Introduction
Statistics is the unsung hero of data interpretation. Without it, we’d be lost in a sea of numbers, floundering like a fish out of water. Two of the most important branches in this realm are descriptive and inferential statistics. Both play a vital role in how we understand and analyze data.
Descriptive statistics serve as the first line of defense. They summarize and describe the main features of a dataset. Think of it as a snapshot; it captures the essence of the data without making any predictions or guesses. For instance, it tells us the average score of students in a class or the percentage of customers who prefer chocolate over vanilla.
On the flip side, inferential statistics take us a step further. They allow us to make predictions and generalizations about a larger population based on a sample of data. It’s like peeking into a magic crystal ball and trying to predict the future based on what you see. For example, if we survey 100 people about their favorite ice cream flavor, we can infer the preferences of a much larger group.
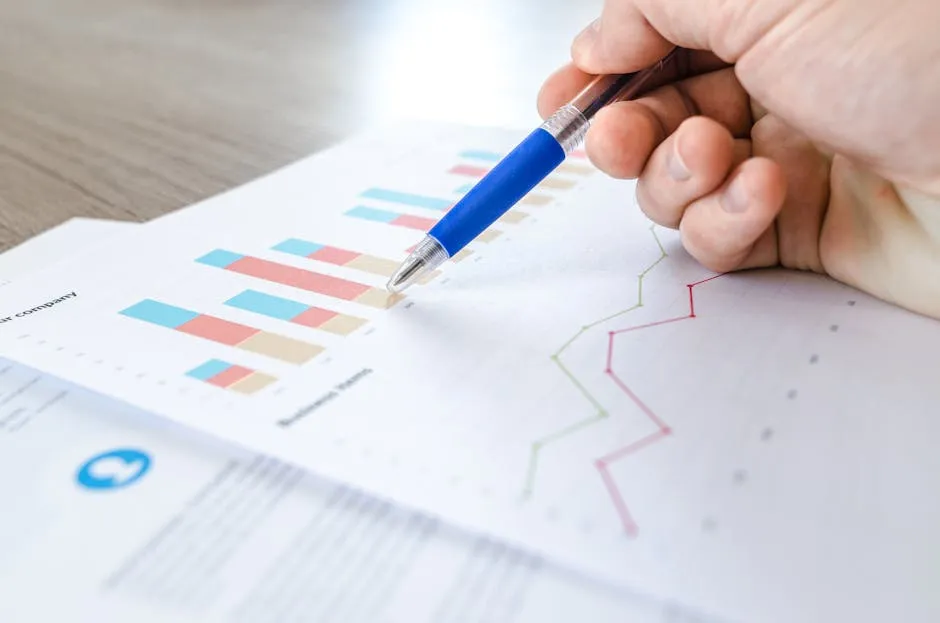
If you’re looking to delve deeper into the world of statistics, grab a copy of Statistics for Dummies. This book breaks down complex concepts into bite-sized pieces, perfect for beginners and seasoned pros alike!
Understanding the differences between these two types of statistics is crucial for effective data-driven decision-making. It empowers researchers, businesses, and even everyday people to draw meaningful conclusions from data. So, buckle up as we navigate through the fascinating world of statistics, where numbers tell stories and data reveals truths!
Understanding Statistics
Definition of Statistics
Statistics is the mathematical science of collecting, analyzing, interpreting, and presenting data. It provides us with tools to understand patterns, make decisions, and draw conclusions based on numerical evidence. Whether in healthcare, business, or social sciences, statistics help professionals tackle complex problems with clarity and precision.
In healthcare, for example, statistics play a vital role in clinical trials, helping researchers determine the effectiveness of new treatments. In business, they guide market research, revealing consumer preferences and trends. Without statistics, making informed decisions would feel like trying to find your way in the dark without a flashlight!
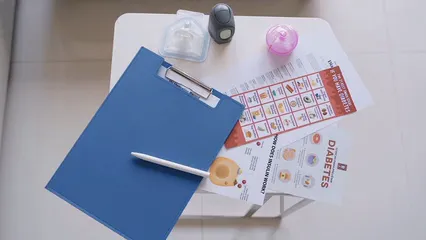
For those looking to enhance their data analysis skills, check out Excel 2021 for Dummies. This guide will help you master Excel’s statistical functions, making data manipulation a breeze!
Types of Statistics
Statistics can be divided into two main categories: descriptive and inferential statistics. Descriptive statistics focus on summarizing and presenting the characteristics of a dataset. They provide a clear and concise view of the data, helping us understand its basic features.
Inferential statistics, on the other hand, go beyond mere description. They allow us to make predictions and generalizations about a larger population based on sample data. This type of statistics involves hypothesis testing, confidence intervals, and regression analysis, to name a few.
In summary, descriptive statistics provide us with a snapshot of our data, while inferential statistics enable us to make informed predictions and decisions based on that data. Both are essential tools for anyone looking to navigate the world of data analysis effectively.
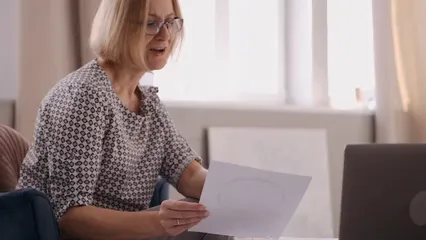
Inferential Statistics
What Are Inferential Statistics?
Inferential statistics is a powerful tool that allows researchers to make predictions about a larger population based on data collected from a sample. Think of it as a crystal ball for data analysis! By analyzing a small group, we can draw conclusions about a larger, often unmeasurable group. This statistical method relies heavily on probability theory to generalize findings from the sample to the broader population.
The importance of inferential statistics cannot be overstated. It plays a crucial role in hypothesis testing, allowing researchers to determine whether their observations are significant or merely due to chance. Imagine testing a new medication; inferential statistics will help ascertain if the results observed in a sample group can be applied to the larger population of patients. This generalization is essential for drawing meaningful conclusions from research findings.
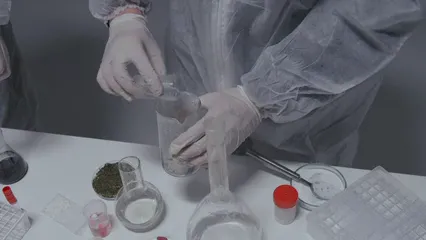
If you’re looking to delve deeper into data analysis techniques, consider picking up SPSS Statistics for Dummies. It’s a fantastic resource for mastering statistical analysis using one of the most popular software packages out there!
Key Techniques in Inferential Statistics
Hypothesis Testing
At the heart of inferential statistics is hypothesis testing. Researchers start by formulating two competing hypotheses: the null hypothesis (H0) and the alternative hypothesis (H1). The null hypothesis typically states that there is no effect or difference, while the alternative suggests that there is. For instance, if we want to know if a new teaching method improves student performance, our null hypothesis might state that it does not lead to any improvement.
Once these hypotheses are established, researchers collect sample data and perform statistical tests, such as t-tests or ANOVA, to determine whether to reject or fail to reject the null hypothesis. The results will help clarify whether any observed differences are statistically significant or simply due to random variation.
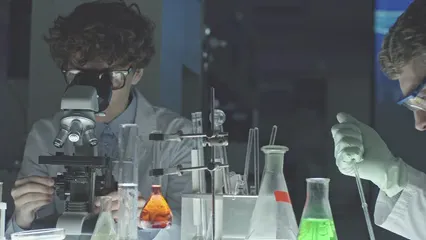
Confidence Intervals
Confidence intervals (CIs) provide a range of values within which we expect the true population parameter to lie. For example, if we calculate a 95% confidence interval for the average height of students in a university, it might range from 170 cm to 180 cm. This means we are 95% confident that the true average height of all students falls within this range.
Confidence intervals are vital because they give a sense of the uncertainty associated with sample estimates. A narrow confidence interval suggests a more precise estimate of the population parameter, while a wider interval indicates more uncertainty.

Regression Analysis
Regression analysis is another key technique in inferential statistics. It examines the relationships between variables, allowing researchers to predict outcomes. For instance, if we want to know how study hours impact exam scores, we can use regression analysis to model this relationship.
By analyzing data, regression helps identify trends and can even assist in making predictions. This technique can uncover valuable insights, such as determining whether increasing study hours leads to better exam performance, thus aiding in effective educational strategies.

Differences Between Descriptive and Inferential Statistics
To clarify the distinctions between descriptive and inferential statistics, here’s a summary comparison:
Aspect | Descriptive Statistics | Inferential Statistics |
---|---|---|
Purpose | Summarizes and describes features of a dataset | Makes inferences and predictions about a population based on sample data |
Data Scope | Focuses on specific sample data | Extends findings to a larger population |
Objective | Describes characteristics of the data without generalizing | Generalizes findings from sample to population |
Examples | Mean, median, mode | Hypothesis testing, regression analysis, confidence intervals |
Statistical Techniques | Central tendency, dispersion measures | T-tests, ANOVA, regression analysis |
Understanding these differences is key to effectively applying statistical methods in research. While descriptive statistics helps summarize the data we have, inferential statistics allows us to make educated guesses about the data we don’t. This combination is essential for making informed decisions and drawing meaningful conclusions in various fields, from healthcare to social sciences.
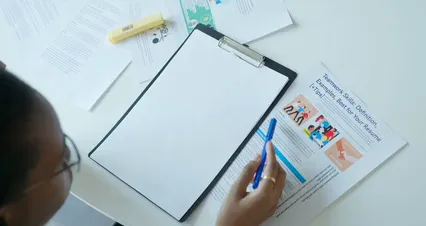
Use Cases in Various Fields
Healthcare
In healthcare, both descriptive and inferential statistics play pivotal roles. Descriptive statistics are employed in clinical trials to summarize patient data. For instance, researchers might report the average age of participants, the proportion of male and female subjects, and the range of health indicators like blood pressure. This summary provides a clear snapshot of the trial population.
Conversely, inferential statistics help in making broader conclusions about treatment effects. By analyzing a sample of patients, researchers can infer how a new drug might affect the entire population. Hypothesis testing and confidence intervals are often used here. For example, if a clinical trial shows that a medication lowers cholesterol levels in a sample, inferential statistics allow healthcare professionals to predict its effectiveness for the larger population.
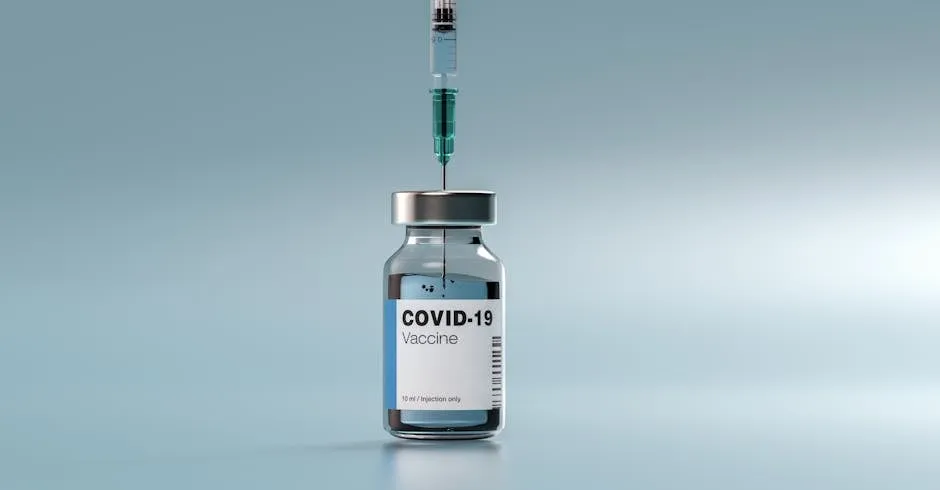
Business
In the business realm, descriptive statistics are invaluable for market research. Companies analyze customer data to understand buying patterns, preferences, and demographics. For example, a retailer might calculate the average purchase amount or the most common items bought. This information helps businesses tailor their marketing strategies effectively.
Inferential statistics, however, take it a step further. Businesses use sampling techniques to predict future sales or customer behavior. If a company surveys a sample of its customers about a new product, it can use inferential statistics to generalize the findings to all customers. This approach assists in decision-making, such as product launches or marketing campaigns, based on data-driven insights.
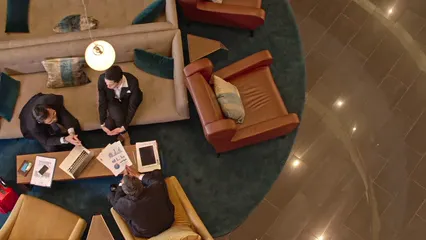
Social Sciences
Social scientists rely heavily on both types of statistics for survey data analysis. Descriptive statistics summarize survey results, providing a clear picture of public opinion on various issues. For example, researchers might report the percentage of respondents who support a specific policy or the average income level within a demographic group.
Inferential statistics help draw conclusions about the broader population based on survey samples. For instance, if a study surveys 1,000 individuals about their voting preferences, inferential statistics allow researchers to make predictions about the entire voting population. Techniques such as regression analysis and confidence intervals are used to understand relationships between variables and to assess the reliability of these predictions.
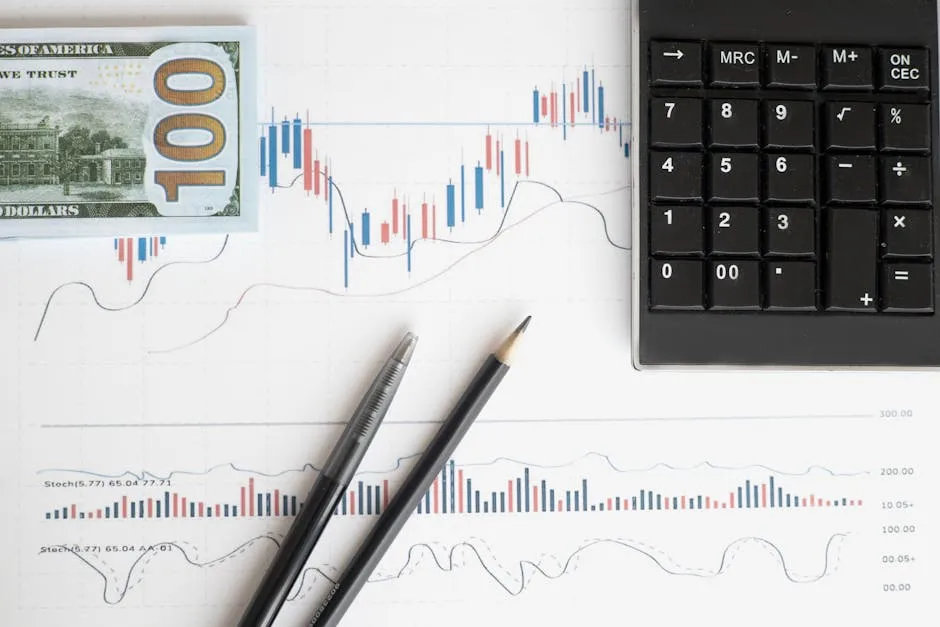
Conclusion
Statistics are the backbone of data analysis. Descriptive and inferential statistics serve distinct roles, yet complement each other in interpreting data. Descriptive statistics provide a concise summary of observed data, making complex information digestible. They highlight key features like central tendency and dispersion, providing insights that are essential for initial data exploration.
On the other hand, inferential statistics empower researchers to make predictions and generalizations about larger populations based on sample data. This branch of statistics introduces concepts like hypothesis testing, confidence intervals, and regression analysis. It allows for conclusions to be drawn, even amidst uncertainty, and provides the tools necessary for making data-driven decisions.
Understanding both types of statistics is crucial for professionals across various fields. In healthcare, they ensure the efficacy of treatments and interventions. In business, they inform market strategies and consumer behavior insights. In social sciences, they reveal public opinions and trends, influencing policy-making and societal understanding.
Final thoughts: statistics are not just about numbers and calculations; they tell stories. By mastering both descriptive and inferential statistics, professionals can harness the power of data to uncover truths, drive innovation, and make informed decisions that impact their fields.
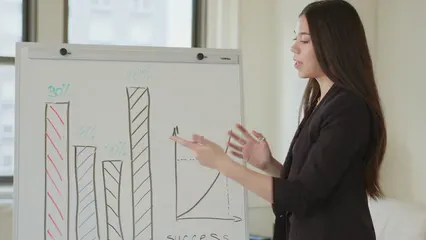
For those who want to dive deeper into the subject, consider grabbing Python for Data Analysis. This book is a fantastic resource for learning how to manipulate data and perform statistical analysis using Python!
FAQs
What is the main difference between descriptive and inferential statistics?
Descriptive statistics summarize characteristics of a dataset, while inferential statistics use sample data to make predictions or generalizations about a larger population. For example, calculating the average height of students in a class (descriptive) versus predicting the height of all students in the school based on that sample (inferential).
When should I use descriptive statistics?
Use descriptive statistics when you need to summarize or describe data that you have collected. For instance, if you want to report the average performance of a group of students on an exam, descriptive statistics like mean, median, and mode are appropriate.
What are some common inferential statistical tests?
Common inferential tests include t-tests, which compare means between two groups; ANOVA, which compares means among three or more groups; and chi-square tests, which assess relationships between categorical variables. These tests help determine if observed differences or relationships are statistically significant.
Can descriptive statistics lead to inferential conclusions?
Yes, descriptive statistics can inform inferential analysis. For instance, understanding the average test score of a sample group can guide predictions about the performance of a larger population. However, it’s essential to consider the limitations of generalizing from descriptive data.
What tools can I use for statistical analysis?
Several software tools facilitate statistical analysis, including Excel for basic calculations, SPSS for comprehensive statistical analysis, R for advanced statistical computing, and Python libraries like Pandas and SciPy for data manipulation and analysis. Each tool has its strengths, depending on the complexity of the data and analysis required.
Can Descriptive Statistics Lead to Inferential Conclusions?
Descriptive statistics, while primarily focused on summarizing data, can indeed pave the way for inferential conclusions. Imagine you have a dataset that outlines the test scores of 100 students. You calculate the average score and standard deviation. This gives you a clear snapshot of how the students performed. But what if you want to infer how this performance might reflect the entire school population?
Here’s where the magic happens! By employing the descriptive statistics you’ve gathered, you can make educated guesses about the broader population. For instance, if your sample’s mean score is significantly high, you might infer that the overall student body also performs well, provided the sample was appropriately random and representative. It’s like reading the first few pages of a book and guessing the plot without knowing the ending.
However, while descriptive stats can inform inferential analysis, remember, they can’t substitute for it. You need to ensure that your sample is large enough and representative enough to avoid misleading conclusions. So, in summary, descriptive statistics serve as the sturdy foundation upon which inferential conclusions can be built, provided you tread carefully!

To delve deeper into the concepts of descriptive and inferential statistics, check out the problem with inferential statistics.
What Tools Can I Use for Statistical Analysis?
When it comes to statistical analysis, having the right tools is half the battle won! Let’s explore some popular software options that can help you crunch those numbers like a pro.
1. Excel: This trusty spreadsheet software is often the first stop for many. With functions for basic statistics and data visualization techniques, it’s user-friendly for beginners.
2. SPSS: Short for Statistical Package for the Social Sciences, SPSS is a powerhouse for data analysis. It’s widely used in social sciences and healthcare research, offering a suite of statistical tests and graphical options.
3. R: If you’re looking for flexibility and power, R is your friend. This programming language is fantastic for statistical computing and graphics. With a vast array of packages, it can handle everything from simple calculations to complex modeling.
4. Python Libraries: Python isn’t just for web development! Libraries like Pandas for data manipulation, NumPy for numerical data, and SciPy for scientific computing make Python a formidable tool for statistical analysis.
5. MATLAB: This high-level language is extensively used for mathematical computations, including statistics. It offers rich toolboxes for data analysis and visualization.
6. SAS: Known for its advanced analytics, SAS provides a comprehensive suite for statistical analysis, data management, and predictive analytics.
7. Stata: This software is particularly strong in econometrics and is favored by researchers in economic and social sciences for its user-friendly interface and powerful statistical capabilities.
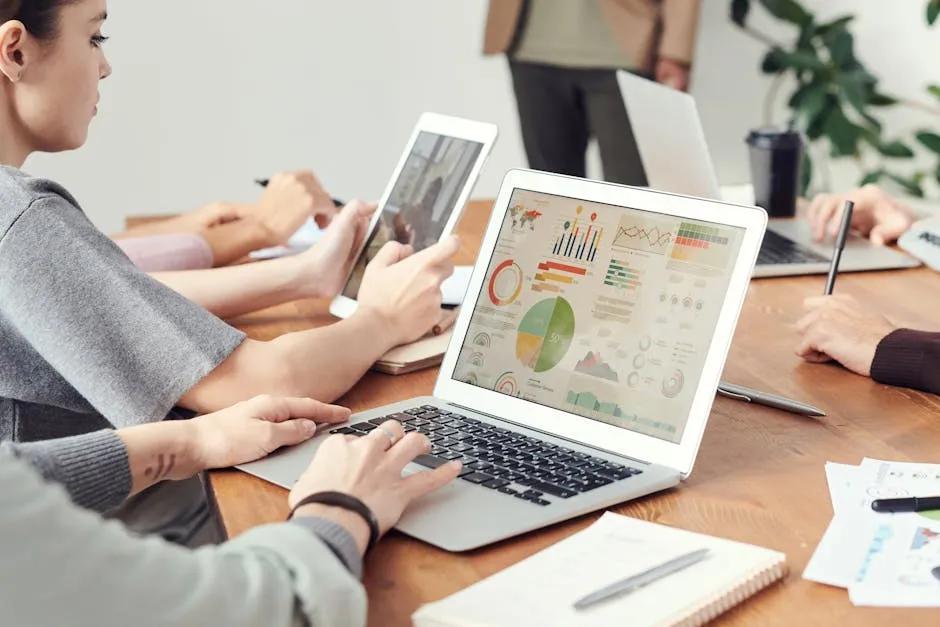
No matter your choice, remember, the best tool is the one that fits your needs and level of expertise. With these at your disposal, you’re well on your way to mastering statistical analysis like a seasoned data wizard!
And if you’re on the lookout for reliable statistical software, consider Statistical Analysis Software. It can help streamline your analysis process and provide you with in-depth insights!
Additional Resources
For those eager to dive deeper into the world of statistics, here are some excellent resources:
Books: “Statistics for Dummies” is a great starting point for beginners. For a more advanced read, try “The Elements of Statistical Learning.” You can find it here.
Online Courses: Websites like Coursera and edX offer courses in statistics ranging from beginner to advanced levels.
Tutorials: Khan Academy provides free tutorials on basic statistics.
Statistical Software Documentation: Each software package mentioned has extensive documentation and user forums to help you troubleshoot and learn.
For those who enjoy jotting down notes, consider a Notebook for Statistics Notes. It’s perfect for organizing your thoughts and findings!
Please let us know what you think about our content by leaving a comment down below!
Thank you for reading till here 🙂
All images from Pexels