Introduction
Statistical analysis involves collecting and evaluating data to uncover insights. It’s crucial in fields like healthcare, business, and social sciences. Understanding statistical methods helps researchers achieve better results. This post will explore various statistical analysis methods, enhancing your research skills.
If you’re looking to deepen your understanding of statistical methods, consider picking up Statistics For Dummies. It’s an approachable guide that breaks down complex concepts into bite-sized pieces, making it perfect for anyone feeling overwhelmed by numbers!
Summary and Overview
Statistical analysis is a systematic approach to understanding data. It applies to various disciplines, from social sciences to business analytics. Selecting the right statistical method is essential for accurate results. This choice depends on data types and research goals.
Two main types of statistical analysis exist: descriptive and inferential. Descriptive statistics summarize data, while inferential statistics draw conclusions from samples. In this post, you can expect clear explanations of key statistical methods and their applications. For a deeper dive into descriptive statistics in manufacturing plant, check out our guide.
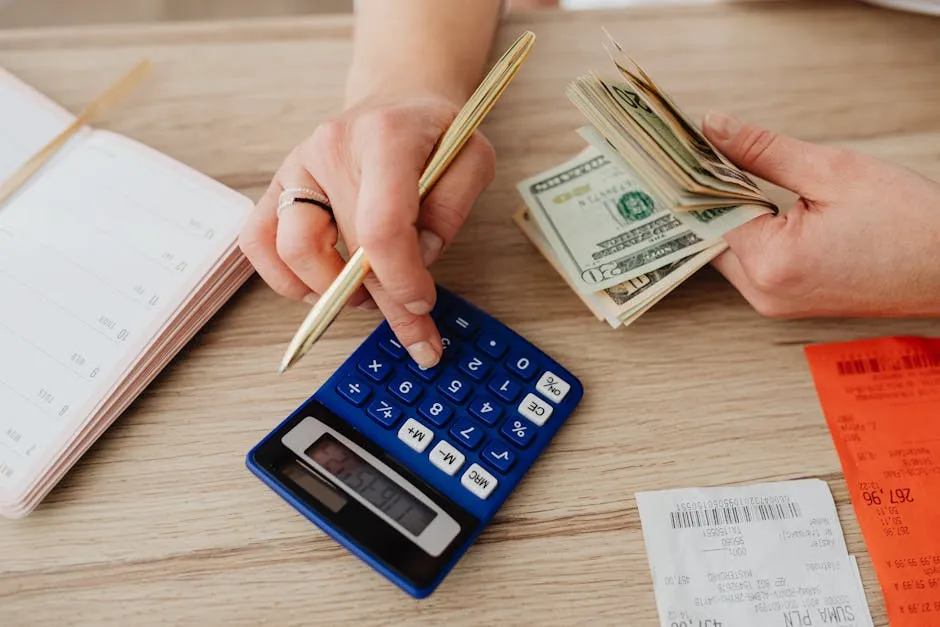
Understanding descriptive statistics is essential for summarizing data effectively. Learn more about descriptive statistics.
What is Statistical Analysis?
Statistical analysis is the process of interpreting data to understand patterns and relationships. It serves as a vital tool for decision-making across different sectors. By applying statistical techniques, researchers can derive meaningful conclusions from raw data.
If you’re new to statistical analysis, you might want to check out The Art of Statistics: Learning from Data. This book offers a fantastic introduction to understanding data and making sound conclusions.
There are two primary categories of statistics: descriptive and inferential. Descriptive statistics provide a summary of data through measures like mean and standard deviation. Conversely, inferential statistics use sample data to make predictions or test hypotheses about a larger population. For a comprehensive understanding of the problem with inferential statistics, refer to our detailed article.
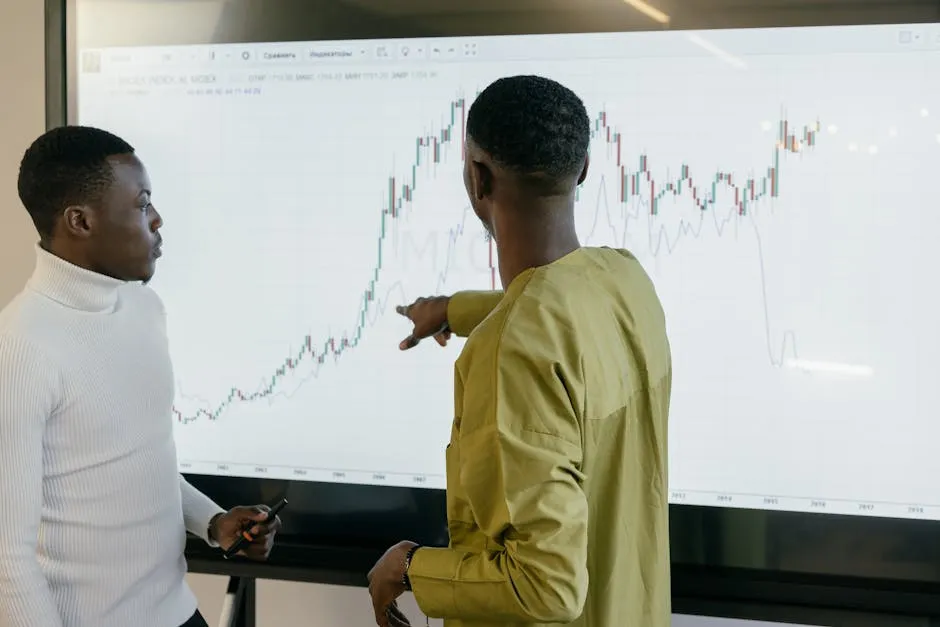
Inferential statistics play a crucial role in making predictions based on sample data. Explore more about inferential statistics.
Types of Statistical Analysis
Descriptive Statistics
Descriptive statistics summarize and simplify data. Their main purpose is to provide clear insights into large datasets. This method helps researchers understand the essential features of data without making predictions or inferences.
Common measures of central tendency include the mean, median, and mode. The mean is the average value, calculated by adding all numbers and dividing by the count. For example, if test scores are 75, 80, and 85, the mean is 80. The median is the middle value when numbers are sorted. In the same example, the median score is also 80. The mode represents the most frequently occurring value. If the scores were 75, 80, 80, and 85, the mode would be 80.
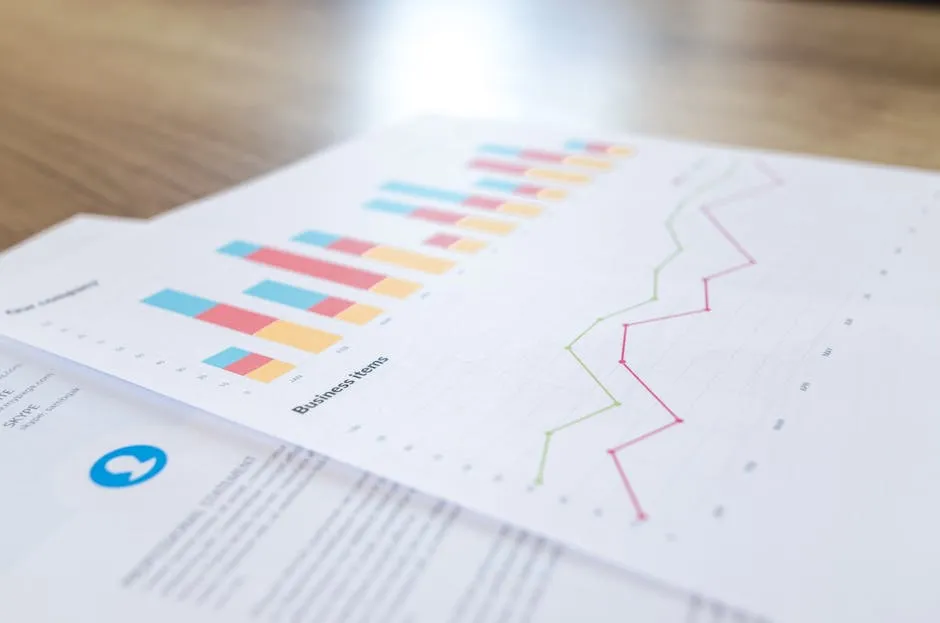
Speaking of statistics, if you want a deeper understanding, Naked Statistics: Stripping the Dread from the Data is a fantastic read that makes statistical concepts approachable and fun!
Measures of dispersion, like standard deviation, reveal the variability of data points. A low standard deviation indicates that data points cluster close to the mean, while a high standard deviation shows a wider spread. For instance, if the scores were 75, 80, and 95, the standard deviation would be higher than with scores of 75, 80, and 81. Tools like Excel or SPSS can help you efficiently calculate these statistics.
Inferential Statistics
Inferential statistics allows researchers to make predictions and draw conclusions about populations based on sample data. This method is crucial for hypothesis testing and determining relationships among variables.
Key concepts include the null hypothesis and the alternative hypothesis. The null hypothesis assumes no effect or relationship exists. Conversely, the alternative hypothesis posits that a significant relationship or effect does exist. For example, researchers might test whether a new drug improves patient outcomes compared to a placebo.
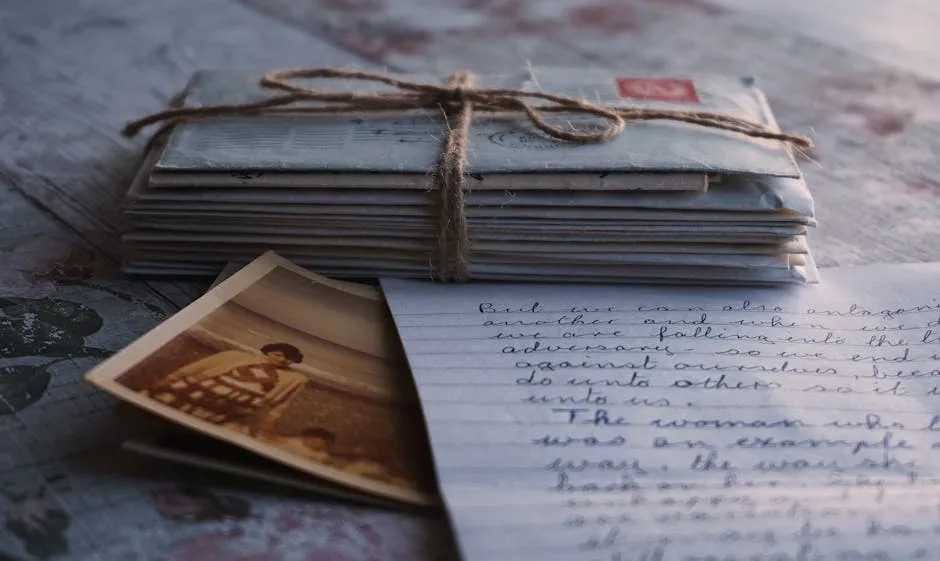
If you’re delving into the intricacies of statistical methods, check out Principles of Statistics. This book delivers foundational knowledge and practical applications that are essential for any aspiring statistician.
The p-value measures the strength of evidence against the null hypothesis. A lower p-value indicates stronger evidence that the null hypothesis should be rejected. Common tests include t-tests and ANOVA, which help compare means across different groups. In research studies, hypothesis testing can reveal whether treatment effects are statistically significant.
In your own research, consider incorporating inferential statistics to validate your findings and contribute to broader knowledge in your field.

Common Statistical Analysis Methods
1. Mean
The mean, often called the average, is a critical measure in statistics. To calculate it, add all the values in a dataset and divide by the total number of values. For example, consider the test scores: 75, 80, and 85. The mean is (75 + 80 + 85) / 3 = 80. For a comprehensive explanation of what it means for data to be identically distributed, refer to this article.
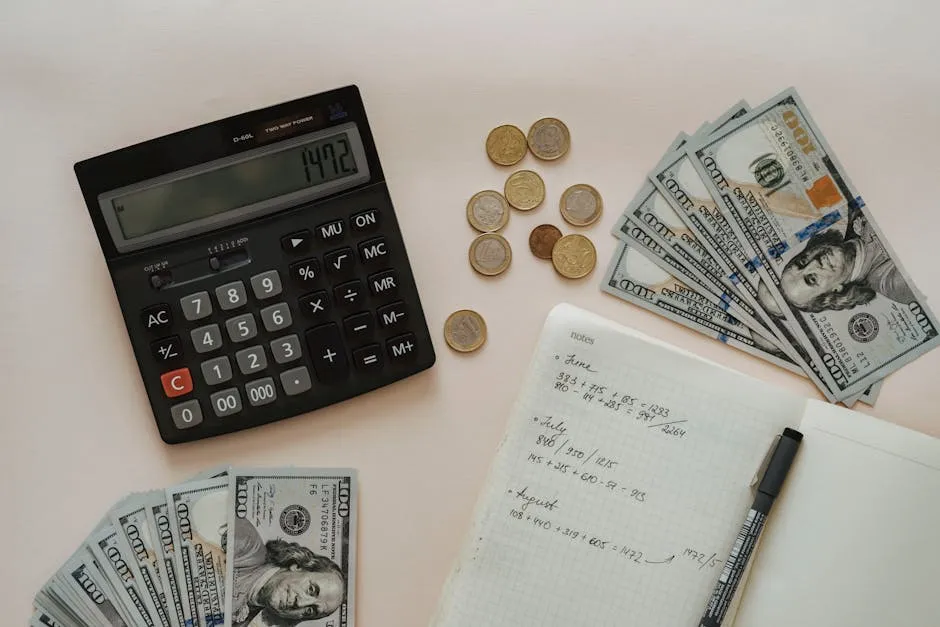
The mean is a fundamental concept in statistics that provides a central value for datasets. Learn more about identically distributed data.
2. Standard Deviation
Standard deviation measures how spread out data points are from the mean. It helps determine whether data points cluster closely or are widely dispersed. To calculate standard deviation, follow these steps: first, find the mean. Then, subtract the mean from each data point and square the result. After that, average these squared differences, and finally take the square root of this average.
If you’re looking for a user-friendly guide to understanding these concepts, grab Statistical Methods for the Social Sciences. This book provides essential tools and techniques for analyzing social data.
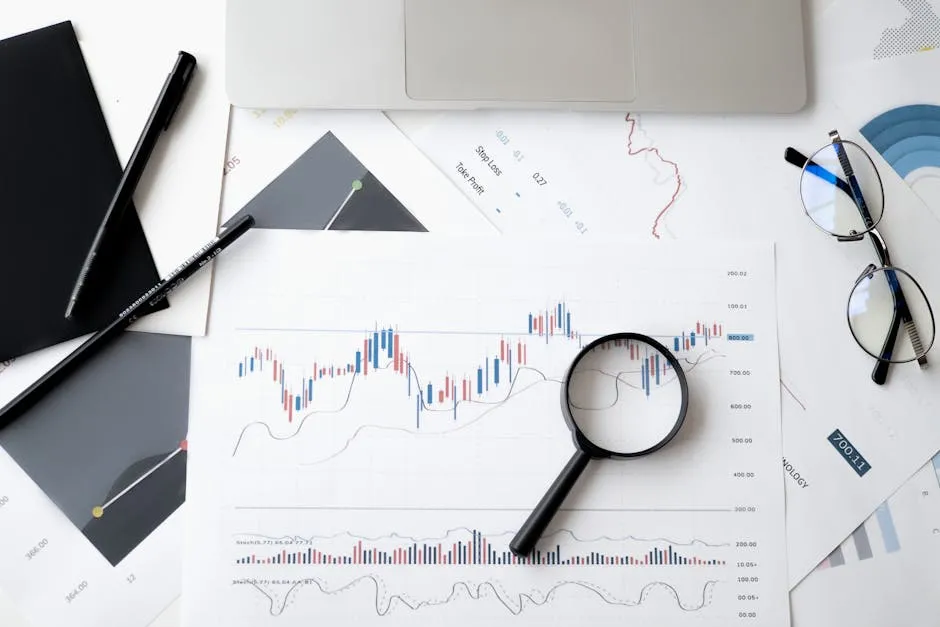
For example, with data points 2, 4, and 8, the mean is 4. The squared differences are: (2-4)^2 = 4, (4-4)^2 = 0, (8-4)^2 = 16. The variance is the average of these squared differences, which is (4 + 0 + 16)/3 ≈ 6.67. The standard deviation is then √6.67 ≈ 2.58.
Understanding standard deviation is vital in research. A low standard deviation indicates consistency, while a high standard deviation suggests variability. Analyze your data’s spread to make informed decisions based on statistical insights.
3. Regression Analysis
Regression analysis is a powerful statistical method used to understand relationships between variables. It helps predict the value of a dependent variable based on one or more independent variables. Simple regression involves one independent variable, while multiple regression includes several. For a detailed guide on regression analysis, visit this resource.

Regression analysis is essential for understanding variable relationships in data. Explore our comprehensive guide on regression analysis.
4. Hypothesis Testing
Hypothesis testing is a statistical method used to make decisions about a population based on sample data. It helps researchers determine if there’s enough evidence to support a specific claim. This method is vital in fields like medicine, psychology, and economics. For a quick reference, check out our statistics hypothesis testing cheat sheet.

Hypothesis testing is crucial for validating research claims. Refer to our cheat sheet for hypothesis testing.
5. Sample Size Determination
Determining the right sample size is crucial for accurate statistical analysis. An adequate sample size ensures that results are reliable and representative of the population. Too small a sample can lead to misleading conclusions, while too large a sample may waste resources.
To calculate sample size, consider several factors, including the expected effect size, desired confidence level, and statistical power. A common approach is using power analysis, which estimates the minimum sample size needed to detect an effect.
For example, if you’re studying the effect of a new educational program, you might calculate the necessary sample size based on prior studies. This ensures that your findings are statistically significant and can be generalized to a broader audience.
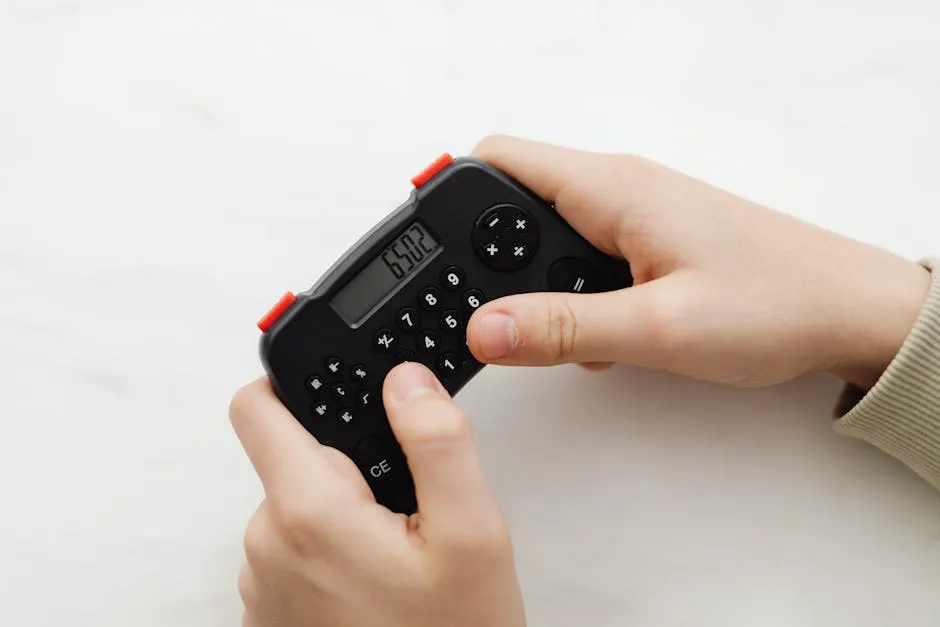
Speaking of understanding sample sizes, check out How to Measure Anything: Finding the Value of “Intangibles” in Business. This book is a classic for understanding the importance of measurement in research.
Choosing the Right Statistical Analysis Method
Selecting the right statistical analysis method can feel daunting. However, understanding your data and objectives makes this process manageable. Start by identifying the type of data you have: nominal, ordinal, or continuous. Each data type has specific statistical methods suited to it.
If you’re serious about mastering statistical methods, consider Statistical Methods in Education and Psychology. This book provides an in-depth look at the application of statistics in these fields, which can be incredibly beneficial for researchers.
Next, consider your research question. Are you comparing groups, examining relationships, or predicting outcomes? This clarity will guide you toward appropriate methods. For instance, use t-tests for comparing means or regression analysis for exploring relationships between variables.
Sample size is another critical factor. Larger samples often yield more reliable results, but consider practicality and resource availability. A flowchart can help visualize your decision-making process, making it easier to match methods to your research goals.
Common pitfalls include misinterpreting data types or using inappropriate tests. Be cautious with assumptions underlying statistical tests, as incorrect assumptions can lead to invalid results. Encourage a reflective approach; consider your data types and choose methods that align with your research objectives to enhance the quality of your analysis.
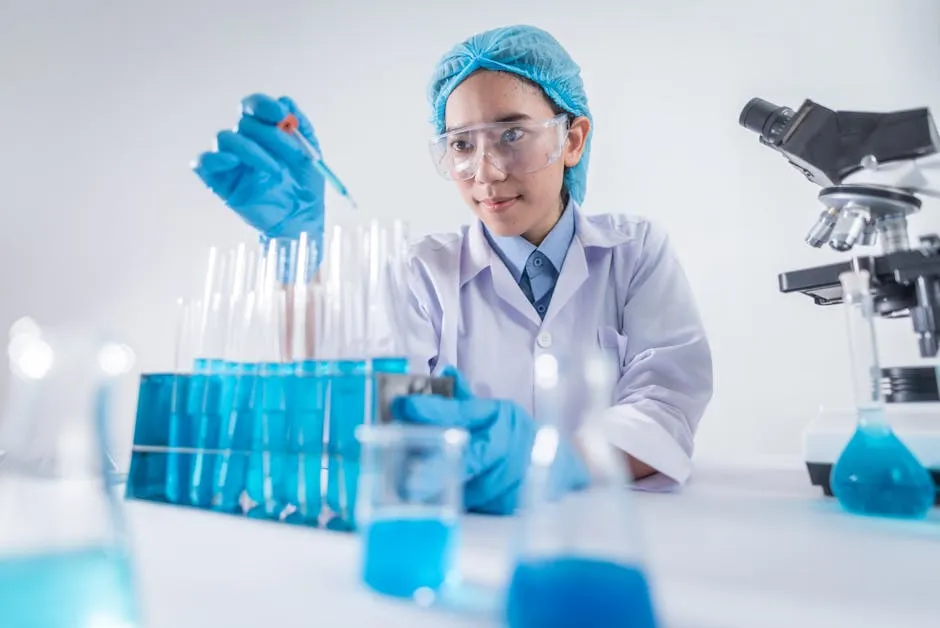
Conclusion
Statistical analysis methods are essential for research in any field. They help us make sense of complex data. Choosing the right method ensures accurate and reliable results. A poor choice can lead to misleading conclusions. Therefore, understanding these methods is vital for effective decision-making. I encourage you to apply these techniques in your work. Whether you’re in business, healthcare, or social sciences, these methods can enhance your insights and outcomes.
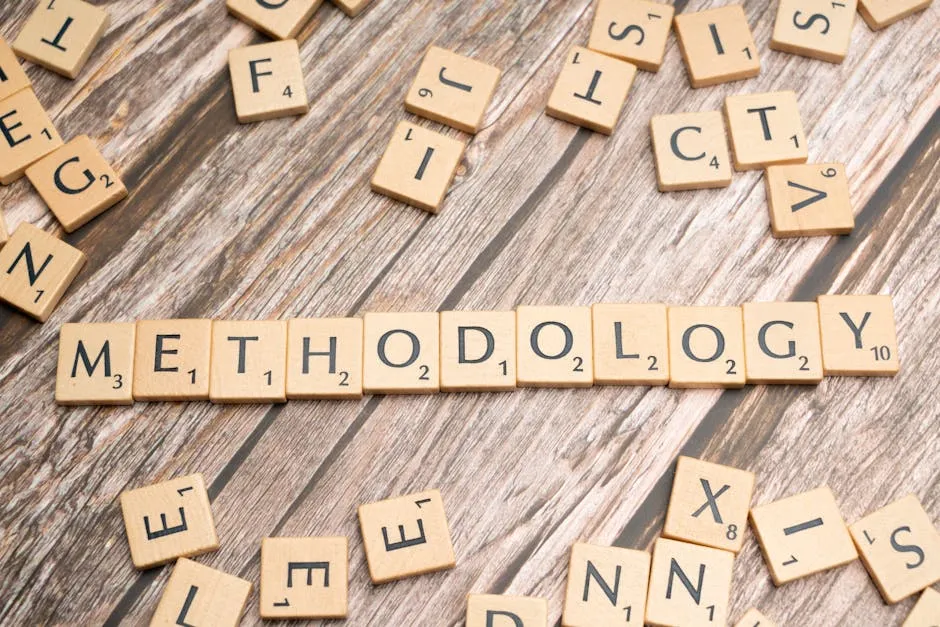
And for those who want to dive deeper into data science, Data Science for Business is a fantastic resource that bridges the gap between data analysis and business strategy.
FAQs
What are the most common statistical analysis methods?
Common statistical methods include mean, standard deviation, regression, and hypothesis testing. Each method serves a unique purpose in data analysis. The mean calculates the average, while standard deviation measures data spread. Regression helps explore relationships between variables. Hypothesis testing evaluates claims about populations.
How do I choose the right statistical method for my data?
Selecting the right statistical method depends on your data type and research goals. Consider whether your data is nominal, ordinal, or continuous. Also, think about your research question—are you comparing groups or predicting outcomes? Understanding these factors will guide your method selection.
What is the difference between descriptive and inferential statistics?
Descriptive statistics summarize and describe data from a sample. Examples include mean, median, and mode. On the other hand, inferential statistics use sample data to make conclusions about a population. This involves hypothesis testing and confidence intervals.
Why is sample size important in statistical analysis?
Sample size significantly impacts research outcomes. A larger sample size leads to more reliable estimates and greater statistical power. Conversely, a small sample size may produce unreliable results. Thus, determining an adequate sample size is crucial for valid conclusions.
How can I improve my statistical analysis skills?
To enhance your statistical analysis skills, consider online courses and workshops. Books and tutorials on statistics are also beneficial. Practice using statistical software like R or SPSS. Regularly applying these concepts in real-world scenarios will deepen your understanding.
Please let us know what you think about our content by leaving a comment down below!
Thank you for reading till here 🙂
All images from Pexels