Introduction
Data annotation might sound like a techie buzzword, but it’s the secret sauce behind the magic of machine learning. In a world where artificial intelligence (AI) is increasingly becoming part of our daily lives, data annotation serves as the unsung hero, meticulously labeling the data that trains AI models to recognize patterns, make decisions, and ultimately, learn. Whether it’s tagging images for self-driving cars or annotating text for chatbots, data annotation is everywhere.
Imagine teaching a child to recognize a cat. You point at a furry creature and say, “This is a cat.” That process of labeling is what data annotation does for AI. It provides context and clarity, allowing algorithms to understand the intricacies of data. High-quality annotated data fuels AI systems, ensuring they can learn effectively and make sense of their surroundings.
The importance of data annotation has surged in recent years, particularly as businesses ramp up their AI initiatives. Companies now rely on vast amounts of labeled data to train sophisticated models that can perform tasks ranging from image recognition to natural language processing. The demand for skilled annotators is skyrocketing, turning this into a lucrative field.
Data annotation isn’t just for data scientists anymore. Many platforms offer flexible opportunities for anyone interested in entering the field. Imagine earning money while helping AI become smarter—sounds like a win-win, right?
This guide will unravel the intricacies of data annotation, its types, importance, tools, and how you can get involved in this lucrative field. If you’ve ever wondered how AI systems learn or what goes into making a chatbot feel human-like, stick around. By the end, you’ll have a solid grasp of data annotation and its pivotal role in shaping the future of technology.
Get ready to explore the fascinating world of data annotation, where every label counts, and where your attention to detail could help change the landscape of AI as we know it. Let’s jump in!

Tools and Technologies for Data Annotation
Data annotation tools are essential in our data-driven world. They vary from simple manual annotation software to sophisticated AI-powered platforms. The right tool can make all the difference in accuracy and efficiency. For those looking to dive deeper into the world of data annotation, consider checking out the “Data Annotation for Dummies” Book. It’s a great starting point for understanding the fundamentals!
Manual Annotation Tools
Manual annotation tools allow users to label data manually. They often feature user-friendly interfaces, making them accessible for beginners. Popular options include:
- LabelImg: Great for image annotation with bounding boxes. If you’re diving into this tool, consider enhancing your experience with High-Quality Headphones for Focus to block out distractions!
- VGG Image Annotator (VIA): A versatile tool for various annotation types.
- LabelMe: An online platform for image labeling and segmentation.
These tools are ideal for small projects or when high precision is required. Manual annotation ensures that human judgment prevails, particularly in nuanced tasks.
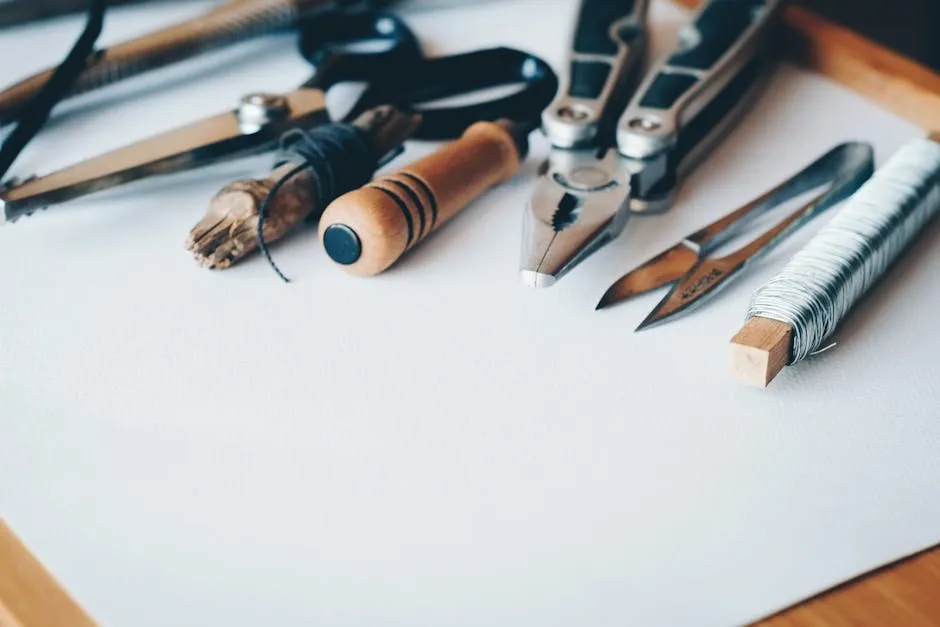
Automated Annotation Tools
With the rise of AI, automated annotation tools have become game-changers. They utilize machine learning algorithms to speed up the labeling process. Some noteworthy platforms include:
- Scale AI: This platform combines human intelligence and AI to improve annotation efficiency.
- SuperAnnotate: It offers various annotation services and integrates automation to enhance productivity.
Automated tools can handle large datasets quickly, but they may require human oversight to maintain quality. If you’re interested in learning more about machine learning, check out the Udemy Course on Machine Learning!

Comparative Analysis
When choosing between manual and automated tools, consider factors like efficiency, accuracy, and cost.
- Efficiency: Automated tools are faster and can manage large volumes of data. Manual tools may slow down the process but often yield higher accuracy for complex tasks.
- Accuracy: Human annotation is generally more precise in nuanced contexts, while automated tools may struggle with subtleties.
- Cost: Manual annotation can be less expensive for small-scale projects, but costs can escalate with larger datasets. Automated tools often have higher upfront costs but can save money in the long run.
In the end, the choice depends on specific project requirements, available resources, and the desired balance between speed and accuracy. If you’re looking for ways to enhance productivity as you work, consider a Productivity Planner to keep your tasks organized!

Getting Started in Data Annotation
Embarking on a data annotation career can be exciting and rewarding. Here are some tips for beginners looking to enter this field.
Educational Resources
Start by educating yourself. Numerous online courses and tutorials cover data annotation fundamentals. Platforms like Coursera and Udemy offer courses tailored to beginners. If you want a comprehensive understanding of the subject, consider grabbing a copy of AI and Machine Learning Books for a deeper dive!

Practical Experience
Hands-on experience is invaluable. Try out annotation platforms like LabelMe or MakeSense.ai. These sites provide an opportunity to practice and hone your skills. Engaging in competitions on platforms like Kaggle can also give you practical experience while building a portfolio. Speaking of competitions, why not check out the Kaggle Data Science Competitions to challenge yourself!
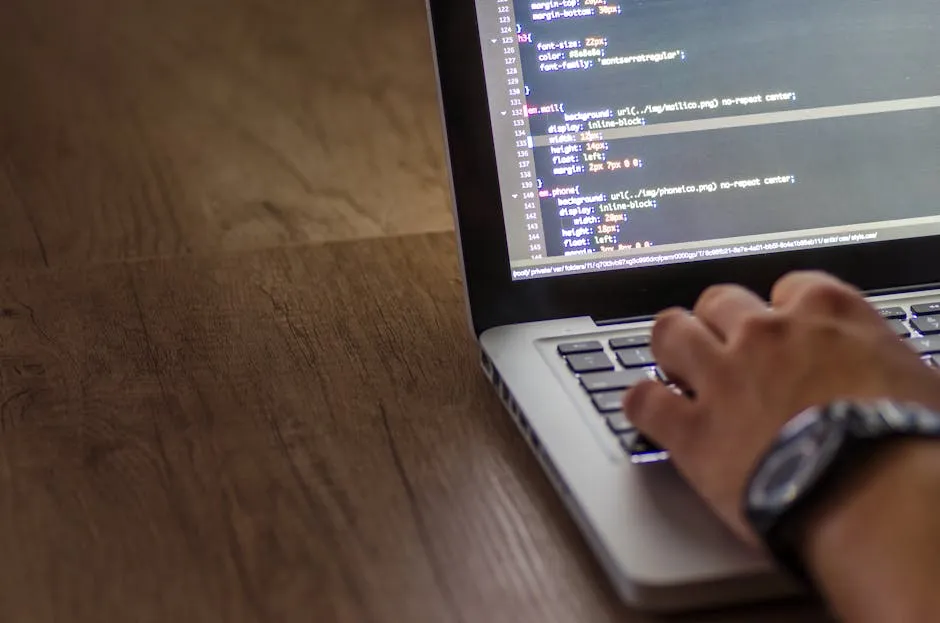
Career Opportunities
The demand for data annotators is booming. Companies are constantly seeking skilled professionals to annotate their datasets. Potential career paths include roles in AI companies, research institutions, and tech firms focused on machine learning. If you’re looking to prepare for your job search, consider a Professional Notebook for Notes to keep track of your journey!
Building a solid foundation in data annotation can open doors to exciting opportunities in the tech industry.

Future of Data Annotation
As AI technology continues to advance, the demand for quality annotated data is set to soar. The future of data annotation looks bright and filled with opportunities.
Growth in Demand
With the increasing reliance on AI across various sectors, high-quality annotated data will become essential. The need for precise labeling will grow as machine learning models become more complex. This trend indicates a bright future for data annotation professionals. To stay ahead, consider exploring Coursera Course on Data Annotation to sharpen your skills!
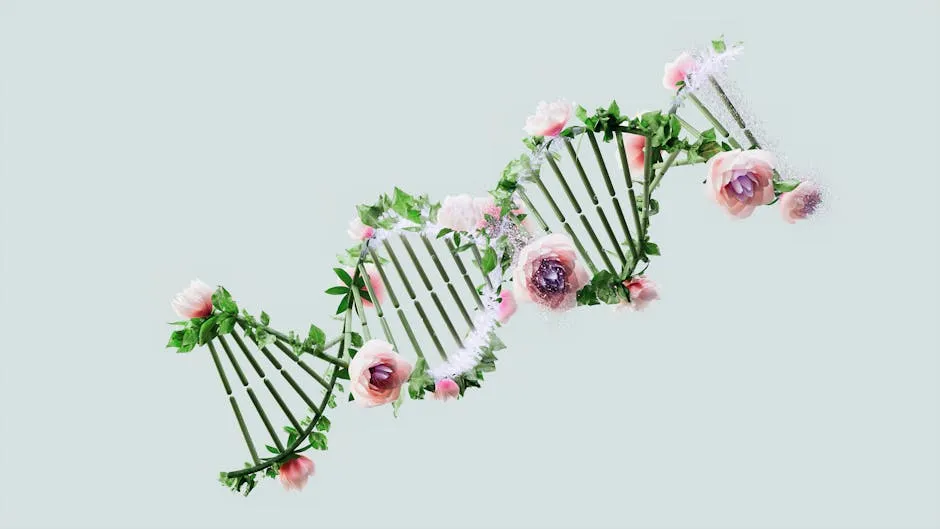
Innovations in Tools
As technology evolves, we can expect more innovative tools to streamline the annotation process. AI will likely play a significant role in enhancing the efficiency of data annotation. These advancements will make it easier for companies to manage large datasets while maintaining quality.
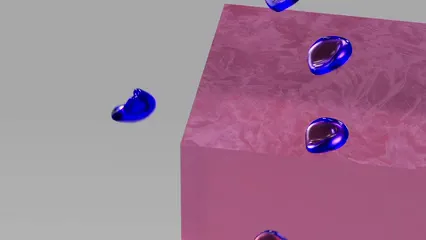
Challenges in Data Annotation
Scale and Complexity
Managing large datasets presents a formidable challenge. As businesses increasingly rely on data for AI, the volume of data continues to swell. Think of it like a never-ending buffet: the more you pile on your plate, the harder it is to balance!
First, consider the sheer size. Large datasets can contain millions of entries. Annotating each piece of data meticulously is no small feat. It requires a solid plan and a dedicated team. Without efficient systems in place, the process can drag on longer than a Monday morning meeting.
Then, there’s complexity. Data comes in various forms: images, text, audio, and video. Each type has unique requirements. For instance, annotating images involves identifying and labeling objects, while text annotation might focus on sentiment or intent. Juggling these diverse tasks can lead to errors and inconsistencies.
Moreover, the need for high-quality annotations increases with data complexity. Algorithms learn from data, and any inaccuracies can mislead them. This can result in poor model performance. It’s like teaching a child incorrect facts; they’ll end up confused!
To tackle these challenges, teams often employ a mix of manual and automated tools. Automation can speed up the process, but human oversight remains crucial for ensuring accuracy. A well-structured workflow is essential to keep things running smoothly.

Subjectivity and Consistency
Subjectivity in data annotation can be a double-edged sword. On one hand, it allows for nuanced understanding; on the other, it can create chaos. When multiple annotators interpret data differently, inconsistencies arise.
Take sentiment analysis, for example. One annotator may label a review as positive, while another sees it as neutral. Such differences can skew the results, leading to unreliable AI models. It’s like playing a game of telephone—what started as a clear message can quickly become garbled.
To combat this, establishing clear guidelines is vital. Annotators need a solid understanding of the criteria for labeling. Regular training and calibration sessions can help keep everyone on the same page. Think of it as a team huddle before the big game!
Quality control measures also play a role. Random checks of annotated data can help identify inconsistencies early. This proactive approach ensures that the final dataset is reliable. Remember, a consistent dataset is key to training effective models.

Balancing Cost and Quality
Achieving a balance between cost and quality in data annotation can feel like walking a tightrope. Organizations want high-quality annotations, but they also face budget constraints.
Manual annotation offers precision but can be labor-intensive and expensive. The more complex the task, the higher the associated costs. On the flip side, automated tools can cut costs significantly. However, they may struggle with nuanced tasks. Imagine an AI trying to understand sarcasm—it’s not always a smooth ride!
Finding the sweet spot requires strategic planning. Organizations often start with manual annotation for complex tasks, then switch to automated tools for more straightforward data. This hybrid approach allows for cost savings while maintaining quality.
Additionally, leveraging crowd-sourcing platforms can provide flexibility. They allow organizations to scale their annotation workforce as needed. This way, businesses can adapt to changing workloads without breaking the bank.
In summary, navigating the challenges in data annotation involves addressing scale, subjectivity, and cost. By implementing structured workflows, training, and hybrid approaches, organizations can enhance the quality of their annotated data while keeping costs manageable. To stay organized while working from home, consider investing in a Ergonomic Office Chair to keep you comfortable during long hours!
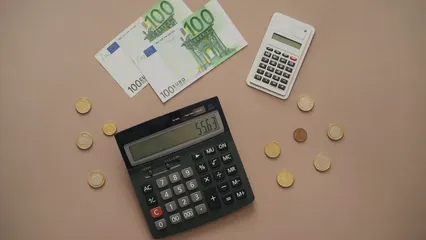
Getting Started with Data Annotation
Educational Resources
Embarking on a career in data annotation? Fantastic! There are plenty of online courses and tutorials to help you get started. Platforms like Coursera, Udemy, and edX offer courses tailored for beginners. These resources cover the fundamentals of data annotation and machine learning principles. If you’re looking for a great read, check out “Artificial Intelligence: A Guide to Intelligent Systems” Book to deepen your understanding!
For instance, you can find courses focused on specific data types, such as image or text annotation. These courses often include hands-on projects, allowing you to practice your skills in real-world scenarios.
YouTube is also a treasure trove of tutorials. Many experts share their insights on data annotation tools and techniques. Watching these videos can provide a practical understanding of the annotation process.
Another excellent resource is online forums and communities. Sites like Reddit and Stack Overflow have dedicated sections for data annotation. Engaging with others in the field can lead to valuable tips and networking opportunities.
Don’t forget about books! There are several comprehensive texts on data annotation and AI that can deepen your understanding. Combining various learning methods will help you grasp the intricacies of this essential field.
So, gear up! With the right educational resources, you’ll be well on your way to becoming a data annotation pro.

Practical Experience
Gaining practical experience in data annotation is essential for anyone looking to thrive in this field. Fortunately, several platforms offer hands-on opportunities to dive into annotation tasks.
Kaggle stands out as a fantastic resource. This platform is well-known for its data science competitions. Participants can engage in real-world projects that require data annotation. You can learn how to label data for machine learning models while competing with others. Plus, you’ll build a portfolio showcasing your skills!
LabelMe is another excellent option. It provides a user-friendly interface for annotating images. With LabelMe, you can practice tagging various elements in pictures. This tool is perfect for beginners looking to hone their skills.
MakeSense.ai offers a free, intuitive platform for annotating images and videos. It’s designed for ease of use, making it ideal for newcomers. You can quickly learn the ropes while working on practical tasks. To enhance your annotation experience further, consider investing in a Digital Drawing Tablet for Annotation to make your work even smoother!
Whether you’re annotating images, text, or audio, these platforms provide valuable experience. They prepare you for the job market by enhancing your practical skills.
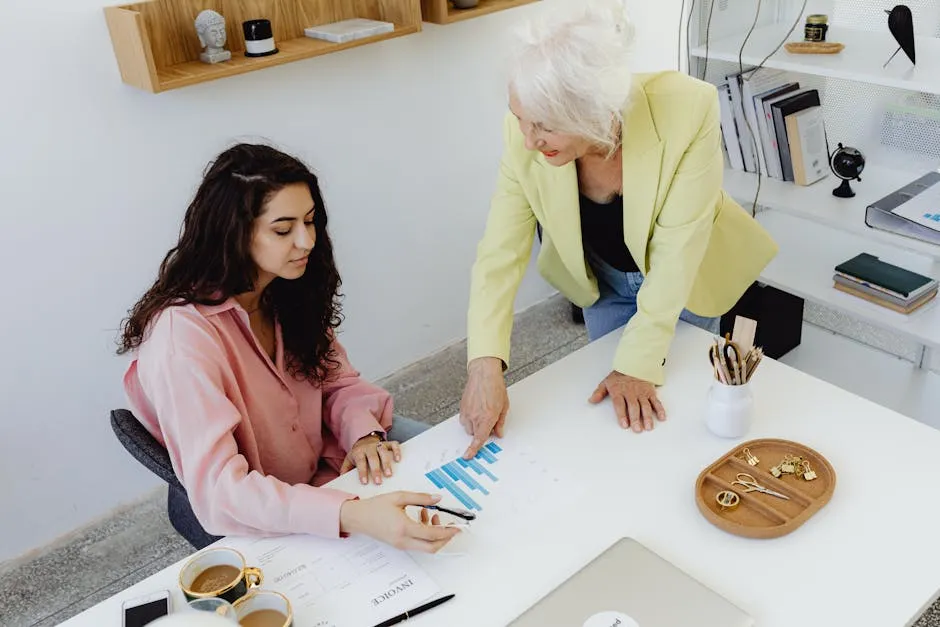
Career Opportunities
The world of data annotation is bursting with career opportunities. As machine learning continues to expand, the demand for skilled annotators is skyrocketing. Here are a few promising career paths in this field.
- Data Annotator: This entry-level role involves labeling data for various machine learning projects. It’s a great starting point for those new to the industry. Annotators often work on diverse datasets, from images to text.
- Quality Assurance Specialist: In this role, you ensure the quality and accuracy of annotated data. You’ll review and refine annotations made by others. Attention to detail is key here, as it directly impacts model performance.
- Project Manager: For those who enjoy organization, project management offers a great opportunity. You’ll oversee data annotation projects, coordinating teams and timelines. Strong leadership skills are essential to ensure smooth operations.
- Data Scientist: With experience in data annotation, you can transition into data science. This role focuses on analyzing data and developing machine learning models. Your background in annotation will provide valuable insights into data quality and preparation.
- Machine Learning Engineer: If you enjoy the technical side of AI, consider becoming a machine learning engineer. This role involves building and optimizing models. Your knowledge of data annotation will help you understand the importance of high-quality training data.
The field of data annotation is thriving, and there are numerous pathways to explore. Investing in your skills can lead to a fulfilling career in this dynamic industry. To stay organized and effective, consider using a Office Desk Organizer that fits your style!
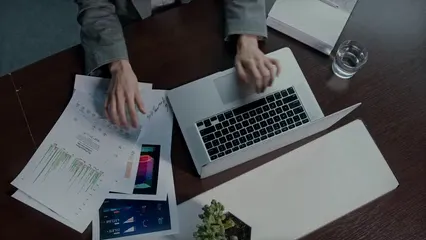
Conclusion
Data annotation is not just a technical task; it’s a crucial step in the journey of machine learning and artificial intelligence. As the AI landscape continues to grow, so does the need for skilled annotators who can provide the high-quality data that models require to thrive. Whether you’re looking to enhance your career prospects or simply curious about the field, understanding data annotation is essential in today’s digital age.
The importance of data annotation cannot be overstated. It serves as the foundation for training algorithms, enabling them to learn from labeled datasets. Imagine teaching a child to recognize fruits. You show them an apple and say, “This is an apple.” Data annotation is like that—it helps machines understand what they are processing.
In various domains—healthcare, autonomous driving, and customer service—data annotation facilitates AI’s ability to make sense of complex information. For instance, in healthcare, accurately annotated medical images can lead to better diagnosis and treatment plans. In self-driving cars, annotated images help vehicles recognize traffic signs and pedestrians.
Furthermore, the rise of data annotation has opened up new job opportunities. Freelance platforms like DataAnnotation.tech and Remotasks offer flexible work options. You can earn money while helping AI become smarter! Many people have found fulfilling careers in this space, often working from home and setting their own hours. To make working from home even more enjoyable, consider investing in a Coffee Maker for Productivity Boost!
As technology continues to evolve, so will the methods and tools for data annotation. Innovations like automated annotation tools are emerging, but human insight remains irreplaceable. Machines still struggle with context, nuance, and subtlety—areas where human annotators excel.
In conclusion, data annotation is a vital cog in the AI machine. It enhances model performance, ensures quality, and drives innovation. Whether you’re a seasoned professional or a curious beginner, there’s a place for you in this exciting field. Understanding the significance of data annotation is not just a career move; it’s an essential part of engaging with the future of technology.
Please let us know what you think about our content by leaving a comment down below!
Thank you for reading till here 🙂
For more insights on how AI models function, check out this article on generative AI models and their statistical nature.
All images from Pexels