Introduction
Statistics can often feel like a labyrinth, especially when dealing with numbers that seem to have a mind of their own. One of the key concepts in this numerical maze is resistance. But what does it mean if a statistic is resistant? Simply put, a statistic is considered resistant if it remains relatively stable, even when faced with extreme values or outliers. Think of it as the tough cookie in a jar full of fragile biscuits—no matter how many times you shake the jar, that cookie stays intact.
Understanding resistant statistics is crucial for effective data analysis. In a world awash with data, where outliers can skew results and lead to erroneous conclusions, knowing which statistics to rely on can save the day. Imagine trying to gauge the average income of a neighborhood when one homeowner is a billionaire. The mean income would shoot up, creating a false narrative. This is where resistant statistics shine, offering a more reliable reflection of the data.
In this post, we’ll tackle the ins and outs of resistant versus non-resistant statistics. We’ll explore definitions, provide examples, and dig into why some statistics are more resilient than others. By the end, you’ll be equipped to make better data-driven decisions and perhaps even dazzle your friends with your newfound statistical savvy! And if you’re looking for a solid starting point for your statistical journey, consider picking up Statistics for Dummies by Deborah J. Rumsey. It’s a great way to break the ice with statistics!
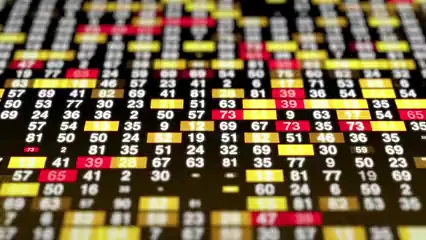
Understanding Resistant and Non-Resistant Statistics
Definition of Resistant Statistics
So, what exactly makes a statistic resistant? The essence lies in its ability to withstand the chaotic influence of outliers. For instance, the median and the interquartile range (IQR) are prime examples of resistant statistics. The median, which represents the middle value in a dataset, is largely unaffected by extreme values. In a dataset like [1, 2, 3, 100], the median remains 2, despite the outlier.
On the flip side, we have statistics that are anything but resistant. The mean is a classic example. When we calculate the mean, we add up all values and divide by the count. If there’s an outlier, such as that pesky 100 in the previous dataset, the mean skyrockets to 26, leading to a skewed perception of the data. Other non-resistant statistics include the standard deviation and the range, which also fall victim to the whims of outliers.
In summary, resistant statistics are the unsung heroes of data analysis, providing a stable foundation even when faced with extreme values. They help researchers and analysts draw more accurate conclusions, ensuring that the narrative told through data remains true to its core. If you want to dive deeper into data science, check out The Art of Statistics: Learning from Data by David Spiegelhalter. It’s a must-read for anyone looking to sharpen their analytical skills!
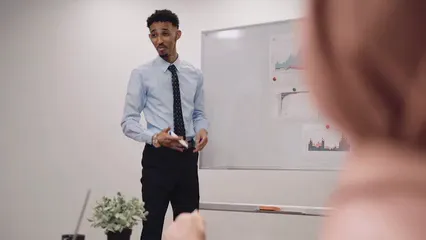
Why Some Statistics Are Resistant
Statistics can be fickle friends, especially when it comes to outliers. You know, those pesky data points that seem to have wandered in from another dimension? Outliers can throw off calculations, leading to misconceptions. Enter resistant statistics, the champions of stability! These statistics laugh in the face of extreme values, maintaining their integrity when the going gets tough.
Outliers can skew the results of non-resistant statistics like the mean or standard deviation. For instance, if we have a dataset representing the ages of a group of friends—let’s say [25, 26, 27, 28, 29, 30, 100]—the mean age skyrockets to around 36. But what about that 100-year-old? They might have a fascinating life story, but they shouldn’t define the group!
In contrast, resistant statistics like the median and interquartile range (IQR) hold strong. In the same dataset, the median age is still 28.5, stubbornly unaffected by that age anomaly. This resistance is crucial for providing a true representation of the data. If you’re fascinated by how to measure everything, check out How to Measure Anything: Finding the Value of “Intangibles” in Business by Douglas W. Hubbard. It’s a real eye-opener!
Let’s consider another example. Imagine a dataset of household incomes: [30,000, 32,000, 34,000, 35,000, 36,000, 38,000, 1,000,000]. Here, the mean income jumps to a jaw-dropping 213,000, thanks to that one millionaire. However, the median income, sitting at 35,000, tells a more realistic story of the group’s financial situation.
Resistant statistics shine in various scenarios, especially when analyzing data prone to outliers, such as real estate prices or income levels. They ensure that the core message of the data remains intact and trustworthy. So, the next time you’re crunching numbers, remember: when the data gets wild, resistant statistics are your reliable allies!
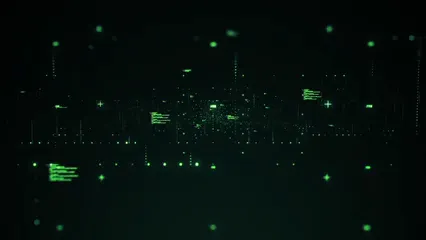
Practical Applications of Resistant Statistics
When it comes to statistics, not all measures are created equal. Some are more reliable than others, especially in real-world scenarios where outliers lurk like ninjas ready to pounce on your data analysis. That’s where resistant statistics come into play, particularly in fields like real estate and income data.
In real estate, property prices can vary dramatically. Picture a neighborhood where most homes are priced at $300,000, but one mansion is listed for a cool $3 million. If you were to calculate the mean price, you’d get a figure that misrepresents the actual market. Instead, using the median gives a more accurate picture, effectively ignoring that fancy mansion’s influence. For those interested in the intersection of data and real estate, Real Estate Investing for Dummies by Eric Tyson and Robert S. Griswold is a fantastic read!
Similarly, organizations like the U.S. Department of Housing and Urban Development (HUD) prefer the median for reporting average home prices. The median provides a truer reflection of the market by minimizing the skew caused by high-end properties. This way, buyers and policymakers can make informed decisions without getting distracted by outliers.
Another area where resistant statistics shine is income data. Imagine a dataset that includes the earnings of a large group of people, with one billionaire skewing the results. Calculating the mean income would give a misleading representation of the typical person’s earnings. By using the median, which remains stable in the presence of extreme values, analysts can offer insights that truly reflect the economic landscape. If you’re curious about the stories behind wealth, check out The Millionaire Next Door by Thomas J. Stanley and William D. Danko. It’s full of surprising insights!
In these scenarios, resistant statistics, such as the median and interquartile range, serve as a beacon for accurate data interpretation. They ensure that the core message remains intact, allowing stakeholders to make decisions based on reliable information rather than being misled by a few outliers.

When to Use Resistant Statistics
Guidelines for Choosing Statistics
Choosing the right statistics can make or break your data analysis. Understanding when to use resistant statistics is key, especially in datasets that are prone to outliers. Here are some guidelines to help you navigate these murky waters.
First, identify outliers in your dataset. Outliers are data points that deviate significantly from the rest. They can distort your results and lead to misleading conclusions. If you spot outliers, it’s time to consider resistant statistics like the median or interquartile range (IQR).
Next, understand when to favor resistant statistics over non-resistant ones. If your data distribution is skewed or contains extreme values, resistant measures will provide a more accurate representation. For example, in income data or real estate prices, where outliers are common, the median is your best friend.
Here are some practical tips for data analysis:
- Visualize Your Data: Use box plots or histograms to identify potential outliers. These visual tools can highlight extreme values that may skew your analysis.
- Calculate Both Types of Statistics: When possible, calculate both resistant and non-resistant statistics. This comparison can provide a clearer picture of how outliers affect your data.
- Focus on the Big Picture: Always remember that the goal is to tell a story with your data. Choose statistics that help reveal that story without distortion from extreme values.
- Consider the Context: Different fields have different norms. For instance, in real estate, the median is the go-to measure, while in scientific research, mean values might be more common. Adapt your approach based on the context of your data.
By following these guidelines, you can ensure that your analysis remains robust, even in the face of unruly data. Choosing resistant statistics allows you to maintain the integrity of your findings, making you a more effective data analyst. And if you’re looking to enhance your data science toolkit, consider Data Science for Business by Foster Provost and Tom Fawcett. It’s a great resource for understanding data analytics!

Case Studies
Let’s stroll through some real-world examples where resistant statistics came to the rescue, saving the day from the drama of outliers.
Case Study 1: Real Estate Pricing
Imagine a neighborhood where most homes are sold for around $300,000. Suddenly, a mansion hits the market for $3 million. If we calculated the average price using the mean, you might think everyone lives like royalty! However, the median price, which remains stable at $300,000, gives a much clearer picture of the actual market. Using the mean in this scenario could mislead buyers and sellers alike, resulting in poor investment decisions. For a deeper dive into how to analyze market trends, consider The Big Short by Michael Lewis. It provides an engaging look at financial markets and risk!
Case Study 2: Income Distribution
Consider a dataset representing the annual incomes of employees at a company. Most earn between $40,000 and $70,000, but one executive earns $1 million. The mean income shoots up to $200,000, making it seem like every employee is rolling in dough. In contrast, the median income sits comfortably at $60,000, reflecting the true earning potential of the majority. Relying on the mean here could lead to inflated salary expectations and dissatisfaction among employees. If you want to understand more about economic disparities, grab a copy of Freakonomics by Steven D. Levitt and Stephen J. Dubner. It’s full of surprising insights!
Case Study 3: Test Scores
Picture a classroom where students score between 60 and 90 on a test. Then, one student scores a 5. The mean score plummets to 55, misrepresenting the class’s overall performance. However, the median score remains at 75, highlighting that most students did well. In this case, using the mean could lead teachers to mistakenly believe that the entire class is struggling, prompting unnecessary interventions. If you’re interested in educational statistics, consider Outliers by Malcolm Gladwell. It explores the factors that lead to high achievement!
These examples illuminate the critical role of resistant statistics in providing accurate insights. Ignoring the power of the median and interquartile range could lead to misguided conclusions and decisions, showcasing that sometimes, less is more when it comes to data analysis.

Related Concepts in Statistics
Robustness vs. Resistance
When navigating the statistical landscape, it’s essential to grasp the nuances between robustness and resistance. While these terms might seem interchangeable at a glance, they carry distinct meanings in statistical analysis.
Robustness refers to the ability of a statistical method to perform well under various conditions, including the presence of outliers and deviations from assumptions. Robust statistics maintain their validity even when the data isn’t perfect. For instance, robust regression techniques can minimize the influence of outliers, ensuring the model remains effective.
On the other hand, resistance specifically deals with how much a statistic is affected by extreme values. A resistant statistic, like the median, remains stable when outliers are present, providing a reliable measure of central tendency. The median’s value shifts minimally, even when a data point goes rogue, making it a steadfast choice for datasets prone to anomalies.
Both concepts relate to outliers, but they have different implications. Resistance is a property of individual statistics, while robustness pertains to the methods used in analysis. For example, while the median is resistant, the mean is not; thus, the median is often preferred in skewed distributions. Conversely, a robust statistical method, like trimmed means, seeks to reduce outlier impact while still utilizing the data effectively.
Understanding these distinctions is crucial for statisticians and analysts. Choosing resistant statistics helps maintain the integrity of data interpretation. Embracing robust methods can enhance the reliability of analyses, even when faced with imperfect data. In statistical storytelling, both resistance and robustness play pivotal roles, ensuring conclusions drawn from data are as accurate as they are insightful. If you’re looking to expand your understanding of statistical learning, consider The Elements of Statistical Learning by Trevor Hastie, Robert Tibshirani, and Jerome Friedman. It’s an excellent resource for anyone interested in data science!
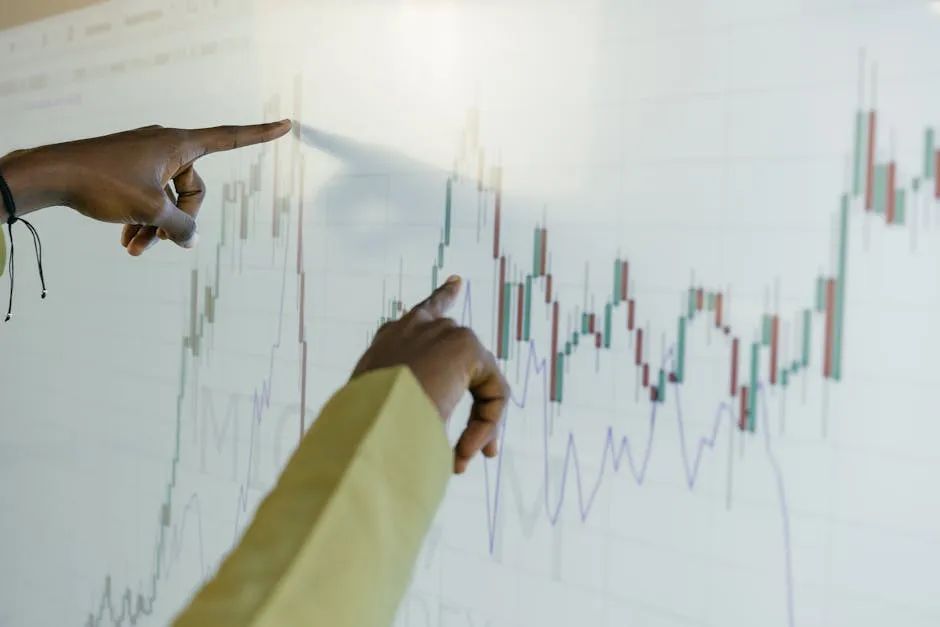
Other Measures of Central Tendency
When discussing central tendency, the mode deserves a mention. It represents the most frequently occurring value in a dataset. Interestingly, the mode can exhibit a touch of semi-resistance. It can remain stable in the presence of outliers, but its sensitivity varies depending on the frequency of values. For instance, if a dataset has a single outlier while most values cluster around a common number, the mode might hold its ground quite well. However, if another value suddenly becomes more frequent, the mode could shift dramatically.
Understanding all measures of central tendency is crucial in statistics. The mean, median, and mode each offer unique perspectives on a dataset. The mean is sensitive to outliers, while the median provides a solid, resistant measure. The mode, with its semi-resistance, can be insightful, especially in categorical data. By examining these measures together, statisticians can paint a more comprehensive picture of the data distribution. This multifaceted approach helps in making well-informed decisions based on accurate and reliable statistics.

Conclusion
In summary, understanding what it means if a statistic is resistant is essential for accurate data interpretation. Resistant statistics, such as the median and interquartile range, maintain their integrity in the face of extreme values or outliers. This resilience makes them valuable tools in data analysis. On the other hand, non-resistant statistics like the mean can be swayed significantly by a few rogue data points, leading to potentially misleading conclusions.
Throughout this article, we’ve explored the definitions and implications of resistant versus non-resistant statistics. We’ve seen how resistant statistics provide a more reliable reflection of the data, allowing analysts to draw meaningful insights without being misled by anomalies. Whether you’re dealing with income data, real estate prices, or test scores, employing resistant statistics can enhance the accuracy of your analysis. And for those looking to expand their knowledge, Naked Statistics: Stripping the Dread from the Data by Charles Wheelan is an entertaining read that demystifies the subject!

As you move forward in your data analysis practices, remember the importance of using resistant statistics. Pay attention to outliers and choose your measures of central tendency wisely. By applying this knowledge, you can ensure that your conclusions are robust and grounded in reality. So, the next time you crunch some numbers, consider the resistance of your statistics—it could make all the difference in your analysis!
FAQs
What does it mean if a statistic is resistant?
A statistic is considered resistant if it is not significantly affected by extreme values. This means that even when outliers are present, the statistic remains stable and provides a reliable representation of the data.
Why is the median a resistant statistic while the mean is not?
The median is resistant because it is the middle value when a dataset is ordered, making it stable against extreme values. On the other hand, the mean is calculated by averaging all data points, which means it can be heavily influenced by outliers.
When should I use resistant statistics?
Resistant statistics should be used when dealing with datasets that contain outliers or are skewed. In such cases, the median and interquartile range provide a more accurate representation of the data’s central tendency and variability.
Can the mode be considered a resistant measure?
The mode can be considered semi-resistant, as it may not be significantly affected by outliers depending on the frequency of values in the dataset. However, its value can change if a different value becomes more frequent due to outliers.
Why is understanding resistant statistics important?
Understanding resistant statistics is crucial because it affects the quality of data interpretation and decision-making. Using the right measures helps prevent misleading conclusions that could arise from relying on non-resistant statistics, especially in fields like finance, healthcare, and policy-making.
Please let us know what you think about our content by leaving a comment down below!
Thank you for reading till here 🙂
For a deeper understanding of the concept of median in statistics, check out this comprehensive guide on statistics poland median salary 2024.
To understand the implications of mean in statistical analysis, you can read more about what does mean identically distributed in statistics.
For effective strategies in data analysis, refer to our tips on tips for effective data analysis in economics and statistics.
For insights into how to interpret your data correctly, explore the differences between inferential statistics vs descriptive statistics in data interpretation.
For finance professionals seeking robust methodologies, check this guide on statistical methods for finance professionals 2024.
All images from Pexels