Introduction
Statistics plays a vital role in our lives. From the medical field to business, it helps us make sense of the endless streams of data we encounter daily. Imagine a doctor determining the effectiveness of a new treatment based on patient data or a business evaluating market trends to understand customer preferences. Statistics is the backbone of informed decision-making.
Understanding statistics is not just for data scientists or mathematicians. It’s essential for everyone. By grasping the two main branches—descriptive and inferential statistics—you can better interpret data and make sound decisions based on evidence. These branches provide the tools to summarize, analyze, and draw conclusions from data sets.
Consider how businesses use statistics for market research. They analyze consumer behaviors and preferences, enabling them to tailor products and marketing strategies effectively. Without statistics, decisions would be based purely on gut feelings rather than solid data. If you’re looking for a comprehensive guide on the subject, check out Statistics for Dummies. It’s a great starting point!
This article will explore the distinctions, purposes, methods, and applications of descriptive and inferential statistics. By the end, you’ll understand how these branches contribute to effective data interpretation and decision-making in various fields.
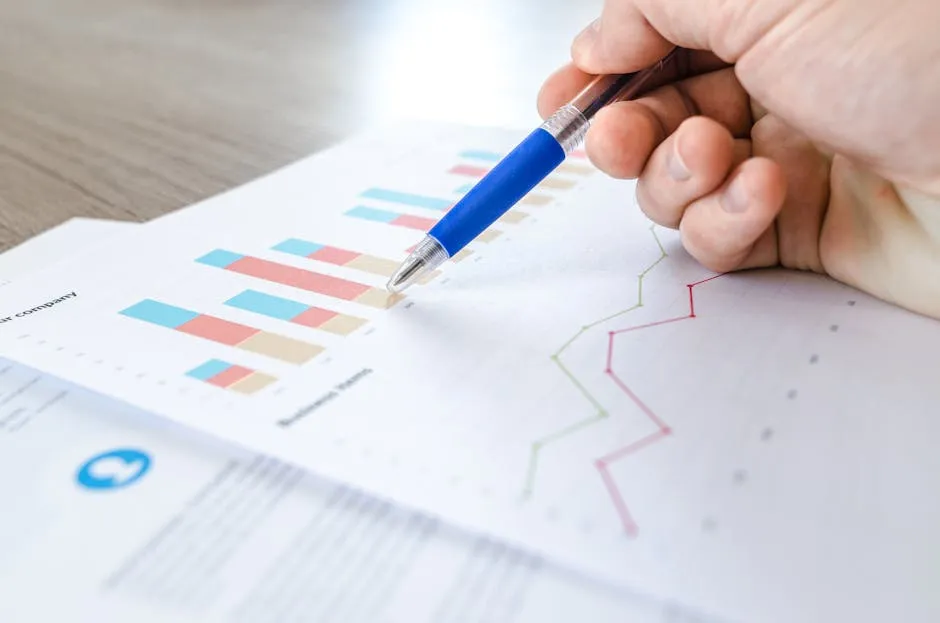
The Two Main Branches of Statistics
Descriptive Statistics
Definition and Purpose
Descriptive statistics serves as the foundation of data analysis. It focuses on summarizing and organizing data to give a clear overview of its main features. Think of it as the friendly tour guide of the data world. It tells you what’s in the dataset without making any wild assumptions about what it means.
In essence, descriptive statistics transforms raw data into meaningful insights. It helps researchers and analysts to present their findings in a straightforward manner, making it easier for others to digest the information. Whether you’re looking at a handful of numbers or a colossal dataset, descriptive statistics provides clarity and context.
To delve deeper into the world of statistics, consider picking up Naked Statistics: Stripping the Dread from the Data. It’s a witty and engaging read that makes statistics approachable!
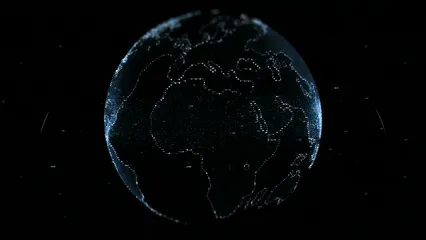
Key Components
Measures of Central Tendency
These measures help us pinpoint the center of a dataset. They include the mean, median, and mode, each offering unique insights.
Mean: The mean is simply the average. You calculate it by adding up all the values and dividing by the number of values. For example, if you have the test scores of five students—85, 90, 75, 80, and 95—the mean score is (85 + 90 + 75 + 80 + 95) / 5 = 85. It’s like a group hug for all the numbers, bringing them together as one. For more insights, you can check out what does mean identically distributed in statistics here.
Understanding the mean is crucial in statistics, as it represents the average of a dataset. what does mean identically distributed in statistics
Median: This is the middle value when all numbers are arranged in order. If we take our earlier test scores and add a score of 70, the ordered list becomes 70, 75, 80, 85, 90, 95. The median score is 82.5, providing a measure that’s less affected by extreme values. For more on median salary insights, check out the statistics poland median salary 2024 here.
The median is a vital measure of central tendency, particularly in datasets with outliers. statistics poland median salary 2024
Mode: The mode is the most frequently occurring value in a dataset. For instance, if the test scores are 85, 90, 85, 80, and 95, the mode is 85. It’s like the popular kid in school—everyone seems to gravitate towards it! For more on residuals in statistics, see the comprehensive guide to statsmodels residuals statistics here.
The mode provides insights into the most common values in a dataset, which can be critical for data analysis. statsmodels residuals statistics

Measures of Dispersion
While measures of central tendency tell us where the center lies, measures of dispersion show us how spread out the data is.
Range: The range is the difference between the highest and lowest values. For our test scores, if the highest score is 95 and the lowest is 70, the range is 95 – 70 = 25. It’s a quick way to gauge how wide the scores are spread out. For a broader look at crime statistics, you can explore the orange county ca crime statistics here.
Understanding the range is essential for grasping the spread of data in any analysis. orange county ca crime statistics
Variance and Standard Deviation: Variance measures how far each number in the set is from the mean and thus from every other number. Standard deviation is the square root of variance, providing a more interpretable figure. A low standard deviation means the data points are close to the mean, while a high standard deviation indicates they are spread out over a wider range. These two metrics are essential for understanding the consistency of the data.
Interquartile Range (IQR): The IQR measures the range within which the middle 50% of values lie. It’s calculated by finding the difference between the first quartile (25th percentile) and the third quartile (75th percentile). For instance, if the 25th percentile is 75 and the 75th percentile is 90, then the IQR is 90 – 75 = 15. The IQR is particularly useful as it helps mitigate the effect of outliers.
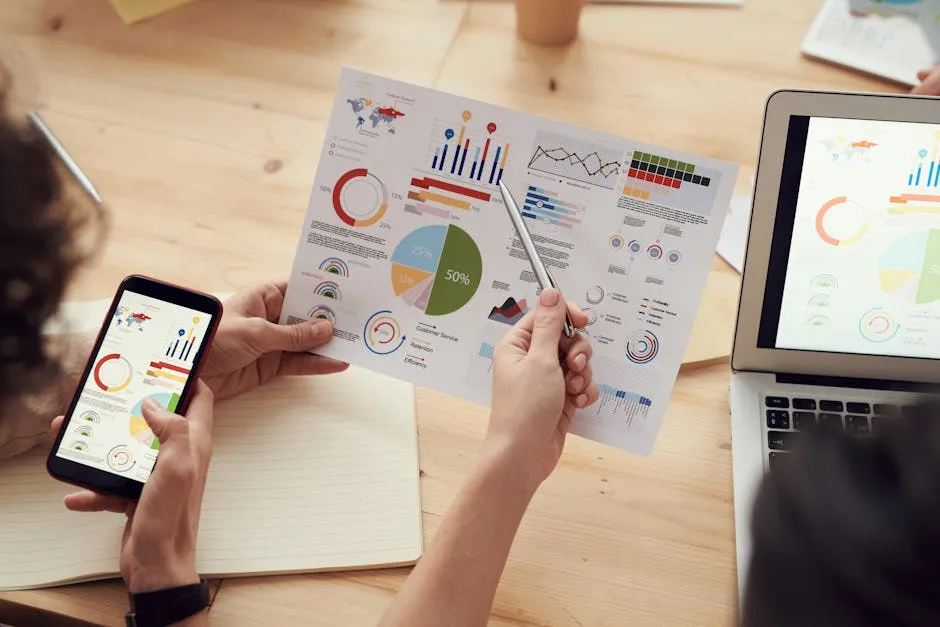
Visualization Techniques
Graphs and charts are the cherry on top of descriptive statistics. They serve as visual aids, making it easier to understand complex data at a glance.
Bar Charts: These are perfect for comparing different categories. Imagine you have data on the number of pets owned by different households. A bar chart can quickly show you which type of pet is the most popular. If you’re interested in learning more about effective data visualization, consider The Visual Display of Quantitative Information. It’s a classic!
Histograms: When you want to see the distribution of a continuous variable, histograms are your best friends. They group data into ranges, making it easier to visualize how many values fall within each range.
Pie Charts: These visually represent proportions of a whole. Want to show how much of your budget goes to food, rent, and entertainment? A pie chart can give your audience a quick snapshot.
By employing these visualization techniques, descriptive statistics not only summarizes data but also presents it in a way that is easy to digest. In a world bursting with information, that’s a pretty big deal.
In summary, descriptive statistics plays a crucial role in simplifying data analysis. It summarizes key aspects of data, providing clarity and understanding. Through various measures of central tendency and dispersion, along with powerful visualization techniques, descriptive statistics transforms raw numbers into meaningful narratives. This foundation sets the stage for the more complex world of inferential statistics, where predictions and conclusions about larger populations can be drawn from the summarized data.

Inferential Statistics
Definition and Purpose
Inferential statistics is like the Sherlock Holmes of data analysis. It uses a sample of data to make predictions or inferences about a larger population. Imagine you’re at a party and want to guess how many people like chocolate ice cream. You can’t ask everyone, but you can ask a few. Based on their responses, you can make an educated guess about the entire party.
The primary role of inferential statistics is to provide insights beyond the immediate data at hand. It helps researchers and analysts draw conclusions that apply to a broader group without needing to collect data from every individual. This is particularly useful in fields where surveying an entire population is impractical or impossible. If you’re interested in a deeper understanding of these concepts, check out The Data Warehouse Toolkit: The Definitive Guide to Dimensional Modeling. It’s a fantastic resource!
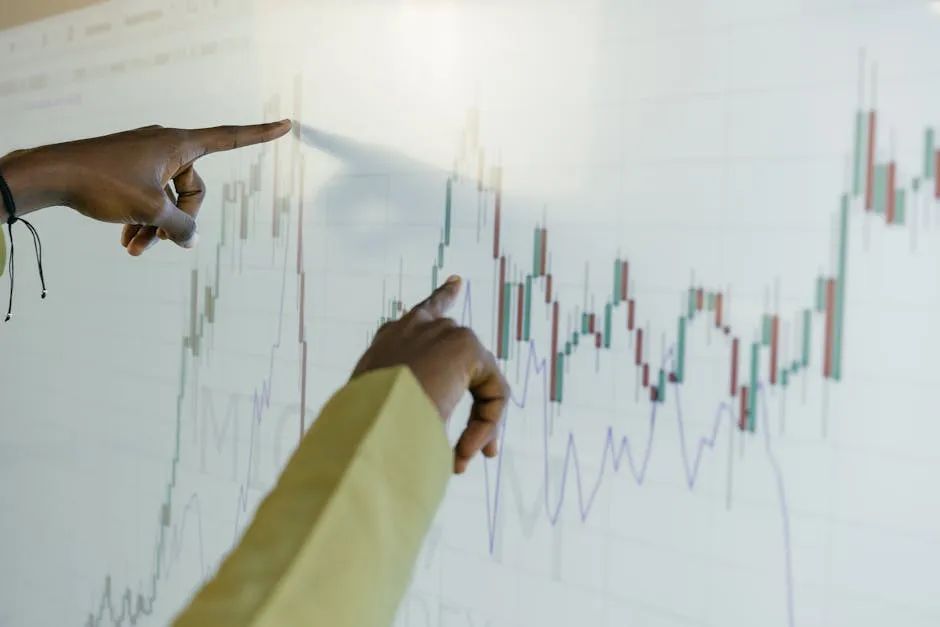
Key Techniques
Hypothesis Testing
Hypothesis testing is a cornerstone of inferential statistics. It involves making an initial assumption (the null hypothesis) and testing it against data. For a comprehensive guide, refer to the statistics hypothesis testing cheat sheet here.
Hypothesis testing is critical for validating assumptions in data analysis. statistics hypothesis testing cheat sheet
Null vs. Alternative Hypothesis: The null hypothesis (H0) posits that there’s no effect or difference. In contrast, the alternative hypothesis (Ha) suggests there is a significant effect or difference. For example, if a new teaching method is implemented, H0 might state that it has no effect on student performance, while Ha would argue that it does improve performance.
If enough evidence from sample data supports Ha, we reject H0. If not, we fail to reject it. This process is akin to a courtroom trial where the evidence determines the verdict.

Confidence Intervals
Confidence intervals provide a range of values within which we expect the true population parameter to lie. By conducting a survey, you might find that the average height of teenage boys in your town is 65 inches, with a confidence interval of 63 to 67 inches. This means you can be, say, 95% confident that the true average height of all teenage boys in the town falls within this range. It’s like setting up a safety net—a way of expressing uncertainty while still providing useful information.
If you want to enhance your understanding of data science principles, consider reading Data Science for Business: What You Need to Know about Data Mining and Data-Analytic Thinking. It’s a fantastic resource for understanding how to apply statistical principles in business!
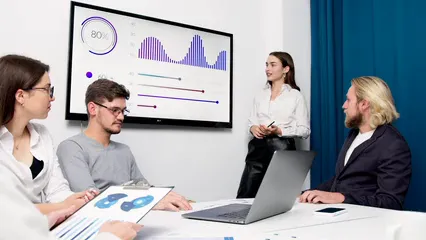
Regression Analysis
Regression analysis is another powerful technique in inferential statistics. It examines the relationship between variables.
Overview: Linear regression, for instance, helps predict one variable based on another. Suppose you want to predict a person’s weight based on their height. By plotting height against weight, you can draw a line that best fits the data points. This line helps you estimate weight for any given height, making it a handy tool in various fields, from health to economics.

Sampling Techniques
Sampling methods are crucial in inferential statistics. They ensure that the sample accurately represents the larger population.
Types of Sampling:
- Random Sampling: Every member of the population has an equal chance of being selected. It’s like drawing names from a hat—fair and square.
- Stratified Sampling: This approach divides the population into subgroups and samples from each one. Imagine you want opinions from different age groups; stratified sampling ensures every age group is represented.
- Systematic Sampling: In this method, you select every nth member from a list. If you have a list of 100 people and want to select every 10th person, you’d end up with a systematic sample that maintains an orderly selection process.
For those who want a solid foundation in statistical inference, consider reading Statistical Inference for Data Science. It’s a great way to expand your knowledge!
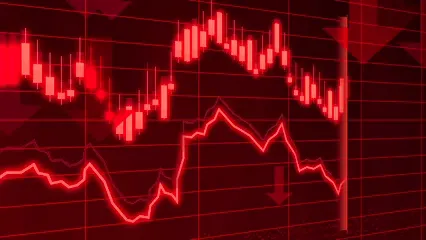
Importance of Inferential Statistics
Generalization: The magic of inferential statistics lies in its ability to generalize findings from a sample to a larger population. This generalization allows researchers to make educated guesses and predictions based on limited data, which is particularly valuable in fields such as medicine, where testing every individual is often unfeasible.
Real-Life Applications: Inferential statistics shines in various real-world scenarios. Consider polling results during elections. Pollsters use inferential statistics to predict the outcome by surveying a small portion of the electorate.
Another example is market research. Companies often rely on inferential statistics to gauge consumer preferences. By surveying a sample of customers, businesses can infer trends and make strategic decisions about product launches or marketing campaigns. For a deeper dive into effective data analysis, check out The Complete Guide to Data Analysis with Python. It’s a fantastic resource for practical applications!
In summary, inferential statistics plays an essential role in interpreting data and drawing conclusions about larger populations. Through techniques like hypothesis testing, confidence intervals, regression analysis, and various sampling methods, it empowers researchers to make informed decisions based on limited information. Whether predicting election outcomes or assessing consumer behavior, inferential statistics is an invaluable tool in the data analyst’s toolkit.

Comparison of Descriptive vs. Inferential Statistics
Understanding the differences between descriptive and inferential statistics is essential for anyone working with data. Let’s break down these two powerful branches.
Key Differences
Purpose: The primary aim of descriptive statistics is to summarize data. Think of it as a highlighter, drawing attention to key features of the dataset. It gives you the big picture without diving into the nitty-gritty. On the other hand, inferential statistics takes a step further. It allows analysts to make inferences or predictions about a larger population based on a sample. It’s like looking at a tiny piece of a puzzle and guessing the whole picture.
Data Used: Descriptive statistics works with complete datasets. It’s the equivalent of having all the pieces of a jigsaw puzzle laid out in front of you. You can easily describe the entire image. In contrast, inferential statistics relies on sample data. You gather a small portion and use it to draw conclusions about the larger group. It’s a bit like tasting a spoonful of soup to determine if the whole pot is seasoned just right.
Outcome: The outcome of descriptive statistics is the presentation of data in a clear, understandable format. This includes graphs, charts, and summary statistics. It’s all about making the information digestible. Conversely, the outcome of inferential statistics is predictions or conclusions about a broader population. It’s the leap from the known to the unknown, giving you insights that extend beyond the immediate data.
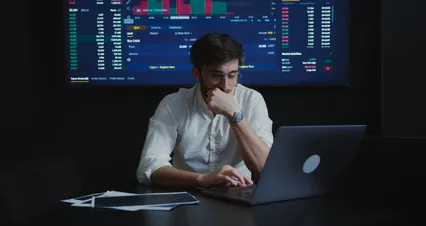
When to Use Which
So, when should you use descriptive versus inferential statistics? Here are some guidelines.
- Descriptive Statistics: Use this branch when you have all the data available. It’s perfect for summarizing and presenting information in reports. For instance, if you’re preparing a business report on last quarter’s sales figures, descriptive statistics will help you showcase trends and averages clearly.
- Inferential Statistics: This is your go-to when you want to make predictions or generalizations. For example, if you’re conducting a survey about customer satisfaction, you might only poll a sample of your customers. Inferential statistics can help you infer how the entire customer base feels based on that sample.
For a practical guide to data visualization techniques, consider Data Visualization: A Practical Introduction. It’s a great resource for improving your data presentation skills!
In summary, both branches are crucial in the world of statistics. Descriptive statistics gives clarity, while inferential statistics provides deeper insights. Knowing when to use each can enhance your data analysis and decision-making processes. Whether you’re reporting on data trends or making projections about future outcomes, these statistical tools are indispensable.
With these differences in mind, you’re better equipped to tackle your data analysis challenges. Whether summarizing data or making predictions, mastering both descriptive and inferential statistics will serve you well in any analytical endeavor.
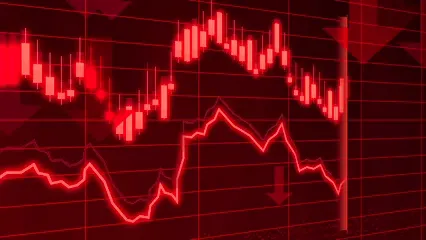
FAQs
What is the difference between descriptive and inferential statistics?
Descriptive statistics summarizes and organizes data. It highlights key features of a dataset, often using visual aids like graphs and charts. Think of it as a data snapshot. On the flip side, inferential statistics makes predictions about a population based on sample data. It’s like using a small slice of cake to guess the flavor of the whole cake. While descriptive statistics provides clarity, inferential statistics enables broader conclusions.
Why is inferential statistics important?
Inferential statistics is crucial for making predictions and generalizations. It allows researchers to infer trends and patterns that extend beyond their sample. For example, in healthcare, a study may analyze a small group of patients to predict treatment outcomes for a larger population. This branch of statistics informs decisions in various fields, from politics to marketing, empowering organizations to strategize based on data-driven insights.
How can I apply descriptive statistics in everyday life?
Descriptive statistics pops up everywhere in daily life! When you check your favorite restaurant’s ratings online, you’re looking at averages and distributions of reviews. If you track your monthly expenses using a spreadsheet, you summarize your spending habits with averages and ranges. Even tracking your daily steps on a fitness app uses descriptive statistics to show your progress over time. These everyday examples illustrate how descriptive statistics helps us make informed choices.
Can you give examples of both branches in real-life scenarios?
Absolutely! In healthcare, descriptive statistics might summarize patient demographics in a hospital. For instance, a report could show that 60% of patients are aged 50 or older. On the inferential side, researchers might conduct a study on a sample of patients to predict the effectiveness of a new drug for the entire population. In business, descriptive statistics can analyze sales data by showing average monthly sales, while inferential statistics can help forecast future sales trends based on sample data.
What tools are commonly used for statistical analysis?
Several tools are popular among statisticians for data analysis. R and Python are versatile programming languages that offer powerful libraries for statistical modeling. SPSS is user-friendly software ideal for social sciences, while Excel is widely used for basic statistical functions and data visualization. Each of these tools has unique capabilities, catering to both beginners and seasoned analysts, making data analysis accessible for everyone.

Please let us know what you think about our content by leaving a comment down below!
Thank you for reading till here 🙂
All images from Pexels