Introduction
Lurking variables are sneaky little devils in the world of statistics. They’re the uninvited guests at the party of data analysis. These variables aren’t included in a study, yet they can significantly impact the relationships being examined. Think of them as the shadowy figures lurking in the background, quietly influencing outcomes without anyone noticing.
Ignoring lurking variables is like attempting to bake a cake without considering the oven temperature. It may seem fine on the surface, but you’re likely to end up with a gooey mess. The consequences of neglecting these variables can be severe. They can introduce bias, distort statistical results, and lead to misleading conclusions. Imagine concluding that coffee consumption increases heart disease while ignoring that smoking, a lurking variable, could be the real culprit. Oops!
In this article, you’ll dive deeper into the world of lurking variables. You’ll learn what they are, how they differ from confounding variables, and the havoc they can wreak on your analysis. We’ll also explore the concept of omitted variable bias, and of course, we’ll sprinkle in some amusing examples along the way. By the end, you’ll be equipped with knowledge that can save your research from the clutches of lurking variables. Buckle up for an enlightening journey!
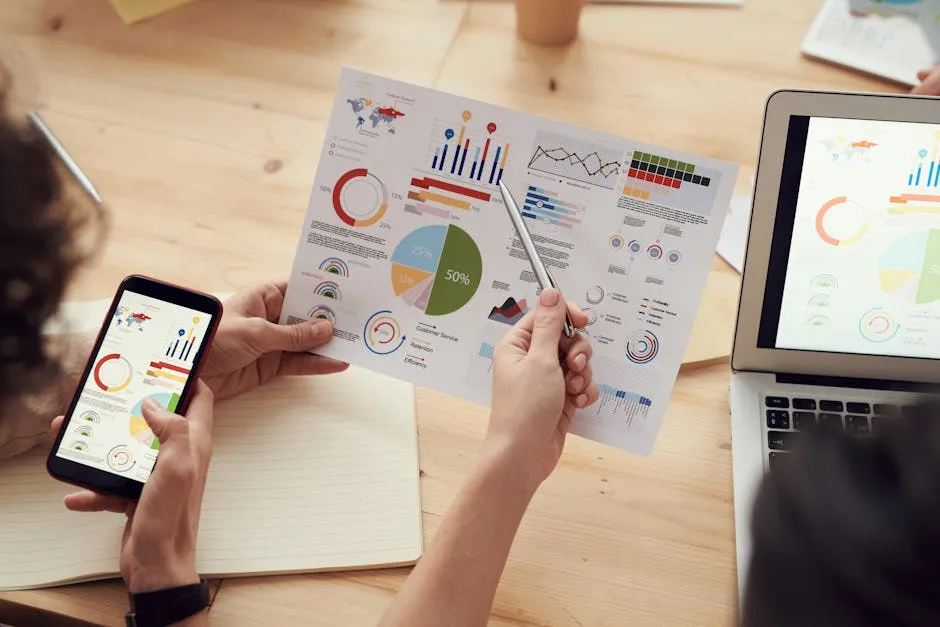
What is a Lurking Variable?
A lurking variable is a hidden factor that isn’t accounted for in a statistical analysis but can influence the relationship between the variables being studied. Imagine you’re investigating the correlation between education level and income. If you don’t consider work experience—a lurking variable—you might conclude that education directly causes higher income. In reality, work experience plays a significant role in salary, and ignoring it can lead to misleading interpretations.
Now, let’s differentiate lurking variables from confounding variables. While both can affect your results, the key distinction lies in awareness. Lurking variables are unknown to researchers and thus excluded from the analysis. Conversely, confounding variables are recognized and often included in the model. For instance, if a researcher knows that age influences both exercise and heart health but fails to include it in the study, age is a confounding variable.
Omitted variable bias is another concept closely tied to lurking variables. It occurs when a variable that should be included in the analysis is left out, resulting in distorted estimates of the effects of other variables. Picture this: a study examines how diet affects blood pressure, neglecting to account for stress levels. Stress, acting as a lurking variable, could influence blood pressure independently, leading to incorrect conclusions about diet alone.
To illustrate lurking variables, let’s look at some examples. In health studies, researchers might find a positive correlation between physical activity and lower blood pressure. However, if they overlook weight as a lurking variable, they may miss that the correlation exists because lighter individuals tend to be more active. In social science research, consider ice cream sales and crime rates. Both tend to rise during warm weather, but if researchers fail to account for temperature as a lurking variable, they might mistakenly infer a direct relationship between the two.
Lurking variables can wreak havoc in statistical analyses. By remaining unidentified, they obscure true relationships and lead to erroneous conclusions. Therefore, it’s essential to understand and identify these hidden factors to ensure your research is robust and reliable. And speaking of being robust, if you’re looking to level up your data analysis skills, consider picking up a copy of Statistics for Dummies. It’s a great resource for anyone who wants to demystify the world of numbers!
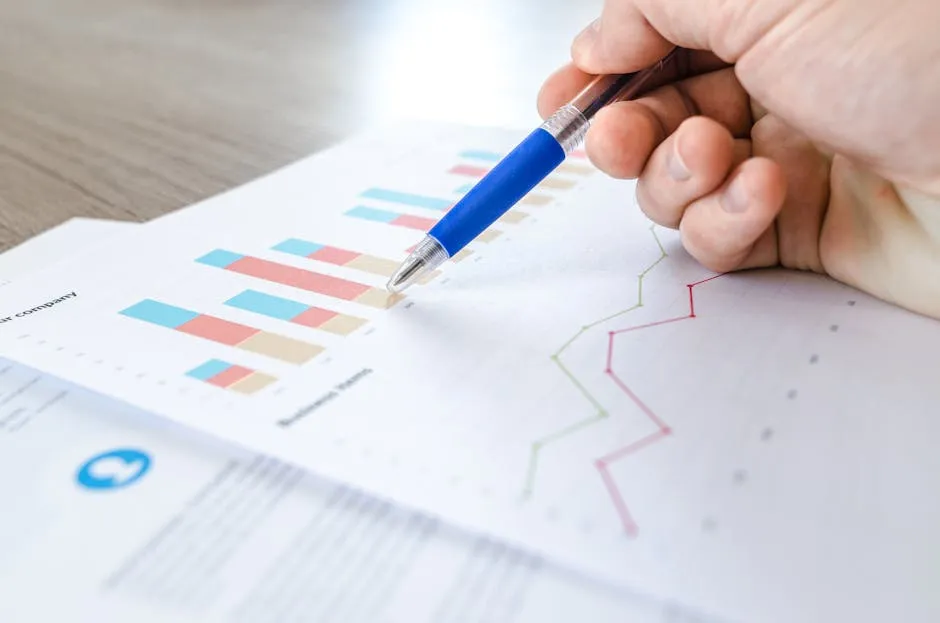
The Importance of Identifying Lurking Variables
Identifying lurking variables is essential for research accuracy and credibility. These sneaky factors can distort relationships and mislead interpretations. Ignoring them is like trying to spot a unicorn in a crowded zoo—you might think you found one, but it’s just a horse wearing a party hat!
Lurking variables can obscure the truth behind the data. For instance, consider a study examining the link between exercise and weight loss. If researchers fail to account for dietary habits—a lurking variable—they might incorrectly conclude that exercise alone drives weight loss. In reality, diet plays a pivotal role. If you’re serious about understanding how to analyze these relationships, you might benefit from The Data Warehouse Toolkit. It’s a fantastic guide for mastering data modeling!
When lurking variables lurk unnoticed, they can magnify or diminish the perceived relationship between independent and dependent variables. Let’s say you’re investigating the connection between education level and income. If you don’t consider work experience—a lurking variable—you might think education directly dictates income. But work experience can significantly influence earning potential, leading to misguided conclusions.
The consequences of neglecting lurking variables can be severe. For example, a study might find a positive correlation between coffee consumption and heart disease. However, if smoking, a lurking variable, is overlooked, researchers might falsely attribute heart disease risks solely to coffee. In this case, smoking is the real culprit, clouding the analysis. To delve deeper into the complexities of data science, check out The Data Science Handbook. It’s packed with insights from industry leaders!
Real-world examples abound. Researchers have explored the relationship between ice cream sales and shark attacks. Both tend to rise during summer months. But if the temperature, a lurking variable, isn’t considered, it could lead to the erroneous conclusion that ice cream consumption causes shark attacks. Spoiler alert: it doesn’t! Warmer weather increases beachgoers and ice cream sales alike.
In summary, identifying lurking variables is crucial for accurate research. They can distort relationships, lead to incorrect conclusions, and ultimately undermine the credibility of findings. By shining a light on these hidden influences, researchers can ensure their analyses reflect the true picture. So, keep those lurking variables in check to avoid research pitfalls and unnecessary surprises!
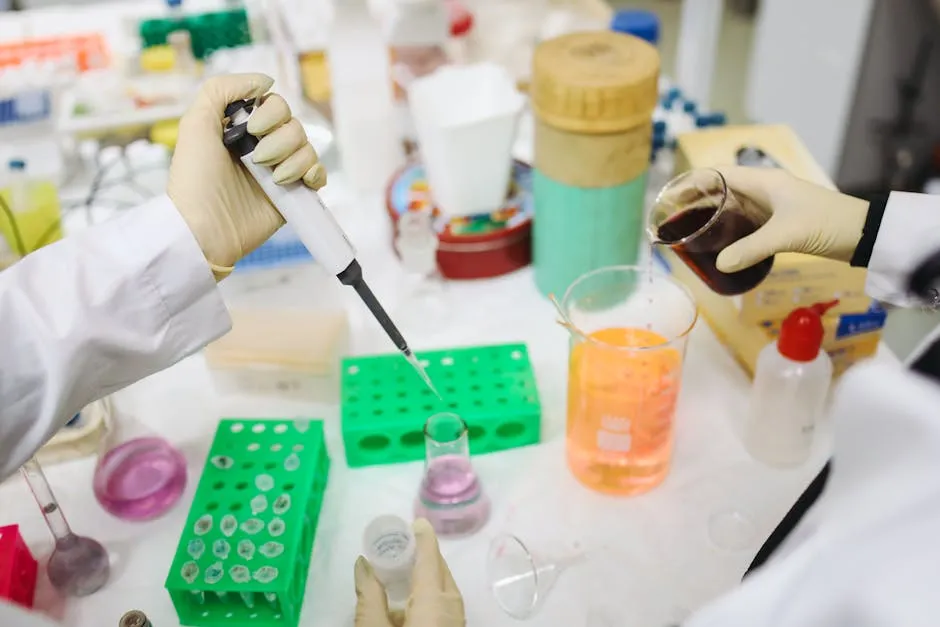
Consequences of Ignoring Lurking Variables
Ignoring lurking variables can lead to a host of problems. First and foremost, it can falsely indicate strong relationships between variables. Imagine a study that finds a correlation between hours spent studying and test scores. If researchers overlook the lurking variable of prior knowledge, they might mistakenly conclude that studying is the sole factor affecting performance. In reality, students with stronger backgrounds may score higher, skewing results. If you’re looking to boost academic performance, consider investing in a Graphing Calculator to help visualize complex equations!
Next, lurking variables can hide true relationships. Consider a study linking physical activity to improved health. If researchers neglect to account for age as a lurking variable, they might miss the fact that younger individuals tend to be more active and healthier. Thus, the apparent health benefits of physical activity may be misrepresented.
Phantom correlations are another consequence of ignoring lurking variables. Take the infamous example of ice cream sales and shark attacks. Both increase during summer months, leading to a spurious correlation. The lurking variable here is temperature. Warmer weather drives both ice cream sales and more people at the beach, inadvertently increasing shark attacks. Without recognizing temperature’s influence, researchers might conclude that ice cream consumption somehow attracts sharks. Yikes!
Real-world implications of these consequences are significant. For instance, a public health study might mistakenly recommend increased exercise to combat obesity while overlooking dietary factors. This could lead to ineffective health policies and misguided public behavior. Similarly, businesses might misinterpret correlations in sales data, causing flawed marketing strategies based on misunderstood relationships. If you’re in the business sector, consider grabbing a copy of Data Science for Business to navigate the data-driven landscape!
In conclusion, ignoring lurking variables can distort analyses, obscure genuine relationships, and create misleading correlations. Researchers must be vigilant in identifying these hidden factors to ensure their conclusions are accurate and actionable. By doing so, they can avoid the pitfalls of faulty interpretations and ultimately improve decision-making processes in various fields.
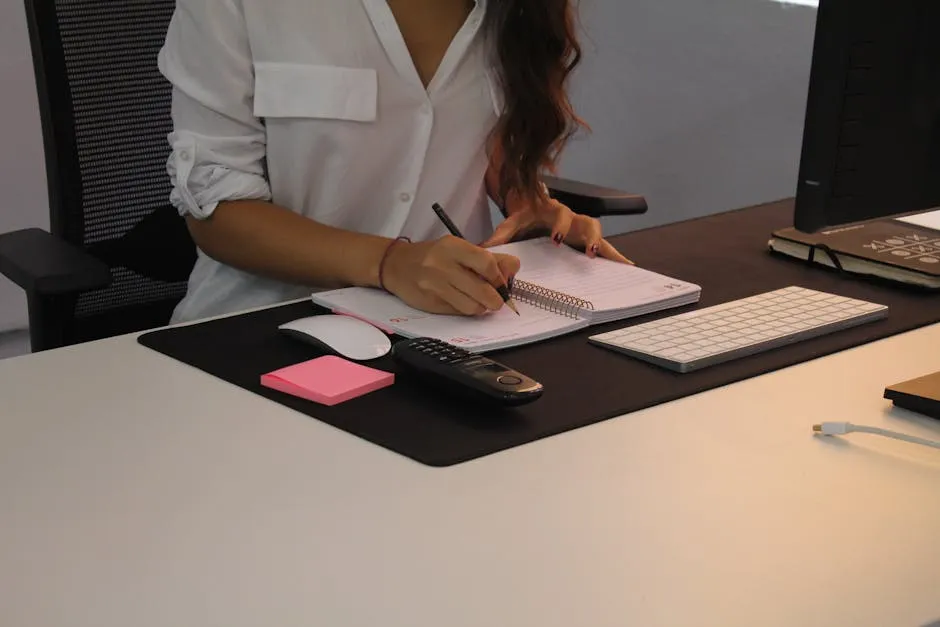
Strategies for Identifying Lurking Variables
Identifying lurking variables can feel like searching for a needle in a haystack. But fear not! Several strategies can help researchers uncover these hidden influences. Let’s break them down.
First up, domain expertise and literature reviews are invaluable tools. Researchers should lean on their knowledge of the field. By understanding the specific context, they can anticipate potential lurking variables that might affect their analysis. Conducting thorough literature reviews helps as well. Previous studies often highlight overlooked variables, serving as a valuable guide. After all, if someone else has tripped over the same rock, you can learn to walk around it!
Next, statistical methods come into play. Regression analysis is a powerful technique for identifying lurking variables. By examining relationships between variables, researchers can detect patterns that reveal hidden influences. For instance, plotting residuals can unveil non-linear trends, indicating lurking variables at work. A residual plot that shows a pattern suggests that something is amiss. It’s like finding out your favorite song has a hidden verse—there’s more to the story!
Randomization in experimental design is another critical strategy. By randomly assigning subjects to treatment groups, researchers can minimize the impact of lurking variables. This technique ensures that lurking variables are evenly distributed, making it easier to isolate the effects of the independent variable. Think of it as distributing candy at a party; if everyone gets the same amount, you can confidently say the fun times were due to the games, not the sweets!
To illustrate these strategies, let’s look at some studies that successfully identified lurking variables. In one health study, researchers initially found no correlation between activity level and bone density. By considering weight as a lurking variable, they revised their analysis. Including weight revealed the true relationship between activity levels and bone density. Voilà! A hidden connection was uncovered. If you’re looking to dive into data science, Data Science from Scratch is a great read!
Another example comes from education research. Analysts found a positive correlation between education level and income. However, they later realized work experience was a lurking variable skewing their results. By adjusting for work experience, they clarified the true impact of education on income. Who knew a little adjustment could lead to such big revelations?
By employing these strategies, researchers can uncover lurking variables that might otherwise go unnoticed. With a keen eye and the right tools, they can ensure their analyses are robust and credible. Remember, lurking variables are like the spice in a recipe—necessary to bring out the best flavors in the data! And if you’re looking to spice up your data analysis, consider getting Python for Data Analysis!
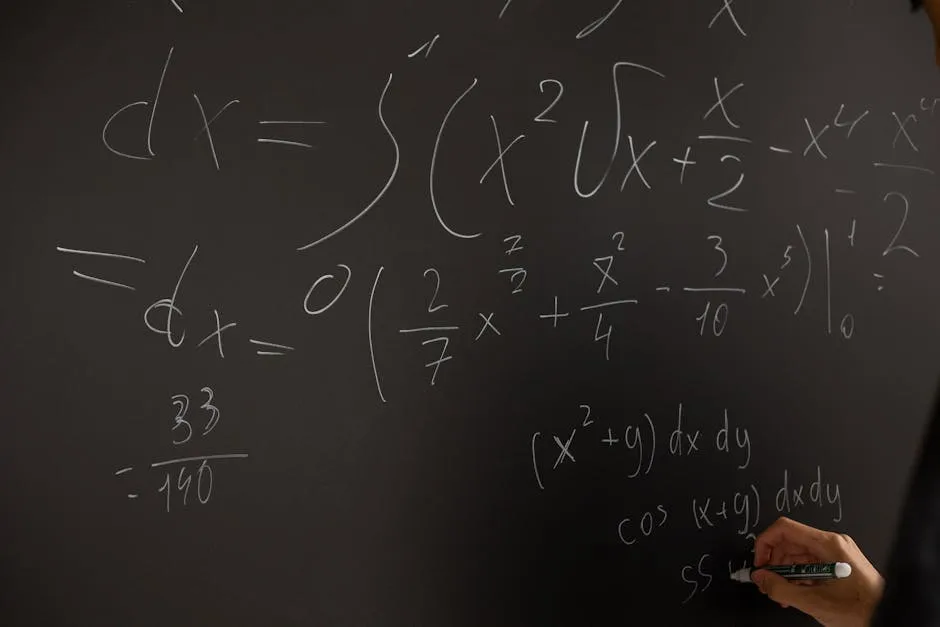
Best Practices for Controlling Lurking Variables
Controlling lurking variables is like putting on a seatbelt; it’s a small action that can save you from a world of trouble. Here are some best practices to keep lurking variables in check during research.
First, include known lurking variables in statistical models. This is key to separating their effects from the main variables of interest. By doing so, researchers can account for their influence, leading to more accurate results. Think of it like having a co-pilot who helps navigate the twists and turns of your research journey.
Next, holding constant known variables is crucial. This means standardizing conditions across observations. For instance, in an exercise study, researchers could maintain similar dietary habits among participants. By controlling for diet, they can isolate the effects of exercise on health outcomes. It’s like ensuring everyone at a dinner party eats the same meal so you can truly appreciate the dessert!
Randomized controlled trials (RCTs) are another powerful tool in the researcher’s arsenal. RCTs randomly assign subjects to different groups, minimizing the influence of lurking variables. This method enhances the validity of results, allowing researchers to confidently attribute effects to the treatment being studied. It’s akin to flipping a coin to decide who gets the last piece of pizza—everyone has an equal chance, making the outcome fair!
Careful study design is crucial for addressing lurking variables effectively. This includes thorough literature reviews and expert consultations to identify potential confounders. When researchers take the time to plan, they can anticipate lurking variables and design their studies accordingly. It’s like preparing a detailed map before heading on a road trip—you’re less likely to get lost!
Lastly, replication and validation of studies can help confirm findings across different contexts. Encouraging others to replicate research helps bolster the credibility of results. If multiple studies yield similar outcomes, researchers can feel more confident that lurking variables have been adequately controlled. It’s the scientific equivalent of a group hug—everyone feels better together! For those who want a solid foundation in statistics, consider getting Introduction to the Practice of Statistics.
In summary, controlling lurking variables is essential for robust research. By incorporating known variables in models, holding constants, and employing RCTs, researchers can improve the reliability of their findings. With careful planning and adherence to best practices, they can navigate the complexities of data analysis and emerge with clear, accurate insights. Remember, a little diligence goes a long way in avoiding the lurking shadows of confusion!

Emerging Methods and Tools for Managing Lurking Variables
In the world of statistics, managing lurking variables has become a hot topic. The good news? We’re not stuck in the dark ages! Recent advancements in statistical analysis, particularly machine learning and causal inference frameworks, have paved the way for more precise research methodologies. Think of these tools as the superheroes of data analysis, swooping in to save researchers from the clutches of lurking variables!
Machine learning is making waves in identifying patterns and correlations that traditional methods might miss. By utilizing algorithms, researchers can sift through vast datasets to detect lurking variables that could otherwise go unnoticed. Imagine having a data detective on your team, tirelessly working to reveal hidden insights. That’s machine learning for you! It can help uncover relationships and dependencies that even seasoned analysts might overlook. To get started with machine learning, check out The Art of Data Science!
Causal inference frameworks are another exciting development. These frameworks allow researchers to model causal relationships more accurately, helping to isolate the effects of independent variables from those pesky lurking variables. With these frameworks, researchers can not only understand whether a relationship exists but also how strong that relationship is. It’s like putting on a pair of glasses that brings everything into sharp focus!
Now, let’s talk about technology and software. New tools are emerging every day, designed specifically to assist researchers in identifying and managing lurking variables. Statistical software packages like R and Python libraries provide powerful functions for regression analysis, making it easier to visualize and control for lurking variables. With these tools, researchers can engage in complex analyses without the fear of getting lost in the numbers. If you’re looking to master R, consider getting The R Book!
Open data and reproducibility are also crucial in the fight against lurking variables. When researchers share their data openly, they allow others to validate findings and check for lurking variables that may have been overlooked. This transparency fosters collaboration and encourages a culture of accountability in research. In a way, it’s like inviting others to your party and asking them to keep an eye out for any uninvited guests trying to crash!
Moreover, the push for reproducibility ensures that studies can be replicated by others. When researchers publish their methods and data, it opens the door for scrutiny and validation. If lurking variables are present, they can be identified in subsequent studies. This process enhances the credibility of research findings and helps build a solid foundation for future studies.
In conclusion, the advancements in statistical analysis, machine learning, and causal inference frameworks are revolutionizing how researchers manage lurking variables. The emergence of new technologies and software empowers researchers to tackle these hidden factors head-on. Emphasizing open data and reproducibility not only promotes transparency but also strengthens the overall integrity of research. With these tools and methods at their disposal, researchers can confidently navigate the murky waters of lurking variables, leading to clearer insights and more reliable conclusions.
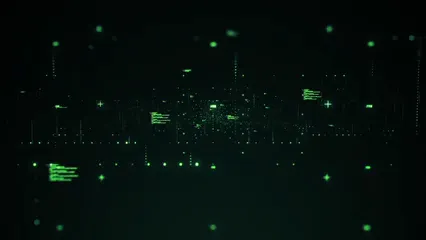
Ethical Considerations in Research
Ignoring lurking variables in research poses significant ethical implications. Researchers have a profound responsibility to design studies that account for these hidden influences. Failing to do so can mislead stakeholders, resulting in misguided policies or ineffective interventions.
When lurking variables are overlooked, they can distort the true relationships between studied variables. This distortion can create false narratives that potentially harm individuals or communities. For instance, if a health study correlates a specific diet with lower disease rates without considering exercise as a lurking variable, the findings could mislead the public about dietary choices. This misrepresentation could lead to poor health decisions, ultimately endangering lives. To ensure you’re well-informed, consider reading Statistical Methods for the Social Sciences!
Researchers must prioritize transparency in their methods. Disclosing potential lurking variables and the steps taken to address them fosters trust. It enables peers and the public to scrutinize the validity of the findings. In a world where information travels fast, it’s crucial to ensure that research is reliable and credible. Misleading conclusions can have far-reaching consequences, affecting everything from personal health choices to government funding.
Interdisciplinary collaboration is also vital in addressing lurking variables. Engaging experts from various fields can provide insights that a single discipline might overlook. For example, sociologists might identify societal influences that affect health outcomes, while statisticians can contribute methods to control for these variables. By working together, researchers can create a more comprehensive understanding of the factors influencing their studies.
Moreover, ethical research practices extend to considering the implications of findings. Researchers must ponder how their conclusions might be interpreted and used. This responsibility includes understanding the potential for misuse or misinterpretation of results. When researchers account for lurking variables, they not only enhance their study’s accuracy but also uphold their ethical obligations to society.
In summary, the ethical implications of ignoring lurking variables are profound. Researchers must recognize their responsibility to design rigorous studies that incorporate potential lurking influences. Transparency and interdisciplinary collaboration are essential in this pursuit. By acknowledging and addressing lurking variables, researchers can improve the credibility of their findings, ultimately leading to better decision-making and outcomes for all.
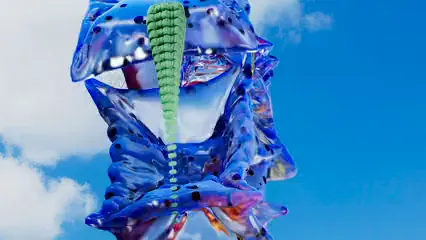
Conclusion
Understanding lurking variables is paramount for researchers. These hidden factors can significantly distort statistical analyses, leading to incorrect conclusions. Throughout this article, we’ve highlighted the importance of identifying and managing lurking variables.
Ignoring these variables can result in misleading correlations. For example, studies linking ice cream sales to shark attacks fail to account for temperature, a lurking variable that drives both. By recognizing such influences, researchers can avoid falling into the trap of erroneous interpretations.
Managing lurking variables enhances the credibility of research findings. By employing strategies like inclusion in statistical models and randomization, researchers can mitigate the effects of these hidden factors. This proactive approach ensures that the relationships identified in research are genuine and reflective of reality.
Moreover, as research continues to evolve, so too should our methods for addressing lurking variables. The field is ripe for innovation, with advancements in statistical techniques and interdisciplinary collaboration paving the way for more robust analyses. Researchers should remain curious and committed to exploring new methodologies for identifying and controlling lurking variables. If you’re looking for a comprehensive introduction to statistical learning, you might want to check out An Introduction to Statistical Learning.
In conclusion, understanding and managing lurking variables is crucial for accurate statistical analyses. Researchers must strive to enhance their studies by recognizing hidden influences that could skew results. By doing so, they contribute to the reliability and credibility of their findings, ultimately benefiting society at large. So, let’s keep our eyes peeled for those lurking variables and ensure our research stands up to scrutiny!

FAQs
What is the difference between a lurking variable and a confounding variable?
Lurking variables are unknown factors not included in the analysis but can affect relationships. Confounding variables are recognized and included in the study design. For example, in a study on exercise and heart health, age might be a confounding variable if accounted for, while diet could be a lurking variable if ignored.
How can I identify lurking variables in my research?
Start with a thorough literature review in your field. This will help you recognize potential lurking variables. Use statistical methods like regression analysis to examine relationships. Look at residual plots; patterns may indicate hidden influences. Additionally, consult experts who can provide insights into your study context.
What can happen if I ignore lurking variables in my analysis?
Ignoring lurking variables can lead to biased results and misleading conclusions. It may falsely indicate strong relationships between variables or hide real effects. For example, you might conclude that exercise alone improves health, overlooking diet as a lurking variable that plays a substantial role.
Are there software tools available to help with lurking variable analysis?
Yes! Popular statistical software like R, SPSS, and Minitab can assist in analyzing lurking variables. These tools provide functionalities for regression analysis and visualization, helping researchers identify hidden influences and improve the accuracy of their findings.
Can lurking variables be beneficial in any way?
While lurking variables are typically seen as a challenge, understanding them can provide valuable insights. For instance, recognizing that weather influences both ice cream sales and shark attacks can lead to better public safety measures during warm months. Understanding the context of lurking variables can help researchers craft more effective interventions and policies.
Please let us know what you think about our content by leaving a comment down below!
Thank you for reading till here 🙂
For more insights on effective data analysis, check out this resource on tips for effective data analysis in economics and statistics.
All images from Pexels