Introduction
In the fast-paced world of healthcare, data is not just king; it’s the entire kingdom. With the increasing volume of patient data, healthcare professionals need powerful tools to make sense of it all. Enter Excel—a versatile spreadsheet software that can help you harness the power of descriptive statistics to improve patient care and operational efficiency. To kickstart your Excel journey, consider grabbing Microsoft Excel 2021 for Windows. It’s got all the bells and whistles to make your data dance!
Descriptive statistics summarize and simplify complex data, making it accessible and actionable. Think of it as a way to take a mountain of patient information and condense it into digestible bites. From understanding average patient ages to tracking treatment outcomes, descriptive statistics provide a solid foundation for clinical decision-making.
Descriptive statistics are vital in healthcare for summarizing complex patient data. Learn more about descriptive statistics.
This guide will explore how healthcare professionals can leverage descriptive statistics in Excel to extract valuable insights, streamline processes, and ultimately enhance patient outcomes. Whether you’re a physician, nurse, or healthcare administrator, mastering these statistics can lead to better patient care and improved operational efficiency. So, buckle up as we embark on this data-driven adventure that turns numbers into narratives!
Excel offers a treasure trove of functions to calculate key statistics, including mean, median, mode, and standard deviation. But fear not—this isn’t just a numbers game. We’ll illustrate how these tools can make your life easier and your data more compelling. You’ll learn how to visualize your findings, making your presentations not only more informative but also captivating. Let’s dive into the world of Excel and descriptive statistics, where data transforms from a daunting challenge into a powerful ally in healthcare.

Summary of Key Points
Understanding descriptive statistics is crucial for healthcare professionals, as it allows them to summarize and interpret complex data sets. This guide will cover:
- What Descriptive Statistics Are: Define key concepts, including mean, median, mode, variance, and standard deviation. These terms represent the fundamental building blocks of data analysis, providing insights into patient demographics and treatment effectiveness.
- Setting Up Excel for Descriptive Statistics: Step-by-step instructions on enabling the Data Analysis Toolpak and inputting data. This will ensure you have the right tools at your fingertips for efficient analysis.
- Key Excel Functions: Detailed explanations of essential functions such as AVERAGE, MEDIAN, and STDEV, with practical examples relevant to healthcare. Knowing how to use these functions can dramatically enhance your analytical capabilities. To dive deeper, check out Excel Formulas and Functions for Dummies.
- Visualizing Descriptive Statistics: Tips for creating effective charts and graphs to present data clearly and engagingly. Well-designed visuals can turn raw data into compelling stories.
- Real-World Applications: Case studies showcasing how healthcare professionals have successfully implemented descriptive statistics in their practice. These examples will inspire you to explore new ways to use data in your own work.
- Advanced Techniques and Tools: An overview of Excel add-ins and shortcuts to streamline your data analysis tasks. Efficiency is key in healthcare, and these tools will save you time.
- Troubleshooting Common Issues: Solutions for common problems faced when working with statistical data in Excel. Knowing how to overcome these hurdles will keep your analysis on track.
By the end of this guide, you will be equipped with the knowledge and skills to transform your data analysis capabilities, enabling you to make more informed decisions and improve patient care. Whether you’re analyzing patient outcomes or assessing operational efficiency, descriptive statistics in Excel will be your trusty sidekick.
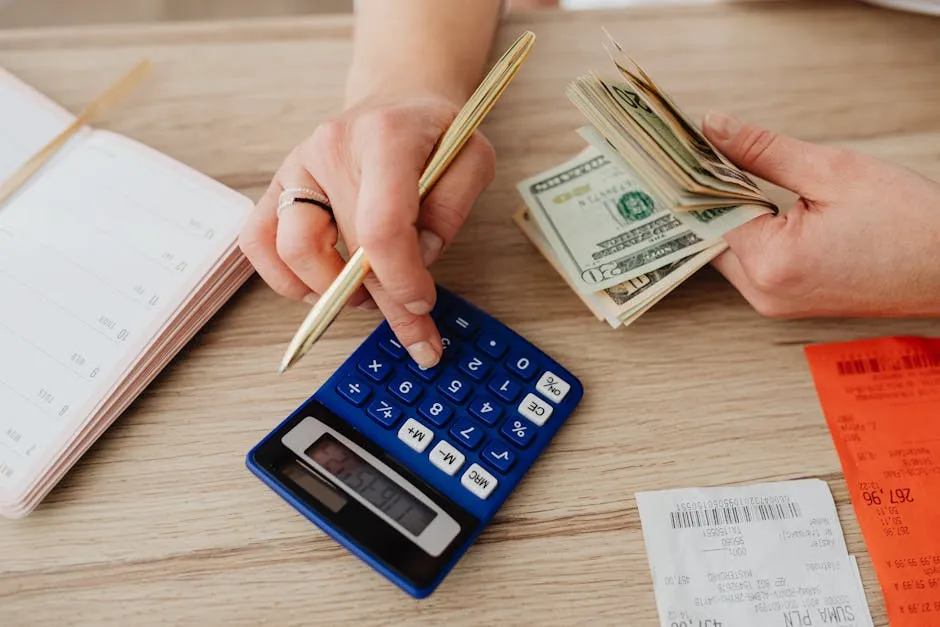
Understanding Descriptive Statistics in Healthcare
What Are Descriptive Statistics?
Descriptive statistics are essential for healthcare professionals. They help summarize and interpret complex data sets effectively. Imagine trying to understand a 100-page report on patient outcomes. Descriptive statistics condense this information into bite-sized pieces that make sense.
Key measures of descriptive statistics include:
- Mean: This is the average value. For instance, if you want to find the average age of patients in a clinic, you’d sum their ages and divide by the number of patients. Learn more about the mean in statistics.
- Median: This represents the middle value in a data set when ordered. It’s particularly useful in healthcare since it’s less affected by extreme values. For example, in a small sample of patient ages, the median might give a better sense of the typical age than the mean. Discover insights on median statistics.
- Mode: This is the most frequently occurring value in a data set. In healthcare, it can reveal the most common diagnosis among patients, helping identify prevalent health issues. Explore more about mode and statistics.
- Range: The range shows the difference between the highest and lowest values. Knowing the range of blood pressure readings can indicate the variability among patients.
- Variance: This measures how far each number in the set is from the mean. In clinical trials, variance helps understand the consistency of treatment effects.
- Standard Deviation: This is the square root of variance. It provides insights into how spread out the data is. A high standard deviation indicates high variability in patient outcomes.
Understanding these measures is crucial. They help healthcare professionals make informed decisions based on patient data, leading to improved patient care and operational efficiency. For a comprehensive guide to these concepts, consider Statistical Analysis with Excel for Dummies.
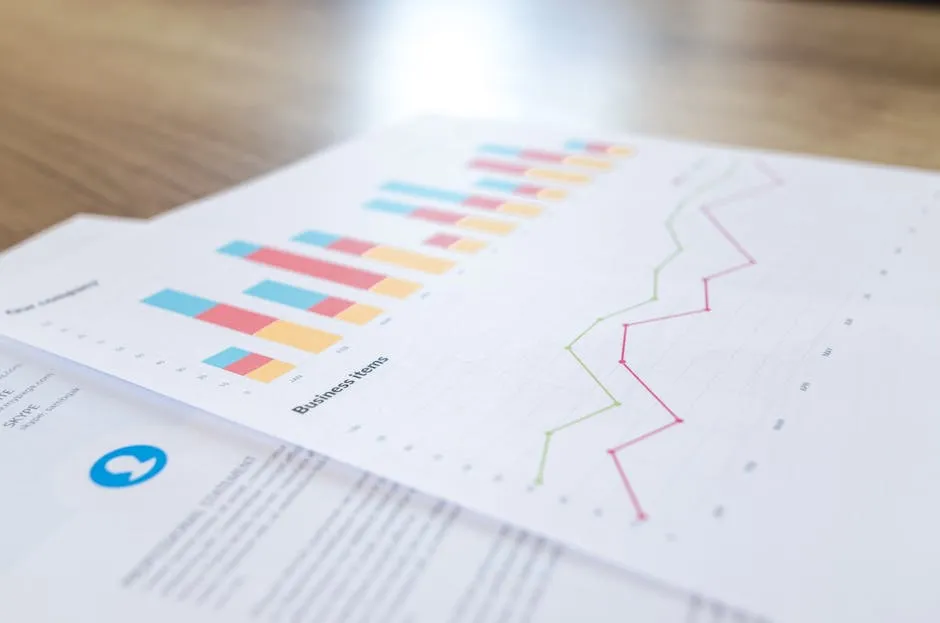
Types of Data Commonly Used
Healthcare data can be categorized into four types: nominal, ordinal, interval, and ratio data.
- Nominal Data: This type represents categories without any order. For example, a list of different blood types (A, B, AB, O) is nominal data. You can count occurrences but cannot rank them.
- Ordinal Data: Here, the categories have a meaningful order but no fixed interval. An example is a pain scale (mild, moderate, severe). While you know one is worse than the other, the difference between them isn’t quantifiable.
- Interval Data: This type includes numeric data with consistent intervals but no true zero point. A common example is temperature in Celsius. You can say that 30°C is hotter than 20°C, but 0°C doesn’t mean “no temperature.”
- Ratio Data: Ratio data has all the properties of interval data, plus a true zero point. An example is weight. Saying someone weighs 0 kg means they have no weight, and you can perform all mathematical operations on this data.
In healthcare settings, examples of these data types are abundant. Patient demographics often fall under nominal data, while clinical measurements like blood pressure can represent ratio data. Understanding these distinctions helps in selecting appropriate statistical methods for analysis.
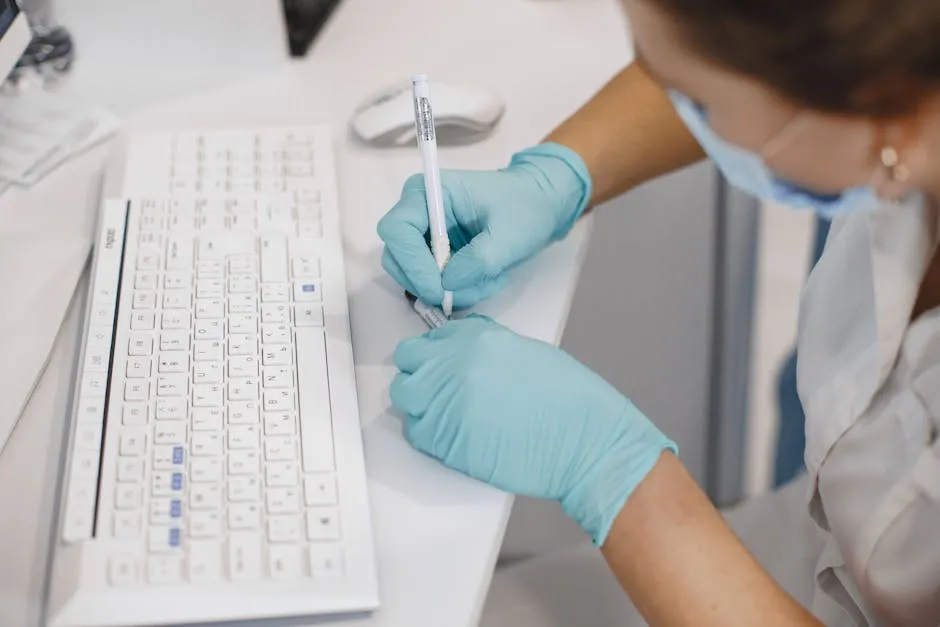
Setting Up Excel for Descriptive Statistics
Enabling the Data Analysis Toolpak
To take advantage of Excel’s powerful statistical capabilities, you need to enable the Data Analysis Toolpak. Here’s how to do it:
- Open Excel and click on the File tab.
- Choose Options from the menu.
- In the Excel Options dialog, select Add-Ins.
- At the bottom of the window, you’ll see a box labeled Manage. Select Excel Add-ins from the dropdown and click Go.
- In the Add-Ins box, check the box next to Analysis ToolPak and click OK.
- You should now see the Data Analysis option in the Data tab.
With this tool activated, you can easily perform various statistical analyses, including descriptive statistics, with just a few clicks. If you’re looking for a comprehensive guide on this process, consider Excel 2021: A Comprehensive Guide.
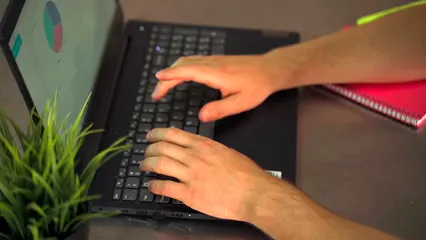
Inputting Data for Analysis
Organizing your data correctly is vital for effective analysis. Here are some best practices:
- Structured Data Sheets: Create a clean and structured layout for your data. Use clear headers for each column, such as patient ID, age, diagnosis, and treatment outcome. Consistency is key!
- Data Validation: Implement data validation rules to minimize errors. For instance, use dropdown lists for categorical variables like diagnoses to ensure uniformity in data entry.
- Best Practices for Data Input: Input data in a way that’s easy to analyze. Avoid merging cells, as it complicates data extraction. Each variable should have its own column.
- Error Checking: Regularly check for errors in your data entry. Use Excel’s conditional formatting to highlight anomalies, such as unusually high or low values, which may indicate input errors.
By following these practices, you ensure that your data is not only well-organized but also ready for insightful analysis, making your Excel experience smoother and more productive. For those looking to elevate their data visualization game, Data Visualization for Dummies is a great resource!

Key Excel Functions for Descriptive Statistics
Essential Functions and Their Applications
Excel is a powerful tool for healthcare professionals looking to analyze patient data effectively. Here are some essential functions that can help you extract meaningful insights from your data:
- AVERAGE: This function calculates the mean of a set of numbers. For instance, in a clinic, you can use
=AVERAGE(A2:A100)
to find the average age of patients. Knowing the average age can help in tailoring healthcare services to the specific demographic needs of your patient population. - MEDIAN: The median function,
=MEDIAN(A2:A100)
, provides the middle value in a dataset when arranged in order. This is particularly useful in healthcare, where outliers can skew results. For example, if you’re analyzing patient blood pressure readings, the median will give you a better sense of the typical reading than the mean, especially if a few patients have unusually high or low readings. - MODE: The mode function,
=MODE(A2:A100)
, identifies the most frequently occurring value in your data. This can be invaluable when trying to determine the most common conditions or treatments among your patients. For instance, if you want to find the most common diagnosis in your patient records, this function will quickly highlight that. - STDEV: Standard deviation measures the amount of variation or dispersion in your dataset. Using
=STDEV(A2:A100)
helps quantify how much patient outcomes vary. This is crucial in clinical trials where understanding variability can inform treatment efficacy and safety. - VARIANCE: Variance, calculated with
=VAR(A2:A100)
, provides insight into the data spread. In clinical trials, knowing the variance helps researchers understand the consistency of treatment effects. A low variance indicates that patient outcomes are similar, while a high variance suggests more diversity in responses.
Examples and case studies:
Let’s look at a few real-world applications of these functions:
- Patient Age Analysis: A hospital used the AVERAGE function to determine the average age of its patients. This data informed staff training programs to better address age-specific health concerns.
- Understanding Treatment Outcomes: A clinic analyzed the effectiveness of a new medication by calculating the MEDIAN of patient recovery times. The median provided a clearer picture of typical recovery experiences, guiding future treatment plans.
- Identifying Common Conditions: Using the MODE function, a healthcare provider identified the most common conditions treated in their facility. This information was pivotal in adjusting resource allocation and training staff to meet prevalent health issues effectively.
- Clinical Trial Variability: Researchers used STDEV to measure patient responses during a trial for a new drug. Understanding the variability in outcomes enabled them to tailor the treatment to specific patient demographics effectively.
- Evaluating Treatment Consistency: By calculating VARIANCE in a dataset of treatment outcomes, a hospital could identify which therapies produced consistent results and which ones exhibited wide variability, leading to more informed decisions on patient care protocols.
These functions are just the tip of the iceberg when it comes to using Excel for healthcare analytics, but they provide a robust foundation for understanding your patient population and improving care outcomes. To further enhance your skills, consider Excel 2021: The Most Updated Guide.
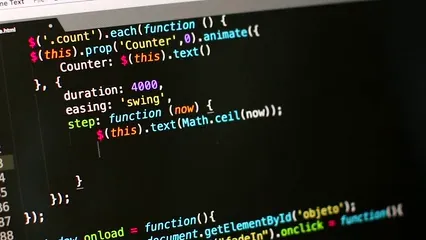
Visualizing Descriptive Statistics
Importance of Data Visualization in Healthcare
Data visualization plays a pivotal role in healthcare decision-making. Simply put, visuals make the data more comprehensible. A well-constructed chart or graph can convey complex information at a glance, enabling healthcare professionals to spot trends, patterns, and outliers swiftly. This clarity is essential when discussing patient data with colleagues or during presentations to stakeholders. In a field where every second counts, effective visualization can lead to faster, more informed decisions that ultimately enhance patient care.
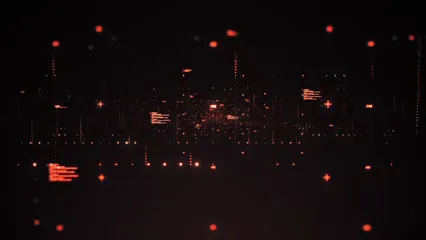
Creating Effective Charts and Graphs
To maximize the impact of your data, follow these steps to create various charts in Excel:
- Histograms: To visualize the distribution of a dataset, such as patient ages, select your data range, go to the Insert tab, choose Charts, and select Histogram. This chart will show the frequency distribution, helping you understand age demographics at a glance.
- Pie Charts: For categorical data, such as the percentage of different diagnoses, select your data, navigate to the Insert tab, and choose Pie Chart. This type of chart effectively illustrates how each category contributes to the whole, making it easy to communicate prevalence.
- Box Plots: Box plots are excellent for showing the spread and skewness of data, such as blood pressure readings. To create one, select your data, go to the Insert tab, and choose Box and Whisker. This visual helps identify outliers and understand the interquartile range.
Enhancing Presentations with Sparklines and Conditional Formatting
Sparklines are tiny charts that fit within a single cell. They provide a quick visual summary of trends. For instance, in a patient dashboard, sparklines can show blood pressure changes over time, allowing healthcare professionals to spot trends without sifting through numbers.
Conditional formatting is another powerful feature. Use it to highlight critical values in your dataset. For example, you can set rules to automatically color-code cells based on patient vitals. If a patient’s blood pressure is above a certain threshold, the cell can turn red, alerting staff to potential issues without the need for extensive data review.
Incorporating these visualization techniques into your data analysis process not only enhances understanding but also improves communication with colleagues, ensuring that vital information is conveyed effectively and efficiently. For a deeper understanding of data visualization techniques, consider The Complete Guide to Data Visualization with Excel.

Real-World Applications of Descriptive Statistics in Healthcare
Case Studies
Descriptive statistics play a vital role in analyzing patient outcomes. Hospitals rely on these statistics to track treatment effectiveness and improve patient care. For instance, a renowned hospital analyzed its patient recovery data using descriptive statistics. By calculating the average recovery time after surgery, they identified trends and implemented changes to reduce this time, enhancing patient satisfaction.
Another compelling example comes from a clinic specializing in chronic disease management. They used descriptive statistics to assess patient adherence to medication regimens. By tracking the median adherence rates, the clinic pinpointed specific demographics with lower compliance and targeted interventions. As a result, they improved adherence rates significantly, leading to better health outcomes.
Moreover, a community health center utilized descriptive statistics to evaluate vaccination rates among local populations. They calculated the mode of vaccinations administered and compared it to national averages. This analysis revealed gaps in coverage, prompting outreach initiatives that successfully increased vaccination rates in underserved areas. These case studies showcase how descriptive statistics can drive meaningful improvements in patient care.
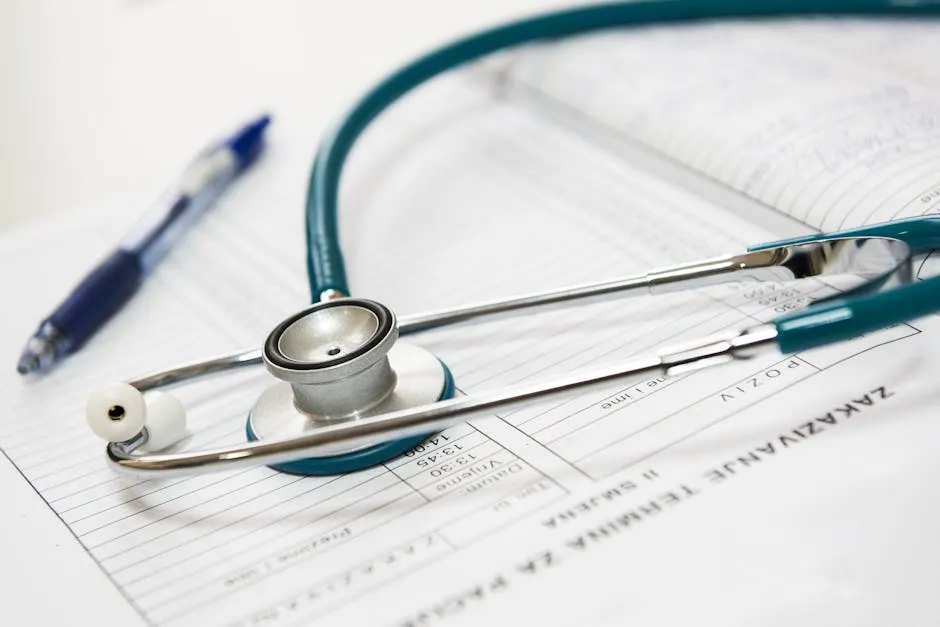
Benefits Realized by Healthcare Providers
Healthcare providers have witnessed numerous benefits from leveraging descriptive statistics. One major advantage is enhanced patient engagement. By presenting data on treatment outcomes in clear, understandable formats, providers can empower patients to take an active role in their health decisions. When patients see statistics regarding the effectiveness of a treatment plan, they are more likely to adhere to it.
Additionally, descriptive statistics contribute to better-informed clinical decisions. Healthcare providers can analyze data trends to identify which treatments yield the best results for specific conditions. This data-driven approach enables personalized treatment plans that cater to individual patient needs.
Furthermore, descriptive statistics foster operational efficiency within healthcare organizations. By analyzing patient flow and resource utilization, administrators can optimize staffing levels and reduce wait times. The result? A smoother experience for both patients and healthcare staff.
In summary, the effective use of descriptive statistics in healthcare leads to improved patient engagement, informed clinical decisions, and enhanced operational efficiency. As more healthcare professionals embrace data analytics, the potential for positive change in patient outcomes continues to expand. If you’re interested in exploring data science further, consider Data Science for Business: What You Need to Know about Data Mining and Data-Analytic Thinking.
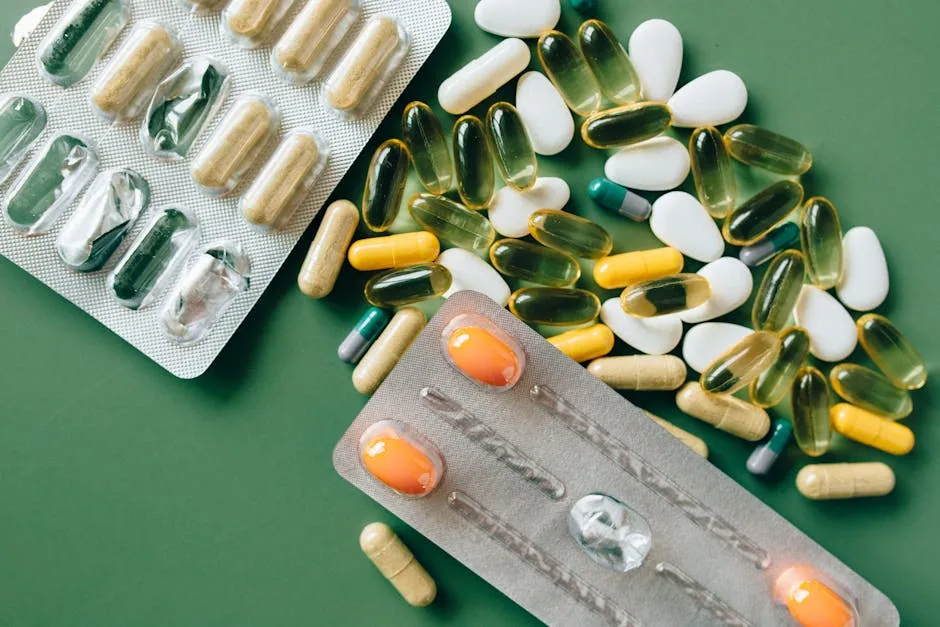
Advanced Techniques and Tools in Excel
Useful Excel Add-ins for Healthcare Professionals
Excel add-ins are invaluable tools for healthcare professionals seeking to enhance their data analysis capabilities. One standout add-in is the Analysis ToolPak. This powerful tool simplifies complex statistical analyses, including regression and descriptive statistics. With just a few clicks, healthcare providers can access essential functions that provide insights into patient data.
Another highly beneficial add-in is Power Query. This tool streamlines the process of importing and transforming data from different sources. For healthcare professionals managing large datasets from electronic health records, Power Query makes data cleaning and preparation a breeze, allowing for more focus on analysis rather than data wrangling. If you’re looking for a deeper understanding of this tool, Excel Power Query for Dummies is a great resource!
Power Pivot is another essential add-in that enhances data modeling capabilities. It enables users to work with large datasets and perform complex calculations efficiently. For example, healthcare organizations can easily analyze patient demographics and treatment outcomes simultaneously, leading to more comprehensive insights.
In addition to these tools, add-ins like Solver can optimize resource allocation, while Lucidchart helps create flowcharts for clearer presentations. By utilizing these Excel add-ins, healthcare professionals can elevate their data analysis, leading to more informed decisions and improved patient care. If you’re interested in mastering this software, don’t forget to check out Excel 2021: The Ultimate Guide for Beginners.
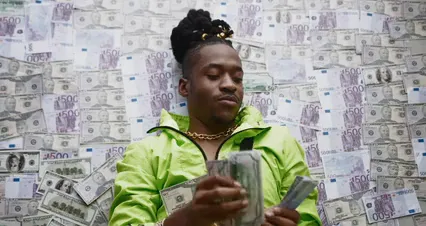
Excel Shortcuts for Efficiency
Efficiency is key when working with data in Excel. Familiarity with essential shortcuts can save time and streamline analysis. One of the most useful shortcuts is Ctrl + C to copy data and Ctrl + V to paste it. This basic function speeds up data entry and manipulation.
For those frequently navigating through large datasets, Ctrl + Arrow keys allows for quick movement across cells. This can be especially handy when analyzing patient data spread over multiple rows and columns. Another great shortcut is Alt + E, S, V, which opens the Paste Special dialog, enabling users to paste values or formats selectively.
Additionally, using F2 allows for quick editing of the selected cell, while Ctrl + Z undoes the last action—an absolute lifesaver when making mistakes. Lastly, Ctrl + Shift + L applies filters to your data, helping to focus on specific subsets of information quickly.
By mastering these Excel shortcuts, healthcare professionals can enhance their productivity, making data analysis not only faster but also more efficient. For those eager to expand their knowledge, Excel 2021: Tips and Tricks for Beginners is a fantastic resource!
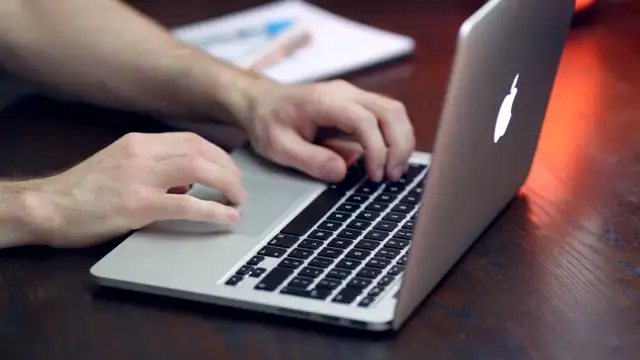
Troubleshooting Common Issues
Common Problems and Solutions
When using Excel for descriptive statistics, you might encounter a few hiccups along the way. Let’s tackle some common problems and their solutions!
First up, data formatting errors. If your numbers look like text, Excel will throw a tantrum when you try to calculate averages or standard deviations. To fix this, highlight your cells, right-click, select Format Cells, and choose the appropriate number format. Voila! Your data is now ready for action.
Next, there are pesky function errors. Ever typed =AVERAGE(A1:A10)
and received an error message instead of a number? This could be due to referencing an empty cell or a cell containing text. Double-check your range and make sure all the cells contain valid numerical data.
How about dealing with blank cells? They can skew your calculations. If you want to avoid this, try using the =AVERAGEIF(range, criteria)
function, where you can specify conditions to exclude blanks. Keeping your calculations neat and tidy is essential!
Lastly, if you encounter errors when using the Data Analysis Toolpak, ensure it’s enabled. Go to File > Options > Add-Ins, and check if it’s activated. If not, add it from the Excel Add-ins list. This Toolpak is your trusty sidekick for statistical analysis and should always be at your disposal!
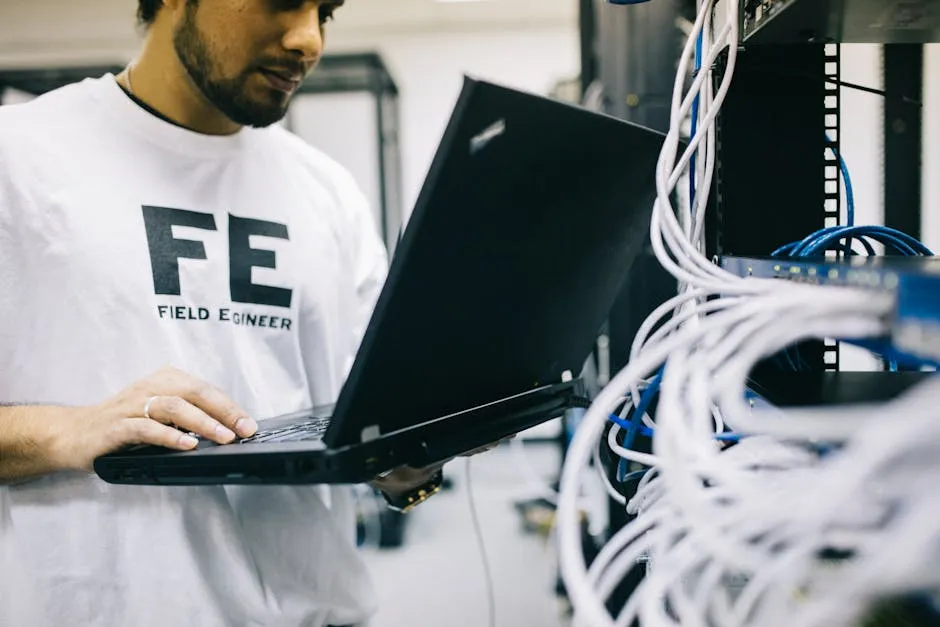
Best Practices for Maintaining Data Integrity
Maintaining data integrity is crucial for accurate analysis. Here are some best practices to keep your data reliable and robust!
- Start by implementing data validation. This feature allows you to restrict entries in your Excel sheets. For instance, if you have a column for patient age, set rules to ensure only numeric values within a certain range can be entered. This way, you avoid those embarrassing moments when your age column has letters—oops!
- Next, regularly back up your data. Save your workbook in multiple locations, such as a cloud service or an external drive. You’d be surprised how often computers decide to misbehave, and losing data can be a nightmare!
- Also, use consistent naming conventions for your columns. This helps you and your team quickly identify what each column represents. Avoid cryptic abbreviations. Clear names lead to fewer misunderstandings down the line.
- Finally, check your work! Regularly review your data for anomalies. Use Excel’s conditional formatting to highlight outliers or unexpected values. This way, you can catch errors before they derail your analysis. Keeping your data clean ensures that your insights are as accurate as possible.
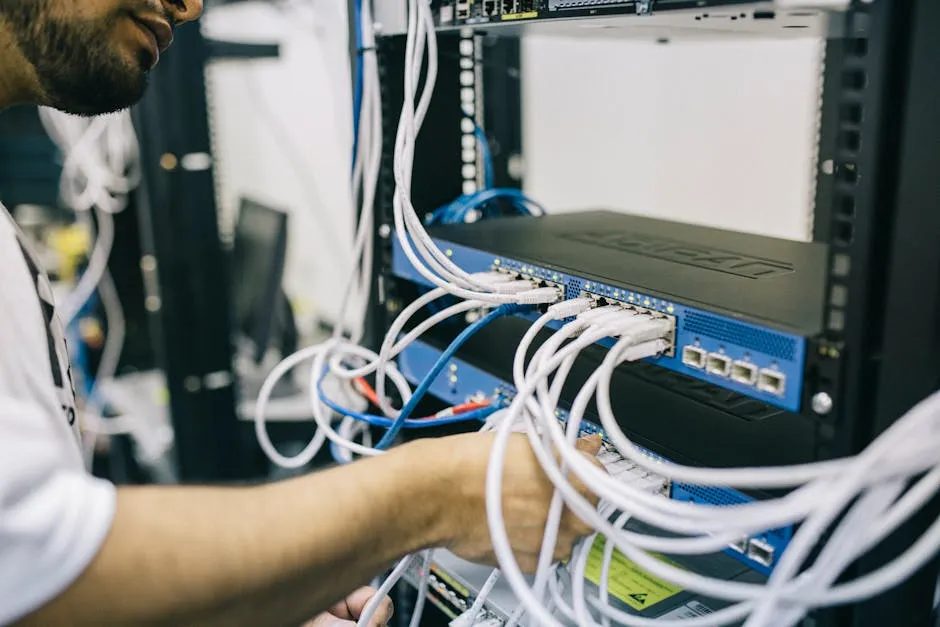
Conclusion
Descriptive statistics in Excel are indispensable tools for healthcare professionals seeking to make data-driven decisions. By mastering the use of these statistics, you can enhance your understanding of patient data, inform clinical decisions, and improve overall patient care.
Remember, the healthcare landscape is continuously evolving, and being adept at data analysis is not just an advantage; it’s a necessity. Embrace the power of Excel and descriptive statistics to transform your practice and ultimately improve patient outcomes. For those looking to dive deeper into data analysis techniques, The Excel Analyst’s Guide to Access is a must-read!
In summary, leveraging Excel for descriptive statistics means you’re not just crunching numbers; you’re unlocking insights that can lead to better patient care. So, roll up your sleeves and dive into your data—you’ll be amazed at what you can uncover!
FAQs
What is the difference between descriptive and inferential statistics?
Descriptive statistics summarize the characteristics of a dataset, while inferential statistics draw conclusions and make predictions about a population based on sample data.
Can I use Excel for large datasets in healthcare?
Yes, Excel can handle large datasets, especially with tools like Power Query and Power Pivot, which allow for efficient data manipulation and analysis.
What are some common mistakes to avoid when using descriptive statistics in Excel?
Common mistakes include using inappropriate functions for data types, neglecting data validation, and misinterpreting results. It’s crucial to understand the context of your data before analysis.
How can I learn more about Excel for healthcare data analysis?
There are numerous online courses, tutorials, and books focused on Excel for healthcare professionals, along with community forums where you can seek advice and share experiences.
Please let us know what you think about our content by leaving a comment down below!
Thank you for reading till here 🙂
All images from Pexels