Introduction
Statistics are the backbone of decision-making in business. Managers rely on data to guide strategies, forecast trends, and measure performance. What’s the secret sauce for effective decision-making? It’s all about understanding and applying statistics.
In today’s fast-paced business environment, the ability to interpret data accurately is crucial. Statistics empower managers to make informed choices based on empirical evidence rather than gut feelings. This leads to smarter strategies and improved outcomes, whether it’s launching a new product or optimizing marketing campaigns.
Enter Microsoft Excel 2021 for Windows, the unsung hero of statistical analysis. This software isn’t just for budgeting or creating nifty spreadsheets. Excel is packed with powerful statistical tools and functions that can transform raw data into actionable insights. From simple calculations to complex analyses, Excel makes statistics accessible to everyone, even to those who may not have a math degree.
This article aims to be your trusty guide through the world of statistics in Excel. We’ll break down essential statistical concepts, demonstrate how to harness Excel’s powerful features, and provide practical examples tailored for managers. Whether you’re a seasoned expert or just starting out, this comprehensive guide will equip you with the skills you need to leverage statistics effectively in your business management. Let’s dive in!
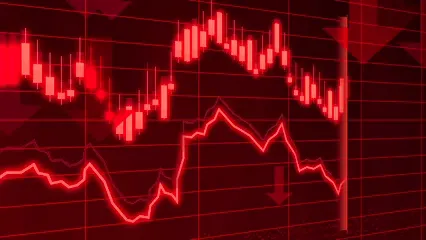
For a deeper understanding of statistical learning, check out this introduction to statistical learning with Python.
Understanding Statistics in Management
The Role of Statistics in Business
Statistics play a vital role in the world of business. They provide a framework for analyzing data, enabling managers to make informed decisions based on quantifiable evidence. Imagine trying to navigate a ship without a map—it’s risky and often leads to disaster. Statistics serve as a map, guiding managers through the murky waters of uncertainty.
One of the primary applications of statistics is in decision-making. For instance, companies use sales data to predict future trends, helping them allocate resources effectively. If you notice a spike in sales during certain seasons, that’s your cue to stock up. Alternatively, if sales are plummeting, you might need to reassess your marketing strategy.
Forecasting is another critical area where statistics shine. Businesses use statistical models to project future sales, customer behavior, and market trends. Think of it as crystal ball gazing, but with a whole lot more data and a lot less guesswork. For example, retailers analyze purchasing patterns to anticipate demand for specific products. This helps them avoid stockouts or overstock situations. To master these forecasting techniques, consider reading Statistics for Business and Economics. It’s a great resource that dives deep into these essential concepts.
Performance measurement is yet another essential function of statistics. Managers track key performance indicators (KPIs) to assess how well their teams or departments are performing. By analyzing data, you can pinpoint areas that need improvement. For instance, if customer satisfaction scores are dipping, it’s time to investigate and implement changes.
Statistics find applications across various business areas. In marketing, A/B tests help determine which advertisements perform better. In finance, statistical analysis aids in risk assessment and investment strategies. Even in human resources, statistics help analyze employee performance and retention rates.
In essence, statistics provide the insights that drive business success, enabling managers to make data-informed decisions that propel their organizations forward. By understanding and leveraging statistics, managers can stay ahead of the competition and adapt to changing market conditions. So, buckle up and let’s get ready to harness the power of statistics with Microsoft Excel!
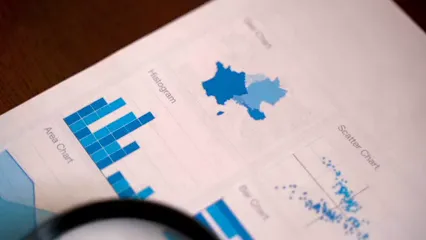
Key Statistical Concepts for Managers
Statistics can feel like a foreign language, but fear not, dear managers! Here we’ll break down essential statistical concepts that every savvy business leader should know: mean, median, mode, variance, and standard deviation. With a sprinkle of real-world magic, you’ll see just how these concepts can impact your daily decision-making.
Mean: This is the average. To find it, sum all your data points and divide by the number of points. For instance, if your sales figures for five months are $10,000, $12,000, $9,000, $15,000, and $14,000, the mean sales figure is $12,000. Easy peasy!
Median: This is the middle value when your data is arranged in order. If you have an odd number of values, the median is the middle one. If even, it’s the average of the two middle values. Say your quarterly profits are $5,000, $7,000, $9,000, and $12,000. The median is $8,000. This helps you understand what a “typical” month looks like, cutting out the extremes.
Mode: This refers to the value that appears most frequently in your data set. If your customer feedback scores are 4, 5, 5, 3, and 4, the mode is 5. Knowing the mode is particularly useful in marketing because it tells you the most common customer sentiment.
Variance: This measures how spread out your data points are from the mean. A high variance means your data points are wildly different, while a low variance indicates they are closer to the mean. For instance, if your sales numbers fluctuate wildly from month to month, you’ll see higher variance. This helps managers assess risk. If you’re looking to dive deeper into these concepts, grab a copy of The Data Warehouse Toolkit: The Definitive Guide to Dimensional Modeling. It’s a great resource for understanding data analysis.
Standard Deviation: This is simply the square root of the variance. It provides insight into how much your data deviates from the average. A low standard deviation indicates that the data points are close to the mean, while a high standard deviation suggests a lot of variability. For example, if your sales figures have a standard deviation of $2,000, you can expect most month’s sales to fall within $2,000 of the mean.
These concepts are not just numbers; they tell a story about your business’s performance. Understanding them arms you with the knowledge to make informed decisions that drive your company forward. So, whether you’re deciding on a new marketing strategy or analyzing team performance, these statistical tools are your trusty sidekicks!
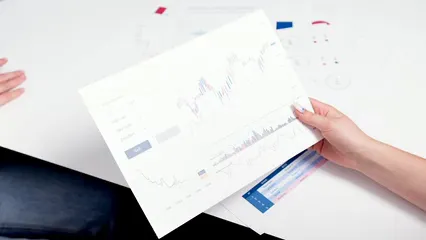
Data Collection and Preparation
Defining and Collecting Data
Data collection is essential for any analysis. Managers must choose the right method to gather accurate information. Common techniques include surveys, observations, and experiments. Each method has its strengths.
Surveys are popular for collecting data quickly. You can use online tools like Google Forms or SurveyMonkey. These platforms allow you to reach a wide audience. Plus, they make it easy to analyze responses. However, crafting clear questions is vital. Ambiguous questions can lead to misleading results.
Observations involve watching people or events. This method can provide insights that surveys might miss. For instance, a store manager might observe customer behavior to improve layout. It’s a hands-on approach, but it can be time-consuming and subject to bias.
Experiments, on the other hand, allow managers to test hypotheses. By manipulating variables, you can measure the effects. For example, a marketing manager might change ad placements to see which generates more sales. This method provides strong evidence but requires careful planning. If you’re looking for tools to assist in data analysis, consider the Minitab Statistical Software. It’s a fantastic resource for statistical analysis!
When collecting data, defining variables is crucial. Variables are the characteristics you measure. They can be quantitative (like sales numbers) or qualitative (like customer satisfaction). Understanding these types helps in choosing the right analysis techniques.
Types of data also matter. Categorical data represents groups or categories, like product types or customer demographics. Numerical data, however, involves measurable quantities, such as revenue or expenses. A solid grasp of these concepts ensures effective data collection and analysis.
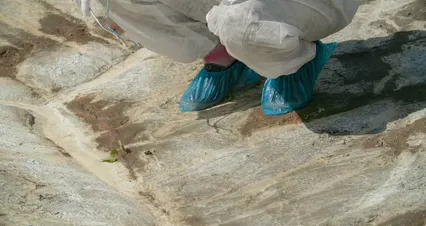
Data Cleaning and Preprocessing
Once data is collected, cleaning and preprocessing are next. This step is critical for accurate analysis. Data often comes with missing values and outliers that can skew results.
Handling missing values is a common challenge. You can choose to ignore them, replace them, or use statistical methods to estimate them. Excel provides useful functions for this. The IF function can help you set conditions for replacing missing data. For example, you could replace missing sales figures with the average of existing values.
Outliers are data points that differ significantly from others. They can result from errors or unique circumstances. Identifying outliers is essential; otherwise, they might distort your analysis. Excel’s ISERROR function can assist in identifying these anomalies. By flagging errors, managers can decide whether to keep or remove outliers.
Another technique for data cleaning is standardization. This process ensures that data is consistent in format. For example, converting all dates to a single format, like YYYY-MM-DD, simplifies analysis. Excel’s formatting tools can assist in this task.
Data preprocessing also involves transforming data. This could mean normalizing values, like converting all currency to a single type. Or, you might want to create new variables from existing ones. For instance, calculating profit margins from revenue and costs can provide added insights.
In summary, effective data cleaning and preprocessing are crucial. By using Excel functions like IF and ISERROR, managers can ensure their data is reliable. Clean data leads to better insights, making decision-making smoother and more informed.
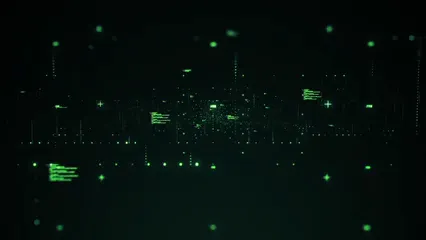
Organizing and Visualizing Data
Organizing Data in Excel
Once your data is clean, it’s time to organize it in Excel for analysis. Properly structuring your data is essential for efficient analysis and visualization. Think of it as creating a solid foundation for a house; without it, everything else can crumble.
Using tables is one of the best ways to organize data in Excel. Tables allow you to manage data efficiently. They automatically expand as you add more data, which helps maintain organization. Plus, tables come with built-in sorting and filtering options, making it easy to find specific information.
Named ranges are another powerful tool. By assigning names to specific data ranges, you can simplify formulas and functions. For example, instead of using “A1:A100,” you could name that range “SalesData.” This makes your formulas more readable and easier to maintain.
Consistency is key when organizing data. Ensure that similar data types are grouped together. For instance, keep all sales data in one column, customer information in another, and so on. This makes it easier to analyze and visualize data later.
Excel also allows for data validation. This feature ensures that the data entered meets specific criteria. For example, you can restrict a column to only accept dates or specific categories. This minimizes errors and helps maintain data integrity.
Moreover, consider using color coding or conditional formatting. This visual approach can help highlight important trends or outliers. For instance, you might use green for high sales figures and red for low ones. This immediate visual cue can enhance your data analysis process.

In conclusion, organizing data in Excel is vital for effective analysis. Utilizing tables, named ranges, and validation tools can streamline your workflow. A well-organized dataset not only boosts efficiency but also leads to clearer insights and better decision-making.
Visualization Techniques
Once your data is organized, it’s time to make it visually appealing! Visualization techniques like charts and graphs are essential for interpreting data. They transform raw numbers into visual stories that everyone can understand. After all, a picture speaks a thousand words, right?
Common visualization methods include bar charts, line graphs, pie charts, and histograms. Each serves a different purpose. Bar charts are great for comparing categories, while line graphs show trends over time. Pie charts illustrate proportions, and histograms reveal distributions. By choosing the right visualization, you help your audience digest complex data readily.
Let’s create a histogram in Excel—a fantastic way to visualize frequency distributions. Here’s how to do it step by step:
- Select Your Data: Highlight the data you want to visualize. This could be sales figures, customer ages, or any other numerical dataset.
- Insert a Histogram: Go to the “Insert” tab in the Ribbon. Click on “Insert Statistic Chart” and select “Histogram.” Voilà! Excel will generate a histogram based on your selected data.
- Adjust Bin Sizes: To customize the histogram, right-click on the horizontal axis and choose “Format Axis.” Here, you can adjust the bin width to better reflect your data’s distribution.
- Add Titles and Labels: Don’t forget to make it clear! Add a chart title and label your axes. This helps your audience understand what they’re looking at.
- Format for Clarity: Use colors and styles that make your chart easy to read. Consider using contrasting colors for different bars or lines to enhance visual appeal.
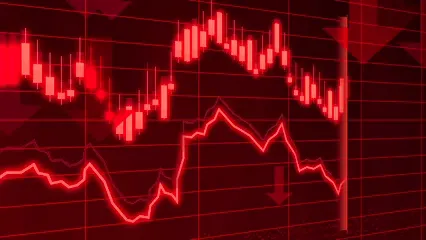
Now, let’s talk about scatter plots. They’re perfect for showing relationships between two numerical variables. Here’s how to create one:
- Select Your Data: Just like before, highlight the two columns of data you want to analyze.
- Insert a Scatter Plot: Head to the “Insert” tab, click on “Insert Scatter (X, Y) or Bubble Chart,” and select “Scatter.” Excel will plot the points for you.
- Add Trendline: To visualize the relationship, right-click on any data point and select “Add Trendline.” You can even display the equation on the chart!
- Customize: Don’t just stop there! Add chart titles, axis labels, and other formatting options to make your scatter plot informative and eye-catching.
In conclusion, effective data visualization is your secret weapon in data interpretation. By employing techniques like histograms and scatter plots, you bring your data to life, making it easier for stakeholders to understand and act on insights. So roll up your sleeves and get ready to transform your data into compelling visual narratives!
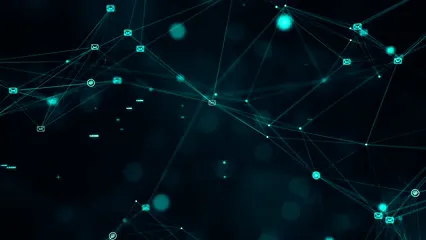
Descriptive Statistics
Descriptive statistics provide a summary of your dataset. They help managers make sense of raw data, transforming it into clear insights. Let’s say you have a dataset of monthly sales figures for the past year. These figures are $10,000, $12,000, $15,000, $9,000, $11,000, $14,000, $13,000, $16,000, $12,000, $10,000, $14,000, and $15,000.
To calculate key descriptive statistics using Excel, follow these steps:
- Mean: This is your average sales. Use the formula
=AVERAGE(A1:A12)
where A1:A12 contains your sales data. In this case, the mean sales amount to $12,083.33. - Median: The middle value when all sales figures are sorted. Use
=MEDIAN(A1:A12)
. The median calculates to $12,000, providing insight into typical performance. - Mode: This indicates the most frequently occurring sales figure. Use
=MODE(A1:A12)
. Here, the mode is $10,000 and $12,000, showing two peaks in your sales data. - Variance: This measures how spread out your sales figures are. Use
=VAR.P(A1:A12)
for population variance. In this example, variance equals $5,027,777.78. A higher variance indicates more fluctuation in sales. - Standard Deviation: This is the square root of the variance. Use
=STDEV.P(A1:A12)
. The standard deviation calculates to $2,241.12, helping you gauge the reliability of your average sales figure.
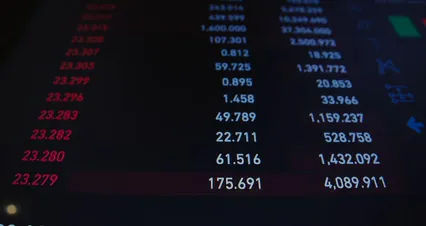
By analyzing these statistics, you gain insights into your sales performance. Understanding the mean helps you set realistic targets. The median offers a clearer picture of typical sales, while mode reveals popular sales levels. Variance and standard deviation inform you of consistency, allowing better forecasting and decision-making. Thus, descriptive statistics offer a powerful tool for managers using Excel to interpret their data effectively. For further reading, Naked Statistics: Stripping the Dread from the Data is a great book that explains these concepts in a fun and engaging way.
For a comprehensive guide on descriptive statistics in manufacturing plants, visit descriptive statistics in manufacturing plant.
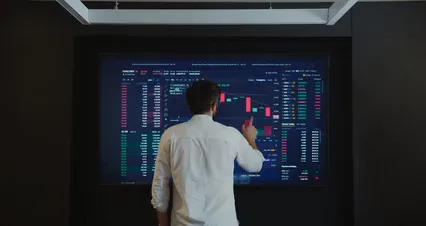
Inferential Statistics
Inferential statistics take us beyond mere observations. They allow managers to generalize findings from a sample to a larger population. Key concepts include hypothesis testing, confidence intervals, and p-values.
Hypothesis Testing: This involves formulating a null hypothesis (H0) and an alternative hypothesis (H1). For example, let’s say you want to evaluate whether a new marketing strategy has increased sales. Your H0 could be “there’s no difference in sales,” while H1 would suggest “sales have increased.”
Using Excel, you can perform a t-test. Suppose your sales data before the strategy was $10,000, and after it became $12,000. To perform a t-test, use the formula =T.TEST(array1, array2, tails, type)
. Set array1 as your pre-strategy data and array2 as your post-strategy data. This test will reveal if the difference in means is statistically significant.
Confidence Intervals: These provide a range of values that likely contain the population mean. For instance, if your sample mean is $12,000 with a standard deviation of $2,000, using Excel’s =CONFIDENCE(alpha, standard_dev, size)
function can help you calculate the confidence interval. This way, you can say, “I’m 95% confident that the true mean sales lie between $11,200 and $12,800.
P-values: These indicate the probability of obtaining results at least as extreme as those observed, under the assumption that the null hypothesis is true. A p-value less than 0.05 typically suggests rejecting H0. Use the output from your t-test to determine this value.
ANOVA: This method compares means across multiple groups. For instance, if you have sales data from three different regions, use the =ANOVA
function in Excel to analyze differences in sales performance across these areas.
Regression Analysis: This technique assesses relationships between variables. Suppose you want to see how advertising spend affects sales. Use Excel’s =LINEST(known_y's, known_x's)
function to find relationships and make predictions. For those looking to get deeper into regression, How to Measure Anything: Finding the Value of “Intangibles” in Business is a must-read!
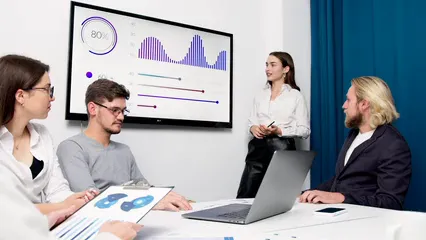
By mastering these concepts, managers can draw meaningful conclusions and make data-driven decisions. Excel’s capabilities make it easier to implement these statistical techniques, allowing for quicker and more informed choices.
Advanced Statistical Techniques
As managers grow comfortable with foundational statistics, exploring advanced techniques can unlock new insights. Multiple regression, time-series analysis, and business analytics are powerful tools that can enhance decision-making.
Multiple Regression: This technique assesses the relationship between one dependent variable and two or more independent variables. For instance, a manager may want to predict sales based on advertising spend, seasonality, and economic indicators. In Excel, you can use the =LINEST
function to find the regression coefficients. This allows you to determine how much each variable influences sales.
Time-Series Analysis: This technique analyzes data points collected or recorded at specific time intervals. For example, a business could analyze monthly sales over several years. Excel’s =FORECAST
function helps project future sales based on historical data. This method aids in identifying seasonal trends, allowing managers to plan inventory accordingly. If you’re also interested in learning about data science, check out Data Science for Business: What You Need to Know about Data Mining and Data-Analytic Thinking. It’s incredibly insightful!
Business Analytics: This integrates statistical analysis, predictive modeling, and data mining to improve business outcomes. For example, a manager might use Excel to segment customers based on purchasing behavior. Using clustering techniques can identify high-value customers, allowing for targeted marketing strategies.
In practical scenarios, these techniques can drive improvements. For example, a retailer could use multiple regression analysis to optimize marketing budgets across various channels. A company analyzing time-series data might adjust staffing during peak seasons based on historical trends.
By leveraging these advanced techniques, managers can make proactive decisions. Excel provides the tools to perform these analyses effectively, ensuring that businesses stay ahead of the competition. Engaging with these statistical methods can lead to greater efficiency and profitability, paving the way for success in a data-driven world.
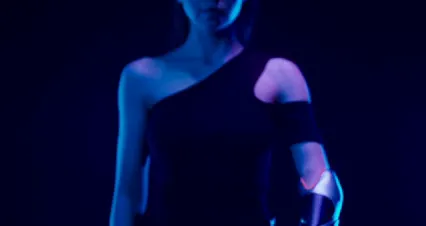
Please let us know what you think about our content by leaving a comment down below!
Thank you for reading till here 🙂
All images from Pexels