Introduction
Descriptive statistics are vital in data analysis. They help summarize and interpret large datasets effectively. By providing clear insights, they enable informed decision-making and deeper understanding of data.
Summary and Overview
Descriptive statistics summarize key features of a dataset. They provide a snapshot of data, making complex information accessible. Unlike inferential statistics, which make predictions about a population, descriptive statistics focus solely on the data at hand.
Key components include measures of central tendency, such as mean, median, and mode, which identify the center of the data. Variability measures, like range and standard deviation, show how spread out the data is. Descriptive statistics find applications in fields like business, healthcare, and social sciences. Visualizations, such as graphs and charts, enhance understanding and reveal patterns in the data.
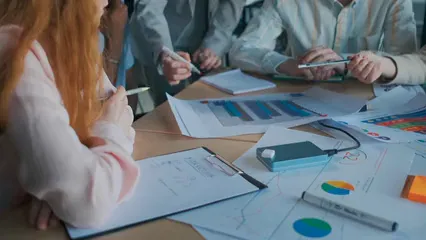
These examples illustrate the importance of descriptive statistics in reporting and decision-making. Think about how you can apply these concepts in your own field. How might understanding data help you make more informed decisions?
Descriptive statistics are crucial in various fields, including education. my education data
Conclusion
Descriptive statistics play a vital role in data analysis. They simplify complex datasets, making the information more digestible. By summarizing key features, these statistics help you interpret data effectively. This understanding is crucial for making informed decisions, whether in business, healthcare, or research.
As you continue your learning journey, apply these concepts in your work. Practicing descriptive statistics can enhance your analytical skills and boost your confidence. Remember, these statistics form the foundation for more advanced statistical analysis. Embrace this knowledge and watch your understanding of data grow! If you’re interested in enhancing your data skills, consider a Python for Data Analysis course to dive into programming for data science!
FAQs
What Are Descriptive Statistics?
Descriptive statistics summarize and describe the main features of a dataset. They include measures of central tendency, like mean, median, and mode, as well as measures of variability, such as range and standard deviation. These statistics help you understand the characteristics of your data quickly.
How Are Descriptive Statistics Used?
Descriptive statistics are widely used across various fields. In business, they analyze customer preferences and sales trends. In healthcare, they summarize patient data, like average recovery times. Social scientists use them to interpret survey results. Their applications are vast, helping professionals make informed decisions.
What Is the Difference Between Descriptive and Inferential Statistics?
Descriptive statistics summarize the characteristics of a dataset. They do not make predictions or inferences about a larger population. Inferential statistics, on the other hand, use sample data to make generalizations or predictions about a population. This distinction is crucial in statistical analysis.
Can Descriptive Statistics Help in Making Predictions?
While descriptive statistics provide valuable insights, they have limitations in making predictions. They focus on summarizing past data rather than forecasting future outcomes. For predictions, inferential statistics are more appropriate, as they analyze relationships and trends within the data.
How Do You Calculate Descriptive Statistics?
Calculating descriptive statistics involves a few key steps. To find the mean, sum all values and divide by the count. The median is identified by ordering values and finding the middle point. The mode is the most frequently occurring value. Variability measures like range and standard deviation quantify data spread, providing a comprehensive overview of the dataset.
Please let us know what you think about our content by leaving a comment down below!
Thank you for reading till here 🙂
All images from Pexels
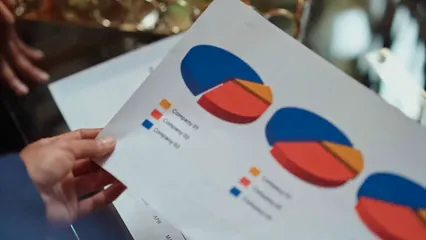
Finance is another domain where descriptive statistics shine. An investment firm may evaluate the average return on portfolios. This helps clients understand their potential earnings.
Consider a case study in education. A school district might analyze student test scores. Descriptive statistics help identify performance gaps among different groups. This informs targeted interventions. If you’re looking for a comprehensive guide on statistics, check out Statistics for Dummies. It’s a beginner-friendly resource that covers the essentials!
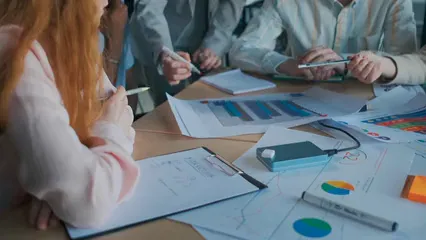
These examples illustrate the importance of descriptive statistics in reporting and decision-making. Think about how you can apply these concepts in your own field. How might understanding data help you make more informed decisions?
Descriptive statistics are crucial in various fields, including education. my education data
Conclusion
Descriptive statistics play a vital role in data analysis. They simplify complex datasets, making the information more digestible. By summarizing key features, these statistics help you interpret data effectively. This understanding is crucial for making informed decisions, whether in business, healthcare, or research.
As you continue your learning journey, apply these concepts in your work. Practicing descriptive statistics can enhance your analytical skills and boost your confidence. Remember, these statistics form the foundation for more advanced statistical analysis. Embrace this knowledge and watch your understanding of data grow! If you’re interested in enhancing your data skills, consider a Python for Data Analysis course to dive into programming for data science!
FAQs
Please let us know what you think about our content by leaving a comment down below!
Thank you for reading till here 🙂
All images from Pexels
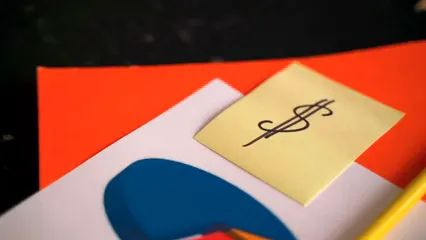
Effective data visualization techniques are essential for clear insights. visualizing data with ap statistics formula sheet techniques
Descriptive Statistics in Practice
Real-World Applications
Descriptive statistics apply across various fields, providing valuable insights. In healthcare, they summarize patient data. For instance, average recovery times can guide treatment protocols.
In marketing, businesses use descriptive statistics to analyze customer preferences. A company might track average purchase amounts to identify spending trends. This helps tailor marketing strategies to specific demographics. To boost your marketing analysis, consider picking up Data Science for Business: What You Need to Know about Data Mining and Data-Analytic Thinking. It’s a great resource to understand the intersection of data science and business!
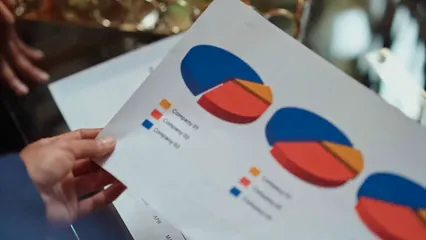
Finance is another domain where descriptive statistics shine. An investment firm may evaluate the average return on portfolios. This helps clients understand their potential earnings.
Consider a case study in education. A school district might analyze student test scores. Descriptive statistics help identify performance gaps among different groups. This informs targeted interventions. If you’re looking for a comprehensive guide on statistics, check out Statistics for Dummies. It’s a beginner-friendly resource that covers the essentials!
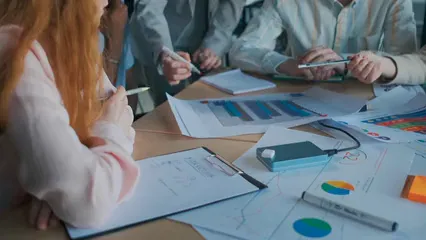
These examples illustrate the importance of descriptive statistics in reporting and decision-making. Think about how you can apply these concepts in your own field. How might understanding data help you make more informed decisions?
Descriptive statistics are crucial in various fields, including education. my education data
Conclusion
Descriptive statistics play a vital role in data analysis. They simplify complex datasets, making the information more digestible. By summarizing key features, these statistics help you interpret data effectively. This understanding is crucial for making informed decisions, whether in business, healthcare, or research.
As you continue your learning journey, apply these concepts in your work. Practicing descriptive statistics can enhance your analytical skills and boost your confidence. Remember, these statistics form the foundation for more advanced statistical analysis. Embrace this knowledge and watch your understanding of data grow! If you’re interested in enhancing your data skills, consider a Python for Data Analysis course to dive into programming for data science!
FAQs
Please let us know what you think about our content by leaving a comment down below!
Thank you for reading till here 🙂
All images from Pexels
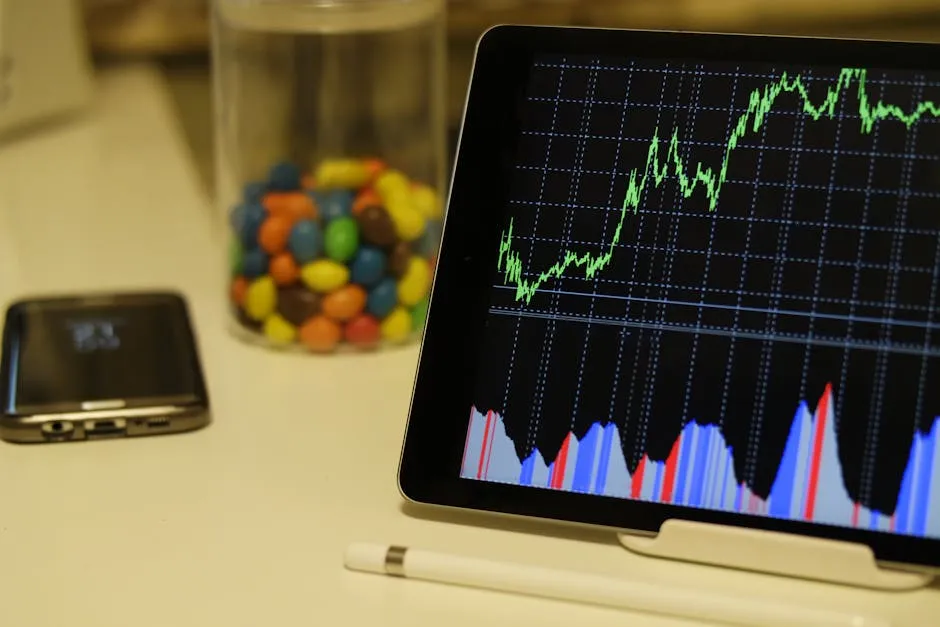
Scatter plots are fantastic for examining relationships between two variables. They can visually display correlations, making it easy to spot trends.
The benefits of graphical representation are numerous. They simplify data interpretation and enhance communication. Visuals can convey insights that raw data often obscures. For example, a well-designed chart can reveal trends that numbers alone might hide.
So, consider using visualization tools in your analysis. They can elevate your understanding and presentation of data. Embrace these techniques to enhance your descriptive statistics journey! If you’re keen on mastering visualization, you might want to explore Tableau Desktop, a powerful tool for creating stunning visuals!
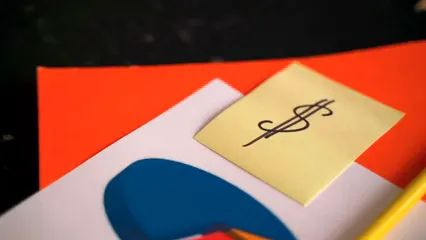
Effective data visualization techniques are essential for clear insights. visualizing data with ap statistics formula sheet techniques
Descriptive Statistics in Practice
Real-World Applications
Descriptive statistics apply across various fields, providing valuable insights. In healthcare, they summarize patient data. For instance, average recovery times can guide treatment protocols.
In marketing, businesses use descriptive statistics to analyze customer preferences. A company might track average purchase amounts to identify spending trends. This helps tailor marketing strategies to specific demographics. To boost your marketing analysis, consider picking up Data Science for Business: What You Need to Know about Data Mining and Data-Analytic Thinking. It’s a great resource to understand the intersection of data science and business!
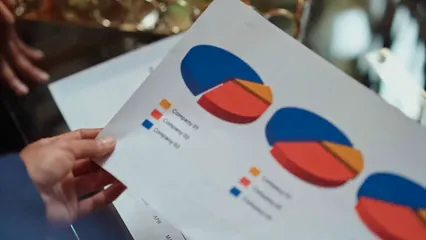
Finance is another domain where descriptive statistics shine. An investment firm may evaluate the average return on portfolios. This helps clients understand their potential earnings.
Consider a case study in education. A school district might analyze student test scores. Descriptive statistics help identify performance gaps among different groups. This informs targeted interventions. If you’re looking for a comprehensive guide on statistics, check out Statistics for Dummies. It’s a beginner-friendly resource that covers the essentials!
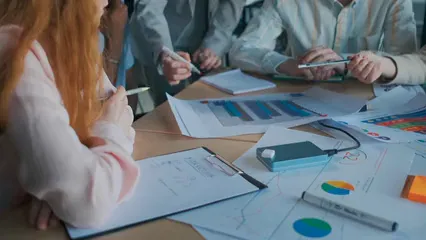
These examples illustrate the importance of descriptive statistics in reporting and decision-making. Think about how you can apply these concepts in your own field. How might understanding data help you make more informed decisions?
Descriptive statistics are crucial in various fields, including education. my education data
Conclusion
Descriptive statistics play a vital role in data analysis. They simplify complex datasets, making the information more digestible. By summarizing key features, these statistics help you interpret data effectively. This understanding is crucial for making informed decisions, whether in business, healthcare, or research.
As you continue your learning journey, apply these concepts in your work. Practicing descriptive statistics can enhance your analytical skills and boost your confidence. Remember, these statistics form the foundation for more advanced statistical analysis. Embrace this knowledge and watch your understanding of data grow! If you’re interested in enhancing your data skills, consider a Python for Data Analysis course to dive into programming for data science!
FAQs
Please let us know what you think about our content by leaving a comment down below!
Thank you for reading till here 🙂
All images from Pexels
Creating and interpreting these tables helps summarize data effectively. Try making your own frequency distribution table with your data. It’s a great way to see patterns and distributions clearly!
Visualizing Data
Importance of Data Visualization
Visual aids are essential for grasping descriptive statistics. They transform complex numbers into digestible images. This makes data easier to understand and analyze.
Common visualizations include histograms, box plots, and scatter plots.
Histograms display the frequency distribution of numerical data. They help identify patterns and trends within the dataset.
Box plots summarize data through quartiles, highlighting key stats like median and outliers. They show how data points spread and where they cluster. If you’re interested in creating box plots, a Box Plot Chart Maker might be just what you need!
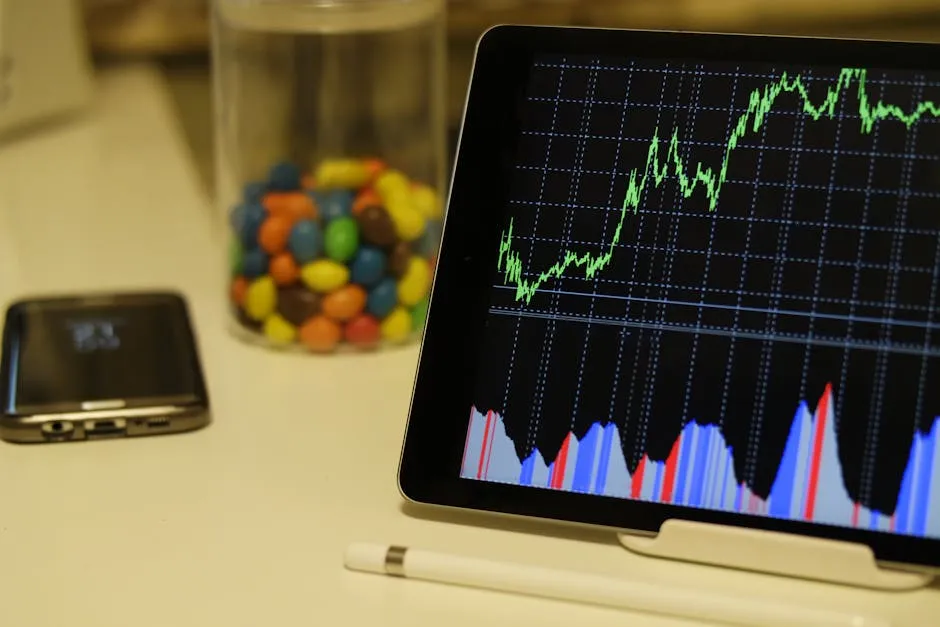
Scatter plots are fantastic for examining relationships between two variables. They can visually display correlations, making it easy to spot trends.
The benefits of graphical representation are numerous. They simplify data interpretation and enhance communication. Visuals can convey insights that raw data often obscures. For example, a well-designed chart can reveal trends that numbers alone might hide.
So, consider using visualization tools in your analysis. They can elevate your understanding and presentation of data. Embrace these techniques to enhance your descriptive statistics journey! If you’re keen on mastering visualization, you might want to explore Tableau Desktop, a powerful tool for creating stunning visuals!
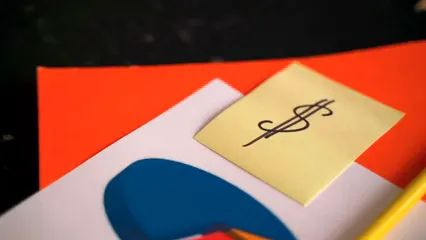
Effective data visualization techniques are essential for clear insights. visualizing data with ap statistics formula sheet techniques
Descriptive Statistics in Practice
Real-World Applications
Descriptive statistics apply across various fields, providing valuable insights. In healthcare, they summarize patient data. For instance, average recovery times can guide treatment protocols.
In marketing, businesses use descriptive statistics to analyze customer preferences. A company might track average purchase amounts to identify spending trends. This helps tailor marketing strategies to specific demographics. To boost your marketing analysis, consider picking up Data Science for Business: What You Need to Know about Data Mining and Data-Analytic Thinking. It’s a great resource to understand the intersection of data science and business!
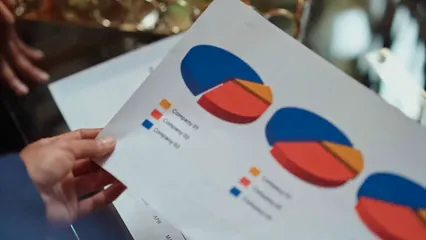
Finance is another domain where descriptive statistics shine. An investment firm may evaluate the average return on portfolios. This helps clients understand their potential earnings.
Consider a case study in education. A school district might analyze student test scores. Descriptive statistics help identify performance gaps among different groups. This informs targeted interventions. If you’re looking for a comprehensive guide on statistics, check out Statistics for Dummies. It’s a beginner-friendly resource that covers the essentials!
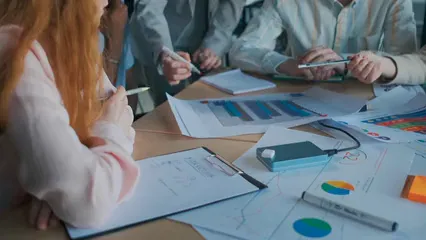
These examples illustrate the importance of descriptive statistics in reporting and decision-making. Think about how you can apply these concepts in your own field. How might understanding data help you make more informed decisions?
Descriptive statistics are crucial in various fields, including education. my education data
Conclusion
Descriptive statistics play a vital role in data analysis. They simplify complex datasets, making the information more digestible. By summarizing key features, these statistics help you interpret data effectively. This understanding is crucial for making informed decisions, whether in business, healthcare, or research.
As you continue your learning journey, apply these concepts in your work. Practicing descriptive statistics can enhance your analytical skills and boost your confidence. Remember, these statistics form the foundation for more advanced statistical analysis. Embrace this knowledge and watch your understanding of data grow! If you’re interested in enhancing your data skills, consider a Python for Data Analysis course to dive into programming for data science!
FAQs
Please let us know what you think about our content by leaving a comment down below!
Thank you for reading till here 🙂
All images from Pexels
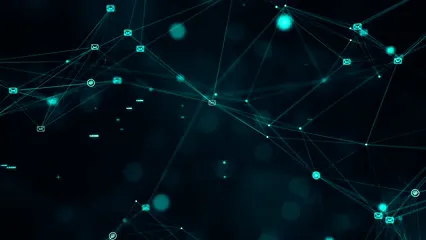
Evaluating variability is crucial for a comprehensive understanding of data. orange county ca crime statistics
Frequency Distribution
A frequency distribution is a summary of how often different values occur within a dataset. It organizes data into categories, showing the count for each category. This tool is crucial in descriptive statistics.
There are two main types of frequency distribution: simple and grouped. A simple frequency distribution lists each unique value with its count. For example, in the dataset {A, A, B, B, B, C}, the simple frequency table looks like this:
Value | Frequency |
---|---|
A | 2 |
B | 3 |
C | 1 |
A grouped frequency distribution combines values into ranges. For instance, if we have ages ranging from 1 to 100, we might group them into intervals like 1-10, 11-20, etc., making it easier to visualize the data. If you’re looking for software to assist in creating these distributions, consider Graphing Software that can help you visualize your data effectively!
Creating and interpreting these tables helps summarize data effectively. Try making your own frequency distribution table with your data. It’s a great way to see patterns and distributions clearly!
Visualizing Data
Importance of Data Visualization
Visual aids are essential for grasping descriptive statistics. They transform complex numbers into digestible images. This makes data easier to understand and analyze.
Common visualizations include histograms, box plots, and scatter plots.
Histograms display the frequency distribution of numerical data. They help identify patterns and trends within the dataset.
Box plots summarize data through quartiles, highlighting key stats like median and outliers. They show how data points spread and where they cluster. If you’re interested in creating box plots, a Box Plot Chart Maker might be just what you need!
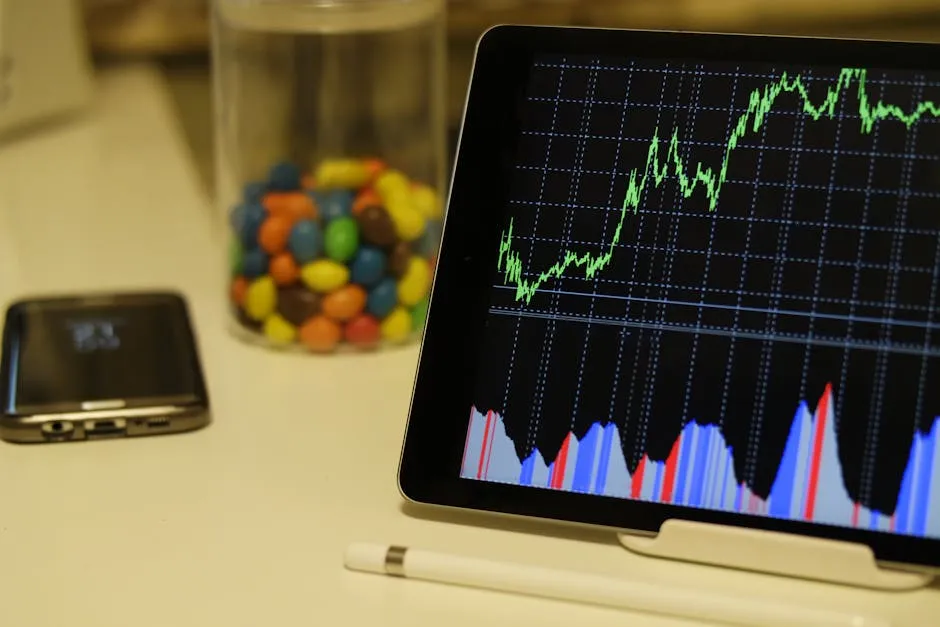
Scatter plots are fantastic for examining relationships between two variables. They can visually display correlations, making it easy to spot trends.
The benefits of graphical representation are numerous. They simplify data interpretation and enhance communication. Visuals can convey insights that raw data often obscures. For example, a well-designed chart can reveal trends that numbers alone might hide.
So, consider using visualization tools in your analysis. They can elevate your understanding and presentation of data. Embrace these techniques to enhance your descriptive statistics journey! If you’re keen on mastering visualization, you might want to explore Tableau Desktop, a powerful tool for creating stunning visuals!
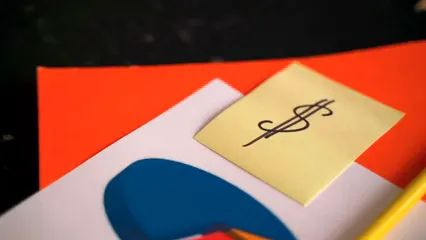
Effective data visualization techniques are essential for clear insights. visualizing data with ap statistics formula sheet techniques
Descriptive Statistics in Practice
Real-World Applications
Descriptive statistics apply across various fields, providing valuable insights. In healthcare, they summarize patient data. For instance, average recovery times can guide treatment protocols.
In marketing, businesses use descriptive statistics to analyze customer preferences. A company might track average purchase amounts to identify spending trends. This helps tailor marketing strategies to specific demographics. To boost your marketing analysis, consider picking up Data Science for Business: What You Need to Know about Data Mining and Data-Analytic Thinking. It’s a great resource to understand the intersection of data science and business!
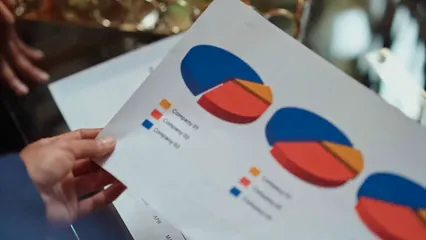
Finance is another domain where descriptive statistics shine. An investment firm may evaluate the average return on portfolios. This helps clients understand their potential earnings.
Consider a case study in education. A school district might analyze student test scores. Descriptive statistics help identify performance gaps among different groups. This informs targeted interventions. If you’re looking for a comprehensive guide on statistics, check out Statistics for Dummies. It’s a beginner-friendly resource that covers the essentials!
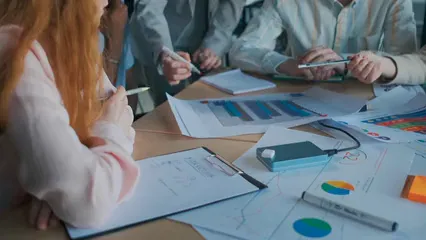
These examples illustrate the importance of descriptive statistics in reporting and decision-making. Think about how you can apply these concepts in your own field. How might understanding data help you make more informed decisions?
Descriptive statistics are crucial in various fields, including education. my education data
Conclusion
Descriptive statistics play a vital role in data analysis. They simplify complex datasets, making the information more digestible. By summarizing key features, these statistics help you interpret data effectively. This understanding is crucial for making informed decisions, whether in business, healthcare, or research.
As you continue your learning journey, apply these concepts in your work. Practicing descriptive statistics can enhance your analytical skills and boost your confidence. Remember, these statistics form the foundation for more advanced statistical analysis. Embrace this knowledge and watch your understanding of data grow! If you’re interested in enhancing your data skills, consider a Python for Data Analysis course to dive into programming for data science!
FAQs
Please let us know what you think about our content by leaving a comment down below!
Thank you for reading till here 🙂
All images from Pexels
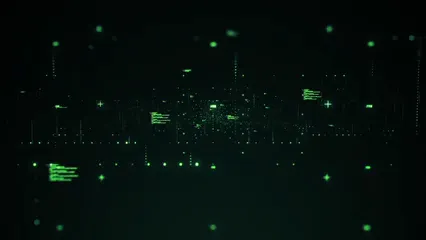
Consider a business analyzing customer feedback scores. By calculating the average score, the company can assess overall satisfaction. They might also review the range of scores to gauge variability in customer experiences.
Ultimately, these statistics aid decision-making across various fields. Whether in business, healthcare, or social research, they provide clarity. Think about your own data analysis needs. How could descriptive statistics improve your understanding of your data?
Descriptive statistics are essential for understanding data characteristics and trends. descriptive statistics in manufacturing plant
Measures of Variability
Range, Variance, Standard Deviation
Measures of variability tell us how spread out data is. They help us understand not just the average but also the differences within the dataset. Key measures include range, variance, and standard deviation.
The range is the simplest measure. It’s calculated by subtracting the smallest value from the largest value. For instance, in the dataset {4, 7, 10, 15}, the range is 15 – 4 = 11. This tells us the extent of the data spread.
Variance measures how far each number in the set is from the mean. It’s calculated by finding the average of the squared differences from the mean. For example, if our data set is {2, 4, 6}, the mean is 4. Variance would be ((2–4)² + (4–4)² + (6–4)²) / 3 = 2.67.
Standard deviation is the square root of variance and is more interpretable. It shows the average distance of each data point from the mean. A low standard deviation means data points are close to the mean, while a high one indicates they are spread out.
Understanding these measures can enhance your analysis. So, consider evaluating the variability in your own data sets! And if you’re looking for tools to visualize this variability, a Histogram Chart Maker can be a great addition to your toolkit!
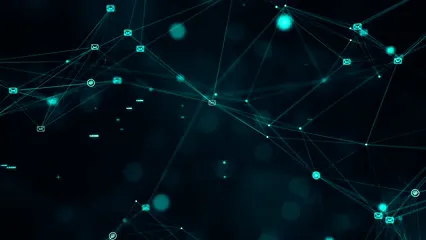
Evaluating variability is crucial for a comprehensive understanding of data. orange county ca crime statistics
Frequency Distribution
A frequency distribution is a summary of how often different values occur within a dataset. It organizes data into categories, showing the count for each category. This tool is crucial in descriptive statistics.
There are two main types of frequency distribution: simple and grouped. A simple frequency distribution lists each unique value with its count. For example, in the dataset {A, A, B, B, B, C}, the simple frequency table looks like this:
Value | Frequency |
---|---|
A | 2 |
B | 3 |
C | 1 |
A grouped frequency distribution combines values into ranges. For instance, if we have ages ranging from 1 to 100, we might group them into intervals like 1-10, 11-20, etc., making it easier to visualize the data. If you’re looking for software to assist in creating these distributions, consider Graphing Software that can help you visualize your data effectively!
Creating and interpreting these tables helps summarize data effectively. Try making your own frequency distribution table with your data. It’s a great way to see patterns and distributions clearly!
Visualizing Data
Importance of Data Visualization
Visual aids are essential for grasping descriptive statistics. They transform complex numbers into digestible images. This makes data easier to understand and analyze.
Common visualizations include histograms, box plots, and scatter plots.
Histograms display the frequency distribution of numerical data. They help identify patterns and trends within the dataset.
Box plots summarize data through quartiles, highlighting key stats like median and outliers. They show how data points spread and where they cluster. If you’re interested in creating box plots, a Box Plot Chart Maker might be just what you need!
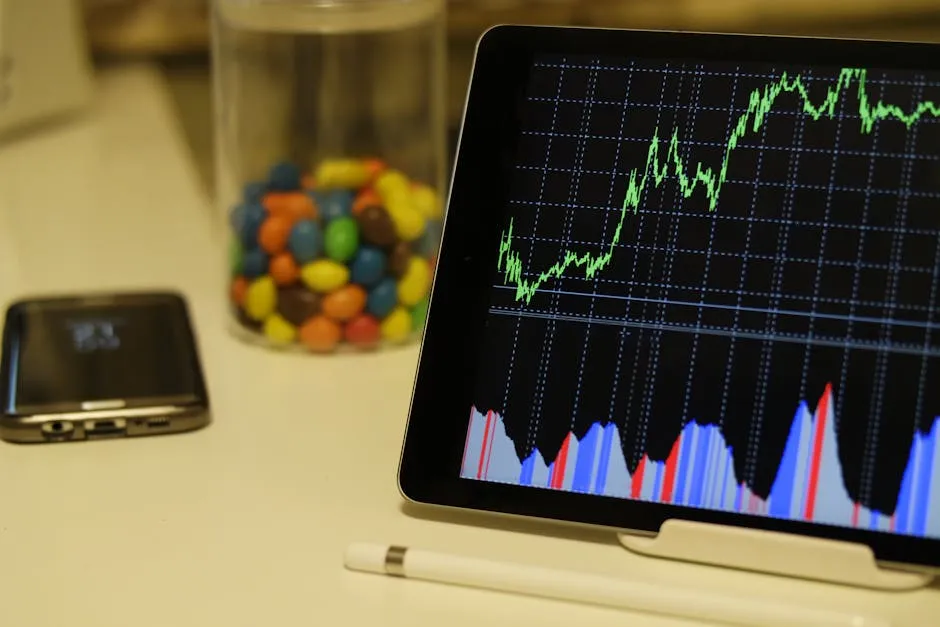
Scatter plots are fantastic for examining relationships between two variables. They can visually display correlations, making it easy to spot trends.
The benefits of graphical representation are numerous. They simplify data interpretation and enhance communication. Visuals can convey insights that raw data often obscures. For example, a well-designed chart can reveal trends that numbers alone might hide.
So, consider using visualization tools in your analysis. They can elevate your understanding and presentation of data. Embrace these techniques to enhance your descriptive statistics journey! If you’re keen on mastering visualization, you might want to explore Tableau Desktop, a powerful tool for creating stunning visuals!
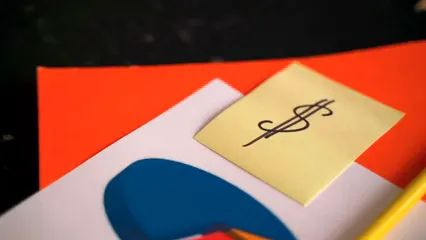
Effective data visualization techniques are essential for clear insights. visualizing data with ap statistics formula sheet techniques
Descriptive Statistics in Practice
Real-World Applications
Descriptive statistics apply across various fields, providing valuable insights. In healthcare, they summarize patient data. For instance, average recovery times can guide treatment protocols.
In marketing, businesses use descriptive statistics to analyze customer preferences. A company might track average purchase amounts to identify spending trends. This helps tailor marketing strategies to specific demographics. To boost your marketing analysis, consider picking up Data Science for Business: What You Need to Know about Data Mining and Data-Analytic Thinking. It’s a great resource to understand the intersection of data science and business!
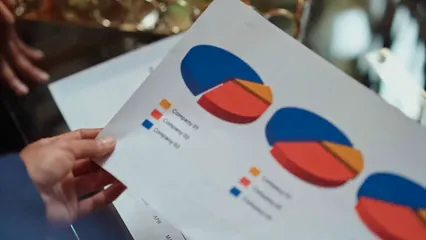
Finance is another domain where descriptive statistics shine. An investment firm may evaluate the average return on portfolios. This helps clients understand their potential earnings.
Consider a case study in education. A school district might analyze student test scores. Descriptive statistics help identify performance gaps among different groups. This informs targeted interventions. If you’re looking for a comprehensive guide on statistics, check out Statistics for Dummies. It’s a beginner-friendly resource that covers the essentials!
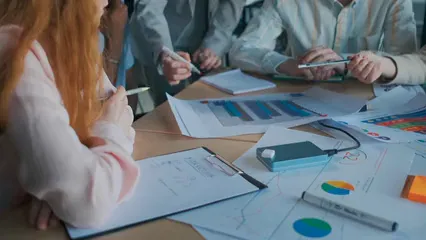
These examples illustrate the importance of descriptive statistics in reporting and decision-making. Think about how you can apply these concepts in your own field. How might understanding data help you make more informed decisions?
Descriptive statistics are crucial in various fields, including education. my education data
Conclusion
Descriptive statistics play a vital role in data analysis. They simplify complex datasets, making the information more digestible. By summarizing key features, these statistics help you interpret data effectively. This understanding is crucial for making informed decisions, whether in business, healthcare, or research.
As you continue your learning journey, apply these concepts in your work. Practicing descriptive statistics can enhance your analytical skills and boost your confidence. Remember, these statistics form the foundation for more advanced statistical analysis. Embrace this knowledge and watch your understanding of data grow! If you’re interested in enhancing your data skills, consider a Python for Data Analysis course to dive into programming for data science!
FAQs
Please let us know what you think about our content by leaving a comment down below!
Thank you for reading till here 🙂
All images from Pexels
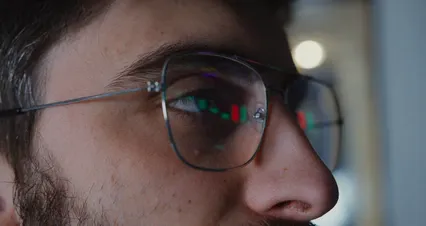
Purpose of Descriptive Statistics
Descriptive statistics serve as a powerful tool in the realm of data analysis. They simplify complex datasets into understandable summaries. This simplification is crucial for effective communication of data insights.
One key objective is initial data assessment. When you gather data, it’s essential to understand its basic characteristics. Descriptive statistics provide a snapshot of trends, patterns, and anomalies, allowing you to identify areas for further investigation.
Data visualization plays a significant role here. Visual representations, like charts and graphs, enhance comprehension. For instance, a bar chart can quickly show sales figures over a period, making it easy to spot trends. If you’re interested in exploring data visualization techniques, check out Data Visualization: A Practical Introduction. It’s a great resource for mastering the art of visual data representation!
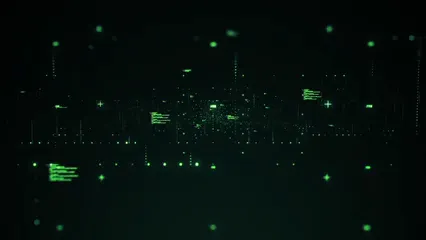
Consider a business analyzing customer feedback scores. By calculating the average score, the company can assess overall satisfaction. They might also review the range of scores to gauge variability in customer experiences.
Ultimately, these statistics aid decision-making across various fields. Whether in business, healthcare, or social research, they provide clarity. Think about your own data analysis needs. How could descriptive statistics improve your understanding of your data?
Descriptive statistics are essential for understanding data characteristics and trends. descriptive statistics in manufacturing plant
Measures of Variability
Range, Variance, Standard Deviation
Measures of variability tell us how spread out data is. They help us understand not just the average but also the differences within the dataset. Key measures include range, variance, and standard deviation.
The range is the simplest measure. It’s calculated by subtracting the smallest value from the largest value. For instance, in the dataset {4, 7, 10, 15}, the range is 15 – 4 = 11. This tells us the extent of the data spread.
Variance measures how far each number in the set is from the mean. It’s calculated by finding the average of the squared differences from the mean. For example, if our data set is {2, 4, 6}, the mean is 4. Variance would be ((2–4)² + (4–4)² + (6–4)²) / 3 = 2.67.
Standard deviation is the square root of variance and is more interpretable. It shows the average distance of each data point from the mean. A low standard deviation means data points are close to the mean, while a high one indicates they are spread out.
Understanding these measures can enhance your analysis. So, consider evaluating the variability in your own data sets! And if you’re looking for tools to visualize this variability, a Histogram Chart Maker can be a great addition to your toolkit!
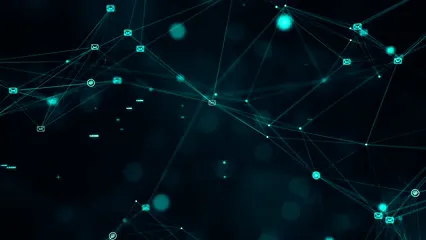
Evaluating variability is crucial for a comprehensive understanding of data. orange county ca crime statistics
Frequency Distribution
A frequency distribution is a summary of how often different values occur within a dataset. It organizes data into categories, showing the count for each category. This tool is crucial in descriptive statistics.
There are two main types of frequency distribution: simple and grouped. A simple frequency distribution lists each unique value with its count. For example, in the dataset {A, A, B, B, B, C}, the simple frequency table looks like this:
Value | Frequency |
---|---|
A | 2 |
B | 3 |
C | 1 |
A grouped frequency distribution combines values into ranges. For instance, if we have ages ranging from 1 to 100, we might group them into intervals like 1-10, 11-20, etc., making it easier to visualize the data. If you’re looking for software to assist in creating these distributions, consider Graphing Software that can help you visualize your data effectively!
Creating and interpreting these tables helps summarize data effectively. Try making your own frequency distribution table with your data. It’s a great way to see patterns and distributions clearly!
Visualizing Data
Importance of Data Visualization
Visual aids are essential for grasping descriptive statistics. They transform complex numbers into digestible images. This makes data easier to understand and analyze.
Common visualizations include histograms, box plots, and scatter plots.
Histograms display the frequency distribution of numerical data. They help identify patterns and trends within the dataset.
Box plots summarize data through quartiles, highlighting key stats like median and outliers. They show how data points spread and where they cluster. If you’re interested in creating box plots, a Box Plot Chart Maker might be just what you need!
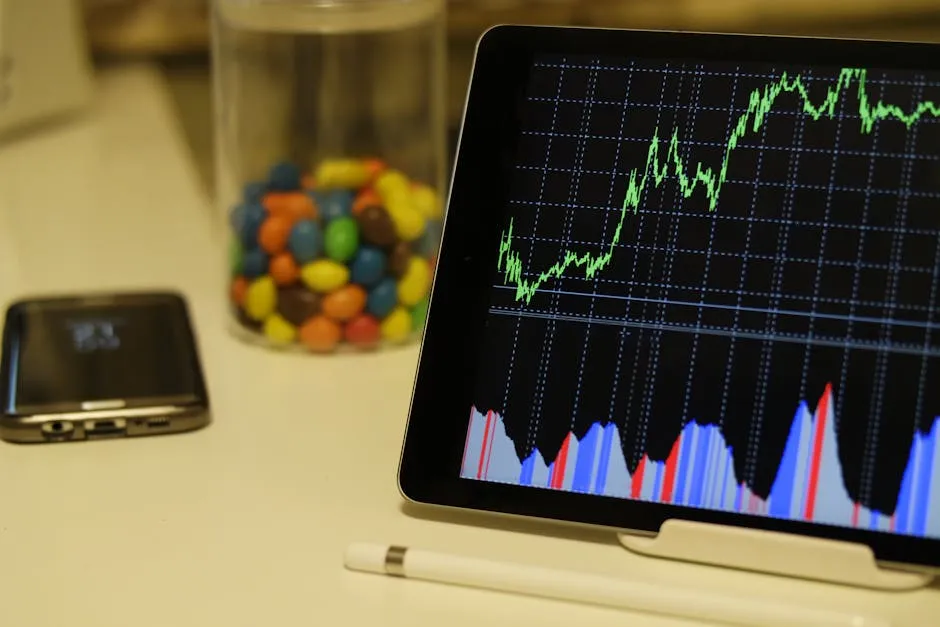
Scatter plots are fantastic for examining relationships between two variables. They can visually display correlations, making it easy to spot trends.
The benefits of graphical representation are numerous. They simplify data interpretation and enhance communication. Visuals can convey insights that raw data often obscures. For example, a well-designed chart can reveal trends that numbers alone might hide.
So, consider using visualization tools in your analysis. They can elevate your understanding and presentation of data. Embrace these techniques to enhance your descriptive statistics journey! If you’re keen on mastering visualization, you might want to explore Tableau Desktop, a powerful tool for creating stunning visuals!
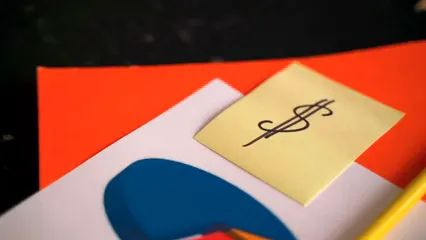
Effective data visualization techniques are essential for clear insights. visualizing data with ap statistics formula sheet techniques
Descriptive Statistics in Practice
Real-World Applications
Descriptive statistics apply across various fields, providing valuable insights. In healthcare, they summarize patient data. For instance, average recovery times can guide treatment protocols.
In marketing, businesses use descriptive statistics to analyze customer preferences. A company might track average purchase amounts to identify spending trends. This helps tailor marketing strategies to specific demographics. To boost your marketing analysis, consider picking up Data Science for Business: What You Need to Know about Data Mining and Data-Analytic Thinking. It’s a great resource to understand the intersection of data science and business!
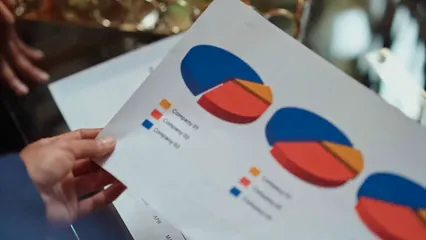
Finance is another domain where descriptive statistics shine. An investment firm may evaluate the average return on portfolios. This helps clients understand their potential earnings.
Consider a case study in education. A school district might analyze student test scores. Descriptive statistics help identify performance gaps among different groups. This informs targeted interventions. If you’re looking for a comprehensive guide on statistics, check out Statistics for Dummies. It’s a beginner-friendly resource that covers the essentials!
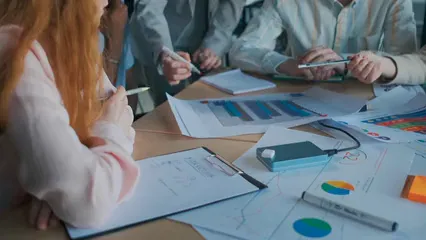
These examples illustrate the importance of descriptive statistics in reporting and decision-making. Think about how you can apply these concepts in your own field. How might understanding data help you make more informed decisions?
Descriptive statistics are crucial in various fields, including education. my education data
Conclusion
Descriptive statistics play a vital role in data analysis. They simplify complex datasets, making the information more digestible. By summarizing key features, these statistics help you interpret data effectively. This understanding is crucial for making informed decisions, whether in business, healthcare, or research.
As you continue your learning journey, apply these concepts in your work. Practicing descriptive statistics can enhance your analytical skills and boost your confidence. Remember, these statistics form the foundation for more advanced statistical analysis. Embrace this knowledge and watch your understanding of data grow! If you’re interested in enhancing your data skills, consider a Python for Data Analysis course to dive into programming for data science!
FAQs
Please let us know what you think about our content by leaving a comment down below!
Thank you for reading till here 🙂
All images from Pexels
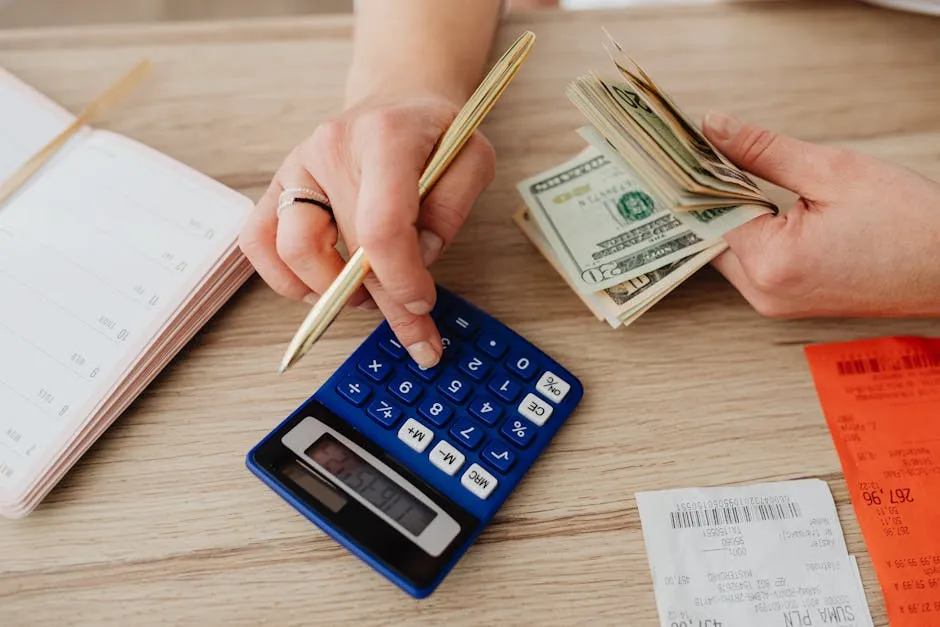
For those who want to dive deeper into the world of descriptive statistics, grab a copy of Descriptive Statistics: A Practical Guide for Researchers. This book breaks down complex concepts into manageable bites, perfect for both novices and seasoned statisticians!
Understanding Descriptive Statistics
What Are Descriptive Statistics?
Descriptive statistics are tools that summarize and describe characteristics of a dataset. They make complex data more understandable and can help identify trends, patterns, and anomalies.
These statistics play a crucial role in data interpretation. By summarizing data, we can focus on essential aspects without getting lost in details. Common examples include calculating average sales figures or summarizing test scores in a classroom.
For instance, consider a dataset of students’ test scores: 70, 75, 80, 85, and 90. The mean score is 80, providing a quick understanding of overall performance. Other statistics, like median and mode, can further clarify the data’s center.
Reflect on how you use descriptive statistics in your own work. Whether in marketing, research, or finance, these tools are foundational for effective data analysis. If you’re looking to enhance your analysis skills, consider Excel for Data Analysis. This guide will empower you to harness the full potential of Excel in your data endeavors!
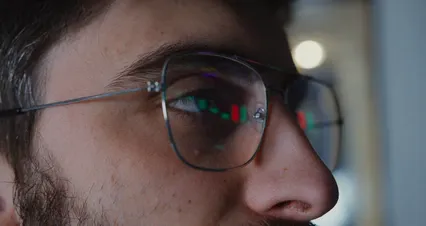
Purpose of Descriptive Statistics
Descriptive statistics serve as a powerful tool in the realm of data analysis. They simplify complex datasets into understandable summaries. This simplification is crucial for effective communication of data insights.
One key objective is initial data assessment. When you gather data, it’s essential to understand its basic characteristics. Descriptive statistics provide a snapshot of trends, patterns, and anomalies, allowing you to identify areas for further investigation.
Data visualization plays a significant role here. Visual representations, like charts and graphs, enhance comprehension. For instance, a bar chart can quickly show sales figures over a period, making it easy to spot trends. If you’re interested in exploring data visualization techniques, check out Data Visualization: A Practical Introduction. It’s a great resource for mastering the art of visual data representation!
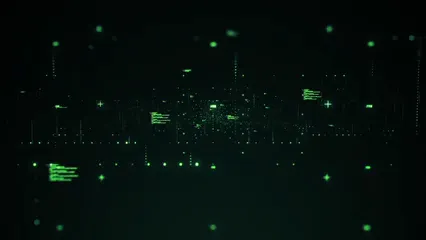
Consider a business analyzing customer feedback scores. By calculating the average score, the company can assess overall satisfaction. They might also review the range of scores to gauge variability in customer experiences.
Ultimately, these statistics aid decision-making across various fields. Whether in business, healthcare, or social research, they provide clarity. Think about your own data analysis needs. How could descriptive statistics improve your understanding of your data?
Descriptive statistics are essential for understanding data characteristics and trends. descriptive statistics in manufacturing plant
Measures of Variability
Range, Variance, Standard Deviation
Measures of variability tell us how spread out data is. They help us understand not just the average but also the differences within the dataset. Key measures include range, variance, and standard deviation.
The range is the simplest measure. It’s calculated by subtracting the smallest value from the largest value. For instance, in the dataset {4, 7, 10, 15}, the range is 15 – 4 = 11. This tells us the extent of the data spread.
Variance measures how far each number in the set is from the mean. It’s calculated by finding the average of the squared differences from the mean. For example, if our data set is {2, 4, 6}, the mean is 4. Variance would be ((2–4)² + (4–4)² + (6–4)²) / 3 = 2.67.
Standard deviation is the square root of variance and is more interpretable. It shows the average distance of each data point from the mean. A low standard deviation means data points are close to the mean, while a high one indicates they are spread out.
Understanding these measures can enhance your analysis. So, consider evaluating the variability in your own data sets! And if you’re looking for tools to visualize this variability, a Histogram Chart Maker can be a great addition to your toolkit!
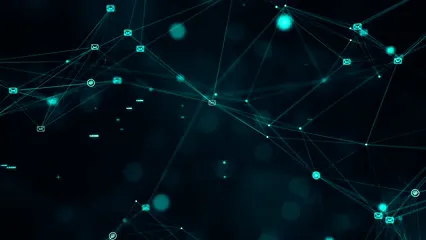
Evaluating variability is crucial for a comprehensive understanding of data. orange county ca crime statistics
Frequency Distribution
A frequency distribution is a summary of how often different values occur within a dataset. It organizes data into categories, showing the count for each category. This tool is crucial in descriptive statistics.
There are two main types of frequency distribution: simple and grouped. A simple frequency distribution lists each unique value with its count. For example, in the dataset {A, A, B, B, B, C}, the simple frequency table looks like this:
Value | Frequency |
---|---|
A | 2 |
B | 3 |
C | 1 |
A grouped frequency distribution combines values into ranges. For instance, if we have ages ranging from 1 to 100, we might group them into intervals like 1-10, 11-20, etc., making it easier to visualize the data. If you’re looking for software to assist in creating these distributions, consider Graphing Software that can help you visualize your data effectively!
Creating and interpreting these tables helps summarize data effectively. Try making your own frequency distribution table with your data. It’s a great way to see patterns and distributions clearly!
Visualizing Data
Importance of Data Visualization
Visual aids are essential for grasping descriptive statistics. They transform complex numbers into digestible images. This makes data easier to understand and analyze.
Common visualizations include histograms, box plots, and scatter plots.
Histograms display the frequency distribution of numerical data. They help identify patterns and trends within the dataset.
Box plots summarize data through quartiles, highlighting key stats like median and outliers. They show how data points spread and where they cluster. If you’re interested in creating box plots, a Box Plot Chart Maker might be just what you need!
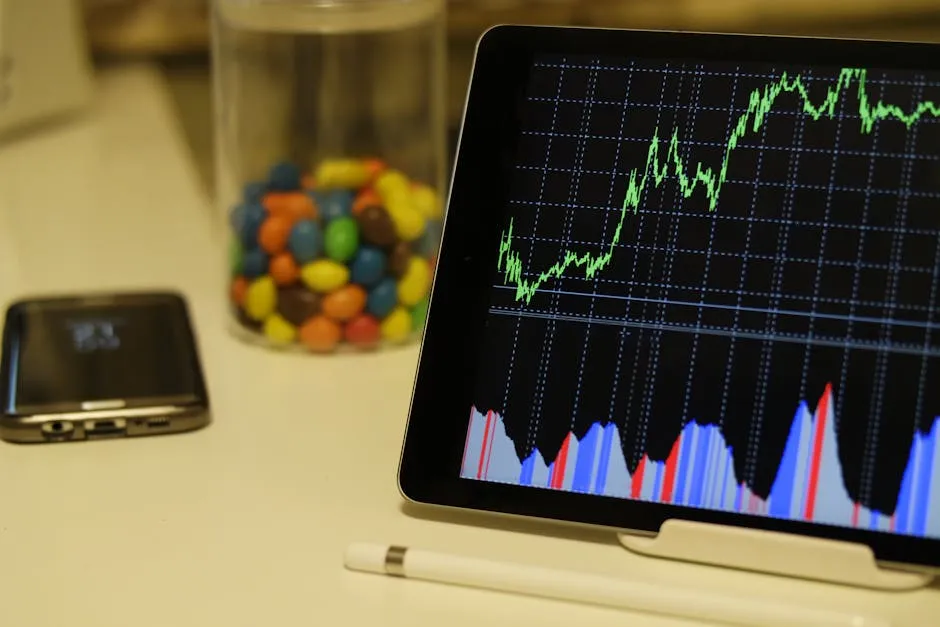
Scatter plots are fantastic for examining relationships between two variables. They can visually display correlations, making it easy to spot trends.
The benefits of graphical representation are numerous. They simplify data interpretation and enhance communication. Visuals can convey insights that raw data often obscures. For example, a well-designed chart can reveal trends that numbers alone might hide.
So, consider using visualization tools in your analysis. They can elevate your understanding and presentation of data. Embrace these techniques to enhance your descriptive statistics journey! If you’re keen on mastering visualization, you might want to explore Tableau Desktop, a powerful tool for creating stunning visuals!
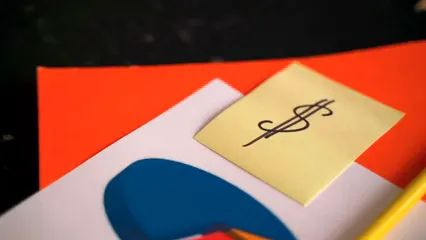
Effective data visualization techniques are essential for clear insights. visualizing data with ap statistics formula sheet techniques
Descriptive Statistics in Practice
Real-World Applications
Descriptive statistics apply across various fields, providing valuable insights. In healthcare, they summarize patient data. For instance, average recovery times can guide treatment protocols.
In marketing, businesses use descriptive statistics to analyze customer preferences. A company might track average purchase amounts to identify spending trends. This helps tailor marketing strategies to specific demographics. To boost your marketing analysis, consider picking up Data Science for Business: What You Need to Know about Data Mining and Data-Analytic Thinking. It’s a great resource to understand the intersection of data science and business!
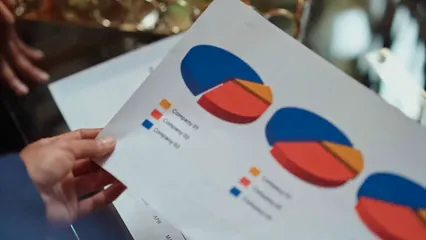
Finance is another domain where descriptive statistics shine. An investment firm may evaluate the average return on portfolios. This helps clients understand their potential earnings.
Consider a case study in education. A school district might analyze student test scores. Descriptive statistics help identify performance gaps among different groups. This informs targeted interventions. If you’re looking for a comprehensive guide on statistics, check out Statistics for Dummies. It’s a beginner-friendly resource that covers the essentials!
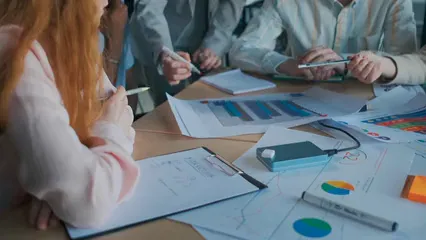
These examples illustrate the importance of descriptive statistics in reporting and decision-making. Think about how you can apply these concepts in your own field. How might understanding data help you make more informed decisions?
Descriptive statistics are crucial in various fields, including education. my education data
Conclusion
Descriptive statistics play a vital role in data analysis. They simplify complex datasets, making the information more digestible. By summarizing key features, these statistics help you interpret data effectively. This understanding is crucial for making informed decisions, whether in business, healthcare, or research.
As you continue your learning journey, apply these concepts in your work. Practicing descriptive statistics can enhance your analytical skills and boost your confidence. Remember, these statistics form the foundation for more advanced statistical analysis. Embrace this knowledge and watch your understanding of data grow! If you’re interested in enhancing your data skills, consider a Python for Data Analysis course to dive into programming for data science!
FAQs
Please let us know what you think about our content by leaving a comment down below!
Thank you for reading till here 🙂
All images from Pexels