Now, applying the formula:
r = \frac{-700}{14.14 \cdot 40} \approx -1.24
This coefficient indicates a strong negative correlation. If you’re looking to enhance your data analysis skills, check out Data Science for Business. It provides a great perspective on applying data science techniques in real-world scenarios!
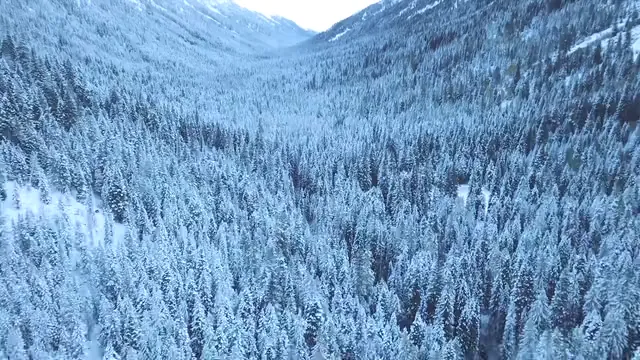
Example 3: No Correlation
Lastly, let’s examine a dataset with no correlation, like shoe size and intelligence score:
Shoe Size (X) | Intelligence Score (Y) |
---|---|
5 | 100 |
6 | 110 |
7 | 105 |
8 | 95 |
9 | 102 |
Calculating the means:
– Mean of X = (5+6+7+8+9)/5 = 7
– Mean of Y = (100+110+105+95+102)/5 = 102.4
Next, we compute the covariance and standard deviations.
- Covariance:
cov(X,Y) = \frac{(5-7)(100-102.4)+(6-7)(110-102.4)+(7-7)(105-102.4)+(8-7)(95-102.4)+(9-7)(102-102.4)}{n} = 0
- Standard Deviations:
s_x = \sqrt{\frac{(5-7)^2+(6-7)^2+(7-7)^2+(8-7)^2+(9-7)^2}{5}} = \sqrt{2} \approx 1.41
s_y = \sqrt{\frac{(100-102.4)^2+(110-102.4)^2+(105-102.4)^2+(95-102.4)^2+(102-102.4)^2}{5}} \approx 4.47
Finally, the correlation coefficient is:
r = \frac{0}{1.41 \cdot 4.47} = 0
This indicates no correlation between shoe size and intelligence. If you want to sharpen your statistical skills further, consider grabbing a copy of Practical Statistics for Data Scientists. It’s an excellent resource for understanding practical applications of statistics!

Interpreting the Results
Understanding the Value of the Correlation Coefficient
The correlation coefficient ranges from -1 to 1. A value of 1 indicates a perfect positive correlation, meaning both variables increase together. Conversely, -1 indicates a perfect negative correlation, where one variable increases as the other decreases. A value around 0 suggests little to no correlation, indicating no linear relationship exists.
In practical terms, a strong positive correlation means that if you know one variable, you can predict the other with confidence. For instance, in the positive correlation example, knowing how many hours a student studied helps predict their exam score. A strong negative correlation, like temperature and hot chocolate sales, suggests that as one variable increases, the other decreases, which can inform business strategies. Understanding these correlations is critical for making informed decisions based on data.

Common Misinterpretations
When discussing correlation, many people confuse it with causation. Just because two variables show a correlation, it doesn’t mean one causes the other. For instance, imagine a study finds that ice cream sales go up in summer. At the same time, drowning incidents increase. This doesn’t imply that buying ice cream causes drownings. Instead, both are influenced by warmer weather.
Another common misunderstanding is the idea that a strong correlation means a perfect relationship. A correlation coefficient of 0.9 suggests a strong positive correlation. It means that as one variable increases, the other tends to do so too. However, this doesn’t mean they move together perfectly. There can still be deviations.
People also think a correlation of zero means no relationship at all. While it indicates no linear relationship, there could still be a non-linear connection. For example, the relationship between the amount of time spent studying and test scores might not be linear.
Understanding these nuances is vital for accurately interpreting data. Always look deeper to see the full picture behind the numbers.
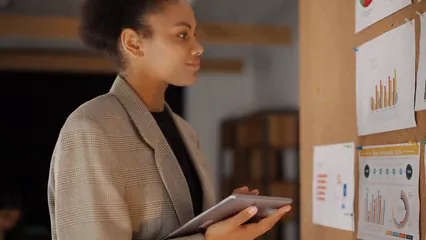
Applications of Correlation Coefficient in Various Fields
Research and Academia
Researchers frequently apply correlation coefficients to analyze data. They use these coefficients to identify relationships between variables, helping to validate hypotheses. For instance, a study might investigate the correlation between study hours and exam performance. A strong positive correlation could support the idea that more study time leads to better grades.
If you’re looking for a comprehensive guide on statistical methods, consider Statistical Methods for Psychology. It’s a great resource for understanding statistical techniques in psychological research!
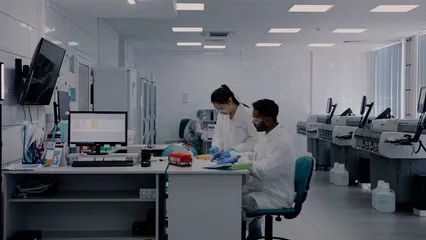
Business and Marketing
In business, correlation analysis is crucial for market research. Companies examine the relationship between advertising spend and sales revenue. If they find a strong positive correlation, they might decide to increase their advertising budget. Understanding consumer behavior also relies on correlation. For instance, businesses can track how customer satisfaction correlates with loyalty.
If you’re looking to enhance your business intelligence capabilities, consider checking out Business Intelligence Guidebook. It provides insights into leveraging data for business success!
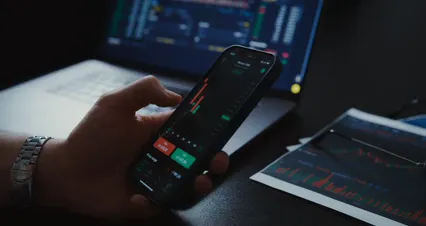
Healthcare and Science
In healthcare, correlation coefficients can reveal important insights. Researchers often study correlations between lifestyle factors and health outcomes. For example, a study might show a negative correlation between physical activity and obesity rates. Such findings can inform public health strategies aimed at reducing obesity.
In scientific research, correlation analysis helps in developing theories. Scientists might explore correlations between environmental factors and species population dynamics. These insights contribute to conservation efforts and ecological understanding. For those interested in a deeper dive into data science, The Data Science Handbook is a must-read!
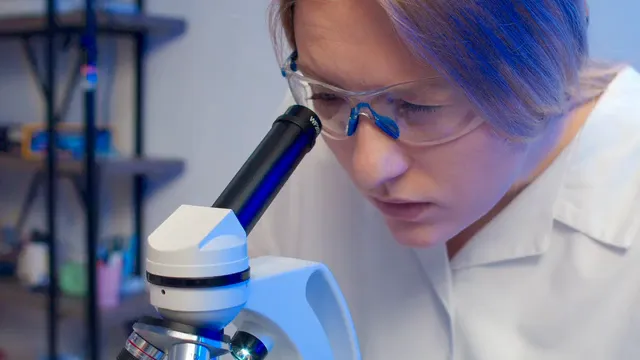
Conclusion
The correlation coefficient is a powerful tool for analyzing relationships between variables. It provides clarity in various fields, from research to business and healthcare. Utilizing correlation analysis can enhance your understanding of data and help inform decisions. For further reading on correlation, you can explore correlation.
Please let us know what you think about our content by leaving a comment down below!
Thank you for reading till here 🙂
All images from Pexels
- Covariance:
cov(X,Y) = \frac{(1-3)(50-70)+(2-3)(60-70)+(3-3)(70-70)+(4-3)(80-70)+(5-3)(90-70)}{n} = 40
- Standard Deviations:
s_x = \sqrt{\frac{(1-3)^2+(2-3)^2+(3-3)^2+(4-3)^2+(5-3)^2}{5}} = \sqrt{2} \approx 1.41
s_y = \sqrt{\frac{(50-70)^2+(60-70)^2+(70-70)^2+(80-70)^2+(90-70)^2}{5}} = \sqrt{400} = 20
Finally, we plug these into the Pearson correlation formula:
r = \frac{cov(X,Y)}{s_x \cdot s_y} = \frac{40}{1.41 \cdot 20} \approx 1.42
The resulting correlation coefficient indicates a strong positive correlation. If you want to dive deeper into statistical concepts, consider picking up Statistics for Dummies. It’s a great resource for beginners!
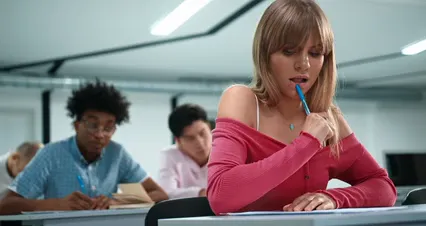
Example 2: Negative Correlation
Now, let’s explore a negative correlation using temperature and hot chocolate sales. Here’s the dataset:
Temperature (°F) | Hot Chocolate Sales (Y) |
---|---|
30 | 100 |
40 | 80 |
50 | 60 |
60 | 30 |
70 | 10 |
Calculating the means:
– Mean of X = (30+40+50+60+70)/5 = 50
– Mean of Y = (100+80+60+30+10)/5 = 58
Next, we find the covariance and standard deviations.
- Covariance:
cov(X,Y) = \frac{(30-50)(100-58)+(40-50)(80-58)+(50-50)(60-58)+(60-50)(30-58)+(70-50)(10-58)}{n} = -700
- Standard Deviations:
s_x = \sqrt{\frac{(30-50)^2+(40-50)^2+(50-50)^2+(60-50)^2+(70-50)^2}{5}} = \sqrt{200} \approx 14.14
s_y = \sqrt{\frac{(100-58)^2+(80-58)^2+(60-58)^2+(30-58)^2+(10-58)^2}{5}} = \sqrt{1600} = 40
Now, applying the formula:
r = \frac{-700}{14.14 \cdot 40} \approx -1.24
This coefficient indicates a strong negative correlation. If you’re looking to enhance your data analysis skills, check out Data Science for Business. It provides a great perspective on applying data science techniques in real-world scenarios!
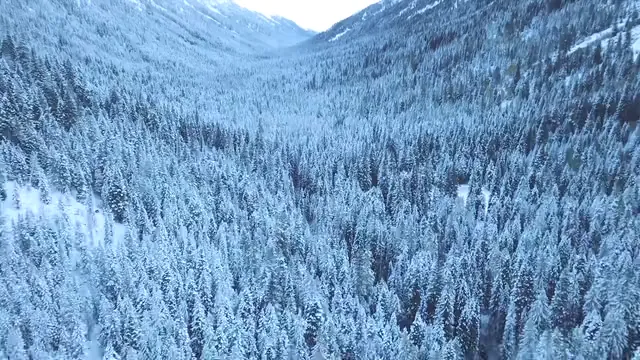
Example 3: No Correlation
Lastly, let’s examine a dataset with no correlation, like shoe size and intelligence score:
Shoe Size (X) | Intelligence Score (Y) |
---|---|
5 | 100 |
6 | 110 |
7 | 105 |
8 | 95 |
9 | 102 |
Calculating the means:
– Mean of X = (5+6+7+8+9)/5 = 7
– Mean of Y = (100+110+105+95+102)/5 = 102.4
Next, we compute the covariance and standard deviations.
- Covariance:
cov(X,Y) = \frac{(5-7)(100-102.4)+(6-7)(110-102.4)+(7-7)(105-102.4)+(8-7)(95-102.4)+(9-7)(102-102.4)}{n} = 0
- Standard Deviations:
s_x = \sqrt{\frac{(5-7)^2+(6-7)^2+(7-7)^2+(8-7)^2+(9-7)^2}{5}} = \sqrt{2} \approx 1.41
s_y = \sqrt{\frac{(100-102.4)^2+(110-102.4)^2+(105-102.4)^2+(95-102.4)^2+(102-102.4)^2}{5}} \approx 4.47
Finally, the correlation coefficient is:
r = \frac{0}{1.41 \cdot 4.47} = 0
This indicates no correlation between shoe size and intelligence. If you want to sharpen your statistical skills further, consider grabbing a copy of Practical Statistics for Data Scientists. It’s an excellent resource for understanding practical applications of statistics!

Interpreting the Results
Understanding the Value of the Correlation Coefficient
The correlation coefficient ranges from -1 to 1. A value of 1 indicates a perfect positive correlation, meaning both variables increase together. Conversely, -1 indicates a perfect negative correlation, where one variable increases as the other decreases. A value around 0 suggests little to no correlation, indicating no linear relationship exists.
In practical terms, a strong positive correlation means that if you know one variable, you can predict the other with confidence. For instance, in the positive correlation example, knowing how many hours a student studied helps predict their exam score. A strong negative correlation, like temperature and hot chocolate sales, suggests that as one variable increases, the other decreases, which can inform business strategies. Understanding these correlations is critical for making informed decisions based on data.

Common Misinterpretations
When discussing correlation, many people confuse it with causation. Just because two variables show a correlation, it doesn’t mean one causes the other. For instance, imagine a study finds that ice cream sales go up in summer. At the same time, drowning incidents increase. This doesn’t imply that buying ice cream causes drownings. Instead, both are influenced by warmer weather.
Another common misunderstanding is the idea that a strong correlation means a perfect relationship. A correlation coefficient of 0.9 suggests a strong positive correlation. It means that as one variable increases, the other tends to do so too. However, this doesn’t mean they move together perfectly. There can still be deviations.
People also think a correlation of zero means no relationship at all. While it indicates no linear relationship, there could still be a non-linear connection. For example, the relationship between the amount of time spent studying and test scores might not be linear.
Understanding these nuances is vital for accurately interpreting data. Always look deeper to see the full picture behind the numbers.
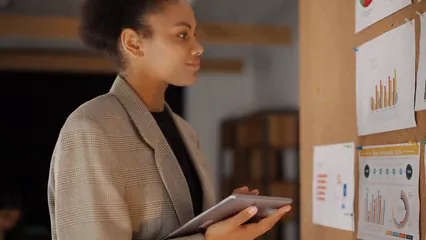
Applications of Correlation Coefficient in Various Fields
Research and Academia
Researchers frequently apply correlation coefficients to analyze data. They use these coefficients to identify relationships between variables, helping to validate hypotheses. For instance, a study might investigate the correlation between study hours and exam performance. A strong positive correlation could support the idea that more study time leads to better grades.
If you’re looking for a comprehensive guide on statistical methods, consider Statistical Methods for Psychology. It’s a great resource for understanding statistical techniques in psychological research!
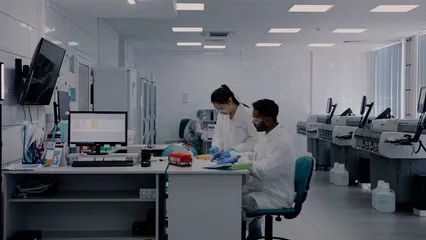
Business and Marketing
In business, correlation analysis is crucial for market research. Companies examine the relationship between advertising spend and sales revenue. If they find a strong positive correlation, they might decide to increase their advertising budget. Understanding consumer behavior also relies on correlation. For instance, businesses can track how customer satisfaction correlates with loyalty.
If you’re looking to enhance your business intelligence capabilities, consider checking out Business Intelligence Guidebook. It provides insights into leveraging data for business success!
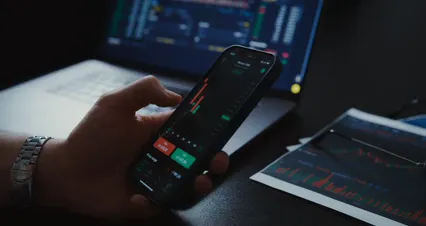
Healthcare and Science
In healthcare, correlation coefficients can reveal important insights. Researchers often study correlations between lifestyle factors and health outcomes. For example, a study might show a negative correlation between physical activity and obesity rates. Such findings can inform public health strategies aimed at reducing obesity.
In scientific research, correlation analysis helps in developing theories. Scientists might explore correlations between environmental factors and species population dynamics. These insights contribute to conservation efforts and ecological understanding. For those interested in a deeper dive into data science, The Data Science Handbook is a must-read!
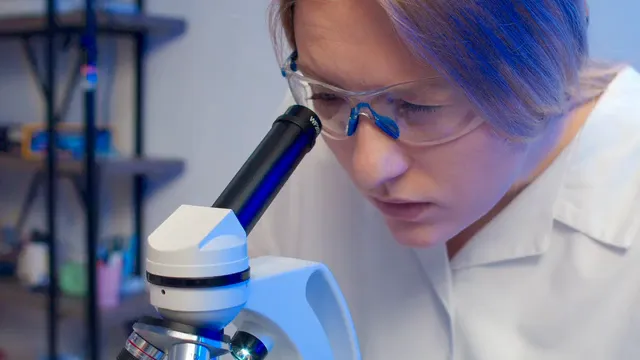
Conclusion
The correlation coefficient is a powerful tool for analyzing relationships between variables. It provides clarity in various fields, from research to business and healthcare. Utilizing correlation analysis can enhance your understanding of data and help inform decisions. For further reading on correlation, you can explore correlation.
Please let us know what you think about our content by leaving a comment down below!
Thank you for reading till here 🙂
All images from Pexels
r = \frac{n(\sum xy) - (\sum x)(\sum y)}{\sqrt{[n\sum x^2 - (\sum x)^2][n\sum y^2 - (\sum y)^2]}}
In this formula:
- n is the number of data pairs.
- ∑xy is the sum of the product of paired scores.
- ∑x and ∑y are the sums of the X and Y scores, respectively.
- ∑x² and ∑y² are the sums of the squares of the X and Y scores.
Each component helps in determining how closely related the two sets of data are.
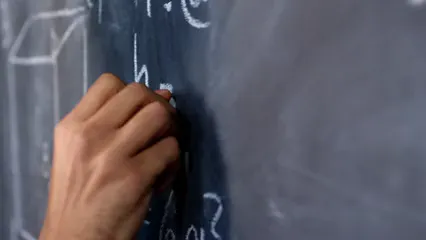
Alternative Formulas
In addition to Pearson’s formula, there are alternative methods to calculate correlation:
- Spearman’s Rank Correlation: This is used for ordinal data or non-linear relationships. It ranks data points and assesses how well the relationship between two variables can be described using a monotonic function.
- Kendall’s Tau: This is another method for ordinal data. It measures the strength of the association between two variables by considering the ranks of the data. It’s particularly useful for small sample sizes.
Choose the appropriate formula based on the nature of your data and the relationships you wish to investigate.
Practical Examples of Correlation Coefficient Calculations
Example 1: Positive Correlation
Let’s consider the relationship between hours studied and exam scores. Here’s a sample dataset:
Hours Studied (X) | Exam Score (Y) |
---|---|
1 | 50 |
2 | 60 |
3 | 70 |
4 | 80 |
5 | 90 |
To calculate the correlation coefficient, we use Pearson’s formula. First, we find the means:
– Mean of X = (1+2+3+4+5)/5 = 3
– Mean of Y = (50+60+70+80+90)/5 = 70
Next, we calculate the covariance and standard deviations.
- Covariance:
cov(X,Y) = \frac{(1-3)(50-70)+(2-3)(60-70)+(3-3)(70-70)+(4-3)(80-70)+(5-3)(90-70)}{n} = 40
- Standard Deviations:
s_x = \sqrt{\frac{(1-3)^2+(2-3)^2+(3-3)^2+(4-3)^2+(5-3)^2}{5}} = \sqrt{2} \approx 1.41
s_y = \sqrt{\frac{(50-70)^2+(60-70)^2+(70-70)^2+(80-70)^2+(90-70)^2}{5}} = \sqrt{400} = 20
Finally, we plug these into the Pearson correlation formula:
r = \frac{cov(X,Y)}{s_x \cdot s_y} = \frac{40}{1.41 \cdot 20} \approx 1.42
The resulting correlation coefficient indicates a strong positive correlation. If you want to dive deeper into statistical concepts, consider picking up Statistics for Dummies. It’s a great resource for beginners!
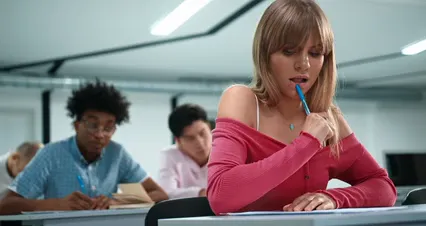
Example 2: Negative Correlation
Now, let’s explore a negative correlation using temperature and hot chocolate sales. Here’s the dataset:
Temperature (°F) | Hot Chocolate Sales (Y) |
---|---|
30 | 100 |
40 | 80 |
50 | 60 |
60 | 30 |
70 | 10 |
Calculating the means:
– Mean of X = (30+40+50+60+70)/5 = 50
– Mean of Y = (100+80+60+30+10)/5 = 58
Next, we find the covariance and standard deviations.
- Covariance:
cov(X,Y) = \frac{(30-50)(100-58)+(40-50)(80-58)+(50-50)(60-58)+(60-50)(30-58)+(70-50)(10-58)}{n} = -700
- Standard Deviations:
s_x = \sqrt{\frac{(30-50)^2+(40-50)^2+(50-50)^2+(60-50)^2+(70-50)^2}{5}} = \sqrt{200} \approx 14.14
s_y = \sqrt{\frac{(100-58)^2+(80-58)^2+(60-58)^2+(30-58)^2+(10-58)^2}{5}} = \sqrt{1600} = 40
Now, applying the formula:
r = \frac{-700}{14.14 \cdot 40} \approx -1.24
This coefficient indicates a strong negative correlation. If you’re looking to enhance your data analysis skills, check out Data Science for Business. It provides a great perspective on applying data science techniques in real-world scenarios!
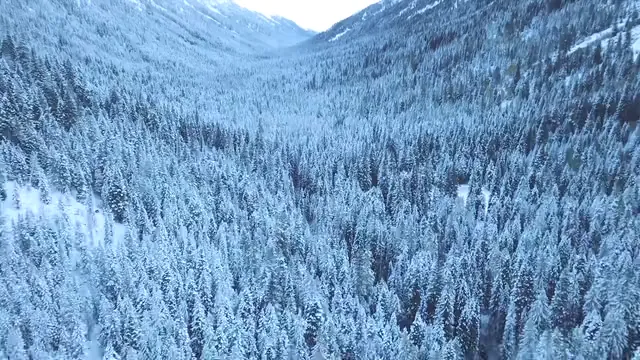
Example 3: No Correlation
Lastly, let’s examine a dataset with no correlation, like shoe size and intelligence score:
Shoe Size (X) | Intelligence Score (Y) |
---|---|
5 | 100 |
6 | 110 |
7 | 105 |
8 | 95 |
9 | 102 |
Calculating the means:
– Mean of X = (5+6+7+8+9)/5 = 7
– Mean of Y = (100+110+105+95+102)/5 = 102.4
Next, we compute the covariance and standard deviations.
- Covariance:
cov(X,Y) = \frac{(5-7)(100-102.4)+(6-7)(110-102.4)+(7-7)(105-102.4)+(8-7)(95-102.4)+(9-7)(102-102.4)}{n} = 0
- Standard Deviations:
s_x = \sqrt{\frac{(5-7)^2+(6-7)^2+(7-7)^2+(8-7)^2+(9-7)^2}{5}} = \sqrt{2} \approx 1.41
s_y = \sqrt{\frac{(100-102.4)^2+(110-102.4)^2+(105-102.4)^2+(95-102.4)^2+(102-102.4)^2}{5}} \approx 4.47
Finally, the correlation coefficient is:
r = \frac{0}{1.41 \cdot 4.47} = 0
This indicates no correlation between shoe size and intelligence. If you want to sharpen your statistical skills further, consider grabbing a copy of Practical Statistics for Data Scientists. It’s an excellent resource for understanding practical applications of statistics!

Interpreting the Results
Understanding the Value of the Correlation Coefficient
The correlation coefficient ranges from -1 to 1. A value of 1 indicates a perfect positive correlation, meaning both variables increase together. Conversely, -1 indicates a perfect negative correlation, where one variable increases as the other decreases. A value around 0 suggests little to no correlation, indicating no linear relationship exists.
In practical terms, a strong positive correlation means that if you know one variable, you can predict the other with confidence. For instance, in the positive correlation example, knowing how many hours a student studied helps predict their exam score. A strong negative correlation, like temperature and hot chocolate sales, suggests that as one variable increases, the other decreases, which can inform business strategies. Understanding these correlations is critical for making informed decisions based on data.

Common Misinterpretations
When discussing correlation, many people confuse it with causation. Just because two variables show a correlation, it doesn’t mean one causes the other. For instance, imagine a study finds that ice cream sales go up in summer. At the same time, drowning incidents increase. This doesn’t imply that buying ice cream causes drownings. Instead, both are influenced by warmer weather.
Another common misunderstanding is the idea that a strong correlation means a perfect relationship. A correlation coefficient of 0.9 suggests a strong positive correlation. It means that as one variable increases, the other tends to do so too. However, this doesn’t mean they move together perfectly. There can still be deviations.
People also think a correlation of zero means no relationship at all. While it indicates no linear relationship, there could still be a non-linear connection. For example, the relationship between the amount of time spent studying and test scores might not be linear.
Understanding these nuances is vital for accurately interpreting data. Always look deeper to see the full picture behind the numbers.
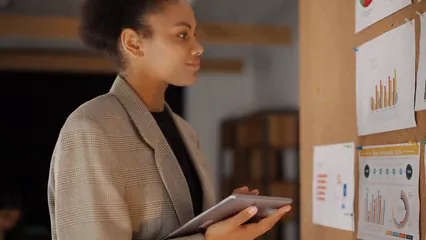
Applications of Correlation Coefficient in Various Fields
Research and Academia
Researchers frequently apply correlation coefficients to analyze data. They use these coefficients to identify relationships between variables, helping to validate hypotheses. For instance, a study might investigate the correlation between study hours and exam performance. A strong positive correlation could support the idea that more study time leads to better grades.
If you’re looking for a comprehensive guide on statistical methods, consider Statistical Methods for Psychology. It’s a great resource for understanding statistical techniques in psychological research!
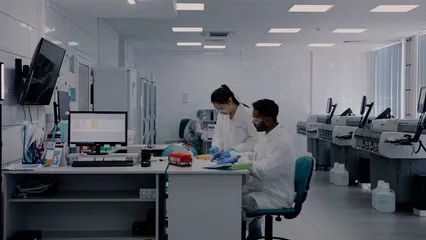
Business and Marketing
In business, correlation analysis is crucial for market research. Companies examine the relationship between advertising spend and sales revenue. If they find a strong positive correlation, they might decide to increase their advertising budget. Understanding consumer behavior also relies on correlation. For instance, businesses can track how customer satisfaction correlates with loyalty.
If you’re looking to enhance your business intelligence capabilities, consider checking out Business Intelligence Guidebook. It provides insights into leveraging data for business success!
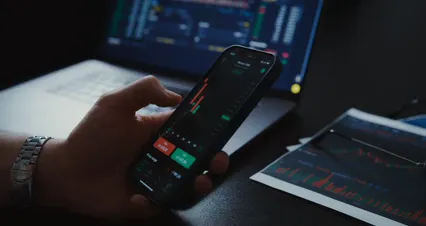
Healthcare and Science
In healthcare, correlation coefficients can reveal important insights. Researchers often study correlations between lifestyle factors and health outcomes. For example, a study might show a negative correlation between physical activity and obesity rates. Such findings can inform public health strategies aimed at reducing obesity.
In scientific research, correlation analysis helps in developing theories. Scientists might explore correlations between environmental factors and species population dynamics. These insights contribute to conservation efforts and ecological understanding. For those interested in a deeper dive into data science, The Data Science Handbook is a must-read!
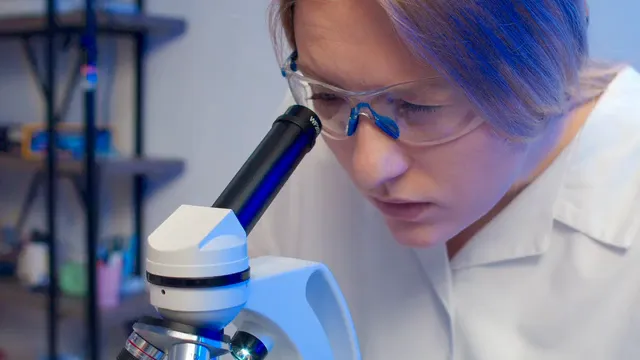
Conclusion
The correlation coefficient is a powerful tool for analyzing relationships between variables. It provides clarity in various fields, from research to business and healthcare. Utilizing correlation analysis can enhance your understanding of data and help inform decisions. For further reading on correlation, you can explore correlation.
Please let us know what you think about our content by leaving a comment down below!
Thank you for reading till here 🙂
All images from Pexels
Introduction
Correlation is a statistical measure that shows relationships between variables. Understanding this concept is essential in data analysis. This article aims to explain how to use a correlation coefficient calculator and its various applications. Grasping correlation is crucial in fields like research, business, and healthcare, as it helps identify trends and make informed decisions.
Understanding correlation is essential for effective data analysis. data analysis
Summary and Overview
The correlation coefficient quantifies the relationship between two variables. Its values range from -1 to 1. A value of 1 indicates a perfect positive correlation, meaning both variables increase together. Conversely, -1 signals a perfect negative correlation, where one variable increases while the other decreases. A value of 0 reflects no correlation.
The correlation coefficient calculator is a valuable tool that helps determine the strength and direction of relationships between data sets. The main types of correlation coefficients include Pearson, Spearman, and Kendall. Each type serves different purposes based on data characteristics.
In the following sections, we will cover the definition and importance of correlation coefficients, how to use the calculator, and practical examples of correlation calculations. Stay tuned for an informative journey through the world of correlation analysis!
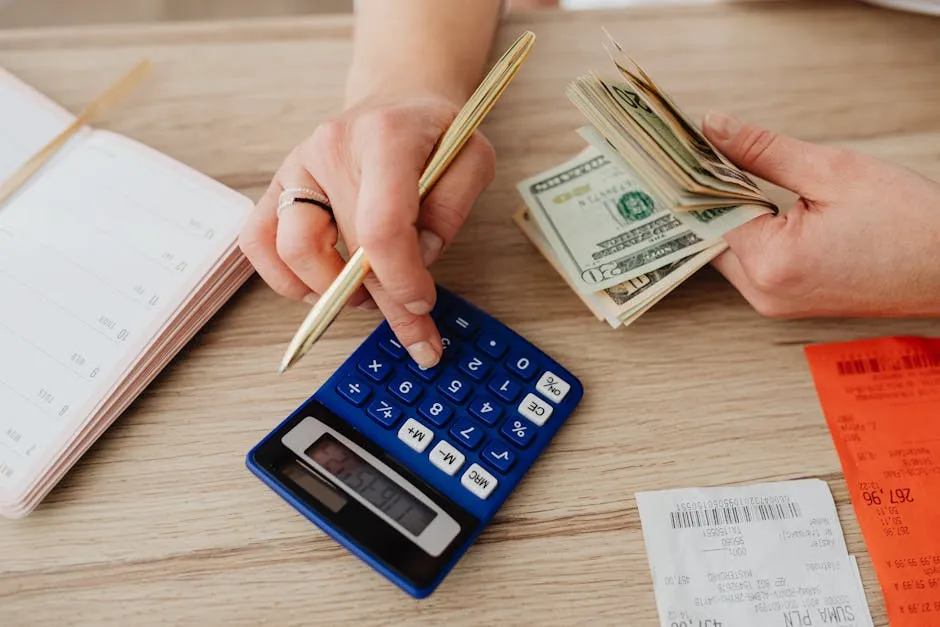
What is the Correlation Coefficient?
Definition and Importance
The correlation coefficient quantifies the relationship between two variables. It indicates how closely changes in one variable relate to changes in another. This statistic is vital in fields like research and data analysis. It helps identify trends and inform decision-making processes.
A correlation coefficient ranges from -1 to 1. A value of 1 implies a perfect positive relationship, while -1 indicates a perfect negative relationship. A value near 0 suggests little or no correlation. Understanding these relationships can reveal insights about various phenomena.
Types of Correlation Coefficients
There are several types of correlation coefficients, each suitable for different data scenarios:
- Pearson’s correlation coefficient: This is the most common type, measuring linear relationships between two continuous variables. It assumes that data is normally distributed and free from outliers. For more information on this, you can check out pearson correlation.
- Spearman’s rank correlation coefficient: This non-parametric measure assesses ordinal data or non-linear relationships. It’s useful when data doesn’t meet the assumptions required for Pearson’s.
- Kendall’s Tau: This coefficient also evaluates ordinal data. It focuses on the strength of the association between two variables by considering the ranks. It’s particularly effective with small sample sizes.
Choosing the right correlation coefficient depends on your data’s nature and the relationship you wish to analyze.
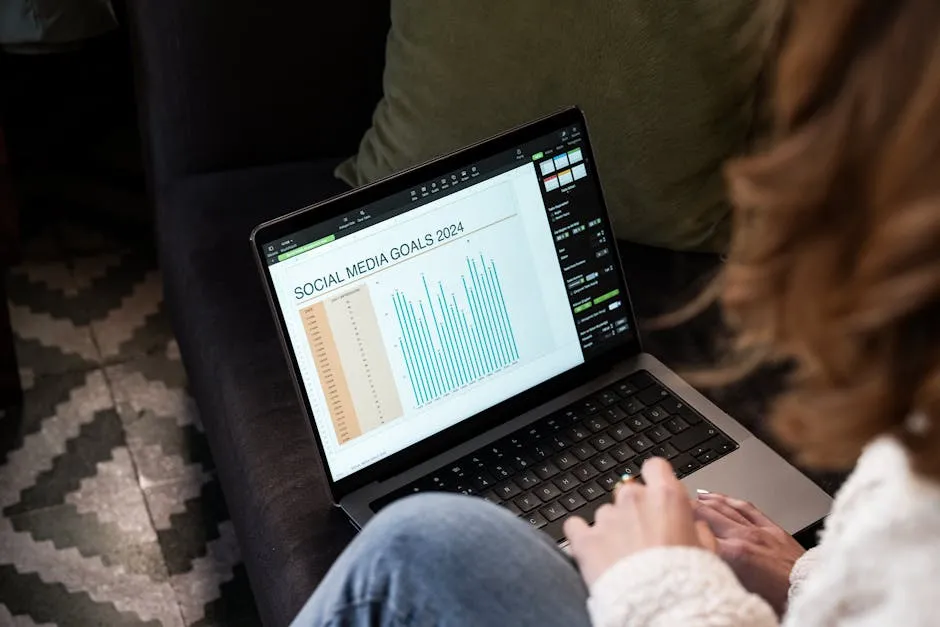
How to Use a Correlation Coefficient Calculator
Step-by-Step Guide
Using a correlation coefficient calculator is straightforward. Here’s how to do it step by step.
- Entering Data: Start by gathering your data sets. You need two lists of numbers, typically referred to as X and Y values. Enter these values into the calculator. You can input them as comma-separated or space-separated values. For example, you might write “10, 20, 30” for X values and “5, 15, 25” for Y values.
- Initiating the Calculation: Once your data is in place, look for the button labeled “Calculate” or “Compute.” Click this button to start the calculation process. The calculator will analyze your data and compute the correlation coefficient.
- Interpreting Results: After the calculation is complete, the calculator will display the correlation coefficient value. This value indicates the strength and direction of the relationship between your two variables. A value close to 1 suggests a strong positive relationship, while a value near -1 indicates a strong negative relationship. A value around 0 implies little to no correlation.
Understanding these results can help you make data-driven decisions in your research or projects.

Correlation Coefficient Formula
Pearson’s Correlation Formula
The formula to calculate Pearson’s correlation coefficient r is:
r = \frac{n(\sum xy) - (\sum x)(\sum y)}{\sqrt{[n\sum x^2 - (\sum x)^2][n\sum y^2 - (\sum y)^2]}}
In this formula:
- n is the number of data pairs.
- ∑xy is the sum of the product of paired scores.
- ∑x and ∑y are the sums of the X and Y scores, respectively.
- ∑x² and ∑y² are the sums of the squares of the X and Y scores.
Each component helps in determining how closely related the two sets of data are.
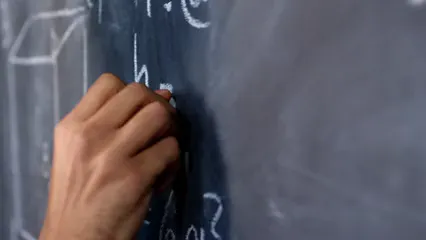
Alternative Formulas
In addition to Pearson’s formula, there are alternative methods to calculate correlation:
- Spearman’s Rank Correlation: This is used for ordinal data or non-linear relationships. It ranks data points and assesses how well the relationship between two variables can be described using a monotonic function.
- Kendall’s Tau: This is another method for ordinal data. It measures the strength of the association between two variables by considering the ranks of the data. It’s particularly useful for small sample sizes.
Choose the appropriate formula based on the nature of your data and the relationships you wish to investigate.
Practical Examples of Correlation Coefficient Calculations
Example 1: Positive Correlation
Let’s consider the relationship between hours studied and exam scores. Here’s a sample dataset:
Hours Studied (X) | Exam Score (Y) |
---|---|
1 | 50 |
2 | 60 |
3 | 70 |
4 | 80 |
5 | 90 |
To calculate the correlation coefficient, we use Pearson’s formula. First, we find the means:
– Mean of X = (1+2+3+4+5)/5 = 3
– Mean of Y = (50+60+70+80+90)/5 = 70
Next, we calculate the covariance and standard deviations.
- Covariance:
cov(X,Y) = \frac{(1-3)(50-70)+(2-3)(60-70)+(3-3)(70-70)+(4-3)(80-70)+(5-3)(90-70)}{n} = 40
- Standard Deviations:
s_x = \sqrt{\frac{(1-3)^2+(2-3)^2+(3-3)^2+(4-3)^2+(5-3)^2}{5}} = \sqrt{2} \approx 1.41
s_y = \sqrt{\frac{(50-70)^2+(60-70)^2+(70-70)^2+(80-70)^2+(90-70)^2}{5}} = \sqrt{400} = 20
Finally, we plug these into the Pearson correlation formula:
r = \frac{cov(X,Y)}{s_x \cdot s_y} = \frac{40}{1.41 \cdot 20} \approx 1.42
The resulting correlation coefficient indicates a strong positive correlation. If you want to dive deeper into statistical concepts, consider picking up Statistics for Dummies. It’s a great resource for beginners!
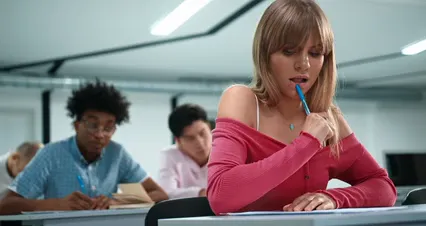
Example 2: Negative Correlation
Now, let’s explore a negative correlation using temperature and hot chocolate sales. Here’s the dataset:
Temperature (°F) | Hot Chocolate Sales (Y) |
---|---|
30 | 100 |
40 | 80 |
50 | 60 |
60 | 30 |
70 | 10 |
Calculating the means:
– Mean of X = (30+40+50+60+70)/5 = 50
– Mean of Y = (100+80+60+30+10)/5 = 58
Next, we find the covariance and standard deviations.
- Covariance:
cov(X,Y) = \frac{(30-50)(100-58)+(40-50)(80-58)+(50-50)(60-58)+(60-50)(30-58)+(70-50)(10-58)}{n} = -700
- Standard Deviations:
s_x = \sqrt{\frac{(30-50)^2+(40-50)^2+(50-50)^2+(60-50)^2+(70-50)^2}{5}} = \sqrt{200} \approx 14.14
s_y = \sqrt{\frac{(100-58)^2+(80-58)^2+(60-58)^2+(30-58)^2+(10-58)^2}{5}} = \sqrt{1600} = 40
Now, applying the formula:
r = \frac{-700}{14.14 \cdot 40} \approx -1.24
This coefficient indicates a strong negative correlation. If you’re looking to enhance your data analysis skills, check out Data Science for Business. It provides a great perspective on applying data science techniques in real-world scenarios!
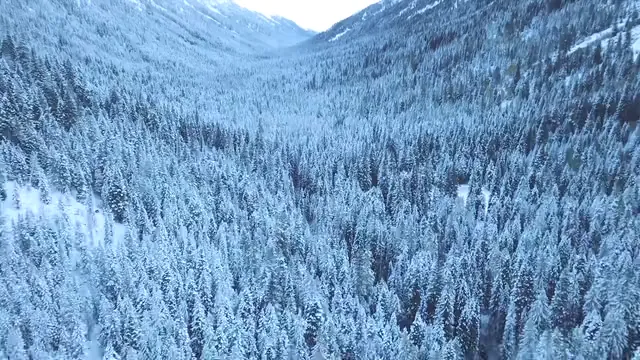
Example 3: No Correlation
Lastly, let’s examine a dataset with no correlation, like shoe size and intelligence score:
Shoe Size (X) | Intelligence Score (Y) |
---|---|
5 | 100 |
6 | 110 |
7 | 105 |
8 | 95 |
9 | 102 |
Calculating the means:
– Mean of X = (5+6+7+8+9)/5 = 7
– Mean of Y = (100+110+105+95+102)/5 = 102.4
Next, we compute the covariance and standard deviations.
- Covariance:
cov(X,Y) = \frac{(5-7)(100-102.4)+(6-7)(110-102.4)+(7-7)(105-102.4)+(8-7)(95-102.4)+(9-7)(102-102.4)}{n} = 0
- Standard Deviations:
s_x = \sqrt{\frac{(5-7)^2+(6-7)^2+(7-7)^2+(8-7)^2+(9-7)^2}{5}} = \sqrt{2} \approx 1.41
s_y = \sqrt{\frac{(100-102.4)^2+(110-102.4)^2+(105-102.4)^2+(95-102.4)^2+(102-102.4)^2}{5}} \approx 4.47
Finally, the correlation coefficient is:
r = \frac{0}{1.41 \cdot 4.47} = 0
This indicates no correlation between shoe size and intelligence. If you want to sharpen your statistical skills further, consider grabbing a copy of Practical Statistics for Data Scientists. It’s an excellent resource for understanding practical applications of statistics!

Interpreting the Results
Understanding the Value of the Correlation Coefficient
The correlation coefficient ranges from -1 to 1. A value of 1 indicates a perfect positive correlation, meaning both variables increase together. Conversely, -1 indicates a perfect negative correlation, where one variable increases as the other decreases. A value around 0 suggests little to no correlation, indicating no linear relationship exists.
In practical terms, a strong positive correlation means that if you know one variable, you can predict the other with confidence. For instance, in the positive correlation example, knowing how many hours a student studied helps predict their exam score. A strong negative correlation, like temperature and hot chocolate sales, suggests that as one variable increases, the other decreases, which can inform business strategies. Understanding these correlations is critical for making informed decisions based on data.

Common Misinterpretations
When discussing correlation, many people confuse it with causation. Just because two variables show a correlation, it doesn’t mean one causes the other. For instance, imagine a study finds that ice cream sales go up in summer. At the same time, drowning incidents increase. This doesn’t imply that buying ice cream causes drownings. Instead, both are influenced by warmer weather.
Another common misunderstanding is the idea that a strong correlation means a perfect relationship. A correlation coefficient of 0.9 suggests a strong positive correlation. It means that as one variable increases, the other tends to do so too. However, this doesn’t mean they move together perfectly. There can still be deviations.
People also think a correlation of zero means no relationship at all. While it indicates no linear relationship, there could still be a non-linear connection. For example, the relationship between the amount of time spent studying and test scores might not be linear.
Understanding these nuances is vital for accurately interpreting data. Always look deeper to see the full picture behind the numbers.
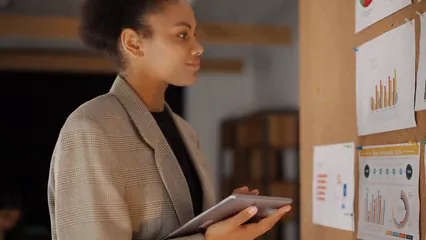
Applications of Correlation Coefficient in Various Fields
Research and Academia
Researchers frequently apply correlation coefficients to analyze data. They use these coefficients to identify relationships between variables, helping to validate hypotheses. For instance, a study might investigate the correlation between study hours and exam performance. A strong positive correlation could support the idea that more study time leads to better grades.
If you’re looking for a comprehensive guide on statistical methods, consider Statistical Methods for Psychology. It’s a great resource for understanding statistical techniques in psychological research!
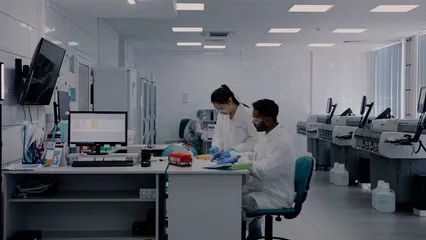
Business and Marketing
In business, correlation analysis is crucial for market research. Companies examine the relationship between advertising spend and sales revenue. If they find a strong positive correlation, they might decide to increase their advertising budget. Understanding consumer behavior also relies on correlation. For instance, businesses can track how customer satisfaction correlates with loyalty.
If you’re looking to enhance your business intelligence capabilities, consider checking out Business Intelligence Guidebook. It provides insights into leveraging data for business success!
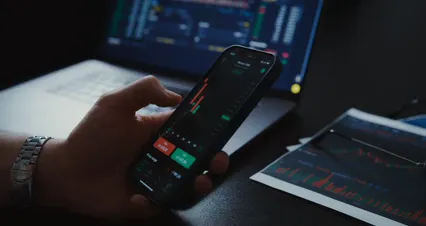
Healthcare and Science
In healthcare, correlation coefficients can reveal important insights. Researchers often study correlations between lifestyle factors and health outcomes. For example, a study might show a negative correlation between physical activity and obesity rates. Such findings can inform public health strategies aimed at reducing obesity.
In scientific research, correlation analysis helps in developing theories. Scientists might explore correlations between environmental factors and species population dynamics. These insights contribute to conservation efforts and ecological understanding. For those interested in a deeper dive into data science, The Data Science Handbook is a must-read!
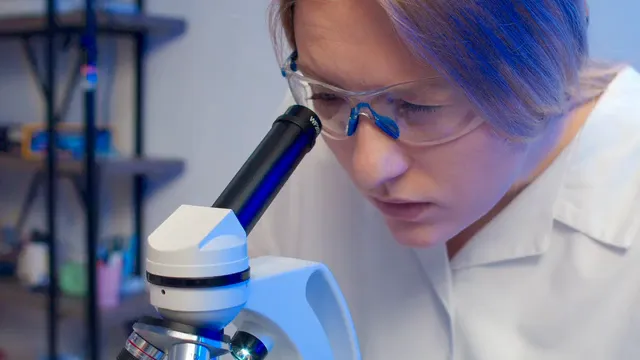
Conclusion
The correlation coefficient is a powerful tool for analyzing relationships between variables. It provides clarity in various fields, from research to business and healthcare. Utilizing correlation analysis can enhance your understanding of data and help inform decisions. For further reading on correlation, you can explore correlation.
Please let us know what you think about our content by leaving a comment down below!
Thank you for reading till here 🙂
All images from Pexels