Introduction
Validity in statistics is a big deal. It’s all about whether your measurement tools actually measure what they claim to. Think of it like a bathroom scale that insists you’re five pounds lighter than reality. You might feel great, but that’s not valid!
Validity statistics pop up in various fields. You’ll see them in psychological testing, scientific research, and even data analysis. In psychology, for example, a test might claim to measure intelligence. If it instead measures how well a person can remember trivia, then we have a problem!
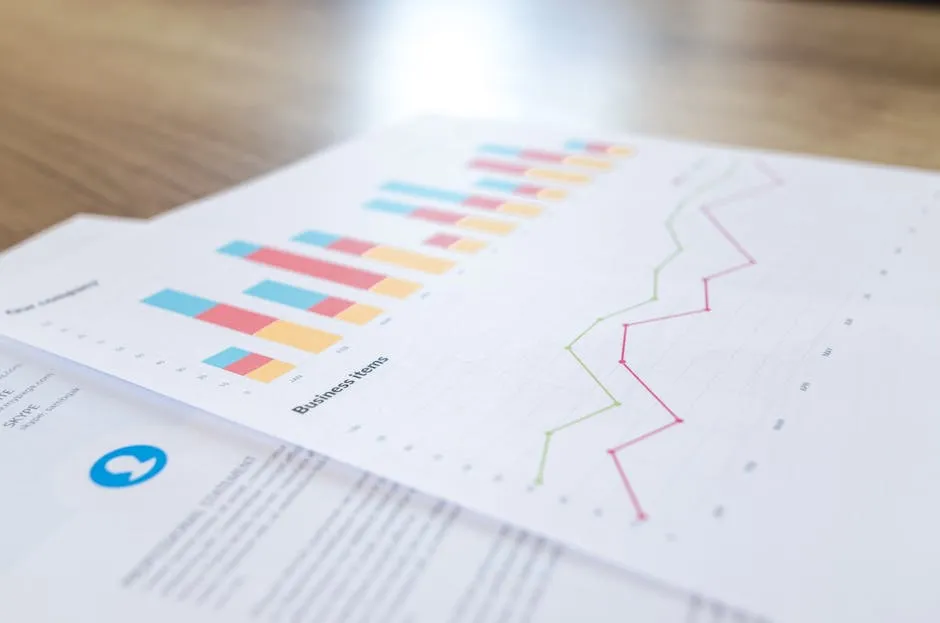
Understanding validity statistics is crucial for reliable results. If researchers misjudge validity, they can end up with conclusions that are as trustworthy as a fortune cookie. So, let’s get to the heart of validity statistics to ensure our results are not just accurate but also meaningful.
When researchers grasp validity, they can confidently interpret their findings. This understanding leads to better decisions in real-world applications, whether that’s developing a new drug or crafting a survey. In short, comprehending validity statistics empowers researchers to make informed choices that resonate with truth and reliability.
For those looking to deepen their understanding of statistical analysis, Statistical Analysis with Excel For Dummies is a fantastic resource. It breaks down complex concepts into digestible bites, making it easier for anyone to grasp statistical principles without feeling overwhelmed.
Understanding Validity Statistics
What Is Validity?
Validity refers to the degree to which a measurement accurately reflects the concept it aims to measure. Imagine a ruler that claims to measure length. If it always adds an inch, it’s not valid!
In statistics, validity is intertwined with reliability and measurement. Reliability is consistency, while validity is about accuracy. You can trust a measurement to be consistent (reliable), but if it’s measuring the wrong thing, it’s still not valid.
The core question of validity is simple yet crucial: “Does the instrument measure what it claims to measure?” For example, if a survey is designed to assess job satisfaction but instead probes social media habits, we have a mismatch.
Validity can be broken down into several types, each addressing different aspects of measurement. It’s a multi-faceted concept that researchers must consider to ensure their findings are sound. If a test is valid, it means the inferences drawn from it can be trusted.
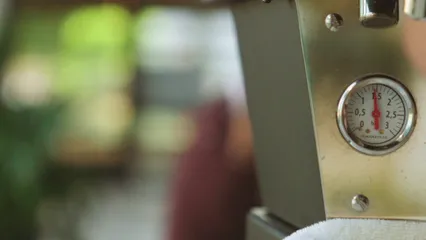
Researchers use various statistical techniques to establish validity. This can include methods like the Pearson correlation coefficient, which identifies relationships between measurements and accepted truths. Factor analysis helps reveal hidden connections among variables.
If you want to dive deeper into research methodologies, look no further than The Researcher’s Toolkit: A Guide to Validity and Reliability. This book offers essential insights into ensuring your research tools are both valid and reliable, helping you avoid those pesky pitfalls that can lead to misleading conclusions.
In summary, validity is the backbone of credible research. Without it, conclusions are as reliable as a weather forecast in a rainstorm. Understanding validity statistics lays the groundwork for more accurate, trustworthy outcomes in research.
Types of Validity
Validity isn’t a one-size-fits-all concept. It comes in several types, each crucial for different research contexts. Let’s break them down.
Construct Validity
Construct validity ensures that a measurement aligns with the theoretical construct it aims to assess. If researchers claim to measure happiness, is their tool really assessing that? Or is it merely gauging something like optimism?
Within construct validity, there are two important subtypes: convergent and divergent validity. Convergent validity checks if similar constructs yield similar results. For example, if two different tests measure intelligence, they should correlate positively. Divergent validity, on the other hand, ensures that different constructs yield distinct results. If an intelligence test correlates strongly with a measure of physical fitness, we might have a problem!
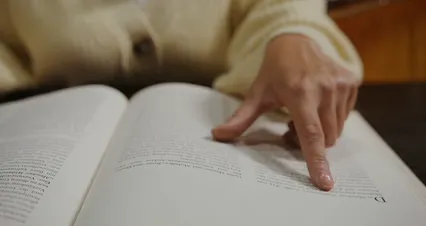
Construct validity is essential in research, especially in psychology, where intangible constructs like personality traits are common. If researchers can’t establish construct validity, their findings might be like trying to catch smoke with bare hands—impossible and elusive.
In research, ensuring strong construct validity means using well-defined measures and robust theoretical frameworks. This helps researchers confidently link their findings to broader theoretical implications, paving the way for more meaningful contributions to their fields.
To solidify your understanding of research designs, check out Research Methods in Psychology: Evaluating a World of Information. This book equips you with the tools to critically assess research findings and apply valid methods in your own studies.
In conclusion, construct validity is a critical aspect of validity statistics that researchers must prioritize. By ensuring their measurements align with the constructs they aim to study, researchers can provide more reliable and meaningful insights into human behavior and other complex phenomena.
Content Validity
Content validity is the measure of how well a test or questionnaire covers the entire range of the variable it aims to assess. Imagine you’re trying to gauge people’s dietary habits. If your survey only asks about fruit and vegetable consumption, it misses out on a whole buffet of eating behaviors, like fast food indulgence or midnight snack raids. That’s a recipe for incomplete data!
To ensure content validity, experts often review each item on a test or survey. They analyze whether the questions accurately represent the entire domain of the concept being measured. For instance, if a survey intends to assess job satisfaction, it should cover factors like work environment, salary, and growth opportunities—not just whether employees like the free coffee.
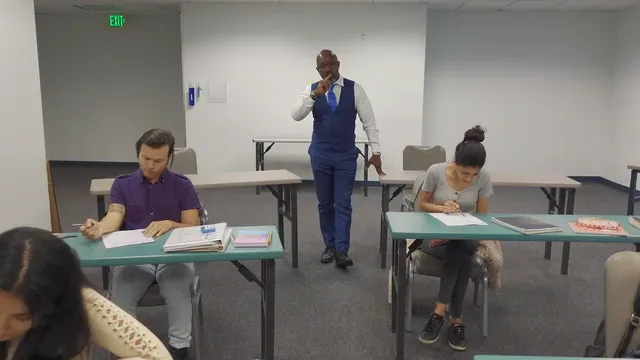
A classic example of content validity can be found in educational assessments. When designing a math test, educators must ensure it evaluates all necessary skills, from basic arithmetic to complex problem-solving. If the test only includes addition and subtraction, students might pass with flying colors, but their overall mathematical competence remains untested. By encompassing various topics, the test achieves better content validity.
If you’re interested in honing your skills in data analysis, grab a copy of The Essentials of Research Design and Data Analysis. This book provides a solid foundation for designing research that accurately captures the variables of interest.
In surveys, the same applies. A well-structured questionnaire on mental health should include questions about various aspects such as mood, sleep patterns, and social interactions. If it overlooks any of these areas, the results might paint a skewed picture of respondents’ mental health. Ultimately, achieving content validity is about ensuring comprehensive coverage of the variable, leading to richer and more reliable data.
Face Validity
Face validity refers to whether a test appears, at first glance, to measure what it purports to measure. It’s like the “gut feeling” we get when we look at a survey and think, “Yep, this looks like it’ll do the trick!” However, this form of validity is somewhat superficial and relies heavily on subjective judgment.
For example, consider a survey aimed at assessing anxiety levels. If it asks questions like, “Do you feel butterflies in your stomach?” and “Do you often feel restless?” it might seem appropriate on the surface. However, face validity does not guarantee that the questions effectively measure anxiety; they merely look like they do.
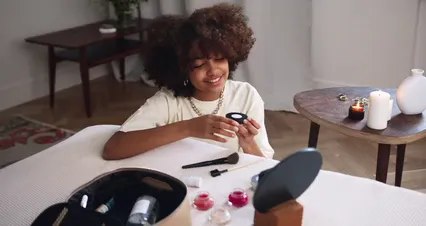
Assessing face validity often involves gathering feedback from experts or potential test-takers. Researchers might conduct focus groups or pilot studies to see if respondents feel the questions adequately capture the intended construct. If participants express confusion or suggest that some questions feel irrelevant, that’s a red flag!
While face validity helps in refining a measurement tool, it shouldn’t be the sole indicator of a test’s effectiveness. Just because something looks good doesn’t mean it is! Thus, researchers should complement face validity evaluations with more rigorous forms, such as content and construct validity, to ensure their measurements are not just pretty but also precise.
Internal Validity
Internal validity is the backbone of experimental research. It examines whether the relationship observed in a study genuinely reflects a cause-and-effect relationship rather than being influenced by extraneous variables. Essentially, it’s about ensuring that the independent variable is the main player in the observed changes in the dependent variable.
Imagine a study evaluating whether new teaching methods improve student performance. High internal validity means that changes in performance are directly linked to the teaching methods, not other factors like student motivation or prior knowledge. Researchers can enhance internal validity by using control groups, random assignment, and blinding techniques, which help filter out potential confounding variables.
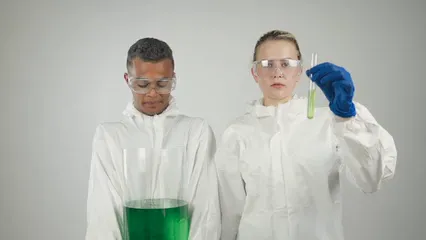
However, threats to internal validity lurk around every corner. One common culprit is selection bias, where the participants included in the study are not representative of the larger population. For instance, if only highly motivated students enroll in a study about a new educational approach, results may not apply to less motivated peers.
Another threat is history effects, where an outside event (like a school-wide initiative) coincides with the intervention, skewing results. To mitigate these risks, researchers can implement pre-tests and post-tests, ensuring that any observed changes are attributed to the intervention itself.
Ultimately, ensuring high internal validity allows researchers to confidently assert that their findings are meaningful. If researchers can’t control for these threats, their findings might be as reliable as a two-headed coin—flipping for luck rather than evidence.
External Validity
External validity pertains to the generalizability of research findings beyond the specific contexts in which they were conducted. Think of it as the ultimate test: can the results from your study apply to other people, places, and times? If the answer is yes, congratulations! You’ve achieved external validity.

However, various factors can threaten external validity. One major factor is the sample selection. If a study exclusively examines college students, its findings may not apply to older adults or children. Similarly, results from a study conducted in one geographical area might not translate to another region with different cultural dynamics.
Another potential threat is the experimental setting. Laboratory studies often create controlled environments that can differ significantly from real-world situations. For instance, if a study on stress management is conducted in a serene lab, the findings may not hold true for a chaotic office environment.
To bolster external validity, researchers can use diverse samples, replicate studies in different settings, and conduct longitudinal studies that observe changes over time. By doing so, they enhance the likelihood that their findings can be applied broadly, making their research more impactful.
For those interested in data analysis, SPSS for Dummies is a great starting point. This user-friendly guide breaks down complex statistical concepts into easily understandable chunks, making it a perfect companion for beginners.
In conclusion, understanding both internal and external validity is crucial for robust research. Researchers must navigate these waters carefully to ensure their findings resonate beyond the pages of a study, influencing real-world applications and decisions.
Statistical Conclusion Validity
Statistical conclusion validity (SCV) is a critical component of research. It assesses how well the statistical analyses support the conclusions drawn from data. Think of it as the glue that holds a study’s findings together. When SCV is strong, you can trust that your data analysis accurately reflects the reality of the findings.
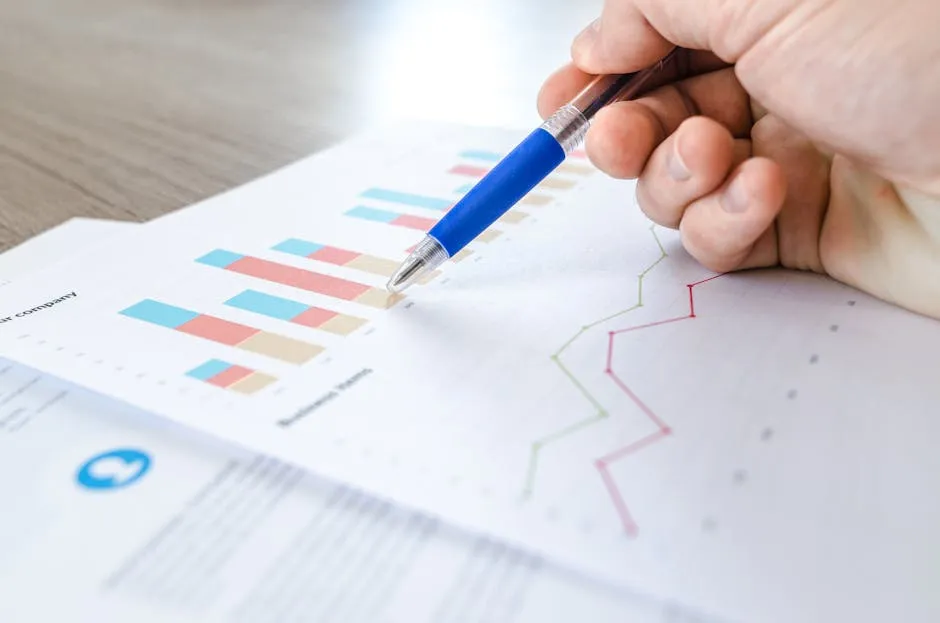
However, threats to SCV can rear their ugly heads. One common issue is the problem of low statistical power. If your study doesn’t have enough participants, you might miss significant effects, leading to Type II errors. This is like trying to catch a fish with a tiny net; you might end up with nothing. To counteract this, researchers should plan for an adequate sample size from the get-go.
Another menace is the misuse of statistical tests. Often, researchers may apply inappropriate methods that don’t fit the data characteristics, leading to misleading conclusions. For example, using regression analysis without checking if assumptions hold can produce results that are as trustworthy as a politician’s promise. The remedy here? Always ensure that the statistical tests align with the data type and research questions.
Type I errors—concluding that a relationship exists when it doesn’t—are another significant threat. They often arise from practices like optional stopping or repeated testing without proper controls. To maintain rigor, researchers should stick to fixed sampling protocols, which help keep Type I error rates in check. The COAST rule is one such framework that can guide researchers in this endeavor.
If you’re keen to sharpen your statistical skills, consider picking up Python for Data Analysis: Data Wrangling with Pandas, NumPy, and IPython. This book provides a hands-on approach to working with data, making it a must-have for aspiring data scientists.
Lastly, assumptions underlying statistical tests must be checked before diving into data analysis. Neglecting this step is like driving a car without checking the brakes first—risky and potentially disastrous! Preliminary tests can help identify whether the data meets the necessary conditions for analysis, thus bolstering SCV.
In summary, ensuring statistical conclusion validity requires vigilance. Researchers must be aware of potential pitfalls and employ strategies to mitigate them. By doing so, they can confidently assert that their findings are both accurate and reliable, effectively translating their research into actionable insights.
Challenges in Establishing Validity
Establishing validity is akin to navigating a minefield. Researchers encounter various challenges that can trip them up. One of the most significant hurdles is sample size. A small sample may lead to misleading results. After all, a study with a handful of participants is like trying to judge a blockbuster movie based on a single critic’s review. You need a broader audience to get a true sense of the film’s worth.

Measurement errors also wreak havoc on validity. Imagine conducting a survey about people’s eating habits using a faulty scale. If the scale misreads, the data collected will be skewed. This is true across various fields, from psychology to medicine. Therefore, ensuring precise measurement tools is vital for accurate data interpretation.
Statistical assumptions present another challenge. Researchers often work with specific statistical methods that come with their own set of assumptions. If these assumptions are violated, the results can spiral out of control. For instance, many statistical tests assume that data is normally distributed. If your data resembles a lopsided pancake instead, you might be in trouble.
Moreover, understanding the context in which the research is conducted is crucial. If the study is too context-specific, its findings may lack generalizability. For instance, a study conducted in a small town may not apply to urban populations. This limitation can lead to a false sense of security about the findings.
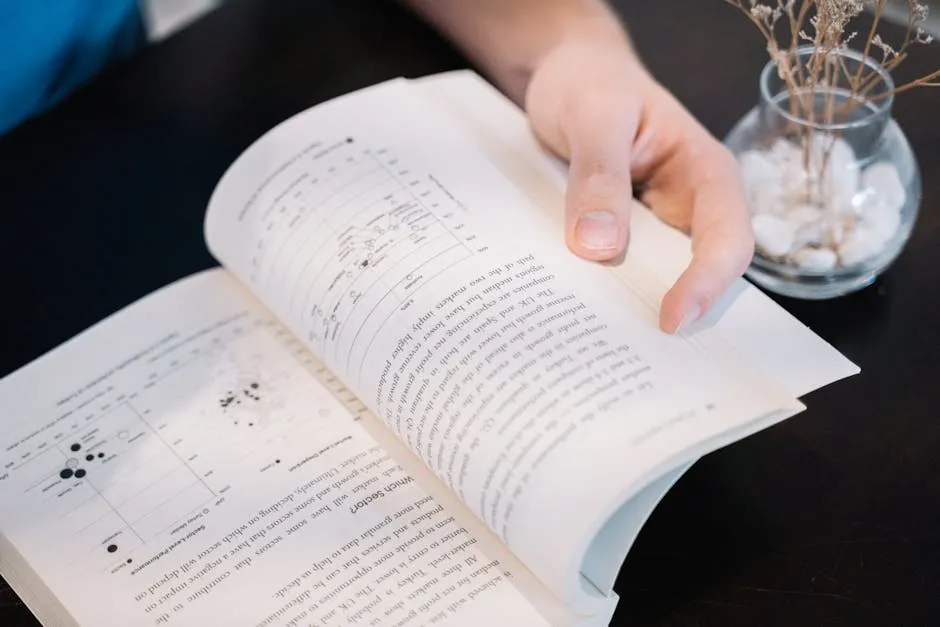
To further enhance your research skills, consider The Essentials of Statistics. This book provides a comprehensive overview of statistical principles that can help strengthen your understanding of measurement and analysis.
Additionally, researchers may face challenges related to bias. Bias can creep in through various channels, such as participant selection or data collection methods. If certain groups are over- or under-represented, the validity of the conclusions drawn can be compromised. It’s like trying to conduct a political poll but only surveying people in one political party; the results will hardly reflect the larger population.
Lastly, the evolving nature of research practices and technologies can complicate validity assessments. As new methodologies emerge, researchers must stay updated. Falling behind could mean relying on outdated practices that undermine the validity of their findings.
In summary, establishing validity is no small feat. Researchers must grapple with sample size, measurement errors, statistical assumptions, context, bias, and evolving methodologies. Addressing these challenges is essential to ensure that their conclusions are both reliable and meaningful.
Conclusion
To wrap things up, understanding validity statistics is essential for conducting credible research. From defining what validity means to exploring its various types, we’ve covered a lot of ground. Each type of validity plays a distinct role in ensuring that research findings accurately reflect reality.
Researchers face multiple challenges in establishing validity, including issues related to sample size, measurement errors, and the need for rigorous statistical assumptions. These challenges can distort results and lead to unreliable conclusions if not adequately addressed. Thus, a deep comprehension of these elements can significantly enhance the quality of research outcomes.

If you want to level up your research design skills, Research Design: Qualitative, Quantitative, and Mixed Methods Approaches is a must-read. This book provides a comprehensive overview of various research designs that can help ensure your studies are robust and valid.
Incorporating the concepts of validity into your research endeavors is not just beneficial but necessary. It ensures that the conclusions drawn are based on sound data and reliable methods. So, the next time you’re designing a study or analyzing data, remember the importance of validity statistics. They are your best friends in the quest for accurate and trustworthy research. Engage with these concepts, and you’ll pave the way for findings that truly reflect the complexities of the world around us. Your future research will thank you!
FAQs
What is the difference between validity and reliability?
Validity and reliability are like the peanut butter and jelly of research. They work best together but serve different purposes. Validity checks if your measurement truly captures what it claims to measure. Reliability, on the other hand, ensures that your measurement produces consistent results over time. You can have a reliable measure that’s still not valid. It’s like a clock that ticks perfectly but is stuck on the wrong time.
Why is face validity considered the weakest form of validity?
Face validity is like judging a book by its cover. It assesses whether a test appears to measure what it’s supposed to measure at first glance. However, this form of validity relies heavily on subjective judgment. Just because a survey looks good doesn’t mean it’s actually measuring the right thing. It’s essential to supplement face validity with more rigorous assessments, such as content and construct validity, to ensure accuracy.
How can researchers ensure high levels of construct validity in their studies?
To achieve high construct validity, researchers should use well-defined measures that align with the theoretical constructs being studied. This means carefully defining what you’re measuring and ensuring that your tools accurately reflect that. Employing techniques like factor analysis can help identify relationships between measurements. Additionally, researchers should validate their tools against other established measures of the same construct to ensure they are capturing the intended concept.
What are common statistical methods used to assess validity?
Several statistical methods help assess validity, each with its unique flair. The Pearson correlation coefficient measures the relationship between two variables, showing how closely they align. Factor analysis helps identify underlying relationships among variables, helping researchers understand the structure of their data. Regression analysis models relationships between variables, allowing researchers to draw conclusions about the influence of one variable on another. By using these techniques, researchers can bolster the validity of their findings.
How does sample size affect the validity of a study?
Sample size is the unsung hero of research validity. A small sample can lead to skewed results, making your conclusions as trustworthy as a rumor. Larger sample sizes help ensure that findings are more representative of the population. They also enhance the statistical power of a study, reducing the risk of Type I and Type II errors. So, when it comes to sample size, bigger is often better for solidifying your study’s validity.
Can validity change over time?
Absolutely! Validity is not a one-and-done deal. As new theories emerge and contexts change, the validity of a measurement can shift. For instance, a test that was once deemed valid may no longer accurately reflect the intended construct in a different cultural or temporal context. Researchers must regularly assess and update their measures to ensure they maintain validity over time. This ongoing evaluation helps keep research relevant and trustworthy.
How can researchers improve the validity of their studies?
Researchers can enhance validity by employing a mixed-methods approach, combining qualitative and quantitative data to provide a fuller picture. They should also pilot test their measures to identify any issues before the main study. Additionally, collaborating with experts in the field can provide valuable insights into the appropriateness of measurement tools. By taking these steps, researchers can significantly improve the validity of their studies, ensuring that their findings are both meaningful and applicable.
Please let us know what you think about our content by leaving a comment down below!
Thank you for reading till here 🙂
All images from Pexels