Introduction
Behavioral statistics is like the GPS for the behavioral sciences. It guides researchers through the chaotic maze of human behavior, ensuring they can analyze data effectively and draw meaningful insights. This branch of statistics specializes in understanding and interpreting data within psychology, sociology, education, and related fields. Its significance lies in transforming raw numbers into actionable knowledge, allowing professionals to make informed decisions.
This article aims to provide a comprehensive overview of the essentials of behavioral statistics. We will cover fundamental concepts, historical context, core principles, and practical applications. By the end, readers will have a solid foundation in behavioral statistics, empowering them to navigate their own research journeys.
Our target audience includes students, researchers, and professionals in the behavioral sciences. Whether you’re just starting out or looking to refresh your knowledge, this guide will serve as a valuable resource. So, grab your notepad and prepare for an enlightening exploration of behavioral statistics!
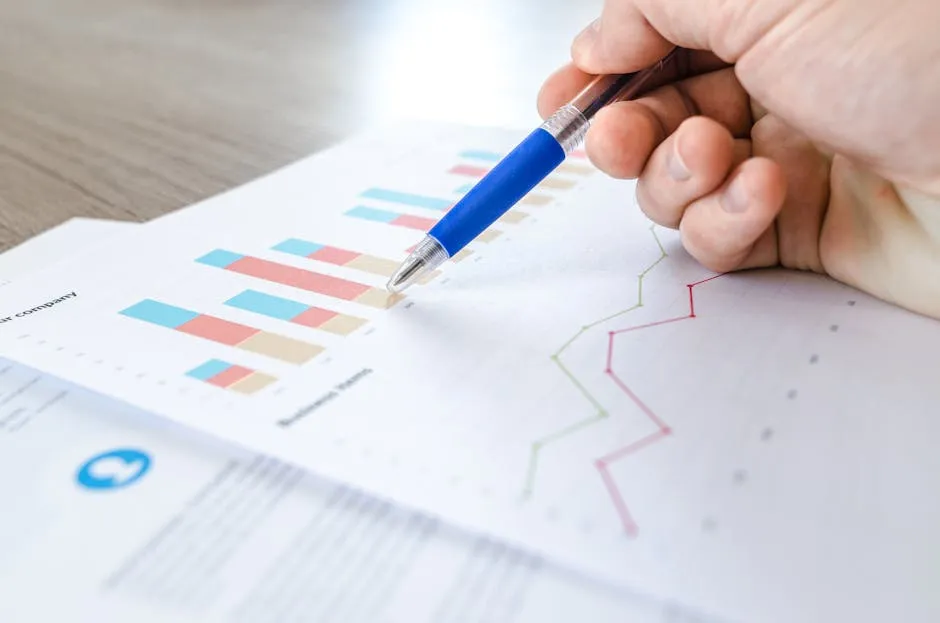
I. Understanding Behavioral Statistics
A. Definition and Importance
Behavioral statistics refers to the application of statistical methods to analyze and interpret data relevant to human behavior. It encompasses a wide range of techniques that help researchers summarize, compare, and make predictions based on data. This field is essential for conducting rigorous research, as it enables professionals to quantify behavior and analyze patterns within data sets.
In research, behavioral statistics plays a crucial role in various stages. First, it helps formulate hypotheses by providing a framework for testing ideas. Then, it offers tools to analyze data, ensuring results are reliable and valid. Finally, behavioral statistics aids in interpreting findings, allowing researchers to draw meaningful conclusions. Without it, our understanding of human behavior would be akin to navigating a dark room without a flashlight—confusing and fraught with errors.
For those looking to dive deeper into the world of statistics, Statistics for Dummies is a fantastic starting point. It breaks down complex concepts into easily digestible pieces, making it perfect for beginners and seasoned pros alike.
B. Historical Context
The evolution of statistics in the behavioral sciences is a fascinating tale full of milestones. In the early days, researchers relied on simple descriptive techniques, but as the field grew, so did the complexity of statistical methods. The late 19th and early 20th centuries marked significant advancements, with pioneers like Karl Pearson and Ronald A. Fisher laying the groundwork for modern statistical analysis.
Pearson introduced correlation coefficients, which measure the strength of relationships between variables. Fisher contributed to hypothesis testing and analysis of variance (ANOVA), crucial tools in behavioral research today. These foundational figures established principles that researchers still utilize, ensuring statistical rigor in behavioral studies.
To understand these principles better, consider picking up Statistical Methods for Psychology. This book offers a comprehensive overview of the methods used in psychological research, ensuring you grasp the historical context and application of these techniques.
The journey of behavioral statistics continues to evolve. With the advent of technology, researchers now have access to powerful software that streamlines data analysis. This evolution has opened doors to new methodologies and innovative ways to interpret behavior in an increasingly complex world. As we progress, the importance of behavioral statistics remains paramount, serving as a cornerstone for research in the behavioral sciences.
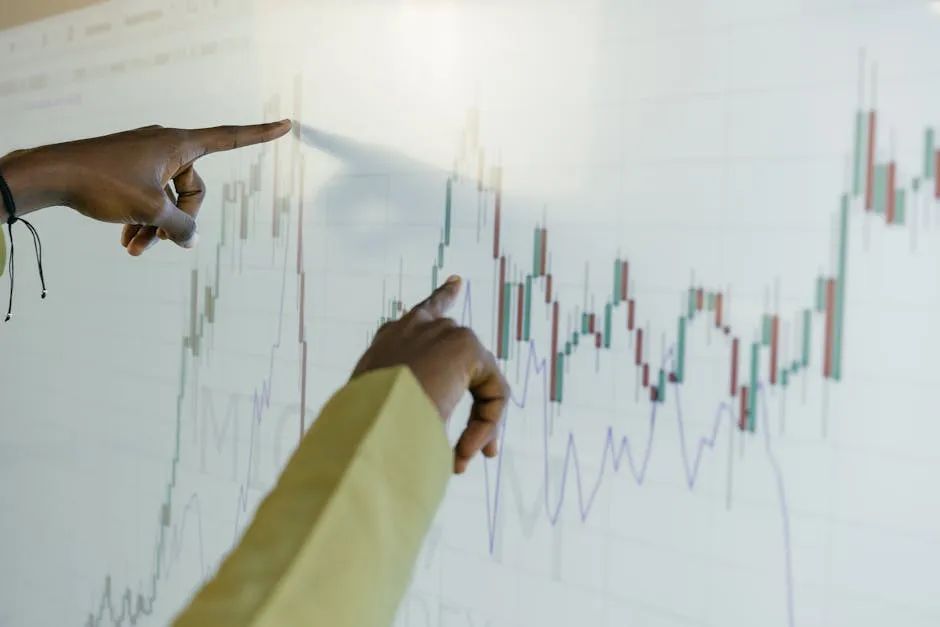
C. Core Principles
Behavioral statistics is governed by several core principles that shape its application in research. Understanding these principles enhances the quality and reliability of findings in the behavioral sciences.
First, the principle of measurement is fundamental. It ensures that researchers accurately quantify variables of interest. This involves defining concepts clearly, selecting appropriate measurement tools, and ensuring consistency in data collection. For example, when measuring anxiety, a researcher might use standardized questionnaires or physiological markers. Consistent measurement leads to more reliable data, which is essential for drawing valid conclusions.
Next, the principle of variability is crucial. Human behavior is not uniform; it varies widely among individuals. Recognizing this variability helps researchers understand that averages may not represent everyone accurately. For instance, when studying stress levels among students, some may exhibit high stress due to exams, while others feel minimal pressure. Accounting for such differences is vital in interpreting results.
Additionally, the principle of sampling is key. Researchers often rely on samples to make inferences about larger populations. A well-chosen sample represents the population adequately, minimizing bias. If a study on student behavior only surveys one classroom, the findings may not apply to all students. Random sampling techniques can help ensure that the sample reflects the population accurately.
Moreover, the relationship between statistics and research methodology cannot be overlooked. Statistics serves as a tool for researchers, allowing them to analyze data and test hypotheses rigorously. Depending on the research design, different statistical methods are employed. For example, experimental studies may utilize t-tests, while observational studies might rely on regression analysis. This interplay between methodology and statistics ensures that researchers use the right tools for their specific questions, leading to more robust and credible findings.
Finally, ethical considerations play a significant role in behavioral statistics. Researchers must ensure their methods do not harm participants and that they handle data responsibly. This includes obtaining informed consent and maintaining confidentiality. Ethical research fosters trust and integrity, essential for the advancement of knowledge in behavioral sciences.
By grasping these core principles, researchers can enhance their statistical literacy and produce high-quality, credible research. These principles guide the choice of methods, the interpretation of data, and the ethical conduct of studies, ensuring that findings contribute meaningfully to our understanding of human behavior.

2. Confidence Intervals
Confidence intervals are a fundamental concept in behavioral research. They offer a range of values that likely contain the true population parameter based on sample data. Think of it as a safety net for researchers, providing an estimated interval where they can feel confident the true value lies.
Imagine you conduct a study to determine the average stress level among college students during finals. You gather data from a sample of 100 students and find that their average stress level is 75 on a scale of 1 to 100. However, this number alone doesn’t tell the whole story. What if the true average stress level is actually higher or lower? That’s where confidence intervals come into play.
By calculating a confidence interval, you might find that you are 95% confident that the true average stress level lies between 72 and 78. This interval gives you a more complete picture than just the average alone. It accounts for potential sampling errors, meaning that if you were to repeat the study multiple times, 95 out of 100 times, the true average would fall within this range.
Confidence intervals are particularly significant in behavioral research, where variability is common. They allow researchers to communicate the reliability of their estimates. For example, if another study finds a confidence interval of 70 to 80 for the same average stress level, it suggests alignment in findings, boosting confidence in the results.
Moreover, confidence intervals can help in decision-making processes. For instance, if a school is considering implementing stress management programs, knowing the average stress level and its confidence interval can guide resource allocation. If the interval indicates high stress levels, it may prompt immediate action.
In summary, confidence intervals are more than just numbers; they are a vital tool in behavioral statistics. They not only enhance the robustness of research findings but also illuminate the complexities inherent in human behavior. By understanding and applying confidence intervals, researchers can draw more meaningful conclusions, making their work not just informative but also impactful.

B. Analysis of Variance (ANOVA)
2. Two-Way ANOVA
Two-way ANOVA is like a party where two independent variables are invited to dance and show their effects on a dependent variable. This statistical method helps researchers understand how two factors interact and influence outcomes simultaneously. Say goodbye to simple comparisons; it’s time to add some flair to your analysis!
Imagine a study examining how study habits and sleep quality impact test performance. Using two-way ANOVA, researchers can assess whether different study methods (like flashcards or group study) and varying sleep durations (such as 6, 7, or 8 hours) impact test scores. The beauty of this method is its ability to reveal not just the main effects of each factor but also any interaction effects between them. In our example, perhaps students who study in groups and get 8 hours of sleep perform significantly better than those with just 6 hours of sleep.
This method is particularly beneficial in behavioral research. It allows for a more nuanced understanding of how multiple variables contribute to behavioral outcomes, providing insights that single-variable studies simply can’t match. To enhance your understanding of these concepts, consider diving into The Art of Statistics: Learning from Data. This book provides valuable insights into the principles of statistical analysis and interpretation.
Real-life studies often utilize two-way ANOVA to analyze complex data. For instance, a psychological experiment might explore how gender and type of reinforcement (positive or negative) affect learning outcomes. By applying two-way ANOVA, researchers can identify if the learning performance differs not only between genders but also how the type of reinforcement interacts with gender to influence learning effectiveness.
C. Correlation and Regression Analysis
1. Correlation Coefficients
Correlation is the lifeblood of behavioral studies. It quantifies the strength and direction of relationships between variables. Picture it as a trusty compass guiding researchers through the intricate web of human behavior. When studying the connection between two variables, correlation coefficients provide a numerical value that indicates how closely they are related.
There are two prominent types of correlation coefficients: Pearson’s and Spearman’s. Pearson’s correlation measures linear relationships and is great for interval or ratio data. It ranges from -1 to +1, indicating perfect negative or positive correlations, respectively. If you find a Pearson correlation of 0.8 between exercise frequency and mood, you can confidently say that as exercise increases, mood tends to improve.
On the other hand, Spearman’s correlation is the go-to for ordinal data or non-linear relationships. It ranks data points and measures how well the relationship between two variables can be described using a monotonic function. So, if researchers are investigating the relationship between stress levels (ranked) and study habits (also ranked), Spearman’s correlation provides valuable insights without the need for strict assumptions.
2. Simple and Multiple Regression
Regression analysis is the crystal ball of statistics, allowing researchers to predict outcomes based on one or more predictors. Simple regression examines the relationship between a single independent variable and a dependent variable, like predicting a student’s final grade based on their hours of study.
Multiple regression takes it a step further by considering multiple predictors. Imagine a researcher looking to predict mental health outcomes based on factors like exercise frequency, diet, and social support. Multiple regression analysis can reveal how each factor contributes to the overall prediction, helping to identify which aspects are most influential.
An intriguing example can be found in psychological research that investigates the effects of various lifestyle factors on well-being. By employing multiple regression, researchers can create a comprehensive model accounting for exercise, sleep quality, and social interactions, unveiling how these elements collectively impact mental health. For more insight into statistical methods for finance professionals, check out this statistical methods for finance professionals 2024 resource.
Understanding statistical methods can significantly enhance your research capabilities. statistical methods for finance professionals 2024
In summary, both correlation and regression analyses are pivotal tools in behavioral statistics. They not only enhance our understanding of relationships but also empower researchers to make informed predictions about human behavior, paving the way for effective interventions and solutions. If you want to delve deeper into the subject, consider reading Practical Statistics for Data Scientists. It combines practical applications with core statistical principles, making it a must-read for aspiring data professionals.
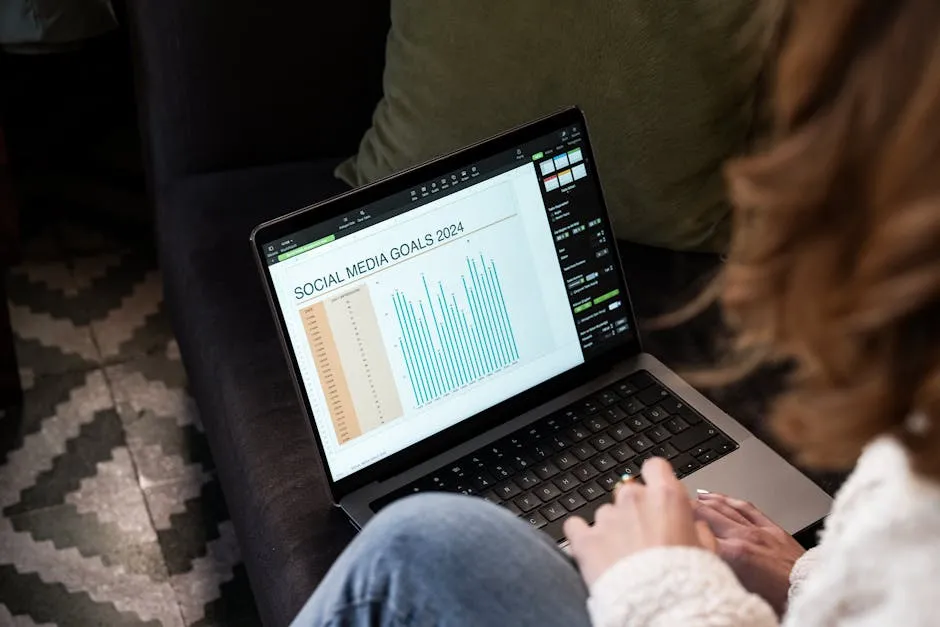
B. Tools and Software for Statistical Analysis
When it comes to analyzing behavioral statistics, having the right tools is crucial. Think of these software options as your trusty sidekicks, ready to tackle data like superheroes. Let’s take a peek at some of the most popular choices:
SPSS (Statistical Package for the Social Sciences)
SPSS is the go-to for many researchers. It offers a user-friendly interface that makes statistical analysis feel like a walk in the park. With SPSS, you can easily conduct various statistical tests, from t-tests to regression analysis. It also provides detailed output, which is essential for interpreting results. However, some might find its pricing a bit steep, especially for students. For troubleshooting and solutions related to SPSS, refer to this macos ibm spss statistics fix 28.1.1 guide.
For anyone using SPSS, having a reliable troubleshooting guide can make a significant difference. macos ibm spss statistics fix 28.1.1
R
Ah, R—the beloved open-source software! This tool is like a Swiss army knife for statisticians. R offers a vast library of packages designed for various statistical analyses. Its flexibility allows users to perform complex analyses and generate stunning visualizations. While R may have a steeper learning curve, its capabilities are unmatched. Once you get the hang of it, you’ll wonder how you ever managed without it. For a thorough introduction, consider reading A Beginner’s Guide to R to get started!
Python
Python is not just for coding wizards anymore. With libraries like Pandas, NumPy, and SciPy, it has become a powerful tool for statistical analysis. Python’s versatility allows researchers to blend data manipulation, statistical analysis, and machine learning all in one place. Plus, its growing community means you can find countless resources and tutorials to help you along the way. It’s like having a personal tutor at your fingertips! If you’re looking for a great resource on data analysis, check out Data Analysis with Python and PySpark.
Each of these tools has its strengths, making them suitable for different types of analysis. SPSS is great for those who prefer a straightforward approach. R is ideal for those who want flexibility and depth, while Python shines in its versatility and integration capabilities. Choosing the right software ultimately depends on your specific needs and comfort level.
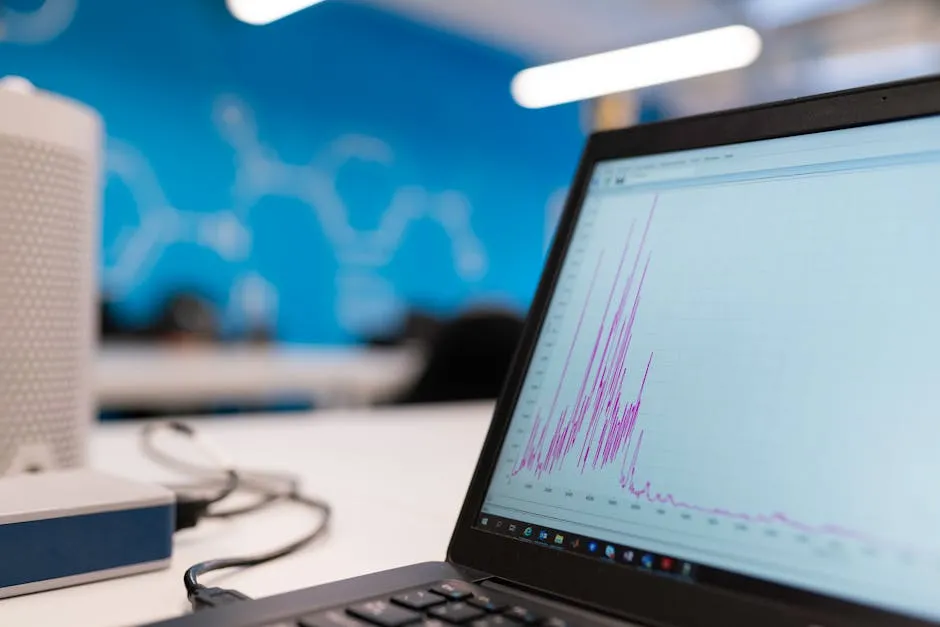
C. Challenges and Limitations
While behavioral statistics offers incredible insights, it’s not without its challenges. One common issue is the misinterpretation of results. Numbers can be deceiving, and without proper context, they may lead to incorrect conclusions. For instance, a significant correlation doesn’t imply causation, yet many fall into this trap.
Another hurdle is the complexity of human behavior itself. People are unpredictable, and this variability can complicate data analysis. It’s crucial to remember that statistical methods can only provide a partial picture. They can highlight trends but cannot account for every nuance of human behavior. To navigate this complexity, consider reading How to Lie with Statistics. This book humorously explores the misuse of statistics and how to avoid falling for misleading claims.
Statistical methods also have limitations. For instance, assumptions underlying many tests, such as normality or homogeneity of variance, may not always hold true. Ignoring these assumptions can skew results, leading to faulty conclusions. Thus, proper interpretation is vital. Researchers must approach their findings with a critical eye, ensuring they consider the broader context and limitations of their analyses.
In summary, while behavioral statistics is a powerful tool, it requires careful application and interpretation. Understanding its challenges and limitations is essential for producing valid and reliable results in research. And if you’re looking for ways to keep your mind sharp while working, why not try out a Noise-Cancelling Headphones? They can help you focus amidst distractions!

Please let us know what you think about our content by leaving a comment down below!
Thank you for reading till here 🙂
All images from Pexels