Introduction
In today’s fast-paced world, accurate forecasting is akin to having a crystal ball. The Department of Statistics Singapore (DOS) plays a pivotal role in this arena. With data being the new currency, DOS transforms raw numbers into foresight that helps businesses, government, and individuals make informed decisions.
The Department of Statistics Singapore is crucial for transforming data into actionable insights. Comparing statistical models from the Department of Statistics Singapore vs global standards
At the heart of DOS’s operations lies a treasure trove of methodologies. These methods enable them to sift through mountains of data, extracting valuable insights that guide Singapore’s strategic initiatives. But what’s the secret sauce behind this statistical wizardry?
Let’s unpack this! DOS employs a mix of traditional and contemporary techniques to forecast economic trends. From classical statistical methods to cutting-edge machine learning algorithms, they’ve got it all covered. Speaking of cutting-edge, if you want to dive deeper into predictive analytics, check out Predictive Analytics: The Power to Predict Who Will Click, Buy, Lie, or Die by Eric Siegel. This book is a must-read for anyone looking to understand the future of data-driven decision-making.
Why is statistical forecasting so crucial? Imagine planning your vacation without knowing the weather forecast. Would you pack your umbrella or your sunglasses? Similarly, economic forecasting helps policymakers understand trends and make decisions that affect everything from GDP growth to employment rates.
In this article, we’ll shine a light on how DOS’s forecasting methodologies work. We’ll take a closer look at the significance of these techniques in shaping Singapore’s economic landscape. Furthermore, we’ll explore how the integration of data analytics and machine learning propels the nation ahead in a competitive global market.
So buckle up! We’re diving into the fascinating world of forecasting with the Department of Statistics Singapore. Get ready to discover how they stay ahead of the curve, turning data into future possibilities!
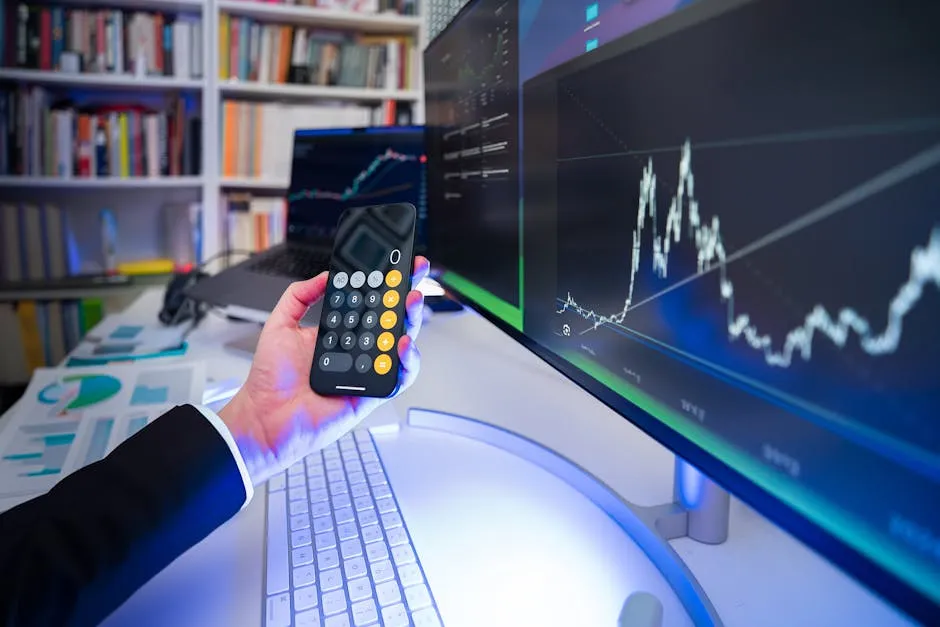
Summary of Key Points
Understanding future trends is essential for everyone, from governments to businesses and individuals. The methodologies used by the Department of Statistics Singapore emphasize their dedication to accuracy and relevance in data interpretation.
- Importance of Accurate Forecasting: Grasping economic indicators is vital for effective policymaking and strategic planning. It’s like having a roadmap on a road trip—essential for reaching your destination without detours.
- Methodologies Employed: DOS utilizes a mix of classical statistical methods, machine learning techniques, and hybrid models. These approaches enhance the accuracy of forecasts, ensuring that insights are both reliable and actionable. If you’re curious about hybrid methods, check out Forecasting: Methods and Applications by Spyros Makridakis.
- Real-World Applications: Numerous case studies showcase successful forecasting initiatives across sectors. From trade and manufacturing to public health, these insights help organizations make informed decisions that drive growth and efficiency.
- Challenges and Solutions: Forecasting isn’t without its hurdles. Common challenges include data quality issues and the need for adaptability in rapidly changing economic conditions. DOS employs innovative solutions to tackle these challenges head-on, ensuring that their forecasts remain relevant.
- Future Trends: As we look ahead, the evolution of forecasting methodologies promises even greater advancements. Emerging technologies and data analytics will play a key role in enhancing Singapore’s analytical capabilities.
By the end of this article, readers will have a thorough understanding of the forecasting methodologies employed by the Department of Statistics Singapore. They’ll appreciate the broader implications for Singapore’s economy and how these insights shape future strategies.
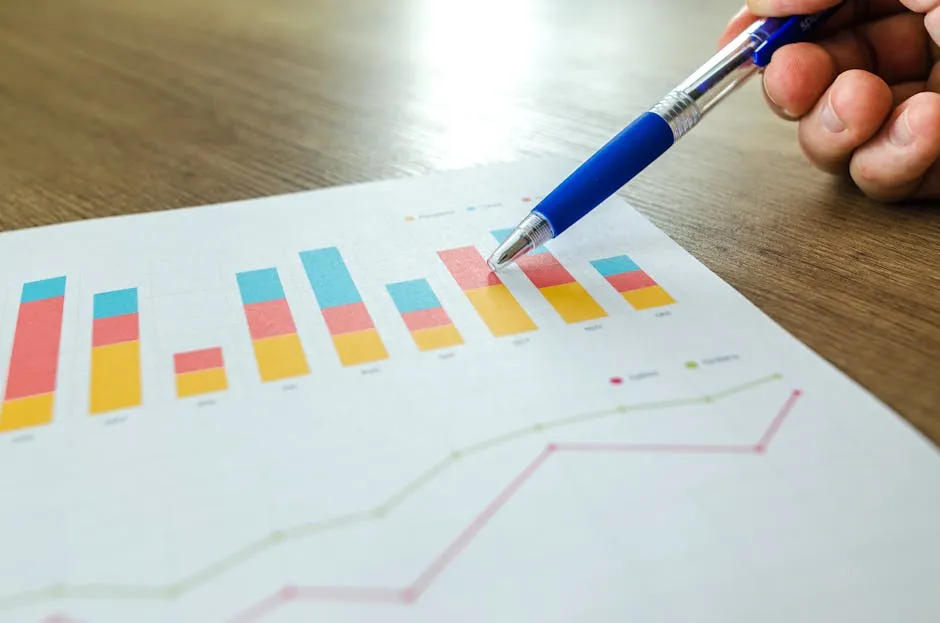
Methodologies of Forecasting Trends
Overview of Statistical Methodologies
Descriptive Statistics
Descriptive statistics serve as the backbone for data analysis. They help summarize and visualize data, making it easier to grasp complex information. Think of it as the “Cliff Notes” for data! These statistics include measures like mean, median, and mode, which provide a snapshot of central tendencies.
Visualization techniques, such as graphs and charts, further enhance understanding. Imagine trying to decipher a mountain of numbers without a pie chart! DOS uses these tools to communicate trends effectively. By presenting data visually, they make it accessible to a wider audience. This clarity aids policymakers in making informed decisions based on accurate data representations.
Moreover, descriptive statistics allow for quick comparisons. By examining different datasets, stakeholders can spot significant trends or anomalies. For instance, a sudden spike in retail sales during a holiday season can be easily identified through these methods. In summary, descriptive statistics are crucial for simplifying complex data, enabling better decision-making. Descriptive statistics in manufacturing plant
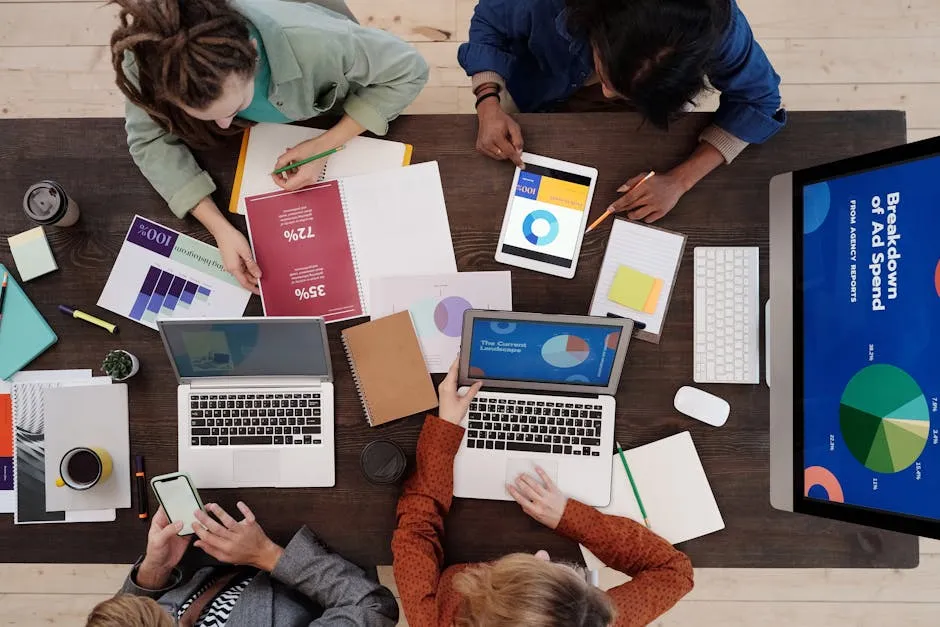
Descriptive statistics are fundamental for data analysis, providing essential summaries and visualizations. Descriptive statistics in manufacturing plant
Inferential Statistics
While descriptive statistics summarize data, inferential statistics take things up a notch. They allow analysts to make predictions about larger populations based on sample data. DOS employs inferential statistics to draw conclusions from data samples, using techniques like hypothesis testing and confidence intervals.
Imagine trying to predict the next hit song using only a small group of radio listeners. This is similar to how inferential statistics work. By analyzing a sample, DOS can infer trends that apply to the entire population. This method is particularly useful when collecting extensive data is impractical or costly.
The beauty of inferential statistics lies in its ability to quantify uncertainty. Analysts can estimate how confident they are in their predictions, offering a margin of error. This is vital for decision-makers who rely on accurate forecasts. Ultimately, inferential statistics enable DOS to transform sample data into actionable insights, shaping policies that impact the nation’s economy. The problem with inferential statistics
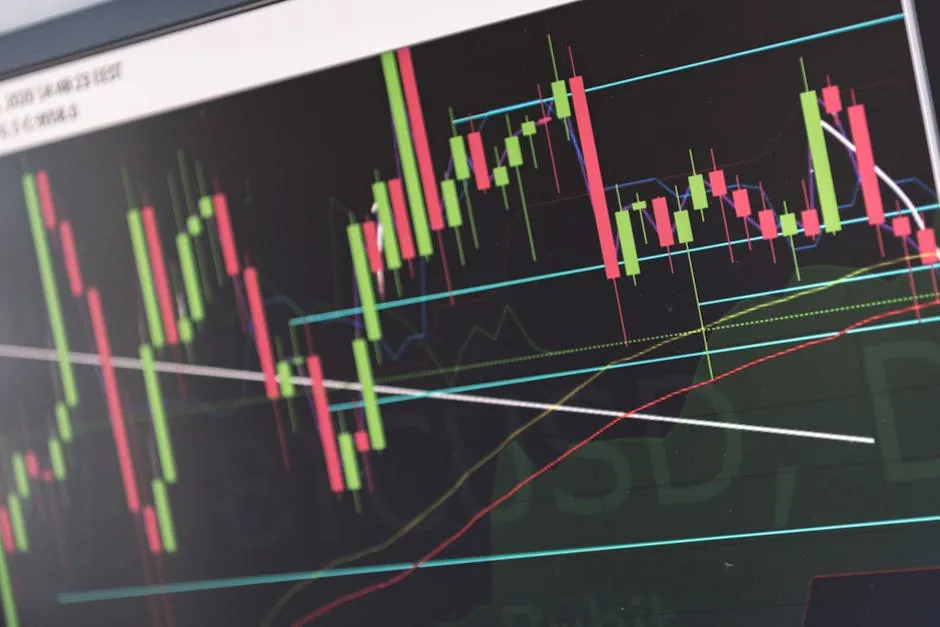
Inferential statistics allow analysts to make predictions about larger populations based on sample data, offering crucial insights for decision-making. The problem with inferential statistics
Time Series Analysis
Introduction to Time Series
Time series data is all about observing how things change over time. It consists of a sequence of data points typically measured at successive intervals. Think stock prices, weather patterns, or sales figures. The beauty of time series analysis lies in its ability to reveal trends and seasonal patterns.
Understanding these patterns can provide valuable insights into future behaviors. For example, if retail sales consistently rise during the holiday season, businesses can prepare accordingly. Time series analysis helps organizations anticipate fluctuations and adjust strategies in real time. If you want to master this topic, grab a copy of Machine Learning for Time Series Forecasting by Francesca Lazzeri.
Moreover, this analysis often involves techniques like seasonal decomposition. By breaking down data into trend, seasonal, and residual components, analysts can better understand underlying factors. This detailed examination allows for more precise forecasting, enabling organizations to make informed decisions. Forecasting trends in manufacturing with time series analysis

Time series analysis provides insights into trends and seasonal patterns, helping organizations prepare for future behaviors. Forecasting trends in manufacturing with time series analysis
ARIMA Models
The Autoregressive Integrated Moving Average (ARIMA) model is a powerful tool for forecasting time series data. It combines three components: autoregression, differencing, and moving averages. This model excels in capturing temporal dependencies, making it ideal for economic forecasting.
In practice, ARIMA models analyze historical data to identify patterns. For instance, if GDP growth tends to follow certain trends, ARIMA can recognize this and help predict future growth. It’s like having a trusty road map for navigating economic landscapes! If you want to learn more about effective forecasting techniques, you can explore The Art of Thinking Clearly by Rolf Dobelli.
ARIMA models also require careful parameter selection. Analysts must determine the order of autoregressive, differencing, and moving average components. This tuning process ensures the model accurately reflects the data’s characteristics. Once set up, ARIMA can generate forecasts that guide policymakers and businesses alike.
One significant advantage of ARIMA is its flexibility. It can adapt to various time series data, making it applicable across sectors. From predicting inflation rates to assessing consumer behavior, ARIMA models are invaluable for informed decision-making.
SARIMA Models
Seasonal ARIMA (SARIMA) builds upon the ARIMA framework by incorporating seasonal variations. It recognizes that some data exhibit patterns that repeat over specific intervals, such as months or quarters. This model is particularly relevant for industries affected by seasonal trends, like agriculture and retail.
SARIMA introduces seasonal parameters, allowing for a more nuanced analysis. By capturing these recurring patterns, analysts can make more accurate predictions. For example, if ice cream sales peak every summer, SARIMA can consider this trend in its forecasts.
Implementing SARIMA involves identifying both non-seasonal and seasonal parameters. This dual-focus ensures analysts can account for every aspect of the data. The model’s versatility makes it a go-to choice for organizations looking to capitalize on seasonal trends.
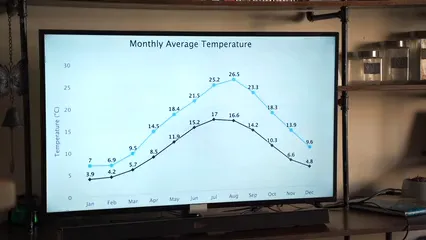
Machine Learning Techniques
Introduction to Machine Learning in Forecasting
Machine learning has revolutionized forecasting methodologies. It enables data-driven predictions by analyzing large datasets for patterns that traditional methods might miss. As technology advances, machine learning applications in forecasting have gained traction, becoming indispensable for organizations.
The evolution of machine learning has led to the development of various algorithms. These include decision trees, support vector machines, and neural networks. By leveraging these techniques, organizations can enhance forecasting accuracy and uncover hidden insights in their data. If you’re interested in getting started with machine learning, check out Introduction to Machine Learning by Ethem Alpaydin.
Moreover, machine learning models can adapt to new data. Unlike traditional methods, which may require retraining from scratch, many machine learning algorithms can learn incrementally. This flexibility ensures that forecasts remain relevant in rapidly changing environments.
As organizations seek to harness the power of data analytics, machine learning plays a pivotal role in shaping future forecasting methodologies. Its ability to analyze vast amounts of information opens doors to innovative insights and informed decision-making.

Popular Models
Several widely-used machine learning models have emerged in the forecasting space. Random Forest is a robust ensemble method that combines multiple decision trees for improved accuracy. It excels in handling complex datasets and capturing non-linear relationships.
Support Vector Regression (SVR) is another popular technique. It finds a hyperplane that best separates data points, making it effective for regression tasks. SVR is particularly useful for datasets with high dimensionality, allowing for accurate forecasts even in challenging scenarios.
Neural networks, especially deep learning models, have gained significant traction in forecasting. These models mimic human brain functions, allowing them to learn complex patterns from data. Long Short-Term Memory (LSTM) networks, a type of recurrent neural network, are particularly adept at capturing long-term dependencies in time series data.
The versatility of these machine learning models enables organizations to tailor their forecasting approaches to specific needs. By selecting the right model, businesses can unlock valuable insights and make data-driven decisions that drive growth. If you’re curious about diving deeper into data science, consider reading Data Science for Business: What You Need to Know about Data Mining and Data-Analytic Thinking by Foster Provost.
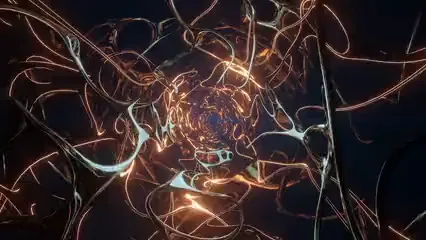
Hybrid Approaches
Combining statistical and machine learning methods leads to hybrid approaches that enhance forecasting accuracy. These models leverage the strengths of both worlds, offering a more comprehensive analysis of data.
For instance, a hybrid model might use a traditional statistical method to identify trends, followed by a machine learning algorithm to refine predictions. This approach captures both the underlying patterns and the complexities of modern datasets.
Hybrid models are particularly effective in real-world applications. They can adapt to changing conditions, ensuring forecasts remain relevant. As organizations face increasingly complex challenges, hybrid methodologies provide a valuable framework for informed decision-making.
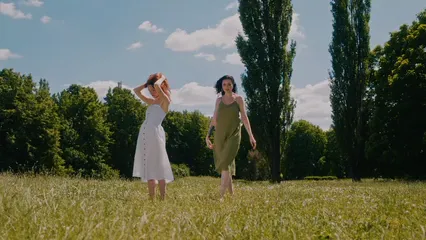
Case Studies
Economic Forecasting
One notable case study involved the Department of Statistics Singapore using statistical models to forecast GDP growth. By analyzing historical GDP data and identifying key economic indicators, DOS employed ARIMA models to predict future growth rates. The results proved instrumental in shaping national economic policies, enabling timely interventions and strategic planning.
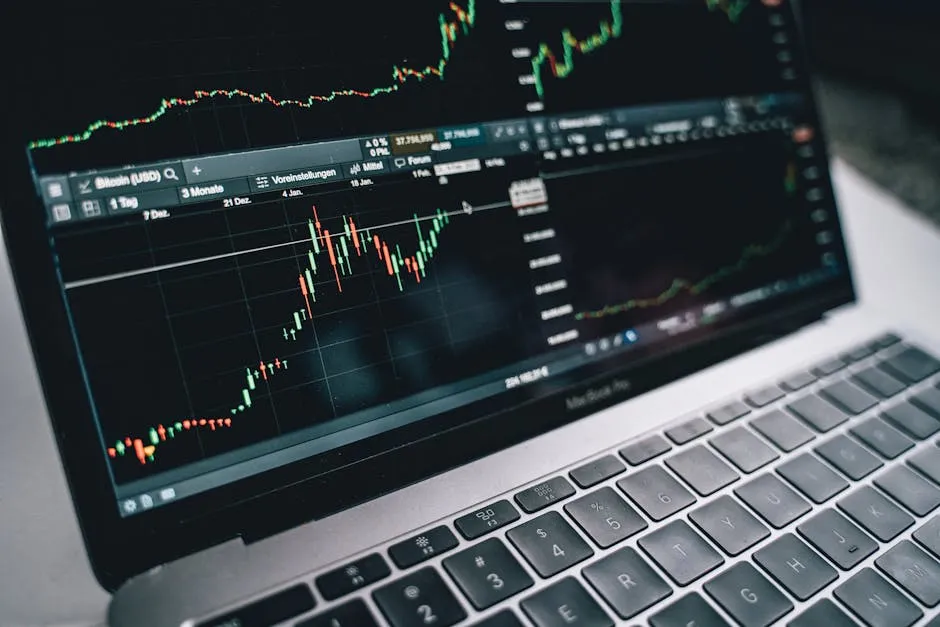
Health Sector Insights
During the COVID-19 pandemic, forecasting methodologies played a crucial role in public health policy. DOS utilized time series analysis to predict infection rates and assess the impact of various interventions. These insights guided decision-makers in implementing measures to control the spread of the virus, ultimately saving lives and protecting the healthcare system.
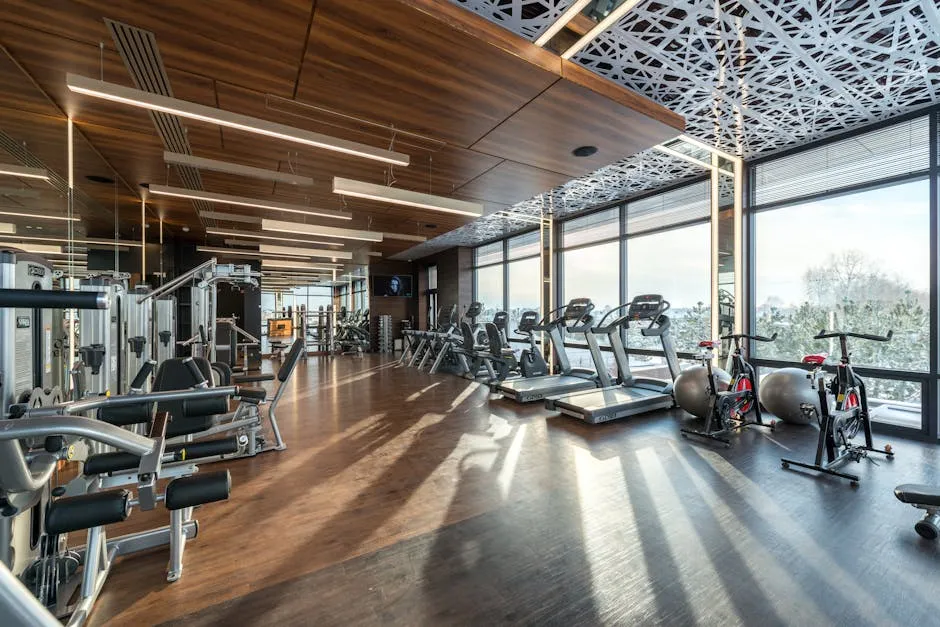
Challenges in Forecasting and Innovative Solutions
Data Quality Issues
In forecasting, high-quality data is like gold. Without it, predictions become as reliable as a weather forecast in Singapore during monsoon season. The Department of Statistics Singapore (DOS) understands this well. They prioritize data integrity to ensure accurate forecasting.
Quality data starts with thorough collection processes. DOS employs multiple methods to gather data from various sources. Surveys, administrative records, and sensors all contribute to a comprehensive data set. But it doesn’t stop there. They also focus on data cleaning. This process involves identifying and correcting errors or inconsistencies in the data. After all, nobody wants to make decisions based on faulty information.
Furthermore, DOS implements strict quality assurance protocols. These protocols ensure that data meets established standards before being used for analysis. Regular audits and checks help maintain data reliability. This ongoing commitment to data quality allows DOS to provide accurate and timely forecasts. In a world driven by data, ensuring quality is a non-negotiable aspect of the forecasting process.
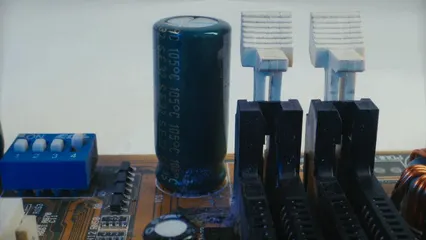
Adaptability to Change
The economic landscape is ever-changing, and forecasting models must keep up. Rapid shifts in the economy—such as sudden market crashes or unexpected growth spurts—pose challenges for traditional forecasting methods. DOS has recognized this need for adaptability and has taken proactive measures.
One key approach involves continuously updating and refining forecasting models. By integrating the latest data, DOS can adjust their models to reflect current conditions. For instance, during the COVID-19 pandemic, traditional models struggled to keep pace. DOS quickly adapted by incorporating real-time data and adjusting their approaches to better predict economic trends.
Moreover, they utilize scenario analysis. This method allows them to forecast various potential outcomes based on different economic conditions. By considering multiple scenarios, DOS can prepare for uncertainty. This adaptability ensures that forecasts remain relevant, allowing stakeholders to make informed decisions in a fluctuating economic environment.

Technological Integration
Technology is a game changer in forecasting. DOS has embraced advancements in technology to enhance accuracy and efficiency. Machine learning, artificial intelligence, and big data analytics are now integral to their forecasting methodologies.
Machine learning algorithms can analyze vast amounts of data quickly. They identify patterns that might escape traditional methods. This capability allows for more accurate predictions and insights. For example, machine learning can detect subtle trends in consumer behavior, helping businesses adjust strategies accordingly. If you’re eager to learn how to analyze data in Python, consider picking up Python for Data Analysis by Wes McKinney.
Additionally, the integration of automated systems streamlines data processing and analysis. This efficiency reduces human error and accelerates the forecasting timeline. By harnessing technology, DOS not only improves the accuracy of forecasts but also ensures that their operations are efficient and responsive to the needs of the economy.
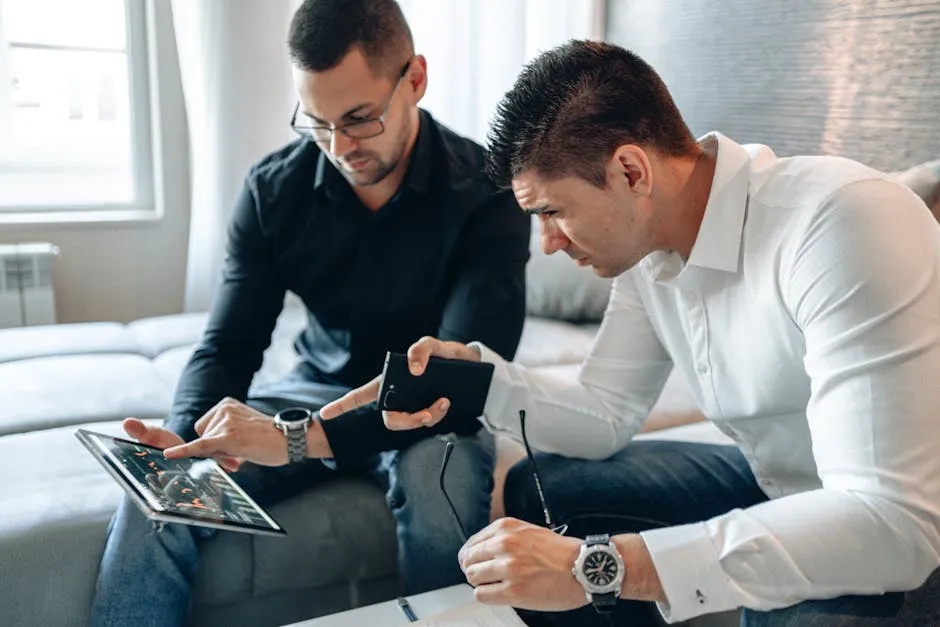
Future Trends in Forecasting Methodologies
Emerging Technologies
As we look ahead, emerging technologies are set to revolutionize forecasting methodologies. Artificial intelligence and big data analytics will play pivotal roles in shaping the future of forecasting. These technologies offer unprecedented capabilities, enabling analysts to harness vast amounts of data for more accurate predictions.
AI algorithms can continuously learn from new data. This learning process allows for the refinement of forecasting models over time. By analyzing historical patterns and real-time data, AI can identify trends and anomalies that traditional methods might overlook. In Singapore, this means that the Department of Statistics can produce forecasts that are not only timely but also highly relevant to current economic conditions.
Moreover, big data analytics allows for the integration of diverse datasets. By combining various data sources—such as social media trends, economic indicators, and consumer behavior—forecasting becomes more holistic. This comprehensive view enables organizations to make informed decisions that align with evolving market dynamics. If you want to explore the future of data-driven organizations, consider reading The Future of Data: A Roadmap for the Data-Driven Organization by David H. Smith.
Policy Implications
Advancements in forecasting methodologies have significant implications for policy-making and resource allocation in Singapore. Accurate forecasts provide essential insights for government agencies, helping them devise effective policies that address economic challenges.
For example, by utilizing refined forecasting models, policymakers can better anticipate economic downturns or growth opportunities. This foresight allows for proactive measures to stimulate the economy or support vulnerable sectors. Additionally, accurate forecasts help allocate resources effectively, ensuring that funding and support reach those who need it most.
Furthermore, as forecasting methodologies evolve, they will increasingly influence strategic planning across various sectors. Businesses can leverage these insights to align their strategies with predicted market trends, enhancing competitiveness. In this way, the continuous improvement of forecasting methodologies will not only benefit the Department of Statistics but also the broader Singaporean economy, fostering resilience and growth in an ever-changing landscape.
FAQs
What is the significance of forecasting in economic planning?
Forecasting is crucial for effective economic planning. Think of it as a GPS for policymakers. Without accurate forecasts, decision-makers would be like drivers without directions—lost and unsure of their next move. Accurate forecasts help governments anticipate economic trends, enabling timely and informed policy-making. This foresight can lead to economic stability and growth.
How does the Department of Statistics Singapore ensure data accuracy?
The Department of Statistics Singapore (DOS) employs rigorous processes to maintain data accuracy. It all starts with robust data collection methods. They gather data from diverse sources, including surveys, administrative records, and sensors, ensuring a comprehensive data set.
What role does machine learning play in forecasting?
Machine learning is a game changer in forecasting methodologies. It enhances traditional methods by analyzing vast datasets for patterns that might be overlooked. Instead of relying solely on historical trends, machine learning algorithms can identify subtle shifts in data, providing businesses and policymakers with sharper insights.
Are there specific industries that benefit the most from these forecasting methodologies?
Absolutely! Various sectors reap the rewards of sophisticated forecasting methodologies. The finance industry, for example, relies heavily on accurate forecasts to manage risks and inform investment strategies.
How can businesses utilize these forecasting methodologies?
Businesses looking to implement forecasting methodologies can start by identifying key performance indicators (KPIs). For instance, a retail company might focus on sales trends, customer behavior, and seasonal patterns.
Conclusion
In summary, the methodologies used by the Department of Statistics Singapore (DOS) combine traditional statistical techniques with modern machine learning approaches. This fusion creates a powerful toolkit for forecasting economic trends, which is crucial for informed policymaking.
These methodologies extend beyond mere number crunching; they influence the lives of Singaporeans daily. For instance, accurate GDP predictions can impact employment rates and national budgets. A well-forecasted economy means better planning for education, healthcare, and infrastructure. So, when DOS puts out their forecasts, they’re essentially shaping the future of the nation.
The power of these methodologies lies in their adaptability. As the global economy evolves, so too do the tools and techniques used to analyze it. For example, DOS is increasingly integrating machine learning into their forecasts. This not only enhances accuracy but also provides deeper insights into complex economic behaviors. Think of it like upgrading from a flip phone to the latest smartphone—suddenly, you have access to apps that make life easier and decisions smarter.
Moreover, the continuous refinement of these methods means that Singapore is not just keeping pace with global trends; it’s often leading the charge. The insights generated from these methodologies can help businesses anticipate market shifts, allowing them to stay ahead—like a savvy shopper who knows when to buy on sale. If you’re interested in further enhancing your understanding of data science, check out Data Science for Dummies by Judith S. Hurwitz.
As we look ahead, we can expect even more innovation from the Department of Statistics. Emerging technologies like artificial intelligence and big data analytics will likely play an increasingly significant role. This evolution promises to enhance forecasting capabilities, providing policymakers and businesses with the tools they need to thrive in a rapidly changing world.
In essence, the methodologies of the Department of Statistics Singapore do more than forecast trends; they provide a roadmap for the nation’s future. With each statistical model and machine learning algorithm, DOS is crafting a narrative that informs and empowers decision-makers. As these methodologies evolve, so too will Singapore’s ability to navigate the complexities of a globalized economy, ensuring a bright future for all its citizens.
Please let us know what you think about our content by leaving a comment down below!
Thank you for reading till here 🙂
All images from Pexels