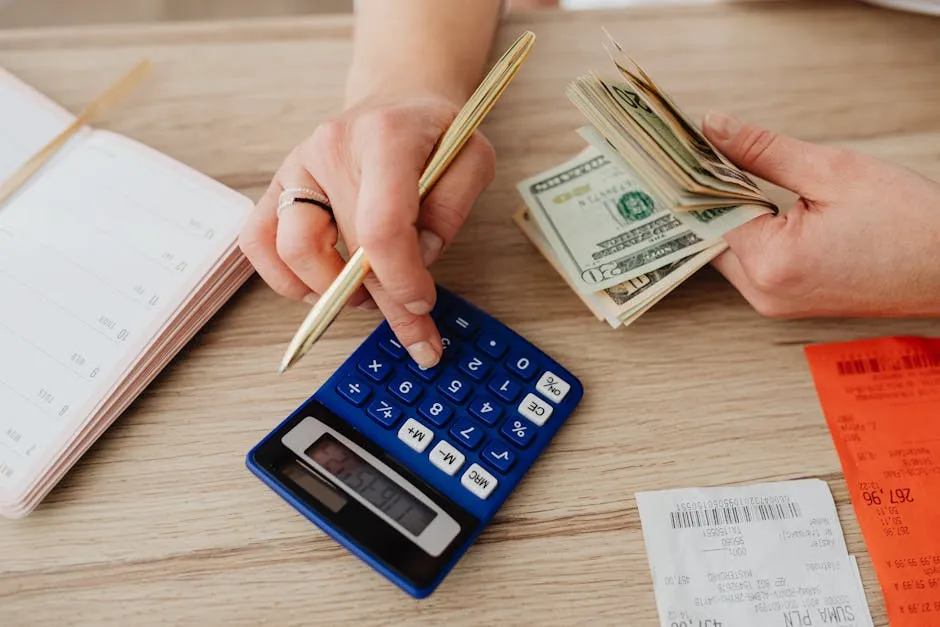
Types of Statistical Errors
Sampling Errors
Sampling errors occur when a sample doesn’t accurately represent the entire population. Various factors contribute to these errors, including sample size and selection methods. For example, if a survey samples only one demographic, it may not reflect the broader group accurately. This leads to biased results.
Using non-representative samples can have serious implications. Decisions based on skewed data can misguide policies and strategies. This is especially true in fields like healthcare or marketing, where understanding the target audience is crucial. If you’re looking for a solid foundation in statistics, consider reading “Practical Statistics for Data Scientists” by Peter Bruce and Andrew Bruce.
Research shows that increasing sample size reduces the risk of sampling errors. A larger sample can better capture variability, providing a more accurate estimate. For instance, studies indicate that sample sizes above 30 yield more reliable results.
Evaluate your sampling methods regularly. Are they inclusive and random? Ensuring representativeness can significantly enhance the quality of your findings. If you’re feeling overwhelmed with data analysis, a Statistical Analysis Software (SAS) might just be the tool you need!
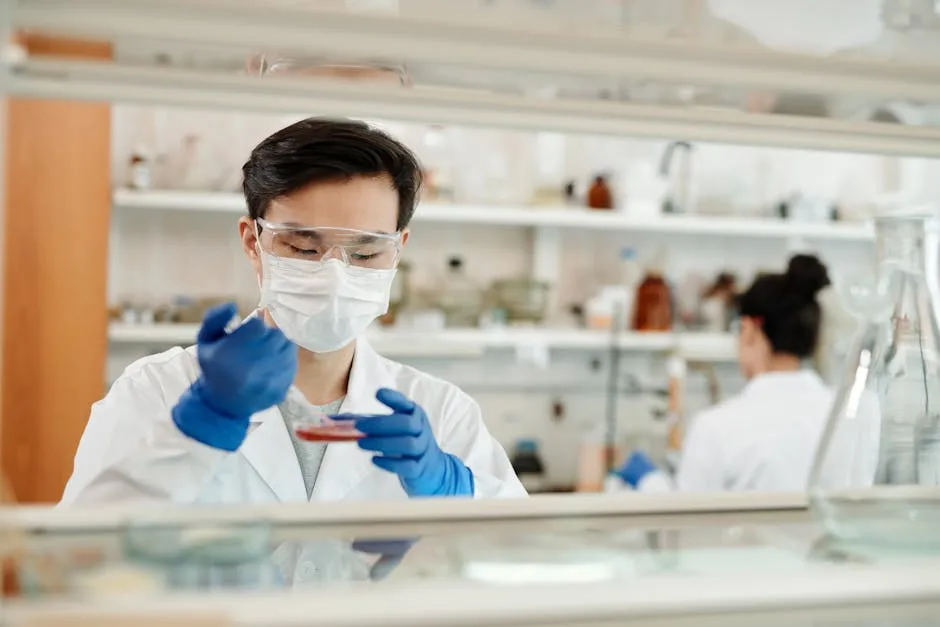
Non-Sampling Errors
Non-sampling errors arise from factors unrelated to sample selection. They can distort results and are often harder to identify. Common types include coverage error, non-response error, measurement error, and processing error.
Coverage Error
Coverage error occurs when some members of the population are excluded or duplicated in the sample. This can happen due to faulty sampling frames. The impact on data integrity can be significant. If certain groups are underrepresented, the findings could be misleading.
Non-Response Error
Non-response error results from failing to collect data from selected participants. This can lead to incomplete information. It raises concerns about the representativeness of the sample. Non-response can distort outcomes, especially if the non-respondents share common traits.
Measurement Error
Measurement errors arise from inaccuracies in data collection methods. These may include poorly designed surveys or faulty instruments. Such errors can skew results, leading to incorrect conclusions about relationships or effects. To improve your measurement accuracy, consider using a Graphing Calculator to assist with calculations!
Processing Error
Processing errors occur during data handling stages like entry, coding, or analysis. Mistakes in these stages can introduce significant inaccuracies. For example, data might be misclassified, leading to erroneous interpretations.
Non-sampling errors are prevalent. Research estimates that about 20% of studies experience such errors. Implementing robust data collection methods can help mitigate these risks. Use tools and software that ensure accuracy during data handling. If you’re looking to enhance your skills in data visualization, consider checking out Data Visualization Tools like Tableau.
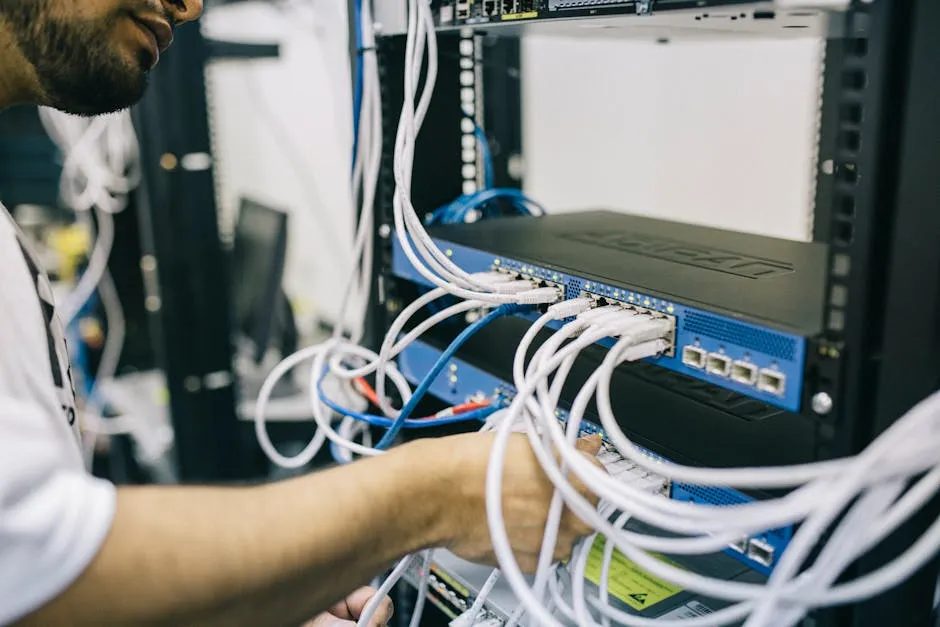
By understanding these errors, you can take proactive steps to enhance data integrity. Regularly review your processes and consider adopting new technologies to minimize errors in your research.
Common Statistical Mistakes
Type I and Type II Errors
Type I errors occur when researchers reject a true null hypothesis. This is often termed a false positive. For example, a study might conclude a new drug is effective when it isn’t. The significance level, usually set at 0.05, controls the probability of making this error.
On the other hand, Type II errors happen when researchers fail to reject a false null hypothesis. This is known as a false negative. For example, a test might show no effect of a treatment that actually works. Power analysis helps determine the likelihood of avoiding this error. Understanding post hoc statistical power is essential in this context.
Understanding statistical power can help researchers avoid Type II errors. post hoc statistical power
Both types of errors impact hypothesis testing significantly. Understanding these errors allows researchers to interpret results accurately. Studies with low power often yield higher Type II errors. This can lead to missed opportunities for discovery and innovation. A great resource to understand this better is “Naked Statistics” by Charles Wheelan.
For instance, a clinical trial that doesn’t enroll enough participants may overlook treatment effectiveness. Checking statistical power before conducting tests is crucial. Ensure your study design minimizes these risks for valid results.
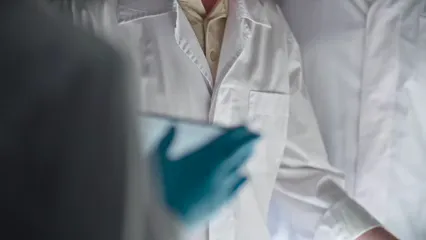
Misinterpretation of Results
Misinterpretation of statistical findings is common. Researchers might mistake correlation for causation. For example, a study might find a correlation between ice cream sales and drowning incidents. However, this does not mean ice cream causes drownings.
Another issue arises when researchers overemphasize p-values. A p-value below 0.05 doesn’t guarantee a meaningful effect. It merely indicates statistical significance. Misleading conclusions can occur when results are reported without context. To help navigate through these complexities, consider reading “The Signal and the Noise” by Nate Silver.
Case studies reveal how misinterpretation can harm research credibility. One study incorrectly concluded a medication was ineffective based solely on non-significant results. This led to unnecessary patient suffering.
Encouraging peer review can catch these misinterpretations. Having others review findings adds a layer of scrutiny. This helps ensure accuracy in data interpretation and reporting. Always consider the broader context of your results to avoid misleading conclusions.
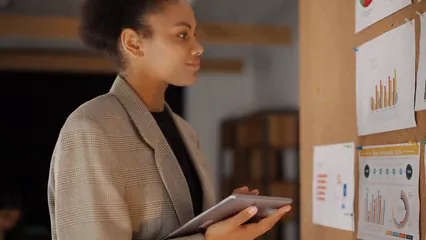
Over-Reliance on P-Values
Many researchers lean heavily on p-values in statistical analysis. However, this focus can lead to oversights. P-values only indicate whether results are statistically significant. They do not reflect practical importance or the size of an effect. This limitation can mislead interpretations.
Effect sizes and confidence intervals are crucial. Effect sizes quantify the strength of a relationship or difference. They provide context that p-values alone cannot. Confidence intervals offer a range of values where the true effect likely lies. This helps researchers gauge precision in their estimates. If you’re looking for a comprehensive understanding of these concepts, don’t miss “Statistics Done Wrong” by Alex Reinhart.
Misuse of p-values is prevalent. Studies show that about 70% of research articles misinterpret them. Such misuse can distort findings and affect decision-making. For instance, a p-value below 0.05 does not guarantee a meaningful effect. It merely suggests statistical significance, which is often misinterpreted.
Training on proper statistical reporting is vital. Researchers should understand the limitations of p-values. By emphasizing effect sizes and confidence intervals, we can foster better research practices. This shift will lead to more accurate conclusions and improved scientific integrity.
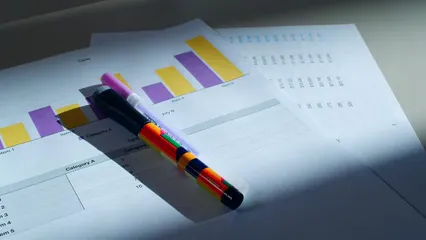
Best Practices for Statistical Analysis
Proper Study Design
A well-structured study design is essential. It minimizes errors and enhances the reliability of results. Researchers must carefully plan their studies to address specific hypotheses. This includes defining clear objectives and determining appropriate sample sizes.
Pre-registration is a key practice in research. This involves publicly documenting study designs and hypotheses before data collection. Studies with pre-registered designs report higher success rates. Research shows that about 80% of pre-registered studies yield conclusive results compared to only 50% of non-registered ones.
Learning about effective study design can improve research outcomes. Resources such as workshops, online courses, and textbooks provide valuable insights. For an excellent textbook, check out “The Lady Tasting Tea” by David Salsburg. Researchers should seek out these materials to elevate their understanding. By doing so, they can enhance the quality of their studies and contribute to more trustworthy research findings.
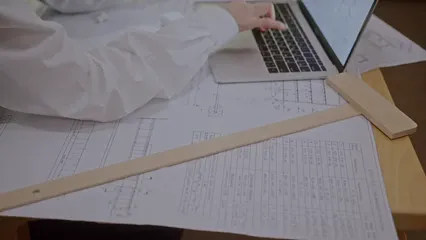
Use of Statistical Software
Using statistical software can transform your data analysis experience. These tools make complex calculations easier and faster. They also help visualize data trends and patterns effectively. However, relying solely on software can lead to misunderstandings and errors.
One common pitfall is assuming the software is infallible. Always double-check your inputs and outputs. Misinterpreting results is another frequent mistake. For instance, a software might provide a statistically significant result, but the practical significance might be negligible.
To minimize errors, familiarize yourself with the software’s functionalities. Attend training sessions or explore online tutorials. This knowledge will enhance your analytical skills and ensure accurate results. If you’re interested in learning R programming for data science, I highly recommend “R Programming for Data Science”.
Research indicates that accuracy varies across different software. For instance, studies show that popular packages like R and SPSS yield reliable outcomes in many scenarios. However, errors can still arise from user input or model assumptions.
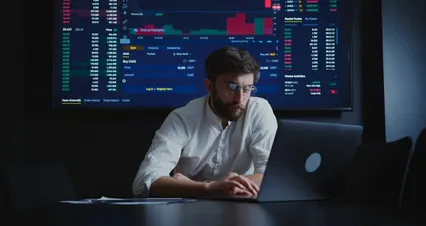
When selecting statistical software, consider your specific needs. Options like R, Python, and SAS are excellent for in-depth analysis. If you prefer user-friendly interfaces, consider software like SPSS or Excel. Each has its strengths, so choose wisely based on your project requirements.
In summary, while statistical software can enhance your data analysis, understanding its limitations is crucial. Invest time in learning and avoid common pitfalls to achieve reliable results. And if you’re looking to manage your data efficiently, a Portable External Hard Drive can be a lifesaver!

FAQs
What are the two main types of statistical errors?
Statistical errors fall into two categories: sampling errors and non-sampling errors. Sampling errors occur due to the sample’s representation of the population, while non-sampling errors arise from data collection issues.
How can I reduce the risk of Type I and Type II errors in my research?
To minimize Type I and Type II errors, ensure an adequate sample size and apply proper hypothesis testing methods. This approach helps maintain the integrity of your research findings.
Why is understanding statistical errors important for researchers?
Awareness of statistical errors is crucial for maintaining research reliability. Errors can undermine the validity of findings, leading to incorrect conclusions and decisions.
What common mistakes do researchers make in statistical analysis?
Researchers often misinterpret results, over-rely on p-values, or fail to recognize sampling biases. These mistakes can skew data interpretations and impact overall research quality.
What resources are available for improving statistical analysis skills?
Numerous resources exist, including books, online courses, and workshops focusing on statistical methods. Engaging with these materials can significantly enhance your analytical capabilities.
Please let us know what you think about our content by leaving a comment down below!
Thank you for reading till here 🙂
All images from Pexels