Introduction
Parametric statistics is the backbone of many survey data analyses. Think of it as the VIP section of your favorite club: exclusive, with a strict guest list! To gain entry, your data must follow some essential assumptions: normality, homogeneity of variance, and independence of observations. If your data doesn’t meet these criteria, it’s like showing up in flip-flops to a black-tie gala; you might just get turned away!
Normality means your data should resemble a bell curve. Homogeneity of variance indicates that different groups should have similar variances. Then there’s independence—each observation should stand solo, like a lone dancer in the spotlight, unaffected by the others.
When these assumptions are violated, researchers risk misinterpretation of their findings. The results can be skewed, leading to incorrect conclusions. Type I errors (false positives) and Type II errors (false negatives) become your unwelcome plus-ones at the party. Thus, understanding these assumptions is crucial for valid results in survey data analysis.
In summary, whether you transform your data, rely on nonparametric tests, bootstrap your way to better estimates, or embrace the flexibility of Bayesian methods, you have a plethora of strategies at your disposal. Each method has its pros and cons, and the key lies in choosing the right tool for the job. So roll up your sleeves and tackle those challenges head-on!

Conclusion
Overcoming challenges related to parametric statistics assumptions in survey data analysis is crucial for generating reliable results. We’ve explored the essential assumptions: normality, homogeneity of variance, and independence of observations. Ignoring these assumptions can lead to statistical mischief—think of it as trying to bake a cake without following the recipe. Sure, you might get something edible, but it’s unlikely to be a masterpiece.
Addressing these challenges ensures that your findings are valid. We discussed the dire consequences of assumption violations, including Type I and Type II errors. Trust me, nobody wants to be the statistician who confidently declares a significant result, only to find out it was a false alarm. By employing alternative methods, such as nonparametric tests or data transformations, researchers can still glean meaningful insights from their data.
I encourage researchers and analysts to be proactive. Regularly evaluate your data for assumption adherence. If it doesn’t meet the criteria, don’t panic! Consider alternative methods like bootstrapping or Bayesian statistics. These approaches can provide robust analyses without the heavy reliance on assumptions.
In the world of data analysis, knowledge is power. Equip yourself with the tools and strategies we’ve discussed. By doing so, you can navigate the statistical landscape confidently. Remember, the goal is to derive accurate insights that can drive informed decisions. So, roll up your sleeves, embrace the challenge, and let your data shine! With robust analysis, you hold the potential to unlock valuable outcomes that could transform your research or business strategies.
FAQs
What are the main assumptions of parametric statistics?
The primary assumptions of parametric statistics include normality (data should follow a normal distribution), homogeneity of variance (equal variances across groups), and independence of observations (individual data points should not influence each other). Meeting these assumptions is vital for the validity of statistical tests.
What should I do if my data violates these assumptions?
If your data violates parametric assumptions, consider several strategies. First, data transformations (like logarithmic or Box-Cox) can help stabilize variance and normalize distributions. Alternatively, switch to nonparametric tests, such as the Mann-Whitney U test or Kruskal-Wallis test, which don’t rely on strict distribution assumptions. Bootstrapping can also be an effective approach, allowing you to estimate statistics through resampling.
How can I ensure the quality of my survey data?
To ensure high-quality survey data, invest time in designing your survey. Use clear, concise questions aligned with your research goals. Pilot your survey with a small group to identify potential issues. Additionally, focus on data cleaning—address missing values, outliers, and duplicates. Employ random sampling techniques to minimize bias and ensure your sample accurately reflects the target population.
Are there specific statistical tests I should use for non-normal data?
Yes! For non-normal data, nonparametric tests are often the best choice. The Mann-Whitney U test is suitable for comparing two independent samples, while the Kruskal-Wallis test is ideal for comparing more than two groups. These tests maintain robustness even when the assumptions of parametric tests are violated.
What is the impact of sample size on parametric tests?
Sample size plays a crucial role in parametric tests. Larger sample sizes tend to better approximate normality due to the Central Limit Theorem. This means that even if the underlying population distribution is not normal, the sampling distribution of the mean will tend to be normal with a sufficiently large sample size. Therefore, increasing your sample size can improve the reliability of your statistical conclusions.
Please let us know what you think about our content by leaving a comment down below!
Thank you for reading till here 🙂
For more insights on effective methods for analyzing data, check out these tips for effective data analysis in economics and statistics.
To understand the flow of statistical tests better, refer to this flow chart for statistical tests.
All images from Pexels
Pros of data transformation include improved adherence to assumptions and increased statistical power. But be cautious—over-transformation can lead to loss of interpretability. Always weigh the benefits against potential pitfalls, and remember, not every outfit works for every event.
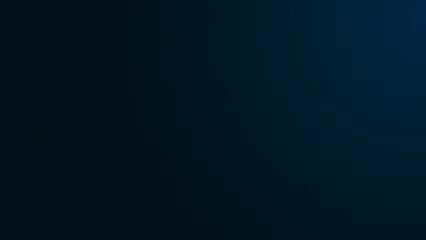
Using Nonparametric Tests
Sometimes, the best way to navigate the tricky terrain of assumption violations is to opt for nonparametric tests. These tests are the rebels of the statistical world. They don’t rely on the assumption of normality, making them preferable in various situations. If you find your data skewed or ordinal, nonparametric tests are your best friends.
Take the Mann-Whitney U test, for instance. It compares two independent groups without the need for normal distribution. It’s like a fair contest where everyone gets a chance to shine, regardless of their starting point. Then there’s the Kruskal-Wallis test, which is the go-to for comparing more than two groups. It’s nonparametric ANOVA but without the frills! If you want to explore more about data science, consider Data Science from Scratch: First Principles with Python by Joel Grus. It’s an excellent guide for getting started in the field!
Nonparametric tests can be less powerful than their parametric counterparts, especially with large samples. However, their flexibility can save you from the woes of assumption violations. They provide a reliable alternative when you need to draw conclusions without the constraints of strict assumptions.
Bootstrapping and Resampling Methods
Enter bootstrapping—a magical resampling technique! Bootstrapping allows you to create multiple samples from your data by sampling with replacement. Think of it as creating your own mini-universe from a single data set. It’s particularly useful when dealing with small sample sizes or suspected assumption violations.
By generating numerous resamples, you can estimate the distribution of a statistic without relying on strict assumptions. This gives you confidence intervals that reflect the uncertainty in your estimates. It’s like being able to predict the weather with a bit more flair and accuracy! If you’re looking for a comprehensive resource on Bayesian methods, check out Bayesian Data Analysis by Andrew Gelman. This book will deepen your understanding of Bayesian techniques!
Resampling methods help to overcome violations by providing a robust framework for inference. They let you focus on the data at hand instead of fretting over whether your assumptions are perfectly met. Just remember, while bootstrapping can be powerful, it’s essential to interpret results with care. The universe you create should still reflect the reality of your original data!
Bayesian Methods
Bayesian statistics are like the wise old sage of the statistical realm. They incorporate prior knowledge and update it with observed data, allowing for a flexible approach to analysis. This method sidesteps many of the strict assumptions required by traditional parametric tests, making it a valuable ally when assumptions are in jeopardy.
One of the key advantages of Bayesian methods is their ability to quantify uncertainty. By using prior distributions, you can incorporate existing knowledge into your analysis, which can be particularly helpful when working with small samples or when data is sparse. Instead of just a pass/fail judgment, you get a richer understanding of the probabilities involved.
Bayesian methods also allow for updating beliefs as new data emerges. As you gather more evidence, you can refine your estimates, much like adjusting your strategy in a game based on the moves of your opponents. This adaptability makes Bayesian statistics an appealing option for overcoming assumption violations and adapting to the ever-changing landscape of data.
In summary, whether you transform your data, rely on nonparametric tests, bootstrap your way to better estimates, or embrace the flexibility of Bayesian methods, you have a plethora of strategies at your disposal. Each method has its pros and cons, and the key lies in choosing the right tool for the job. So roll up your sleeves and tackle those challenges head-on!

Conclusion
Overcoming challenges related to parametric statistics assumptions in survey data analysis is crucial for generating reliable results. We’ve explored the essential assumptions: normality, homogeneity of variance, and independence of observations. Ignoring these assumptions can lead to statistical mischief—think of it as trying to bake a cake without following the recipe. Sure, you might get something edible, but it’s unlikely to be a masterpiece.
Addressing these challenges ensures that your findings are valid. We discussed the dire consequences of assumption violations, including Type I and Type II errors. Trust me, nobody wants to be the statistician who confidently declares a significant result, only to find out it was a false alarm. By employing alternative methods, such as nonparametric tests or data transformations, researchers can still glean meaningful insights from their data.
I encourage researchers and analysts to be proactive. Regularly evaluate your data for assumption adherence. If it doesn’t meet the criteria, don’t panic! Consider alternative methods like bootstrapping or Bayesian statistics. These approaches can provide robust analyses without the heavy reliance on assumptions.
In the world of data analysis, knowledge is power. Equip yourself with the tools and strategies we’ve discussed. By doing so, you can navigate the statistical landscape confidently. Remember, the goal is to derive accurate insights that can drive informed decisions. So, roll up your sleeves, embrace the challenge, and let your data shine! With robust analysis, you hold the potential to unlock valuable outcomes that could transform your research or business strategies.
FAQs
Please let us know what you think about our content by leaving a comment down below!
Thank you for reading till here 🙂
For more insights on effective methods for analyzing data, check out these tips for effective data analysis in economics and statistics.
To understand the flow of statistical tests better, refer to this flow chart for statistical tests.
All images from Pexels
For instance, if normality is not met, your t-tests and ANOVAs may produce misleading results. You could believe you’ve found a significant difference when, in fact, it’s just statistical noise. Picture a marketer concluding their new strategy is a smashing success based on flawed data. Oops!
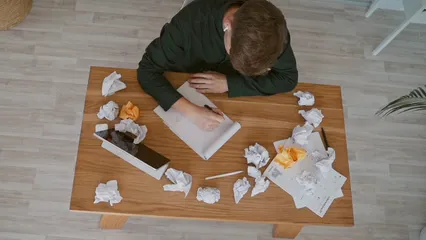
Case studies abound. In one notable example, researchers analyzed survey results on health behaviors. They assumed normal distributions, but the data was skewed due to outliers. Their findings suggested a drastic increase in health awareness, but in reality, the data reflected a few highly engaged respondents. This misinterpretation could lead to wasted resources and misguided public health strategies.
In another instance, a social scientist studied the impact of education on income. They failed to check the homogeneity of variance assumption and, as a result, reported that education had a significant effect on income. However, the analysis masked the fact that variances were vastly different across education levels, leading to an invalid conclusion.
These stories illustrate the importance of rigorously checking assumptions before diving into analysis. Ignoring them can lead to conclusions that not only misinform stakeholders but can also have real-world implications ranging from misguided business strategies to ineffective public policies. So, pay attention to those assumptions; they might just save your research from a rocky fate!
Strategies for Overcoming Challenges
Transforming Data
When your data doesn’t fit the mold, transformations can save the day! Techniques like logarithmic and Box-Cox transformations can help you whip that unruly data into shape. Logarithmic transformation takes the log of your data values, which can be especially useful for right-skewed distributions. It’s a bit like turning a pumpkin into a carriage—quite the transformation!
Box-Cox transformations go a step further. They search for the best transformation to stabilize variance and make the data more normal-like. It’s like finding the perfect outfit that fits just right for a fancy occasion. However, tread carefully! Transformations can change the scale of your data, which can lead to interpretive headaches. While they can help meet assumptions, they might also complicate the communication of your results. If you’re curious about the practical applications of data visualization, I recommend Data Visualization: A Practical Introduction by Kieran Healy. This book is a fantastic resource for honing your data presentation skills!
Pros of data transformation include improved adherence to assumptions and increased statistical power. But be cautious—over-transformation can lead to loss of interpretability. Always weigh the benefits against potential pitfalls, and remember, not every outfit works for every event.
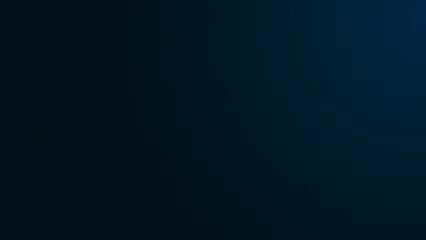
Using Nonparametric Tests
Sometimes, the best way to navigate the tricky terrain of assumption violations is to opt for nonparametric tests. These tests are the rebels of the statistical world. They don’t rely on the assumption of normality, making them preferable in various situations. If you find your data skewed or ordinal, nonparametric tests are your best friends.
Take the Mann-Whitney U test, for instance. It compares two independent groups without the need for normal distribution. It’s like a fair contest where everyone gets a chance to shine, regardless of their starting point. Then there’s the Kruskal-Wallis test, which is the go-to for comparing more than two groups. It’s nonparametric ANOVA but without the frills! If you want to explore more about data science, consider Data Science from Scratch: First Principles with Python by Joel Grus. It’s an excellent guide for getting started in the field!
Nonparametric tests can be less powerful than their parametric counterparts, especially with large samples. However, their flexibility can save you from the woes of assumption violations. They provide a reliable alternative when you need to draw conclusions without the constraints of strict assumptions.
Bootstrapping and Resampling Methods
Enter bootstrapping—a magical resampling technique! Bootstrapping allows you to create multiple samples from your data by sampling with replacement. Think of it as creating your own mini-universe from a single data set. It’s particularly useful when dealing with small sample sizes or suspected assumption violations.
By generating numerous resamples, you can estimate the distribution of a statistic without relying on strict assumptions. This gives you confidence intervals that reflect the uncertainty in your estimates. It’s like being able to predict the weather with a bit more flair and accuracy! If you’re looking for a comprehensive resource on Bayesian methods, check out Bayesian Data Analysis by Andrew Gelman. This book will deepen your understanding of Bayesian techniques!
Resampling methods help to overcome violations by providing a robust framework for inference. They let you focus on the data at hand instead of fretting over whether your assumptions are perfectly met. Just remember, while bootstrapping can be powerful, it’s essential to interpret results with care. The universe you create should still reflect the reality of your original data!
Bayesian Methods
Bayesian statistics are like the wise old sage of the statistical realm. They incorporate prior knowledge and update it with observed data, allowing for a flexible approach to analysis. This method sidesteps many of the strict assumptions required by traditional parametric tests, making it a valuable ally when assumptions are in jeopardy.
One of the key advantages of Bayesian methods is their ability to quantify uncertainty. By using prior distributions, you can incorporate existing knowledge into your analysis, which can be particularly helpful when working with small samples or when data is sparse. Instead of just a pass/fail judgment, you get a richer understanding of the probabilities involved.
Bayesian methods also allow for updating beliefs as new data emerges. As you gather more evidence, you can refine your estimates, much like adjusting your strategy in a game based on the moves of your opponents. This adaptability makes Bayesian statistics an appealing option for overcoming assumption violations and adapting to the ever-changing landscape of data.
In summary, whether you transform your data, rely on nonparametric tests, bootstrap your way to better estimates, or embrace the flexibility of Bayesian methods, you have a plethora of strategies at your disposal. Each method has its pros and cons, and the key lies in choosing the right tool for the job. So roll up your sleeves and tackle those challenges head-on!

Conclusion
Overcoming challenges related to parametric statistics assumptions in survey data analysis is crucial for generating reliable results. We’ve explored the essential assumptions: normality, homogeneity of variance, and independence of observations. Ignoring these assumptions can lead to statistical mischief—think of it as trying to bake a cake without following the recipe. Sure, you might get something edible, but it’s unlikely to be a masterpiece.
Addressing these challenges ensures that your findings are valid. We discussed the dire consequences of assumption violations, including Type I and Type II errors. Trust me, nobody wants to be the statistician who confidently declares a significant result, only to find out it was a false alarm. By employing alternative methods, such as nonparametric tests or data transformations, researchers can still glean meaningful insights from their data.
I encourage researchers and analysts to be proactive. Regularly evaluate your data for assumption adherence. If it doesn’t meet the criteria, don’t panic! Consider alternative methods like bootstrapping or Bayesian statistics. These approaches can provide robust analyses without the heavy reliance on assumptions.
In the world of data analysis, knowledge is power. Equip yourself with the tools and strategies we’ve discussed. By doing so, you can navigate the statistical landscape confidently. Remember, the goal is to derive accurate insights that can drive informed decisions. So, roll up your sleeves, embrace the challenge, and let your data shine! With robust analysis, you hold the potential to unlock valuable outcomes that could transform your research or business strategies.
FAQs
Please let us know what you think about our content by leaving a comment down below!
Thank you for reading till here 🙂
For more insights on effective methods for analyzing data, check out these tips for effective data analysis in economics and statistics.
To understand the flow of statistical tests better, refer to this flow chart for statistical tests.
All images from Pexels
Next, focus on data cleaning. Address missing values, outliers, and inconsistencies. Validate responses to ensure logical coherence. This step is akin to putting on a fresh coat of paint—your data deserves to look its best! Random sampling techniques can also help minimize bias, ensuring your sample represents the broader population. This way, your insights won’t just be pretty on paper; they’ll be solid gold in their relevance!
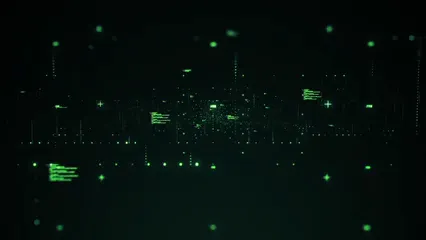
Missing Data
Missing data is like that one piece of a puzzle that just won’t show up. It can significantly impact your analysis, skewing results and leading to misguided conclusions. Understanding the types of missing data can help you decide on a strategic approach. There are three primary types:
1. Missing Completely at Random (MCAR): The missing values have no systematic relationship with the other data. Think of it as a random act of nature—unpredictable and often benign.
2. Missing at Random (MAR): The missingness is related to observed data but not the missing data itself. It’s like a party where some guests didn’t RSVP because they were busy but still left behind an empty seat.
3. Missing Not at Random (MNAR): Here, the missing data is related to the unobserved data. For example, people with lower incomes might skip a question about their salary. This one can be tricky!
To handle missing data effectively, consider different imputation methods. Mean imputation can work wonders for MCAR, while regression imputation or multiple imputation might be better for MAR. Always conduct a sensitivity analysis to assess the robustness of your results and understand the potential impact of missing data. This proactive approach will keep your analysis from derailing!
Consequences of Violating Assumptions
When assumptions of parametric statistics are violated, the consequences can be dire. Imagine preparing for a big test without studying; you’re setting yourself up for failure! Violations can lead to inaccurate estimates, unreliable confidence intervals, and misguided conclusions.
For instance, if normality is not met, your t-tests and ANOVAs may produce misleading results. You could believe you’ve found a significant difference when, in fact, it’s just statistical noise. Picture a marketer concluding their new strategy is a smashing success based on flawed data. Oops!
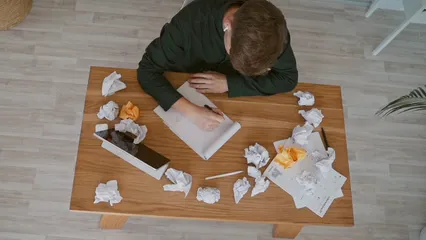
Case studies abound. In one notable example, researchers analyzed survey results on health behaviors. They assumed normal distributions, but the data was skewed due to outliers. Their findings suggested a drastic increase in health awareness, but in reality, the data reflected a few highly engaged respondents. This misinterpretation could lead to wasted resources and misguided public health strategies.
In another instance, a social scientist studied the impact of education on income. They failed to check the homogeneity of variance assumption and, as a result, reported that education had a significant effect on income. However, the analysis masked the fact that variances were vastly different across education levels, leading to an invalid conclusion.
These stories illustrate the importance of rigorously checking assumptions before diving into analysis. Ignoring them can lead to conclusions that not only misinform stakeholders but can also have real-world implications ranging from misguided business strategies to ineffective public policies. So, pay attention to those assumptions; they might just save your research from a rocky fate!
Strategies for Overcoming Challenges
Transforming Data
When your data doesn’t fit the mold, transformations can save the day! Techniques like logarithmic and Box-Cox transformations can help you whip that unruly data into shape. Logarithmic transformation takes the log of your data values, which can be especially useful for right-skewed distributions. It’s a bit like turning a pumpkin into a carriage—quite the transformation!
Box-Cox transformations go a step further. They search for the best transformation to stabilize variance and make the data more normal-like. It’s like finding the perfect outfit that fits just right for a fancy occasion. However, tread carefully! Transformations can change the scale of your data, which can lead to interpretive headaches. While they can help meet assumptions, they might also complicate the communication of your results. If you’re curious about the practical applications of data visualization, I recommend Data Visualization: A Practical Introduction by Kieran Healy. This book is a fantastic resource for honing your data presentation skills!
Pros of data transformation include improved adherence to assumptions and increased statistical power. But be cautious—over-transformation can lead to loss of interpretability. Always weigh the benefits against potential pitfalls, and remember, not every outfit works for every event.
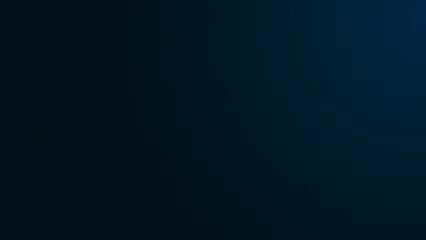
Using Nonparametric Tests
Sometimes, the best way to navigate the tricky terrain of assumption violations is to opt for nonparametric tests. These tests are the rebels of the statistical world. They don’t rely on the assumption of normality, making them preferable in various situations. If you find your data skewed or ordinal, nonparametric tests are your best friends.
Take the Mann-Whitney U test, for instance. It compares two independent groups without the need for normal distribution. It’s like a fair contest where everyone gets a chance to shine, regardless of their starting point. Then there’s the Kruskal-Wallis test, which is the go-to for comparing more than two groups. It’s nonparametric ANOVA but without the frills! If you want to explore more about data science, consider Data Science from Scratch: First Principles with Python by Joel Grus. It’s an excellent guide for getting started in the field!
Nonparametric tests can be less powerful than their parametric counterparts, especially with large samples. However, their flexibility can save you from the woes of assumption violations. They provide a reliable alternative when you need to draw conclusions without the constraints of strict assumptions.
Bootstrapping and Resampling Methods
Enter bootstrapping—a magical resampling technique! Bootstrapping allows you to create multiple samples from your data by sampling with replacement. Think of it as creating your own mini-universe from a single data set. It’s particularly useful when dealing with small sample sizes or suspected assumption violations.
By generating numerous resamples, you can estimate the distribution of a statistic without relying on strict assumptions. This gives you confidence intervals that reflect the uncertainty in your estimates. It’s like being able to predict the weather with a bit more flair and accuracy! If you’re looking for a comprehensive resource on Bayesian methods, check out Bayesian Data Analysis by Andrew Gelman. This book will deepen your understanding of Bayesian techniques!
Resampling methods help to overcome violations by providing a robust framework for inference. They let you focus on the data at hand instead of fretting over whether your assumptions are perfectly met. Just remember, while bootstrapping can be powerful, it’s essential to interpret results with care. The universe you create should still reflect the reality of your original data!
Bayesian Methods
Bayesian statistics are like the wise old sage of the statistical realm. They incorporate prior knowledge and update it with observed data, allowing for a flexible approach to analysis. This method sidesteps many of the strict assumptions required by traditional parametric tests, making it a valuable ally when assumptions are in jeopardy.
One of the key advantages of Bayesian methods is their ability to quantify uncertainty. By using prior distributions, you can incorporate existing knowledge into your analysis, which can be particularly helpful when working with small samples or when data is sparse. Instead of just a pass/fail judgment, you get a richer understanding of the probabilities involved.
Bayesian methods also allow for updating beliefs as new data emerges. As you gather more evidence, you can refine your estimates, much like adjusting your strategy in a game based on the moves of your opponents. This adaptability makes Bayesian statistics an appealing option for overcoming assumption violations and adapting to the ever-changing landscape of data.
In summary, whether you transform your data, rely on nonparametric tests, bootstrap your way to better estimates, or embrace the flexibility of Bayesian methods, you have a plethora of strategies at your disposal. Each method has its pros and cons, and the key lies in choosing the right tool for the job. So roll up your sleeves and tackle those challenges head-on!

Conclusion
Overcoming challenges related to parametric statistics assumptions in survey data analysis is crucial for generating reliable results. We’ve explored the essential assumptions: normality, homogeneity of variance, and independence of observations. Ignoring these assumptions can lead to statistical mischief—think of it as trying to bake a cake without following the recipe. Sure, you might get something edible, but it’s unlikely to be a masterpiece.
Addressing these challenges ensures that your findings are valid. We discussed the dire consequences of assumption violations, including Type I and Type II errors. Trust me, nobody wants to be the statistician who confidently declares a significant result, only to find out it was a false alarm. By employing alternative methods, such as nonparametric tests or data transformations, researchers can still glean meaningful insights from their data.
I encourage researchers and analysts to be proactive. Regularly evaluate your data for assumption adherence. If it doesn’t meet the criteria, don’t panic! Consider alternative methods like bootstrapping or Bayesian statistics. These approaches can provide robust analyses without the heavy reliance on assumptions.
In the world of data analysis, knowledge is power. Equip yourself with the tools and strategies we’ve discussed. By doing so, you can navigate the statistical landscape confidently. Remember, the goal is to derive accurate insights that can drive informed decisions. So, roll up your sleeves, embrace the challenge, and let your data shine! With robust analysis, you hold the potential to unlock valuable outcomes that could transform your research or business strategies.
FAQs
Please let us know what you think about our content by leaving a comment down below!
Thank you for reading till here 🙂
For more insights on effective methods for analyzing data, check out these tips for effective data analysis in economics and statistics.
To understand the flow of statistical tests better, refer to this flow chart for statistical tests.
All images from Pexels
Data transformations can also save the day! Techniques like logarithmic or Box-Cox transformations can help stabilize variance and make data more normal-like. However, these transformations can change the scale of your data, so tread carefully!
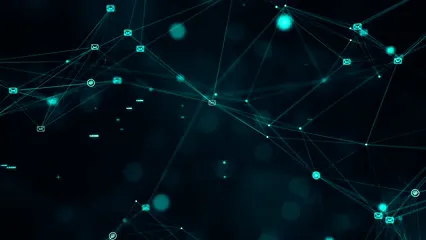
Another powerful tool is bootstrapping. This resampling method allows you to assess the distribution of a statistic without relying on traditional assumptions. It’s like having a statistical safety net, especially useful with small sample sizes or suspected violations. If you’re looking to get a solid grip on bootstrapping techniques, check out The Complete Guide to Data Analysis with R by Michael J. Crawley. It’s a comprehensive resource that can help you navigate the complexities of data analysis.
Ignoring these challenges can lead to misleading conclusions. For instance, if you conclude that a new marketing strategy is effective based on flawed data, you might steer your company in the wrong direction. Proactive evaluation of your data and methodologies is necessary.
In summary, the integrity of your analysis hinges on addressing these assumptions. By understanding the importance of meeting parametric statistics assumptions and employing alternatives when necessary, you can navigate the statistical landscape confidently, ensuring your findings are both valid and reliable. Let’s keep those assumptions in check and party on with robust analysis!
Understanding Parametric Statistics and Assumptions
The Basics of Parametric Statistics
Parametric statistics refers to a category of statistical tests that rely on assumptions about the parameters of the population distribution from which the sample data are drawn. These tests are essential for analyzing data effectively and producing valid results. Imagine using a fancy kitchen appliance—if you don’t follow the manual, you might end up with a kitchen disaster instead of a soufflé!
The significance of parametric statistics lies in its efficiency and power. When the assumptions are met, these tests can provide robust insights. Commonly used parametric tests include the t-test and ANOVA. The t-test compares means between two groups, while ANOVA (Analysis of Variance) is used for three or more groups. Both tests assume that the data follows a normal distribution, which is why understanding their underlying assumptions is key.
Key Assumptions of Parametric Statistics
Normality
At the heart of parametric statistics is the assumption of normality. This means that your data should be distributed in a bell-shaped curve. A normal distribution allows researchers to apply various statistical tests confidently. If your data resembles a rollercoaster instead of a smooth hill, you might want to reconsider your approach.
To test for normality, you can use several methods. The Shapiro-Wilk test is a popular choice, as it provides a statistical measure of normality. Alternatively, Q-Q (quantile-quantile) plots can visually indicate how well the data fits a normal distribution. If the points fall along a straight line, you’re in good shape! If they veer off track, it’s time to think of alternatives. If you’re interested in deepening your understanding of statistical learning, consider reading The Elements of Statistical Learning by Trevor Hastie. This insightful book dives into the principles of statistical learning and can enhance your analytical skills.
Homogeneity of Variance
Another crucial assumption is homogeneity of variance. This means that different groups being compared should have similar variances. If one group is wildly different, it’s like having a party where one guest shows up in a tuxedo and another in gym shorts; it simply doesn’t mesh well!
Testing for homogeneity can be done using Levene’s test or Bartlett’s test. Levene’s test is particularly useful because it is less sensitive to departures from normality. If these tests reveal significant differences in variance, you may need to adjust your analysis or consider using nonparametric methods. If you’re looking for a simple guide to SPSS, check out SPSS Statistics for Dummies by Keith McCormick. It’s a great resource for beginners who want to get started with statistical analysis!
Independence of Observations
The independence of observations is the cherry on top of the parametric statistics cake. Each observation should be unrelated to others. If your data points start gossiping and influencing each other, your results will likely be skewed. For example, if you’re surveying the health of a group of friends, their answers might influence one another, leading to biased results.
Violations of independence can lead to misleading conclusions. In experimental designs, random sampling helps maintain independence. However, if you find yourself in a situation where independence is compromised, rethinking your data collection strategy is essential to avoid potential pitfalls. If you’re interested in a solid introduction to data science, you might find R for Data Science: Import, Tidy, Transform, Visualize, and Model Data by Hadley Wickham to be a valuable resource!
In summary, understanding the assumptions of parametric statistics is like knowing the rules of a game before you play. When all assumptions are met, your analyses will yield more reliable and valid results. So, keep those assumptions in check, and you’ll be well on your way to statistical success!
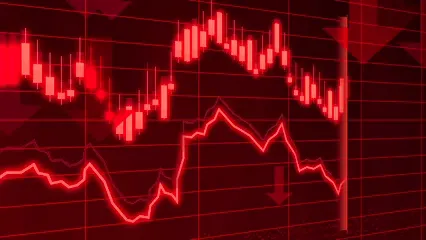
Challenges in Meeting Assumptions
Common Issues in Survey Data Analysis
Data Quality and Integrity
In the world of survey data analysis, the quality of your data is paramount. Imagine a beautifully wrapped gift only to find a rock inside. That’s what poorly designed surveys feel like! When your survey is ambiguous or filled with leading questions, bias sneaks in like an uninvited guest. Respondents’ interpretations can vary wildly, leading to unreliable data.
To tackle these issues, you need a solid plan. Start by investing time in survey design. Craft clear, concise questions that align with your research objectives. Test the survey on a small group to catch potential pitfalls. It’s like a dress rehearsal before the big show!
Next, focus on data cleaning. Address missing values, outliers, and inconsistencies. Validate responses to ensure logical coherence. This step is akin to putting on a fresh coat of paint—your data deserves to look its best! Random sampling techniques can also help minimize bias, ensuring your sample represents the broader population. This way, your insights won’t just be pretty on paper; they’ll be solid gold in their relevance!
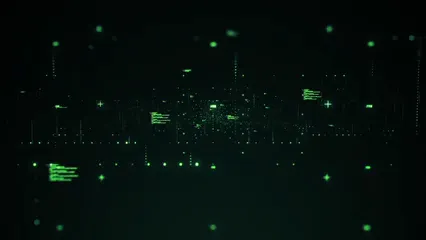
Missing Data
Missing data is like that one piece of a puzzle that just won’t show up. It can significantly impact your analysis, skewing results and leading to misguided conclusions. Understanding the types of missing data can help you decide on a strategic approach. There are three primary types:
1. Missing Completely at Random (MCAR): The missing values have no systematic relationship with the other data. Think of it as a random act of nature—unpredictable and often benign.
2. Missing at Random (MAR): The missingness is related to observed data but not the missing data itself. It’s like a party where some guests didn’t RSVP because they were busy but still left behind an empty seat.
3. Missing Not at Random (MNAR): Here, the missing data is related to the unobserved data. For example, people with lower incomes might skip a question about their salary. This one can be tricky!
To handle missing data effectively, consider different imputation methods. Mean imputation can work wonders for MCAR, while regression imputation or multiple imputation might be better for MAR. Always conduct a sensitivity analysis to assess the robustness of your results and understand the potential impact of missing data. This proactive approach will keep your analysis from derailing!
Consequences of Violating Assumptions
When assumptions of parametric statistics are violated, the consequences can be dire. Imagine preparing for a big test without studying; you’re setting yourself up for failure! Violations can lead to inaccurate estimates, unreliable confidence intervals, and misguided conclusions.
For instance, if normality is not met, your t-tests and ANOVAs may produce misleading results. You could believe you’ve found a significant difference when, in fact, it’s just statistical noise. Picture a marketer concluding their new strategy is a smashing success based on flawed data. Oops!
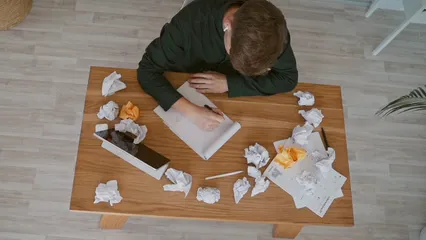
Case studies abound. In one notable example, researchers analyzed survey results on health behaviors. They assumed normal distributions, but the data was skewed due to outliers. Their findings suggested a drastic increase in health awareness, but in reality, the data reflected a few highly engaged respondents. This misinterpretation could lead to wasted resources and misguided public health strategies.
In another instance, a social scientist studied the impact of education on income. They failed to check the homogeneity of variance assumption and, as a result, reported that education had a significant effect on income. However, the analysis masked the fact that variances were vastly different across education levels, leading to an invalid conclusion.
These stories illustrate the importance of rigorously checking assumptions before diving into analysis. Ignoring them can lead to conclusions that not only misinform stakeholders but can also have real-world implications ranging from misguided business strategies to ineffective public policies. So, pay attention to those assumptions; they might just save your research from a rocky fate!
Strategies for Overcoming Challenges
Transforming Data
When your data doesn’t fit the mold, transformations can save the day! Techniques like logarithmic and Box-Cox transformations can help you whip that unruly data into shape. Logarithmic transformation takes the log of your data values, which can be especially useful for right-skewed distributions. It’s a bit like turning a pumpkin into a carriage—quite the transformation!
Box-Cox transformations go a step further. They search for the best transformation to stabilize variance and make the data more normal-like. It’s like finding the perfect outfit that fits just right for a fancy occasion. However, tread carefully! Transformations can change the scale of your data, which can lead to interpretive headaches. While they can help meet assumptions, they might also complicate the communication of your results. If you’re curious about the practical applications of data visualization, I recommend Data Visualization: A Practical Introduction by Kieran Healy. This book is a fantastic resource for honing your data presentation skills!
Pros of data transformation include improved adherence to assumptions and increased statistical power. But be cautious—over-transformation can lead to loss of interpretability. Always weigh the benefits against potential pitfalls, and remember, not every outfit works for every event.
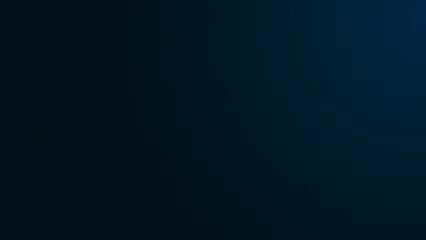
Using Nonparametric Tests
Sometimes, the best way to navigate the tricky terrain of assumption violations is to opt for nonparametric tests. These tests are the rebels of the statistical world. They don’t rely on the assumption of normality, making them preferable in various situations. If you find your data skewed or ordinal, nonparametric tests are your best friends.
Take the Mann-Whitney U test, for instance. It compares two independent groups without the need for normal distribution. It’s like a fair contest where everyone gets a chance to shine, regardless of their starting point. Then there’s the Kruskal-Wallis test, which is the go-to for comparing more than two groups. It’s nonparametric ANOVA but without the frills! If you want to explore more about data science, consider Data Science from Scratch: First Principles with Python by Joel Grus. It’s an excellent guide for getting started in the field!
Nonparametric tests can be less powerful than their parametric counterparts, especially with large samples. However, their flexibility can save you from the woes of assumption violations. They provide a reliable alternative when you need to draw conclusions without the constraints of strict assumptions.
Bootstrapping and Resampling Methods
Enter bootstrapping—a magical resampling technique! Bootstrapping allows you to create multiple samples from your data by sampling with replacement. Think of it as creating your own mini-universe from a single data set. It’s particularly useful when dealing with small sample sizes or suspected assumption violations.
By generating numerous resamples, you can estimate the distribution of a statistic without relying on strict assumptions. This gives you confidence intervals that reflect the uncertainty in your estimates. It’s like being able to predict the weather with a bit more flair and accuracy! If you’re looking for a comprehensive resource on Bayesian methods, check out Bayesian Data Analysis by Andrew Gelman. This book will deepen your understanding of Bayesian techniques!
Resampling methods help to overcome violations by providing a robust framework for inference. They let you focus on the data at hand instead of fretting over whether your assumptions are perfectly met. Just remember, while bootstrapping can be powerful, it’s essential to interpret results with care. The universe you create should still reflect the reality of your original data!
Bayesian Methods
Bayesian statistics are like the wise old sage of the statistical realm. They incorporate prior knowledge and update it with observed data, allowing for a flexible approach to analysis. This method sidesteps many of the strict assumptions required by traditional parametric tests, making it a valuable ally when assumptions are in jeopardy.
One of the key advantages of Bayesian methods is their ability to quantify uncertainty. By using prior distributions, you can incorporate existing knowledge into your analysis, which can be particularly helpful when working with small samples or when data is sparse. Instead of just a pass/fail judgment, you get a richer understanding of the probabilities involved.
Bayesian methods also allow for updating beliefs as new data emerges. As you gather more evidence, you can refine your estimates, much like adjusting your strategy in a game based on the moves of your opponents. This adaptability makes Bayesian statistics an appealing option for overcoming assumption violations and adapting to the ever-changing landscape of data.
In summary, whether you transform your data, rely on nonparametric tests, bootstrap your way to better estimates, or embrace the flexibility of Bayesian methods, you have a plethora of strategies at your disposal. Each method has its pros and cons, and the key lies in choosing the right tool for the job. So roll up your sleeves and tackle those challenges head-on!

Conclusion
Overcoming challenges related to parametric statistics assumptions in survey data analysis is crucial for generating reliable results. We’ve explored the essential assumptions: normality, homogeneity of variance, and independence of observations. Ignoring these assumptions can lead to statistical mischief—think of it as trying to bake a cake without following the recipe. Sure, you might get something edible, but it’s unlikely to be a masterpiece.
Addressing these challenges ensures that your findings are valid. We discussed the dire consequences of assumption violations, including Type I and Type II errors. Trust me, nobody wants to be the statistician who confidently declares a significant result, only to find out it was a false alarm. By employing alternative methods, such as nonparametric tests or data transformations, researchers can still glean meaningful insights from their data.
I encourage researchers and analysts to be proactive. Regularly evaluate your data for assumption adherence. If it doesn’t meet the criteria, don’t panic! Consider alternative methods like bootstrapping or Bayesian statistics. These approaches can provide robust analyses without the heavy reliance on assumptions.
In the world of data analysis, knowledge is power. Equip yourself with the tools and strategies we’ve discussed. By doing so, you can navigate the statistical landscape confidently. Remember, the goal is to derive accurate insights that can drive informed decisions. So, roll up your sleeves, embrace the challenge, and let your data shine! With robust analysis, you hold the potential to unlock valuable outcomes that could transform your research or business strategies.
FAQs
Please let us know what you think about our content by leaving a comment down below!
Thank you for reading till here 🙂
For more insights on effective methods for analyzing data, check out these tips for effective data analysis in economics and statistics.
To understand the flow of statistical tests better, refer to this flow chart for statistical tests.
All images from Pexels
But fear not! Just as there are ways to enhance your party attire, there are strategies to tackle assumption violations in parametric statistics. With the right techniques, you can elevate your analysis and ensure that your data gets the respect it deserves.

If you want to deepen your understanding of statistical methods, grab yourself a copy of Statistical Methods for the Social Sciences by Alan Agresti. This book is like the bible for anyone diving into survey data analysis, offering insights that can help improve your understanding of the assumptions you need to meet.
Let’s dive deeper into the challenges posed by these assumptions and the solutions that can help you overcome them!
Summary of Key Points
When it comes to parametric statistics, meeting assumptions is crucial for achieving valid results. If you ignore them, you might as well flip a coin! The consequences of assumption violations can lead to Type I and Type II errors. A Type I error occurs when you detect a significant effect that doesn’t exist, while a Type II error happens when you fail to detect a real effect.
So, what happens when these assumptions are shaky? In some cases, the data may not follow a normal distribution—think of a skewed income report with outliers galore. Here, using nonparametric tests is a robust alternative. These tests, such as the Mann-Whitney U test or Kruskal-Wallis test, do not rely on distribution assumptions, offering a safety net when parametric tests cannot be trusted. Consider reading Practical Statistics for Data Scientists: 50 Essential Concepts by Peter Bruce. This book provides insightful techniques and methods to strengthen your statistical toolbox!
Data transformations can also save the day! Techniques like logarithmic or Box-Cox transformations can help stabilize variance and make data more normal-like. However, these transformations can change the scale of your data, so tread carefully!
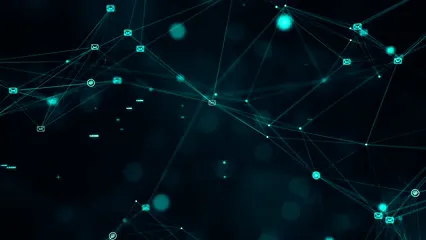
Another powerful tool is bootstrapping. This resampling method allows you to assess the distribution of a statistic without relying on traditional assumptions. It’s like having a statistical safety net, especially useful with small sample sizes or suspected violations. If you’re looking to get a solid grip on bootstrapping techniques, check out The Complete Guide to Data Analysis with R by Michael J. Crawley. It’s a comprehensive resource that can help you navigate the complexities of data analysis.
Ignoring these challenges can lead to misleading conclusions. For instance, if you conclude that a new marketing strategy is effective based on flawed data, you might steer your company in the wrong direction. Proactive evaluation of your data and methodologies is necessary.
In summary, the integrity of your analysis hinges on addressing these assumptions. By understanding the importance of meeting parametric statistics assumptions and employing alternatives when necessary, you can navigate the statistical landscape confidently, ensuring your findings are both valid and reliable. Let’s keep those assumptions in check and party on with robust analysis!
Understanding Parametric Statistics and Assumptions
The Basics of Parametric Statistics
Parametric statistics refers to a category of statistical tests that rely on assumptions about the parameters of the population distribution from which the sample data are drawn. These tests are essential for analyzing data effectively and producing valid results. Imagine using a fancy kitchen appliance—if you don’t follow the manual, you might end up with a kitchen disaster instead of a soufflé!
The significance of parametric statistics lies in its efficiency and power. When the assumptions are met, these tests can provide robust insights. Commonly used parametric tests include the t-test and ANOVA. The t-test compares means between two groups, while ANOVA (Analysis of Variance) is used for three or more groups. Both tests assume that the data follows a normal distribution, which is why understanding their underlying assumptions is key.
Key Assumptions of Parametric Statistics
Normality
At the heart of parametric statistics is the assumption of normality. This means that your data should be distributed in a bell-shaped curve. A normal distribution allows researchers to apply various statistical tests confidently. If your data resembles a rollercoaster instead of a smooth hill, you might want to reconsider your approach.
To test for normality, you can use several methods. The Shapiro-Wilk test is a popular choice, as it provides a statistical measure of normality. Alternatively, Q-Q (quantile-quantile) plots can visually indicate how well the data fits a normal distribution. If the points fall along a straight line, you’re in good shape! If they veer off track, it’s time to think of alternatives. If you’re interested in deepening your understanding of statistical learning, consider reading The Elements of Statistical Learning by Trevor Hastie. This insightful book dives into the principles of statistical learning and can enhance your analytical skills.
Homogeneity of Variance
Another crucial assumption is homogeneity of variance. This means that different groups being compared should have similar variances. If one group is wildly different, it’s like having a party where one guest shows up in a tuxedo and another in gym shorts; it simply doesn’t mesh well!
Testing for homogeneity can be done using Levene’s test or Bartlett’s test. Levene’s test is particularly useful because it is less sensitive to departures from normality. If these tests reveal significant differences in variance, you may need to adjust your analysis or consider using nonparametric methods. If you’re looking for a simple guide to SPSS, check out SPSS Statistics for Dummies by Keith McCormick. It’s a great resource for beginners who want to get started with statistical analysis!
Independence of Observations
The independence of observations is the cherry on top of the parametric statistics cake. Each observation should be unrelated to others. If your data points start gossiping and influencing each other, your results will likely be skewed. For example, if you’re surveying the health of a group of friends, their answers might influence one another, leading to biased results.
Violations of independence can lead to misleading conclusions. In experimental designs, random sampling helps maintain independence. However, if you find yourself in a situation where independence is compromised, rethinking your data collection strategy is essential to avoid potential pitfalls. If you’re interested in a solid introduction to data science, you might find R for Data Science: Import, Tidy, Transform, Visualize, and Model Data by Hadley Wickham to be a valuable resource!
In summary, understanding the assumptions of parametric statistics is like knowing the rules of a game before you play. When all assumptions are met, your analyses will yield more reliable and valid results. So, keep those assumptions in check, and you’ll be well on your way to statistical success!
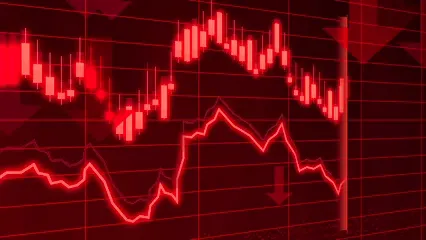
Challenges in Meeting Assumptions
Common Issues in Survey Data Analysis
Data Quality and Integrity
In the world of survey data analysis, the quality of your data is paramount. Imagine a beautifully wrapped gift only to find a rock inside. That’s what poorly designed surveys feel like! When your survey is ambiguous or filled with leading questions, bias sneaks in like an uninvited guest. Respondents’ interpretations can vary wildly, leading to unreliable data.
To tackle these issues, you need a solid plan. Start by investing time in survey design. Craft clear, concise questions that align with your research objectives. Test the survey on a small group to catch potential pitfalls. It’s like a dress rehearsal before the big show!
Next, focus on data cleaning. Address missing values, outliers, and inconsistencies. Validate responses to ensure logical coherence. This step is akin to putting on a fresh coat of paint—your data deserves to look its best! Random sampling techniques can also help minimize bias, ensuring your sample represents the broader population. This way, your insights won’t just be pretty on paper; they’ll be solid gold in their relevance!
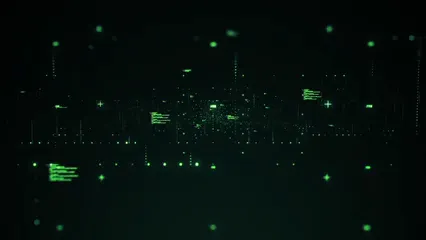
Missing Data
Missing data is like that one piece of a puzzle that just won’t show up. It can significantly impact your analysis, skewing results and leading to misguided conclusions. Understanding the types of missing data can help you decide on a strategic approach. There are three primary types:
1. Missing Completely at Random (MCAR): The missing values have no systematic relationship with the other data. Think of it as a random act of nature—unpredictable and often benign.
2. Missing at Random (MAR): The missingness is related to observed data but not the missing data itself. It’s like a party where some guests didn’t RSVP because they were busy but still left behind an empty seat.
3. Missing Not at Random (MNAR): Here, the missing data is related to the unobserved data. For example, people with lower incomes might skip a question about their salary. This one can be tricky!
To handle missing data effectively, consider different imputation methods. Mean imputation can work wonders for MCAR, while regression imputation or multiple imputation might be better for MAR. Always conduct a sensitivity analysis to assess the robustness of your results and understand the potential impact of missing data. This proactive approach will keep your analysis from derailing!
Consequences of Violating Assumptions
When assumptions of parametric statistics are violated, the consequences can be dire. Imagine preparing for a big test without studying; you’re setting yourself up for failure! Violations can lead to inaccurate estimates, unreliable confidence intervals, and misguided conclusions.
For instance, if normality is not met, your t-tests and ANOVAs may produce misleading results. You could believe you’ve found a significant difference when, in fact, it’s just statistical noise. Picture a marketer concluding their new strategy is a smashing success based on flawed data. Oops!
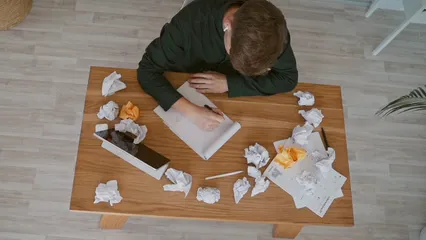
Case studies abound. In one notable example, researchers analyzed survey results on health behaviors. They assumed normal distributions, but the data was skewed due to outliers. Their findings suggested a drastic increase in health awareness, but in reality, the data reflected a few highly engaged respondents. This misinterpretation could lead to wasted resources and misguided public health strategies.
In another instance, a social scientist studied the impact of education on income. They failed to check the homogeneity of variance assumption and, as a result, reported that education had a significant effect on income. However, the analysis masked the fact that variances were vastly different across education levels, leading to an invalid conclusion.
These stories illustrate the importance of rigorously checking assumptions before diving into analysis. Ignoring them can lead to conclusions that not only misinform stakeholders but can also have real-world implications ranging from misguided business strategies to ineffective public policies. So, pay attention to those assumptions; they might just save your research from a rocky fate!
Strategies for Overcoming Challenges
Transforming Data
When your data doesn’t fit the mold, transformations can save the day! Techniques like logarithmic and Box-Cox transformations can help you whip that unruly data into shape. Logarithmic transformation takes the log of your data values, which can be especially useful for right-skewed distributions. It’s a bit like turning a pumpkin into a carriage—quite the transformation!
Box-Cox transformations go a step further. They search for the best transformation to stabilize variance and make the data more normal-like. It’s like finding the perfect outfit that fits just right for a fancy occasion. However, tread carefully! Transformations can change the scale of your data, which can lead to interpretive headaches. While they can help meet assumptions, they might also complicate the communication of your results. If you’re curious about the practical applications of data visualization, I recommend Data Visualization: A Practical Introduction by Kieran Healy. This book is a fantastic resource for honing your data presentation skills!
Pros of data transformation include improved adherence to assumptions and increased statistical power. But be cautious—over-transformation can lead to loss of interpretability. Always weigh the benefits against potential pitfalls, and remember, not every outfit works for every event.
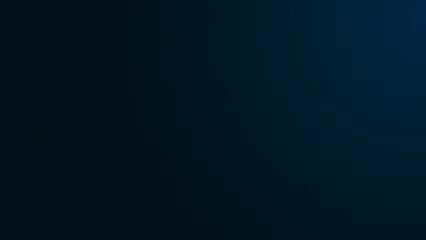
Using Nonparametric Tests
Sometimes, the best way to navigate the tricky terrain of assumption violations is to opt for nonparametric tests. These tests are the rebels of the statistical world. They don’t rely on the assumption of normality, making them preferable in various situations. If you find your data skewed or ordinal, nonparametric tests are your best friends.
Take the Mann-Whitney U test, for instance. It compares two independent groups without the need for normal distribution. It’s like a fair contest where everyone gets a chance to shine, regardless of their starting point. Then there’s the Kruskal-Wallis test, which is the go-to for comparing more than two groups. It’s nonparametric ANOVA but without the frills! If you want to explore more about data science, consider Data Science from Scratch: First Principles with Python by Joel Grus. It’s an excellent guide for getting started in the field!
Nonparametric tests can be less powerful than their parametric counterparts, especially with large samples. However, their flexibility can save you from the woes of assumption violations. They provide a reliable alternative when you need to draw conclusions without the constraints of strict assumptions.
Bootstrapping and Resampling Methods
Enter bootstrapping—a magical resampling technique! Bootstrapping allows you to create multiple samples from your data by sampling with replacement. Think of it as creating your own mini-universe from a single data set. It’s particularly useful when dealing with small sample sizes or suspected assumption violations.
By generating numerous resamples, you can estimate the distribution of a statistic without relying on strict assumptions. This gives you confidence intervals that reflect the uncertainty in your estimates. It’s like being able to predict the weather with a bit more flair and accuracy! If you’re looking for a comprehensive resource on Bayesian methods, check out Bayesian Data Analysis by Andrew Gelman. This book will deepen your understanding of Bayesian techniques!
Resampling methods help to overcome violations by providing a robust framework for inference. They let you focus on the data at hand instead of fretting over whether your assumptions are perfectly met. Just remember, while bootstrapping can be powerful, it’s essential to interpret results with care. The universe you create should still reflect the reality of your original data!
Bayesian Methods
Bayesian statistics are like the wise old sage of the statistical realm. They incorporate prior knowledge and update it with observed data, allowing for a flexible approach to analysis. This method sidesteps many of the strict assumptions required by traditional parametric tests, making it a valuable ally when assumptions are in jeopardy.
One of the key advantages of Bayesian methods is their ability to quantify uncertainty. By using prior distributions, you can incorporate existing knowledge into your analysis, which can be particularly helpful when working with small samples or when data is sparse. Instead of just a pass/fail judgment, you get a richer understanding of the probabilities involved.
Bayesian methods also allow for updating beliefs as new data emerges. As you gather more evidence, you can refine your estimates, much like adjusting your strategy in a game based on the moves of your opponents. This adaptability makes Bayesian statistics an appealing option for overcoming assumption violations and adapting to the ever-changing landscape of data.
In summary, whether you transform your data, rely on nonparametric tests, bootstrap your way to better estimates, or embrace the flexibility of Bayesian methods, you have a plethora of strategies at your disposal. Each method has its pros and cons, and the key lies in choosing the right tool for the job. So roll up your sleeves and tackle those challenges head-on!

Conclusion
Overcoming challenges related to parametric statistics assumptions in survey data analysis is crucial for generating reliable results. We’ve explored the essential assumptions: normality, homogeneity of variance, and independence of observations. Ignoring these assumptions can lead to statistical mischief—think of it as trying to bake a cake without following the recipe. Sure, you might get something edible, but it’s unlikely to be a masterpiece.
Addressing these challenges ensures that your findings are valid. We discussed the dire consequences of assumption violations, including Type I and Type II errors. Trust me, nobody wants to be the statistician who confidently declares a significant result, only to find out it was a false alarm. By employing alternative methods, such as nonparametric tests or data transformations, researchers can still glean meaningful insights from their data.
I encourage researchers and analysts to be proactive. Regularly evaluate your data for assumption adherence. If it doesn’t meet the criteria, don’t panic! Consider alternative methods like bootstrapping or Bayesian statistics. These approaches can provide robust analyses without the heavy reliance on assumptions.
In the world of data analysis, knowledge is power. Equip yourself with the tools and strategies we’ve discussed. By doing so, you can navigate the statistical landscape confidently. Remember, the goal is to derive accurate insights that can drive informed decisions. So, roll up your sleeves, embrace the challenge, and let your data shine! With robust analysis, you hold the potential to unlock valuable outcomes that could transform your research or business strategies.
FAQs
Please let us know what you think about our content by leaving a comment down below!
Thank you for reading till here 🙂
For more insights on effective methods for analyzing data, check out these tips for effective data analysis in economics and statistics.
To understand the flow of statistical tests better, refer to this flow chart for statistical tests.
All images from Pexels