Introduction
In the realm of statistical analysis, designed experiments are pivotal in establishing causation and drawing meaningful conclusions from data. This article will explore the two essential features that characterize all statistically designed experiments: the necessity of having a control group and ensuring adequate sample size. By understanding these features, researchers can enhance the validity and reliability of their experimental findings. This introduction will set the stage for discussing the significance of these features and their implications in various fields. One might wonder why these two features are so crucial. Let’s take a closer look at each aspect, starting with the control group. Imagine you’re testing a new miracle drug. Without a control group, you might mistakenly think the drug works, when in fact, the patients simply got better on their own. Control groups help us avoid such pitfalls. They serve as a benchmark, allowing researchers to compare the effects of treatments accurately. Now, let’s shift our focus to sample size. Picture a party where only a few guests are asked to share their opinions on the cake. If only a handful of people taste the cake, their feedback may not represent the entire party. Similarly, in experiments, having an adequate sample size is vital. A larger sample size ensures that results are not due to chance and increases the study’s power. Speaking of power, if you’re venturing into the world of statistical analysis and need a solid foundation, consider picking up Statistical Methods for Research and Development by R. G. H. K.. This book is like a Swiss Army knife for researchers—packed with tools to help you navigate your statistical needs. In summary, these features are not just technicalities; they form the foundation of credible and reliable research. Understanding their importance can drastically improve the quality of experimental results. Now, let’s dig deeper into the significance of control groups and sample sizes.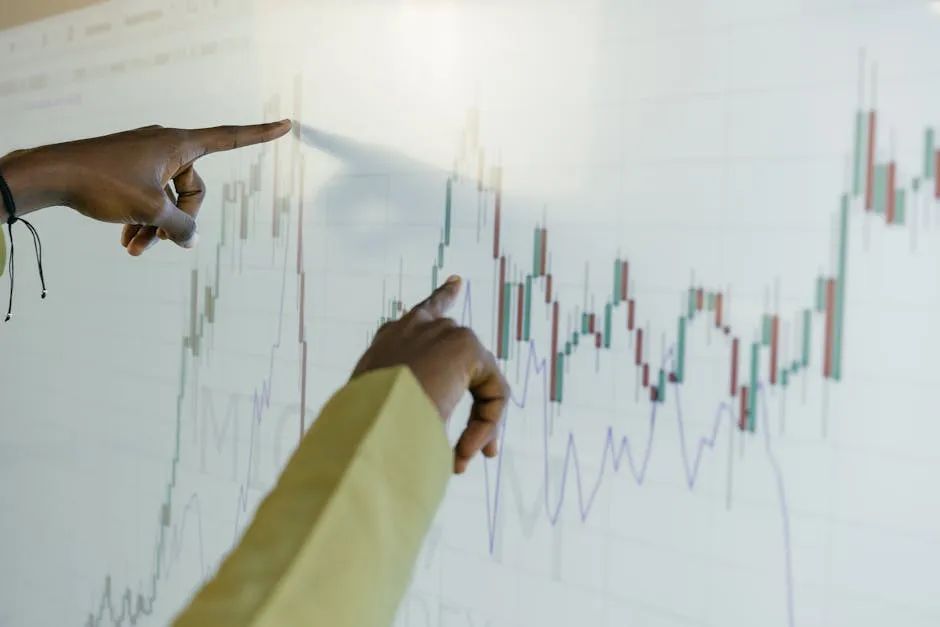
The Importance of Statistically Designed Experiments
Understanding Statistically Designed Experiments
Statistically designed experiments are structured studies aimed at determining how various factors influence outcomes. Unlike observational studies, where researchers simply observe and record data, designed experiments allow for manipulation of variables. This manipulation helps establish clearer cause-and-effect relationships. Randomization plays a crucial role in designed experiments. By randomly assigning subjects to different treatment groups, researchers minimize the effects of confounding variables. This means that any observed effect is more likely due to the treatment rather than some other factor. Replication is another essential component, as repeating experiments helps confirm findings and increases reliability. Overall, these experiments are essential tools in fields ranging from medicine to agriculture, providing a systematic approach to testing hypotheses and drawing meaningful conclusions. Understanding how they differ from observational studies can empower researchers to design more effective experiments and obtain reliable data.Historical Context and Evolution
The history of designed experiments is rich and fascinating. It dates back to the early 20th century, with significant contributions from pioneers like Ronald Fisher. Fisher recognized the importance of randomization and designed the experiments to control variability. His work laid the groundwork for modern statistical methodologies. Over time, methodologies have evolved significantly. From simple designs focusing on single-factor experiments to complex designs involving multiple factors and interactions, the field has grown. Key milestones include the introduction of factorial designs and response surface methods, which allow researchers to explore multiple variables simultaneously. Key figures such as Box and Draper have further advanced the field, emphasizing the importance of experimental design in ensuring robust results. Today, the principles of designed experiments are central to statistical analysis, enabling researchers to draw valid conclusions in various disciplines. In summary, understanding the historical context of designed experiments highlights their evolution and ongoing significance in research. By building on this foundation, researchers can continue to innovate and improve experimental design methodologies in the future.
The Two Essential Features of Statistically Designed Experiments
Feature 1: Use Enough Subjects
Importance of Sample Size
When conducting statistically designed experiments, the sample size is a crucial factor. Think of it this way: if you’re testing a new recipe, would you rather have feedback from just one person or a whole dinner party? Gathering input from a larger group helps ensure your results are reliable. Statistical significance and practical significance often dance around each other. Statistical significance means the results are unlikely to have occurred by chance. Practical significance, however, tells us if the findings matter in real-world scenarios. Imagine a drug that shows statistical significance, but only helps one in a hundred people. Is that practical? Probably not. The impact of sample size on the power and reliability of results can’t be overstated. A small sample size might lead to a false sense of security. Picture this: you conduct an experiment with only five participants. If two of them respond positively, you might think, “Aha! Success!” But with such a tiny group, those results could easily be flukes. Larger sample sizes increase the power of an experiment, providing more confidence in the conclusions drawn. A classic example involves clinical trials. When testing a new medication, researchers often recruit hundreds or thousands of participants. Why? Because small sample sizes can lead to erroneous conclusions. If a trial only includes ten people and a side effect occurs in one, should we panic? Perhaps not! But if that trial included a robust sample, we’d have clearer insights into safety and effectiveness. If you’re looking to delve deeper into sample sizes and their implications, check out Introduction to Statistical Quality Control by Douglas C. Montgomery. It’s an excellent resource that provides insights into quality control processes and emphasizes the importance of sample sizes in ensuring accurate results. Another important consideration is the variability within the sample. If your sample consists of diverse individuals, you’ll likely get a more accurate representation of the general population. Ultimately, having enough subjects is not just a number game; it plays a critical role in the integrity of your findings.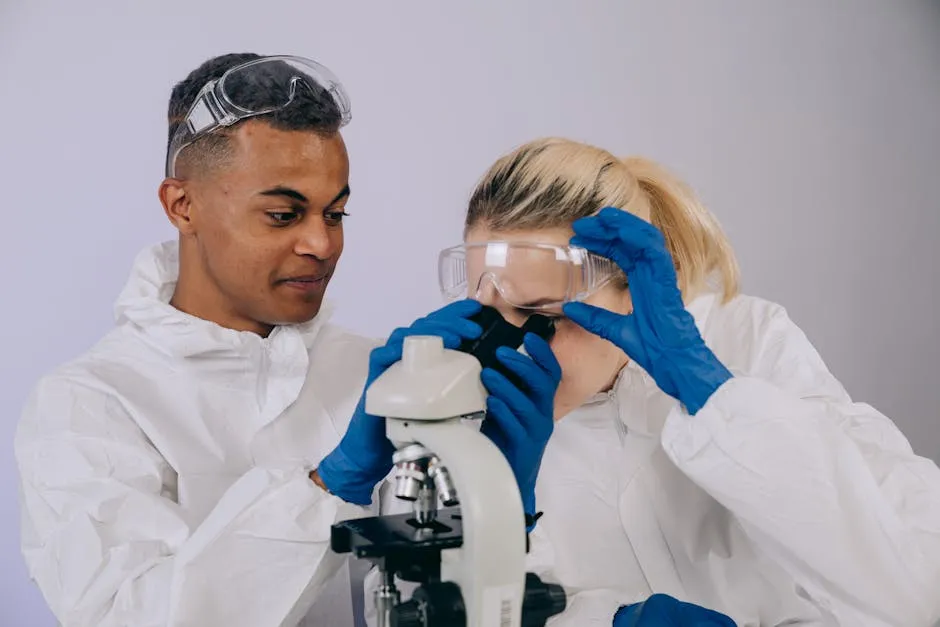
Guidelines for Determining Sample Size
Determining the right sample size can feel like trying to find the perfect outfit for an occasion—too big or too small just won’t do! Several factors come into play. First, consider the effect size, which reflects the magnitude of the difference you’re trying to detect. A larger effect size often requires a smaller sample to achieve significance, while a small effect size needs a larger one. Next, variability is essential. If your subjects vary widely in response, you’ll need a larger sample to capture that diversity. Think of it as trying to guess the average height of students in a school. If you surveyed just a few, you might miss the tall basketball players or the shorter students. Increasing your sample size helps to get a clearer picture. Then there’s the desired power of the test, which is the probability of correctly rejecting the null hypothesis. A common standard is aiming for a power of 0.8. In practical terms, if you want to be 80% confident in your results, you’ll need to calculate accordingly. There are numerous formulas and tools available to assist in sample size calculations. Online calculators and statistical software can be lifesavers! They typically require input on effect size, power, and significance levels. Utilizing these resources can streamline the process and ensure you’re not left guessing. Case studies abound that demonstrate the importance of optimal sample sizes. For instance, in agricultural experiments, researchers often conduct trials across varying conditions with robust sample sizes. These studies yield reliable results that help farmers make informed decisions about crop management. In summary, determining sample size is not just about picking a number out of thin air. It’s a thoughtful process grounded in statistical principles. By considering the effect size, variability, and desired power, researchers can ensure their findings are both valid and meaningful.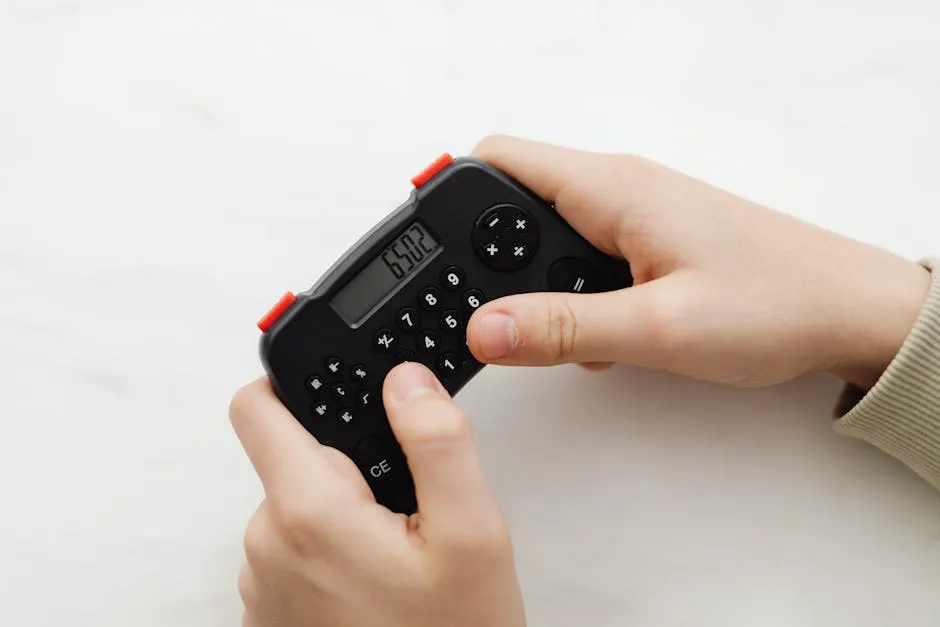
Feature 2: Always Have a Control Group
Role of Control Groups
Control groups are the unsung heroes of statistically designed experiments. Imagine you’re testing a new shampoo. If you only have one group using it, how can you tell if it’s the shampoo that’s improving hair health or just the magic of a good hair day? Control groups eliminate such uncertainties. They serve as a baseline for comparison, allowing researchers to assess the true impact of the treatment. A control group typically does not receive the treatment being tested. Instead, they might receive a placebo or no treatment at all. This setup helps to control for variables that might skew results. When subjects are assigned to different groups, it’s vital to ensure randomization. This ensures that any external factors are evenly distributed, minimizing bias. There are various types of control groups, including placebo, active control, and historical control groups. Placebo groups receive an inactive treatment, helping to gauge the psychological effects of believing you’re receiving treatment. Active control groups are given an existing treatment for comparison, while historical controls use past data to establish a baseline. Consider a case study involving a clinical trial for a new medication. Participants are divided into two groups: one receives the new drug, while the other receives a placebo. This design allows researchers to clearly see how effective the new drug is compared to no treatment. Without this control group, it would be challenging to determine the drug’s actual effectiveness. If you’re interested in learning more about the principles behind these designs, I recommend The Design of Experiments: Statistical Principles of Research Design and Analysis by Robert O. Kuehl. It’s a fantastic resource for anyone looking to deepen their understanding of experimental design. In summary, control groups are essential for establishing causal relationships in experiments. They provide a benchmark, allowing researchers to determine whether changes in the experimental group can be attributed to the treatment. By minimizing confounding variables, control groups enhance the validity and reliability of experimental results.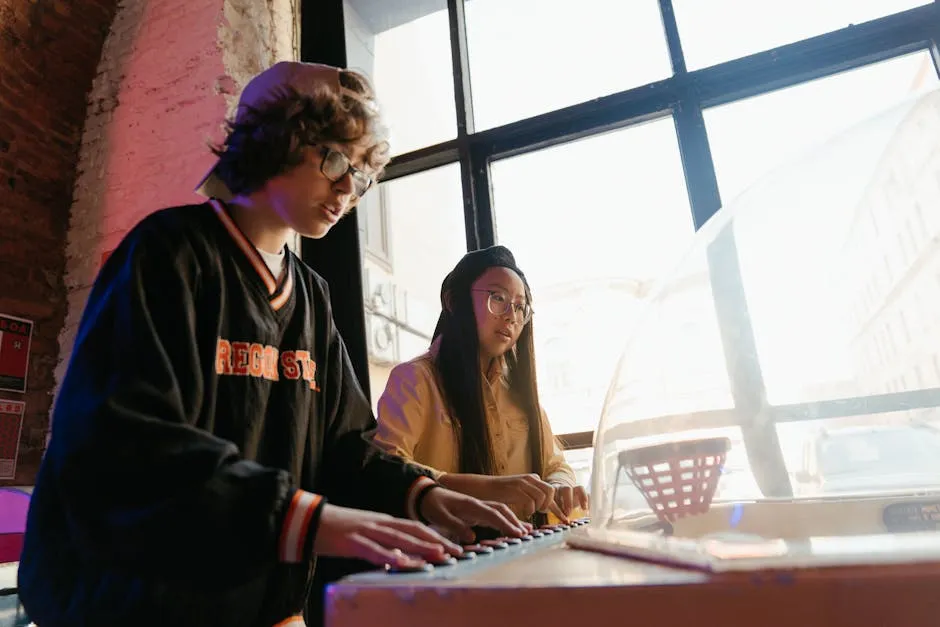
Benefits of Using Control Groups
Control groups are fundamental to the integrity of statistically designed experiments. They serve as the unsung heroes, quietly validating the reliability of our findings. Here’s a closer look at the many benefits of using control groups. First and foremost, control groups minimize bias and confounding variables. Picture this: you’re testing a new energy drink. If you only monitor those consuming the drink, you may overlook the effects of caffeine from their morning coffee. Control groups help level the playing field by providing a baseline against which the effects of the treatment can be compared. This means any differences in outcomes can be attributed directly to the treatment being tested, rather than to external factors. Next, control groups establish a clear baseline for comparing treatment effects. Imagine a clinical trial for a new medication aimed at reducing headaches. The treatment group takes the medication, while the control group receives a placebo. If both groups are monitored for headache reduction, researchers can clearly see how effective the medication is in comparison to no treatment. Without this baseline, determining the true efficacy of the drug would be like trying to find a needle in a haystack—nearly impossible! Moreover, control groups have real-world implications in research. In fields like medicine, psychology, and social sciences, the ability to draw valid conclusions hinges on the use of control groups. They provide critical evidence that helps shape treatment protocols, guide public health policies, and inform clinical practices. The impact of these findings extends beyond the lab, influencing practices that can enhance patient care and improve health outcomes. For instance, consider the famous study on smoking cessation methods. Researchers compared a group using nicotine patches with a control group using a placebo patch. The results highlighted the effectiveness of the nicotine patches, guiding healthcare professionals toward more effective smoking cessation techniques. This is just one example of how control groups can lead to significant advancements in various fields. Let’s not forget the psychological aspect. Participants in studies often change their behavior merely by being part of an experiment—this is known as the Hawthorne effect. Control groups help mitigate this by ensuring that any changes in behavior can be attributed to the treatment rather than the participants’ awareness of being observed. When participants in the treatment group are compared to a control group that is unaware of the actual treatment, researchers can get a clearer picture of the treatment’s true impact. In conclusion, control groups are not merely a box to check on a research study. They are vital components that enhance the validity and reliability of experimental findings. By minimizing bias, establishing baselines, and providing real-world insights, control groups help researchers draw meaningful conclusions that can influence practices and policies across various fields. So, the next time you hear about an experiment, remember the silent, steadfast control group working behind the scenes to uphold the integrity of scientific inquiry.
Conclusion
The two essential features of statistically designed experiments—having enough subjects and always including a control group—are the cornerstones of reliable research. These features ensure that the results obtained from experiments are valid, meaningful, and capable of guiding future investigations or practical applications. First, let’s talk about sample size. Imagine trying to predict the weather by asking just two people if they think it will rain. That’s not a solid plan, right? Similarly, relying on a small sample size in an experiment can lead to erroneous conclusions. Larger sample sizes provide a clearer picture, reducing the likelihood that results are due to random chance. They enable researchers to detect true effects with greater confidence, ultimately leading to more robust findings. A well-sized sample can transform an experiment from a guessing game into a reliable source of information. Now, let’s shift gears to control groups. Think of a control group as your trusty sidekick in the world of experiments. When testing a new treatment or intervention, the control group doesn’t receive the treatment, providing a baseline for comparison. This separation helps to identify whether the outcomes are genuinely due to the treatment or simply the result of external factors. Without a control group, researchers risk attributing changes to the treatment when they might have occurred naturally. This can lead to misleading conclusions, which is about as helpful as a chocolate teapot. Together, these features enhance the integrity of research. They minimize bias, control for confounding variables, and ensure that findings are not just flukes. By prioritizing adequate sample sizes and control groups, researchers can produce results that genuinely inform and improve future studies or real-world applications. Overall, understanding and implementing these essential features will empower researchers to create experiments that not only stand up to scrutiny but also contribute significantly to their fields.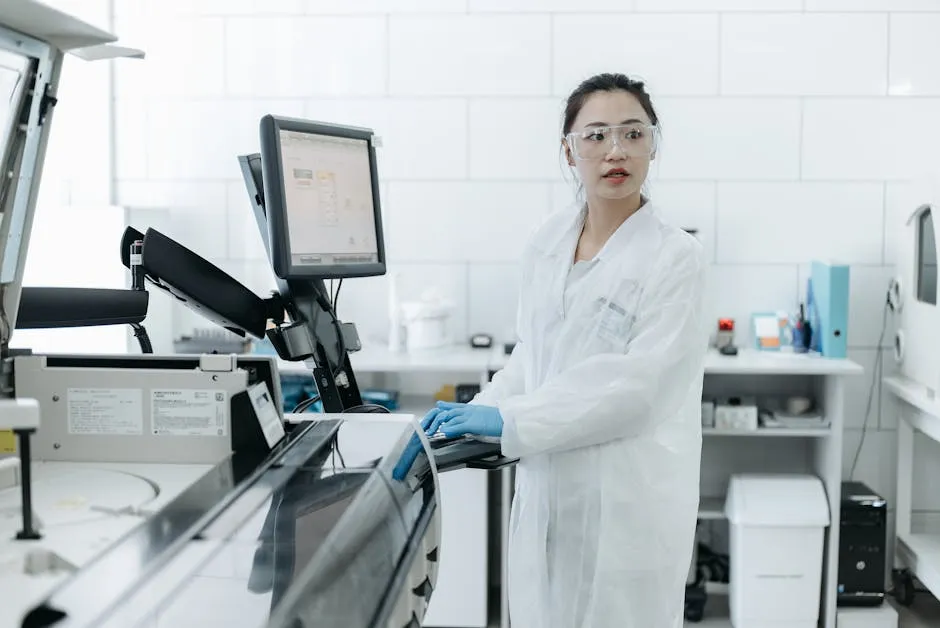
FAQs
What is a statistically designed experiment?
A statistically designed experiment is a structured study aimed at determining the effects of one or more independent variables on a dependent variable. By manipulating these variables and controlling extraneous factors, researchers can establish cause-and-effect relationships. Unlike observational studies, where data is collected without intervention, designed experiments allow for more rigorous testing of hypotheses.
Why is sample size important in experiments?
Sample size plays a crucial role in the reliability of experiment results. A larger sample size reduces the margin of error and increases the statistical power, making it more likely to detect a true effect if one exists. Small sample sizes can lead to misleading conclusions, as they may not accurately represent the population. For instance, if only a few individuals are surveyed, the findings may be skewed by outliers or anomalies, undermining the study’s validity.
How does a control group affect the outcomes of an experiment?
Control groups are essential for establishing a baseline against which the effects of treatments can be compared. They help isolate the effects of the treatment from other variables that could influence the outcome. Without a control group, researchers might attribute changes in the experimental group to the treatment when they could be due to other factors, leading to inaccurate conclusions. Essentially, the control group acts as a safeguard against overestimating or misinterpreting the treatment’s effectiveness.
Can I conduct an experiment without a control group?
While it’s technically possible to conduct an experiment without a control group, doing so is highly discouraged. The absence of a control group limits the ability to draw valid conclusions about the treatment’s effectiveness. Without a point of comparison, researchers risk attributing any observed effects to the treatment when they may not be caused by it at all. This can lead to flawed research and potentially harmful applications, especially in critical fields like medicine or psychology.
What tools can help calculate the appropriate sample size?
Several statistical software packages and online calculators can assist researchers in determining the appropriate sample size for their studies. Tools like G*Power, R, and specialized calculators available on various statistical websites allow for easy input of parameters like effect size, significance level, and desired power. By using these tools, researchers can make informed decisions about sample size, ultimately enhancing the quality and reliability of their experimental findings.
Understanding the differences between practical significance versus statistical significance is crucial for researchers to interpret their results effectively.
All images from Pexels