Introduction
In the intricate world of statistics, numbers can tell stories, but sometimes those stories are misleading. Just like how a magician distracts you with one hand while performing tricks with the other, statistics can deceive if not interpreted correctly. From cherry-picking data to confusing correlation with causation, fallacies in statistics are more common than you might think.
Imagine you’re at a party and someone claims that eating cake causes happiness because every time they eat cake, they feel great. Sounds deliciously convincing, right? But hold your forks! This is a classic case of confusing correlation with causation. Just because two things occur together doesn’t mean one causes the other.
Speaking of cake, if you want to indulge guilt-free, why not try a Instant Pot Duo 7-in-1 Electric Pressure Cooker? You can whip up healthy meals in no time, leaving more room for cake and happiness!
Let’s not forget about cherry-picking data. It’s like a kid choosing only the best candies from a mixed bag while ignoring the yucky ones. In statistics, this approach can create a false narrative, leading to skewed conclusions.
Statistics can also be manipulated in ways that might make even the sharpest minds scratch their heads. Texas Sharpshooter bias is a prime example of this. It occurs when someone takes a large set of data but focuses only on the parts that support their argument, conveniently ignoring everything else.
This article exposes these statistical fallacies, helping you navigate the murky waters of data interpretation. By understanding these pitfalls, you’ll be better equipped to critically analyze statistics, avoiding the traps that often ensnare even the most discerning individuals. So, grab your statistical magnifying glass and let’s illuminate the dark corners of data interpretation, ensuring that you don’t fall victim to these statistical tricks.
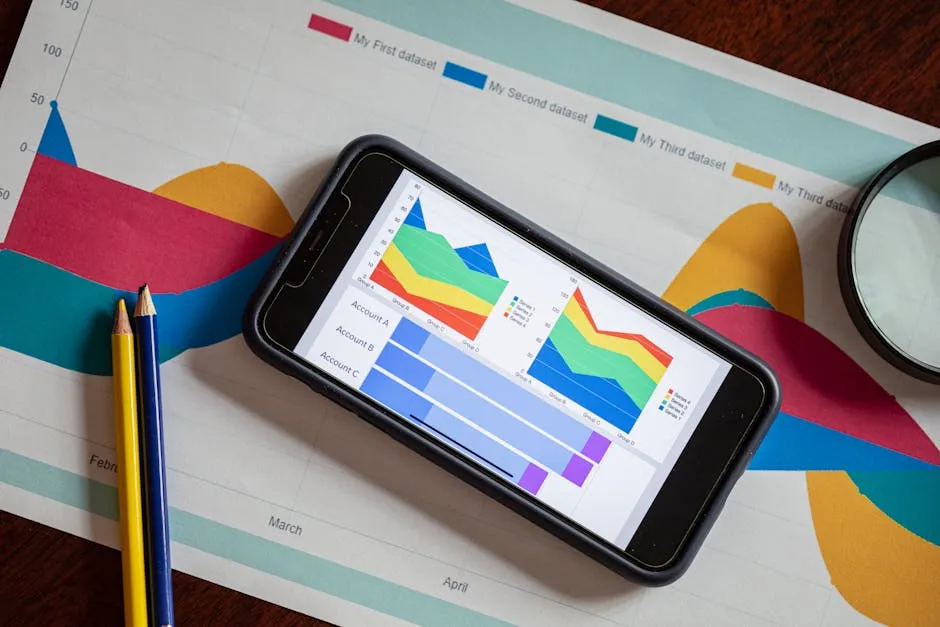
Understanding Statistical Fallacies
Definition of Statistical Fallacies
Statistical fallacies are errors in reasoning that arise from misinterpreting statistical data. They can distort the truth and lead to misguided conclusions. Recognizing these fallacies is essential for accurate data analysis. When we misinterpret data, the implications can be severe, particularly in crucial fields like healthcare, finance, and public policy. Misleading statistics can lead to poor decisions, affecting lives and resources.
Awareness of statistical fallacies is vital. By identifying these pitfalls, we can better analyze data, make informed decisions, and communicate findings effectively. After all, understanding statistics is not just about crunching numbers—it’s about grasping their real-world impact.
Common Types of Statistical Fallacies
1. Correlation vs. Causation
This fallacy occurs when people assume that because two variables correlate, one must cause the other. For example, studies often show a correlation between ice cream sales and drowning incidents. Does that mean ice cream causes drowning? Nope! Both rise in summer, but one doesn’t trigger the other. Media loves this fallacy, frequently misreporting findings that suggest causation where none exists.

2. Cherry Picking
Cherry picking involves selecting data that supports a specific conclusion while ignoring data that contradicts it. Marketers often engage in this, showcasing only the best customer testimonials for advertising. If a company claims their product has a 90% satisfaction rate, but they only cite glowing reviews and ignore negative ones, they’re cherry-picking. This misrepresentation can skew public perception and lead to misguided trust.
3. Texas Sharpshooter Fallacy
The Texas Sharpshooter fallacy occurs when someone highlights a specific subset of data while ignoring the broader context. Imagine a shooter who fires randomly at a barn and then paints a target around the bullet holes to imply accuracy. This fallacy misrepresents data by focusing on patterns that don’t truly reflect reality. Historical examples include studies that misrepresent health benefits by only emphasizing positive outcomes.
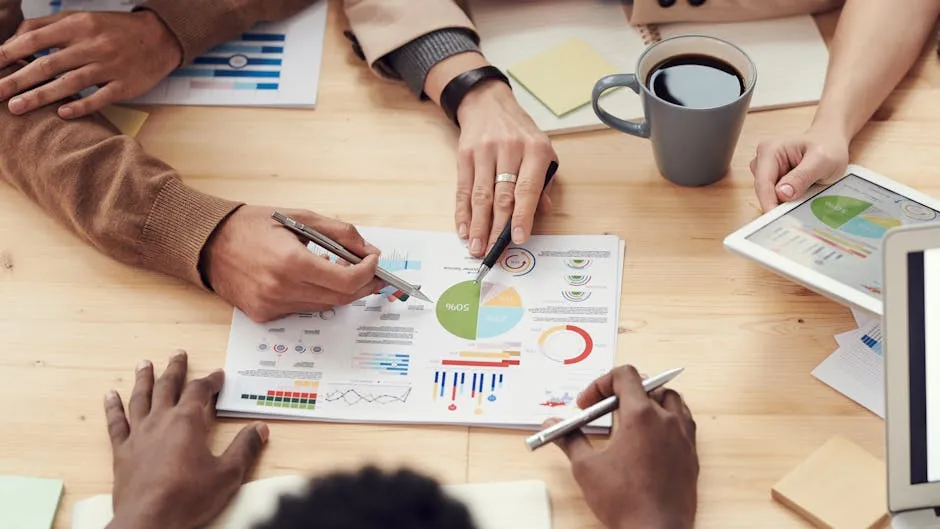
4. Gambler’s Fallacy
The Gambler’s Fallacy is the belief that past random events influence future outcomes. For instance, if a coin lands heads ten times, one might think tails is “due.” In reality, each flip is independent. This fallacy can lead to poor decisions in gambling and finance, where individuals may bet against statistical odds based on previous outcomes.
5. Survivorship Bias
Survivorship bias happens when we focus on successful outcomes while ignoring those that didn’t make it. For example, in business, many only study successful startups, overlooking the countless failed ones. This bias leads to skewed perceptions of success. Investment strategies often fall victim to this fallacy, with investors unknowingly ignoring failures that would have informed smarter decisions.

Understanding these common fallacies helps us navigate the complex world of statistics. By being aware of these pitfalls, we can make better decisions, ensuring our interpretations are grounded in reality rather than misconceptions.
Misuses of Statistical Techniques
1. Data Dredging
Data dredging, also known as “p-hacking,” involves sifting through large datasets to uncover patterns without a defined hypothesis. This practice can lead to spurious correlations that misrepresent the underlying data. Researchers often search high and low for enticing results, like a treasure hunter with a metal detector, only to find fool’s gold.
Imagine a study on diet and health. A researcher runs numerous tests and finds a correlation between eating kale and improved mood. However, without a hypothesis, this result might be coincidental. The truth is, the joy of kale may stem from the fact that people who eat it often also practice yoga—an entirely different variable! This misinterpretation can mislead future studies and lead to flawed conclusions.

2. Sampling Bias
Sampling bias occurs when a sample does not accurately represent the population from which it is drawn. Picture a poll only surveying people at a coffee shop in Beverly Hills. The results will hardly reflect the opinions of everyone in the country.
There are several types of sampling bias, such as self-selection bias, where individuals choose to participate, leading to skewed results. For instance, a survey on weight loss methods might attract only individuals who have succeeded, neglecting those who failed. To combat this, researchers should aim for representative samples that mirror the broader population. Only then can we trust the findings to hold true for the entire group.

3. Misreporting P-Values
P-values are statistical measures that help determine the significance of results in hypothesis testing. However, they often get misinterpreted. A p-value of 0.05 is commonly accepted as the threshold for significance. Yet, many believe it means the results are 100% reliable. Spoiler alert: it doesn’t!
Misunderstanding p-values can lead to overconfidence in findings. Let’s say a study claims a new drug is effective with a p-value of 0.04. Researchers might jump to conclusions, but this doesn’t account for potential errors. Misreporting can inflate the perceived accuracy of results, leading to misguided policies or medical practices.
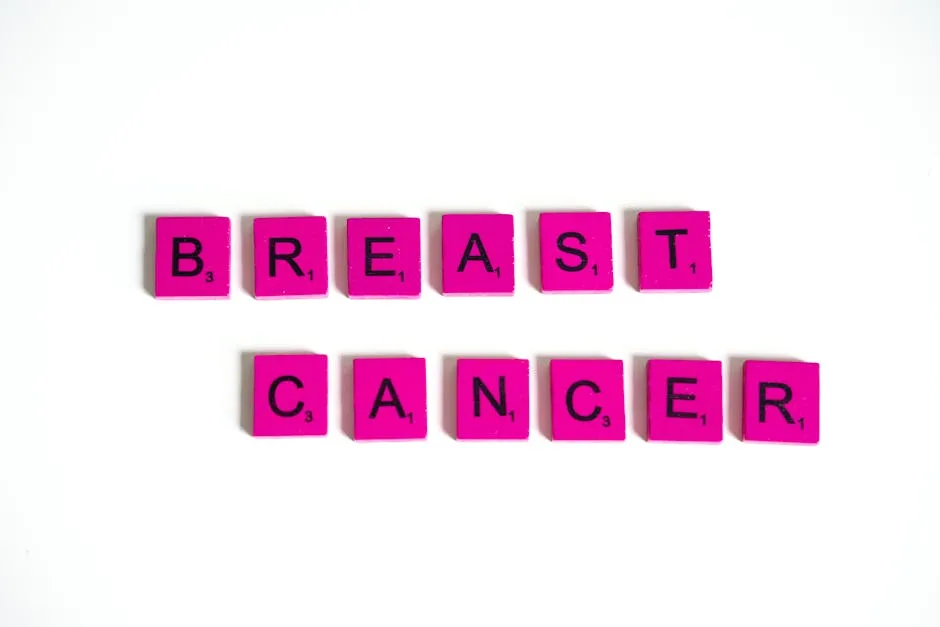
4. Overgeneralization
Overgeneralization happens when conclusions drawn from a specific sample are applied broadly. Imagine studying a group of college students and proclaiming that all young adults have trouble concentrating. That’s a leap worthy of a superhero!
This fallacy can have serious implications in public health statistics. For example, if a study finds that a certain community experiences high rates of diabetes, one might wrongly conclude that the entire population shares the same risk. Ignoring nuances can lead to poor health strategies and wasted resources. Researchers must be cautious and consider the context and limitations of their findings before making sweeping statements.
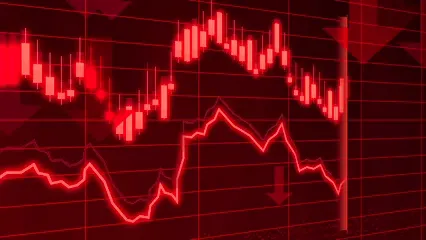
5. Confusing Statistical with Practical Significance
Statistical significance doesn’t always equate to practical significance. A study may yield statistically significant results, but the real-world impact could be negligible. Consider a new medication that lowers blood pressure by 1 mmHg. Statistically significant? Yes! Practically beneficial? Not so much.
This confusion can impact policy-making. Policymakers might enact changes based on statistically significant findings without considering their practical implications. For example, a new law might be based on minor improvements in educational testing without addressing underlying systemic issues. Understanding the difference between statistical and practical significance is crucial for making informed decisions that genuinely improve people’s lives.
Recognizing the distinction between practical significance versus statistical significance is essential for informed decision-making.
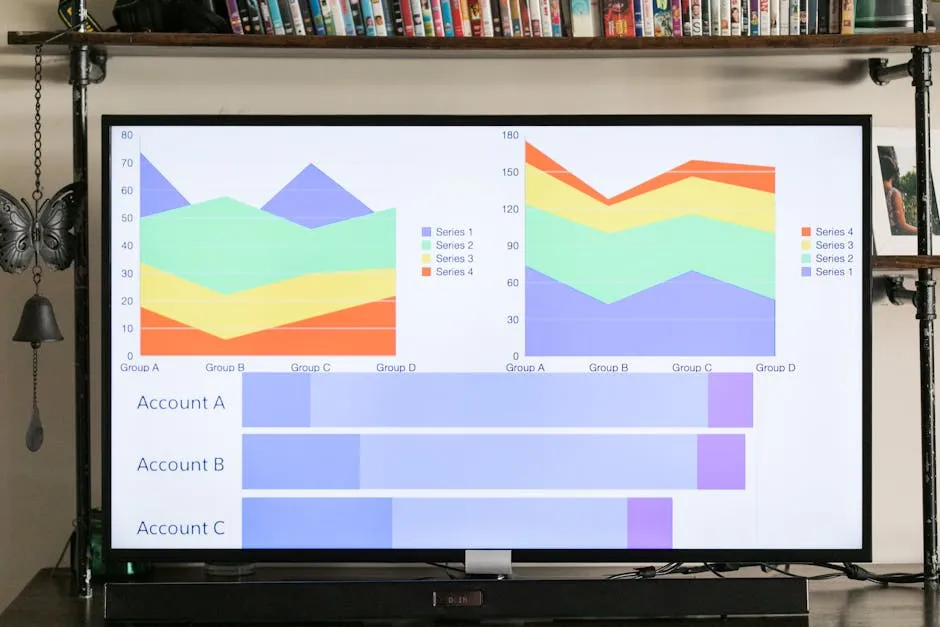
By recognizing these misuses in statistical techniques, we can improve the quality of data interpretation and ensure that conclusions drawn from research are accurate and meaningful. After all, numbers are only as good as the methods used to analyze them. And speaking of good methods, if you want to dive deeper into data analysis, consider checking out R Cookbook: Proven Recipes for Data Analysis. It’s packed with practical recipes to make your data cooking a breeze!

The Role of Statistical Literacy
Importance of Statistical Literacy
Understanding statistics is crucial in our data-driven world. Imagine making decisions based on faulty data—yikes! Statistical literacy helps us navigate this minefield. It’s like having a GPS for your data journey, guiding you through twists and turns while avoiding pitfalls.
Educating ourselves about statistics empowers us to interpret data accurately. With a solid foundation in statistical concepts, we can spot fallacies and misinterpretations. When we recognize these errors, we can make more informed choices. This awareness is especially vital in fields like healthcare, finance, and public policy, where the consequences of misinterpretation can be dire.
Schools and universities play a big role in fostering statistical literacy. By incorporating statistics into curricula, we can equip future generations with the skills needed to analyze data critically. We need to teach students not just to crunch numbers but to understand what those numbers really mean. After all, a well-educated public is our best defense against misleading statistics. And if you’re looking for a great resource to start with, check out Statistics for Dummies. It’s both enlightening and entertaining!

Strategies to Improve Statistical Literacy
Improving statistical literacy doesn’t have to be a chore. There are plenty of resources available to make learning fun and engaging. Online courses, webinars, and workshops can provide valuable insights into statistical concepts. Websites like Khan Academy and Coursera offer free courses that break down complex ideas into bite-sized chunks.
Books are another excellent resource. Classics like “How to Lie with Statistics” by Darrell Huff offer witty insights into the misuses of statistics. These reads are eye-opening and entertaining—who said learning can’t be fun? You can grab your copy here!
In addition to formal resources, critical thinking tools can enhance our ability to analyze data. Familiarize yourself with statistical software like R or Python. These tools can help you visualize data and identify trends. Learning to ask the right questions is essential. Instead of taking data at face value, dig deeper. What assumptions are being made? What data is excluded?
Utilize visual aids as well. Graphs and charts can make data more accessible. However, be wary of misleading visuals! Understanding how to interpret these graphics is key. Always consider the source of the data and its context.
By actively engaging with statistics and seeking out resources, we can build our statistical literacy. This investment pays off by equipping us to make better decisions in our personal and professional lives. And if you’re looking for a fun way to keep track of your notes, you might want to grab a Moleskine Classic Notebook to jot down your thoughts!

Mitigating Fallacies in Practice
Best Practices for Data Analysis
When it comes to data analysis, methodology is everything. A well-designed research process lays the foundation for credible results. Start with a clear hypothesis. This way, you can focus your analysis on answering specific questions. Vague goals lead to vague findings—no one wants that!
Peer review is another essential practice. Collaborating with statisticians or data analysts can catch errors before they spiral out of control. Consider it a safety net for your research. Just like having a buddy when you try something new, having someone else review your work can provide valuable perspectives.
Statisticians play a vital role in ensuring data integrity. Their expertise can guide researchers in selecting appropriate statistical methods and interpreting results accurately. When statisticians and researchers collaborate, it minimizes the chances of falling into statistical traps.
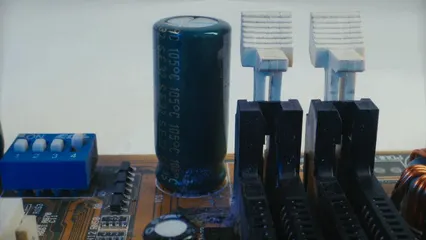
Case Studies
Examining real-life cases can shed light on the impact of statistical fallacies. One notable instance is the infamous Lancet study on vaccines and autism. The study caused widespread panic, leading to a decline in vaccination rates. The research was later retracted due to flawed methodology and data manipulation. Correct interpretation could have saved countless lives and preserved public trust in vaccines.
Another case involves financial forecasting. During the 2008 financial crisis, many analysts relied on faulty statistical models, leading to disastrous consequences. A careful review of data and methodologies could have highlighted the underlying risks. By learning from these examples, we can understand the importance of rigorous analysis.
In conclusion, improving statistical literacy and adhering to best practices can significantly reduce the risk of fallacies. By fostering a culture of critical thinking and collaboration, we empower ourselves and others to navigate the complex world of statistics with confidence. The consequences of our decisions may very well depend on it.

Conclusion
Understanding fallacies in statistics is vital for anyone who engages with data. In an era where information flows like a river, it’s easy to get swept away by misleading numbers. Just as a good detective scrutinizes every clue, we must examine statistical claims closely. Numbers can lie if not analyzed properly, leading us down a rabbit hole of confusion.
By recognizing common statistical pitfalls, you can sharpen your critical thinking skills. This knowledge empowers you to make informed decisions and interpret statistical information accurately. Whether you’re a business professional, a student, or simply someone who enjoys data, being aware of these fallacies is essential. And if you want to dive deeper into the art of statistics, don’t miss out on The Art of Statistics: Learning from Data by David Spiegelhalter. It’s a must-read for anyone serious about understanding data!
Imagine you’re trying to decide between two job offers. One company boasts about its high employee satisfaction rate. However, if that rate is based on selective data, you might be walking into a misleading situation. Similarly, in healthcare, a study may tout the effectiveness of a new drug without mentioning significant side effects. Understanding the statistics behind these claims can save you from making poor choices.
As we navigate this data-driven world, always remember to question and analyze. Are the data sources reliable? Is the sample size adequate? Are there potential biases at play? By equipping yourself with the tools to scrutinize statistical claims, you gain a more informed perspective on the world around you.
In conclusion, enhancing your statistical literacy is not just an academic exercise. It’s a crucial life skill. So, the next time you encounter a dazzling statistic, don’t just take it at face value. Investigate! Your ability to critically analyze data can lead to better decision-making, ensuring you don’t fall victim to the charming yet treacherous world of statistical fallacies.
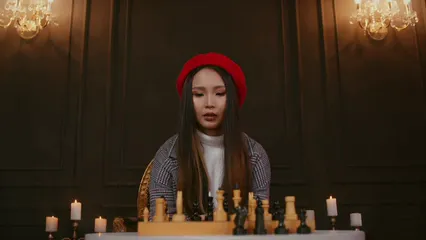
FAQs
What is a statistical fallacy?
A statistical fallacy is an error in reasoning that leads to incorrect conclusions based on statistical data. For instance, claiming that a high rate of ice cream sales causes an increase in drowning incidents is a classic fallacy. In reality, both rise during summer, but one does not cause the other.
How can I identify fallacies in statistics?
To identify fallacies, always scrutinize the data source and look for potential biases. Check if the sample size is appropriate and if any important variables are overlooked. Asking questions about how the data was collected can help uncover misleading interpretations.
Why is understanding statistics important?
Statistics play a crucial role in everyday decision-making. From health choices to financial investments, understanding statistics helps you interpret information correctly. This knowledge can prevent costly mistakes and guide you toward better outcomes.
What are some resources for learning about statistics?
There are many great resources available! Books like ‘How to Lie with Statistics’ by Darrell Huff offer entertaining insights. Online platforms like Khan Academy and Coursera provide free courses on statistics, making learning accessible and enjoyable.
Can statistical fallacies be avoided entirely?
While it may be challenging to avoid all statistical fallacies, rigorous methodology and peer review can significantly reduce their occurrence. Engaging with statisticians and experts in data analysis can help ensure that results are interpreted correctly and responsibly.
Please let us know what you think about our content by leaving a comment down below!
Thank you for reading till here 🙂
All images from Pexels