Introduction
Statistical distributions play a crucial role in agriculture. They’re like the weather forecast for data – helping farmers know what to expect. When it comes to crop yields, livestock health, and resource management, statistical methods help make sense of data, turning confusion into clarity.
In agriculture, understanding productivity is essential. Farmers need to predict how much they’ll harvest. They also need to assess environmental impacts and manage resources wisely. This is where statistical distributions come in handy. They provide a framework for analyzing variations in crop yields and livestock growth, revealing patterns that can shape agricultural practices.
This article will cover the importance of statistical distributions in agriculture. We’ll explore agricultural statistics, types of data collected, and how statistical analysis aids farmers, researchers, and policymakers. Get ready to uncover how these distributions influence decision-making in agriculture, from yield predictions to resource allocation.

Understanding Agricultural Statistics
What Are Agricultural Statistics?
Agricultural statistics encompass data related to farming practices. They include information about crop production, livestock numbers, land use, and economic factors. Essentially, these statistics are the heartbeat of agriculture. They help track trends and support informed decision-making.
The types of data collected are vast. For instance, crop yields tell farmers how much they can expect to harvest. Livestock numbers inform about animal husbandry practices. These statistics are significant as they can influence everything, from market prices to food security policies.

Importance of Statistical Analysis in Agriculture
Statistical analysis is vital for farmers, researchers, and policymakers. Why? Because it transforms raw data into actionable insights. For example, analyzing crop yield data helps farmers make informed decisions about planting and harvesting.
Take yield predictions: statistical models help forecast how much a farmer might produce based on historical data and environmental factors. This information is essential for planning and resource allocation. Without statistical analysis, decisions would be based on guesswork, which can lead to wasted resources and lost profits.
Moreover, policymakers rely on agricultural statistics to develop effective policies. They need to understand trends in food production and consumption to address issues like food security. Thus, statistical analysis is not just useful; it’s essential for the sustainability and efficiency of agricultural practices.
By understanding agricultural statistics and their importance, stakeholders can enhance productivity and resilience in the agricultural sector. The next sections will dive deeper into key statistical concepts and distributions, enriching your understanding of their applications in agriculture.
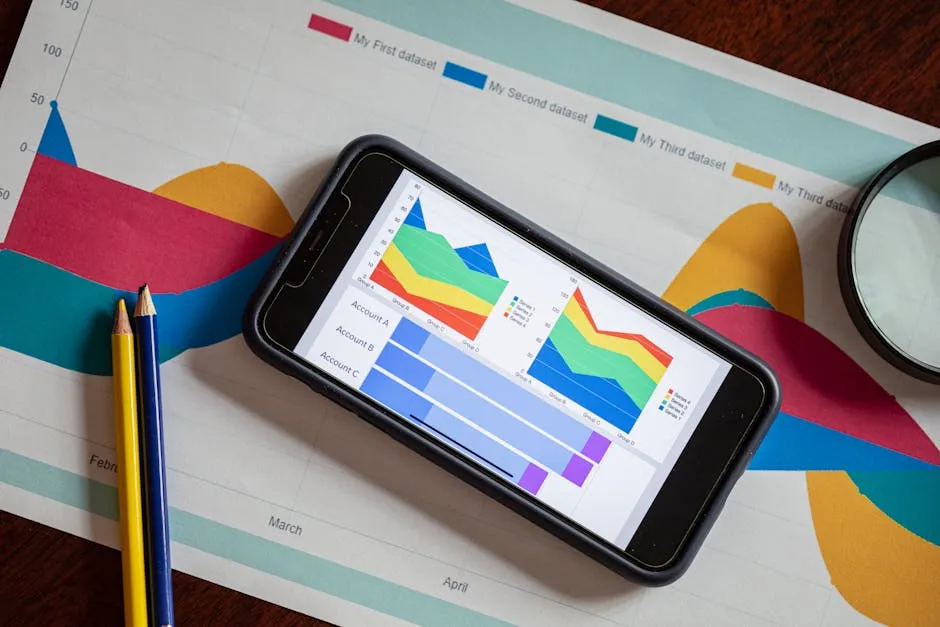
Key Statistical Concepts
Measures of Central Tendency
Understanding statistical measures is like knowing the score in a game. The mean, median, and mode are key players here.
Mean: This is the average. To find it, add all the values and divide by the number of values. For instance, if a farmer wants to know the average yield of corn over five years, they would sum up the yields and divide by five.
Median: This is the middle number in a sorted list. Imagine a farmer has yields of 30, 40, 50, and 60 bushels. The median would be 45 bushels, as it’s right in the center. This is useful for understanding the typical yield without being swayed by extreme values.
Mode: This is the most frequently occurring number. If a farmer records yields of 30, 30, 40, and 50 bushels, the mode is 30. It helps to see which yield is most common, which can assist in planning for future harvests.

Variability and Distribution
Now, let’s talk about variance and standard deviation. These terms sound complex, but they’re simply ways to understand how much the data varies.
Variance: This measures how spread out the data points are around the mean. If all yields are close to the average, variance is low. But if some yields are way higher or lower, variance increases.
Standard Deviation: This is the square root of variance and provides insight into how much individual yields differ from the average. A small standard deviation means most yields are similar, while a large one indicates variability. For instance, if a farmer records yields of 20, 30, and 80 bushels, the standard deviation will be high, indicating inconsistency.
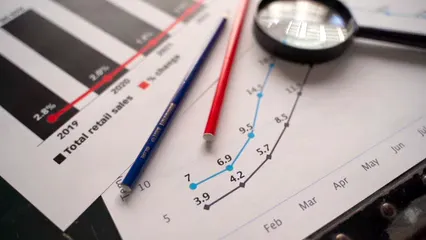
Correlation and Regression
These concepts help farmers analyze relationships between different variables.
Correlation: This shows whether two variables move together. For example, does an increase in fertilizer use lead to higher crop yields? A positive correlation means as one goes up, so does the other.
Regression: This is a statistical method for predicting the value of one variable based on another. For instance, a farmer might use regression analysis to predict future yields based on historical data and factors like rainfall or soil quality. By understanding these relationships, farmers can make informed decisions, enhancing productivity and efficiency.
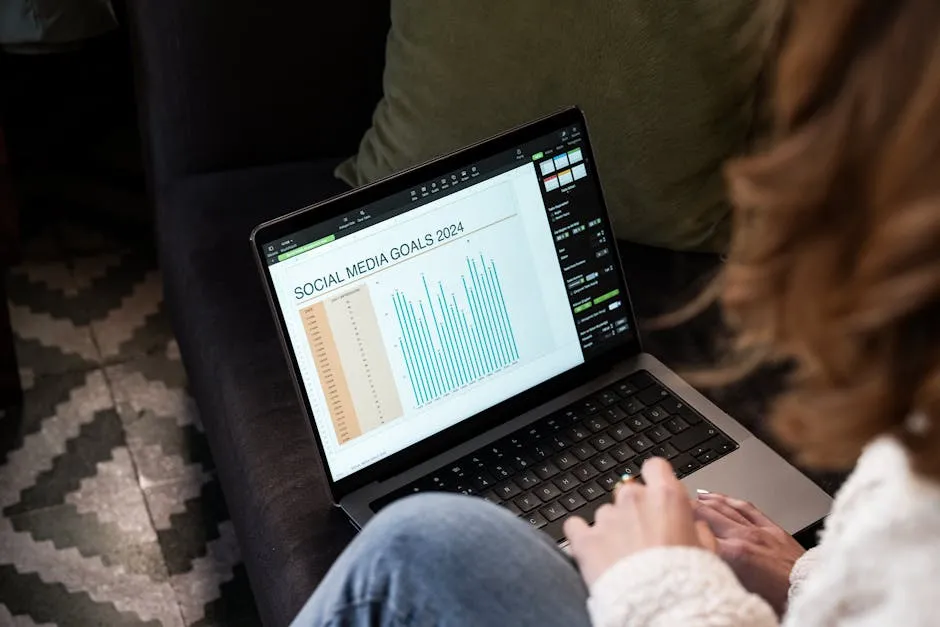
These key statistical concepts lay the groundwork for understanding agricultural data. They provide insights into yields, variability, and relationships between different agricultural factors, equipping stakeholders with the knowledge to make informed decisions.
Statistical Distributions in Agriculture
Binomial Distribution in Agricultural Research
The binomial distribution is all about success and failure. It helps researchers understand scenarios with two possible outcomes. Imagine a farmer observing pest infestations: they might either have a successful crop or face a failure due to pests. In agricultural studies, this distribution is crucial. It allows researchers to model probabilities and outcomes effectively.
For instance, let’s say a farmer sprays pesticide on a crop. The outcome can be categorized as either a successful pest control or a failure. Using binomial distribution, researchers can analyze the likelihood of success after several trials. This analysis helps farmers make informed decisions about pest management strategies, ultimately enhancing crop yields.

Speaking of pest control, have you considered using eco-friendly pest control products? They are great for managing pests without harming beneficial insects, making them a fantastic choice for sustainable farming practices.
Poisson Distribution and Its Applications
Now, let’s talk about the Poisson distribution. This one’s for the rare events – like counting how many times a particular crop disease outbreak occurs in a season. It’s particularly helpful in agriculture, where pest or disease occurrences can be sporadic.
Take a tomato farm, for example. If a farmer wants to know how often disease outbreaks happen, they can use Poisson distribution to model these occurrences. By analyzing historical data on disease outbreaks, farmers can predict the probability of future outbreaks. This understanding can lead to better planning and resource allocation, ensuring crops remain healthy.
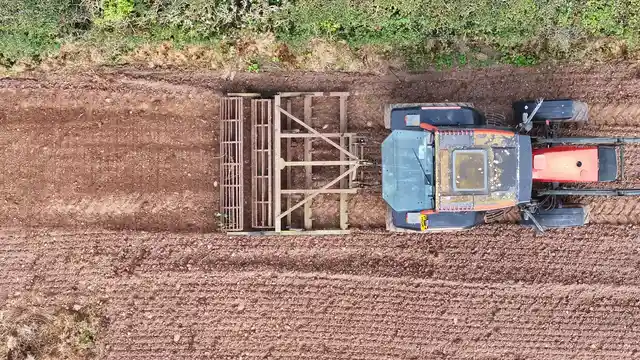
Other Relevant Distributions
Besides binomial and Poisson distributions, several other distributions play a role in agricultural research. The exponential distribution helps model time until an event occurs, like the time between pest infestations. It’s vital for understanding and predicting pest management needs.
Then, there’s the geometric distribution, which is useful for modeling the number of trials until the first success. For example, a farmer might want to know how many attempts it takes before a new crop variety successfully germinates.
Case studies illustrate these distributions in action. In one instance, researchers applied exponential distribution to study the frequency of drought occurrences, influencing irrigation strategies. In another, geometric distribution helped evaluate the success rate of different planting techniques, guiding farmers toward more effective practices.
By understanding these statistical distributions, agricultural researchers and farmers can make data-driven decisions, improving productivity and sustainability. Each distribution provides unique insights, helping tackle challenges in modern agriculture.
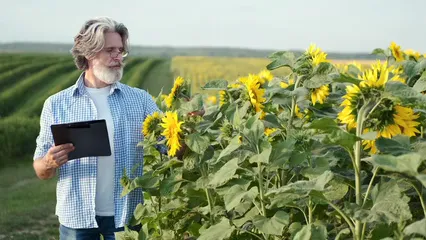
Tools for Statistical Analysis
In the world of agriculture, data is more than just numbers; it’s a lifeline. To make sense of this data, farmers and researchers often turn to statistical software. Enter the champions of data analysis: R, Python, and SAS.
R is like the Swiss Army knife of statistical computing. It’s open-source, meaning it’s free for everyone. You can perform sophisticated analyses and create stunning visualizations. Plus, the R community is vast, offering endless packages tailored for agricultural needs.
Python, on the other hand, is the cool kid on the block. With libraries like Pandas and NumPy, it handles data manipulation with style. Want to build machine learning models to predict crop yields? Python’s got you covered. It’s user-friendly and integrates well with other technologies, making it a favorite among tech-savvy farmers.
Then there’s SAS. This tool is the heavyweight champion in the world of data analytics. It excels in advanced analytics and data management. While it may come with a price tag, its capabilities in handling large datasets make it a worthy investment for serious agricultural research.
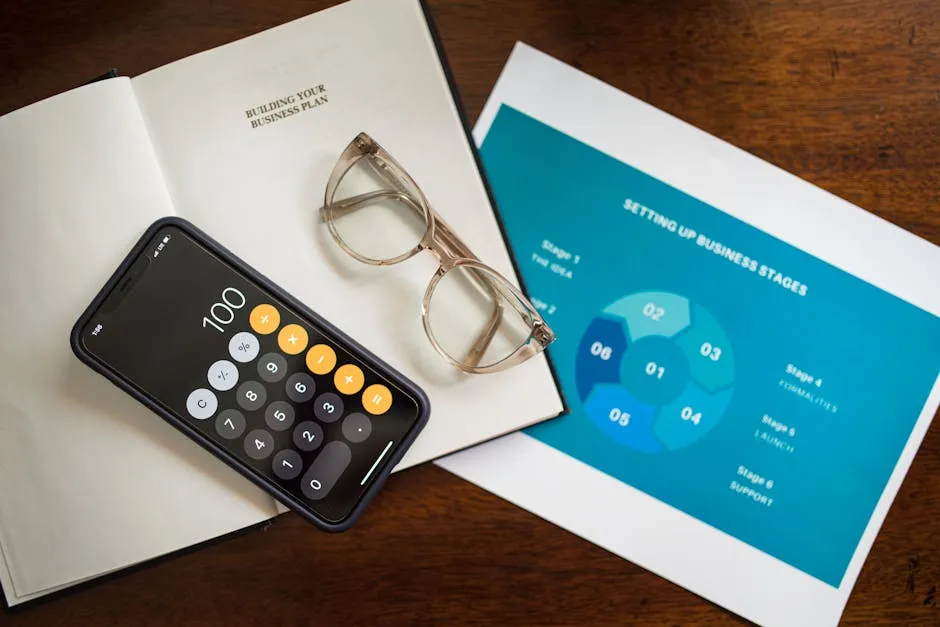
Using these tools has its perks. They allow for quick data analysis, leading to faster decision-making. Imagine being able to assess crop health or predict yields in real-time! This agility can mean the difference between a bumper harvest and a disappointing yield. So, whether you’re a farmer or a researcher, embracing these statistical tools can transform data into actionable insights.
Case Studies: Practical Applications of Statistical Distributions
Statistical distributions aren’t just academic theories; they have real-world implications in agriculture. Let’s look at how they’ve shaped practices.
One notable case study involved analyzing crop yields using the normal distribution. A group of researchers studied corn yields over several years in Iowa. By modeling the yields, they discovered that most fell within a predictable range around the average. This insight helped farmers optimize their planting strategies and manage expectations for harvests.
In another instance, a study on pest control utilized the binomial distribution. Researchers examined the effectiveness of a new pesticide. They categorized the results into successes (pests controlled) and failures (pests remaining). By applying binomial analysis, they accurately predicted the likelihood of pest outbreaks, allowing farmers to implement targeted pest management strategies.
Lastly, consider the Poisson distribution in disease outbreak analysis. A tomato farm in California used this distribution to model the occurrence of a rare disease. By analyzing historical data, they could predict how often outbreaks might occur. This foresight enabled farmers to take preventive measures, reducing the impact of potential losses.
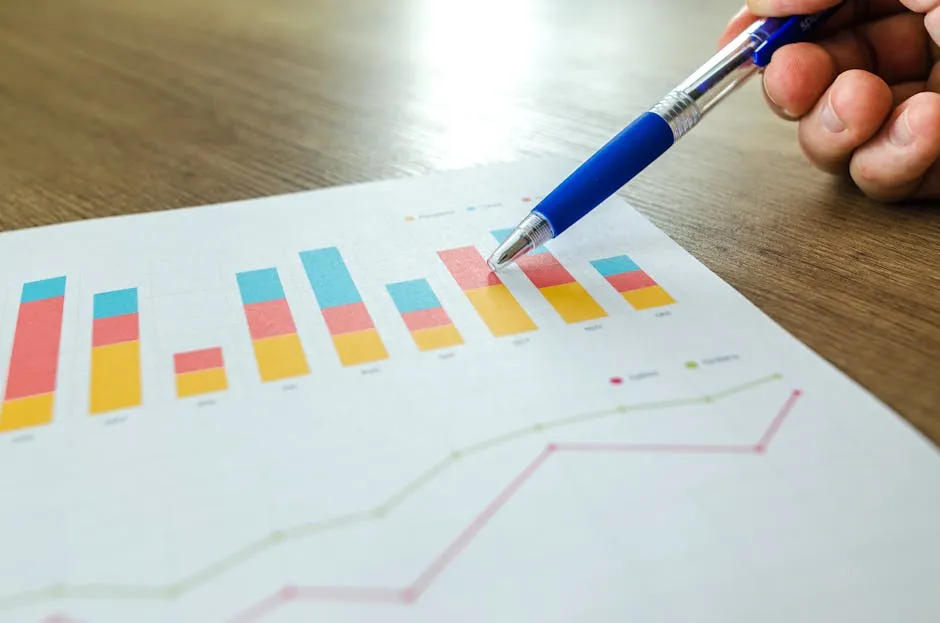
These case studies highlight the power of statistical distributions in agriculture. They guide decision-making, improve resource management, and ultimately lead to more sustainable farming practices. By leveraging these insights, farmers can navigate the unpredictable world of agriculture with confidence.
Applications in Sustainable Agriculture
Statistical distributions are the unsung heroes of sustainable agriculture. They help farmers make informed decisions that benefit the environment and their bottom line. Imagine trying to grow crops without knowing how much rain to expect. That’s where statistics come in.
One way statistical distributions contribute to sustainability is through resource conservation. For instance, normal distribution helps farmers understand the average yield of crops under varying conditions. By analyzing historical data, farmers can predict yields more accurately. This allows them to allocate resources like water and fertilizers more efficiently. Instead of overwatering or over-fertilizing, they can apply just the right amount, reducing waste and environmental impact.
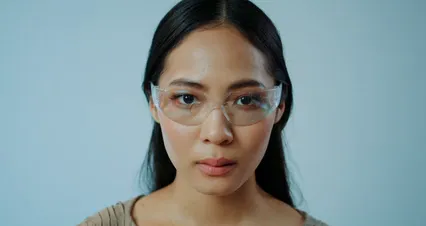
Additionally, data analytics shines a light on environmental protection. Consider the binomial distribution, which models success and failure rates. By using this, farmers can assess the effectiveness of pest control methods. For instance, a farmer might find that a certain pesticide has a 70% success rate in controlling pests in one area but only 40% in another. This insight helps them choose the most effective approach for each field, minimizing pesticide use and potential harm to beneficial insects.
Moreover, statistical analysis supports precision agriculture. With the help of data collected from IoT devices, farmers can monitor soil moisture levels and nutrient content in real-time. By applying statistical methods, they can determine the optimal times for irrigation and fertilization. This approach not only conserves water but also enhances soil health, promoting sustainable farming practices.
And speaking of precision, a Soil Moisture Sensor can be a game-changer. It allows farmers to monitor moisture levels, ensuring that crops receive just the right amount of water, which is crucial for yield optimization.
In conclusion, statistical distributions are more than just numbers; they’re essential tools for building sustainable agricultural practices. From resource conservation to environmental protection, data analytics fosters smarter decisions that embrace both productivity and ecological balance.
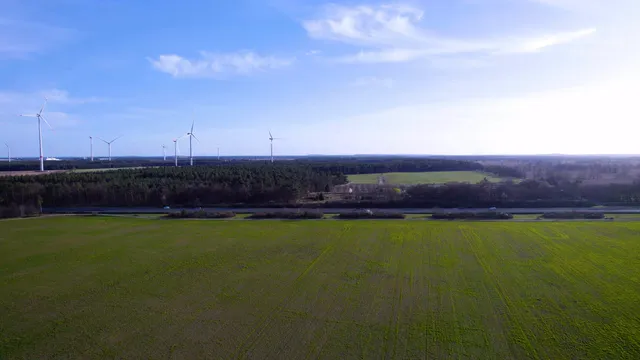
FAQs
What is the role of statistics in agriculture?
Statistics play a pivotal role in improving agricultural efficiency and sustainability. They allow farmers to make informed decisions based on data analysis. By collecting and analyzing data on crop yields, livestock health, and environmental conditions, farmers can optimize their practices. This leads to better resource management, reduced waste, and enhanced productivity. Additionally, statistics help policymakers understand trends and develop effective policies to address food security and environmental challenges.
How do different statistical distributions apply to agricultural research?
Different statistical distributions serve various purposes in agricultural research. The normal distribution, for example, helps model the average yield of crops, capturing the natural variations in data. The binomial distribution is useful for scenarios with two outcomes, such as success or failure in pest control methods. The Poisson distribution assists in understanding the occurrence of rare events, like disease outbreaks. Each distribution provides distinct insights that aid in making data-driven decisions in agriculture.
Why is data accuracy crucial in agricultural statistics?
Data accuracy is paramount in agricultural statistics because it directly impacts the reliability of statistical analyses. Inaccurate data can lead to misguided decisions, resulting in wasted resources and reduced productivity. For example, if yield data is misreported, farmers may overestimate or underestimate their production capacity. This can affect everything from planting decisions to market strategies. Therefore, ensuring data accuracy is essential for making informed choices that enhance agricultural efficiency and sustainability.
What tools can be used for agricultural data analysis?
Several tools are available for agricultural data analysis. Popular statistical software includes R and Python, both of which offer powerful libraries for data manipulation and visualization. R is particularly favored for its extensive statistical packages, while Python is known for its ease of use. Additionally, software like SAS and SPSS provides robust analytics capabilities. Farmers can also use tools like Excel for basic data analysis and visualization. These tools empower stakeholders to analyze data effectively and make informed decisions.
How can agricultural statistics contribute to environmental sustainability?
Agricultural statistics contribute significantly to environmental sustainability by providing insights into resource management and ecological impacts. By analyzing data on water usage, crop yields, and soil health, farmers can adopt practices that minimize waste and conserve resources. For example, statistical analysis can reveal the optimal irrigation levels needed for crops, reducing water consumption. Furthermore, understanding the relationship between agricultural practices and environmental factors helps mitigate negative impacts, promoting sustainable farming practices that benefit both the environment and agricultural productivity.
Conclusion
In this exploration of statistical distributions relevant to agriculture, we’ve uncovered their profound impact on enhancing productivity and sustainability. Statistics are like the compass guiding farmers through the often-choppy waters of agricultural decision-making. By mastering these tools, stakeholders can navigate uncertainties and maximize their resources.
We began by understanding the role of statistical distributions in managing agricultural statistics. By analyzing crop yields, livestock numbers, and environmental impacts, farmers can identify trends and optimize practices. The importance of accurate data collection cannot be overstated. It serves as the foundation for meaningful statistical analysis, which ultimately informs better decision-making.
The various types of statistical distributions, including normal, binomial, and Poisson, offer unique insights. Normal distribution helps farmers grasp the typical variations in crop yields. The binomial distribution aids in evaluating pest control success rates, while the Poisson distribution models rare events like crop disease outbreaks. Each distribution provides valuable data that helps farmers adapt their strategies.
Moreover, the applications of statistical analysis extend beyond individual farms. Policymakers rely on agricultural statistics to develop effective policies. This is particularly crucial in addressing food security, resource management, and environmental sustainability. By leveraging data, policymakers can make informed decisions that benefit the entire agricultural sector.
In our fast-paced world, embracing statistical analysis is no longer optional; it’s essential for success. Farmers and researchers alike must harness these insights to improve agricultural practices. Statistical distributions empower them to make data-driven decisions, ultimately enhancing productivity while safeguarding the environment.
So, if you’re in agriculture, remember this: statistics aren’t just numbers; they’re your allies. Embrace them to transform your farming practices, optimize your resources, and contribute to a more sustainable future. By leveraging statistical analysis, you can ensure that your decisions are informed, effective, and beneficial for both your farm and the planet.
Please let us know what you think about our content by leaving a comment down below!
Thank you for reading till here 🙂
For a deeper understanding of statistical concepts, check out this article on an introduction to statistical learning with Python book length.
And if you’re looking to improve your gardening game, consider investing in a Digital Soil Thermometer. Knowing your soil temperature can help you make better decisions on planting times and crop care.
Lastly, don’t forget about the importance of organic farming. Check out this Organic Fertilizer to enhance your soil health sustainably.
All images from Pexels