Introduction
In a world overflowing with data, statistics reign supreme as the key to unlocking valuable insights. Picture statistics as your trusty GPS, guiding you through the vast ocean of information. Without it, you might drift into confusion, lost among countless numbers and figures. Whether you’re a business leader making critical decisions or a student grappling with academic research, grasping the core concepts of statistics is essential.
Statistics is not just for the mathematically inclined; it’s a universal language that helps us interpret the world. When you see a headline claiming, “90% of people prefer chocolate over vanilla,” you’re witnessing statistics in action. It’s that magical blend of numbers and context that makes sense of our experiences.
From the basics of data collection to the intricate world of statistical analysis, this comprehensive guide aims to illuminate the path ahead. We’ll cover everything from descriptive statistics—think averages and trends—to inferential statistics, where we make predictions about larger populations based on samples.
Statistics is critical in various fields, including healthcare, finance, and education. For example, clinical trials rely heavily on statistical methods to determine the effectiveness of new drugs. This means that behind every medical breakthrough, there’s a statistician ensuring the data is sound.
But wait, there’s more! Beyond the technical aspects, statistics also holds a certain charm. It allows us to uncover stories hidden within data. Did you know that the average person will spend about six months of their life waiting for red lights to turn green? Now, that’s a statistic that puts life into perspective!
So, buckle up as we embark on this enlightening journey. By the end of this guide, you’ll see statistics not as a daunting subject, but as a powerful tool that shapes our understanding of the world. Let’s navigate through the fascinating realm of statistics together and discover how it can unlock insights that matter.
If you’re looking to enhance your data analysis skills, consider getting a book on Statistics for Data Science. It’s a fantastic resource for beginners and experts alike, providing insights that can help you make data-driven decisions.
Summary of Key Points
Statistics is not just a collection of numbers; it’s a powerful tool that helps us interpret the data around us. In this blog post, we will cover:
- The fundamental concepts of statistics, including descriptive and inferential statistics.
- Essential methods for data collection, including sampling techniques and experimental designs.
- An exploration of probability, highlighting its role in statistical inference and various probability distributions.
- The importance of hypothesis testing and the different statistical tests available.
- Real-world applications of statistics across various domains, from healthcare to business.
- Common pitfalls in statistical analysis and how to avoid them.
Curious about how these concepts play out in real life? We’ll also share compelling examples and case studies that illustrate the power of statistics in action. Let’s embark on this enlightening journey!
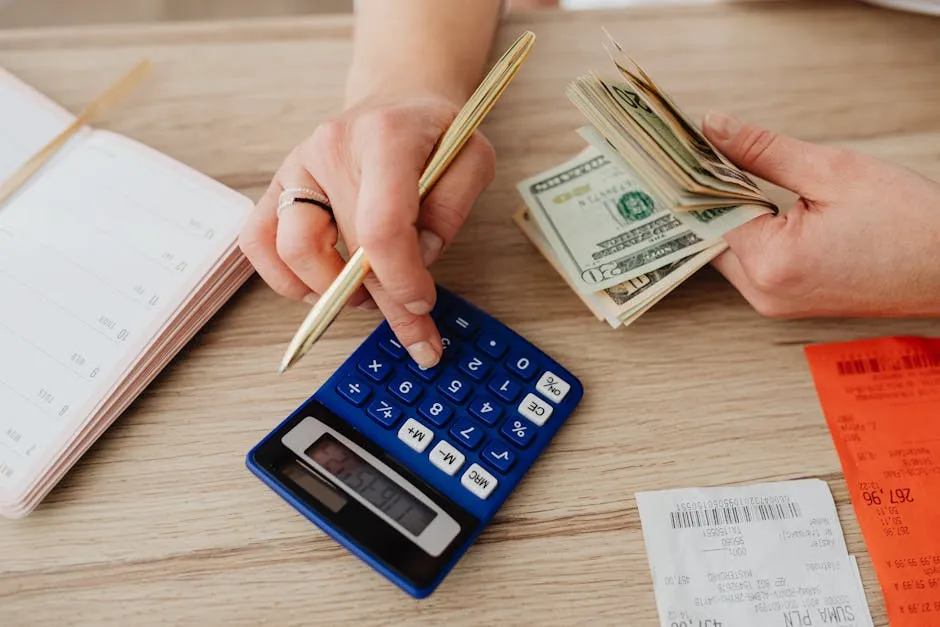
Understanding Statistics
What is Statistics?
Statistics is the science of collecting, organizing, analyzing, interpreting, and presenting data. Imagine it as the translator between raw numbers and meaningful insights! Statistics helps us make sense of the chaos in our data-driven world. Without it, we’d be lost in a sea of numbers, scratching our heads and wondering what it all means.
At its core, statistics is essential for understanding trends, making predictions, and informing decisions. It begins with a statistical population or model to be studied. This could range from “all people living in a country” to “every atom in a crystal.” By systematically gathering data, statisticians can summarize complex information in ways that are digestible and actionable.
Statistics can be broadly categorized into two types: descriptive and inferential statistics. Descriptive statistics focus on summarizing and describing the features of a dataset. Think averages, percentages, and visualizations like graphs. Inferential statistics, on the other hand, goes a step further. It allows us to make predictions or generalizations about a larger population based on a sample. This is where the magic happens—using a small group to infer truths about a bigger one!
The Importance of Statistics
Statistics play a crucial role in decision-making across many fields. From healthcare to business, the ability to analyze data means making informed choices. Imagine a doctor determining treatment options based on statistical outcomes from clinical trials. Or a business leader deciding on market strategies based on customer behavior data. Statistics provide the backbone for these critical decisions.
The power of statistics lies in data-driven decision-making. When organizations rely on data rather than gut feelings, they tend to achieve better outcomes. For instance, a company might analyze consumer trends to optimize its product offerings. They could discover that 70% of customers prefer eco-friendly products. Armed with this knowledge, they can tailor their marketing strategies accordingly.
Moreover, statistics help in identifying patterns and trends that may not be immediately obvious. Take, for example, a city analyzing traffic accident data. By understanding peak times and locations, city planners can implement changes, reducing accidents and saving lives. In essence, statistics transform data into actionable insights, paving the way for smarter choices.
If you’re serious about data analysis, consider investing in a guide on Statistical Analysis with Excel. It’s an incredibly useful tool for anyone looking to perform data analysis efficiently!
Key Concepts in Statistics
Descriptive statistics are all about summarizing data. They provide measures of central tendency, like the mean, median, and mode. The mean is the average; the median is the middle value in a sorted list; and the mode is the most frequently occurring value. These measures give a quick snapshot of the data at hand.
But wait, there’s more! Descriptive statistics also involve measures of dispersion. This includes the range, variance, and standard deviation. The range tells you the difference between the highest and lowest values, while the standard deviation reveals how spread out the data points are from the mean. Together, these concepts paint a comprehensive picture of the dataset.
On the flip side, inferential statistics delve into sampling, estimation, and hypothesis testing. Sampling involves selecting a representative group from a larger population. This is crucial since collecting data from an entire population can be impractical or impossible. Once a sample is collected, statisticians can make estimations about the population’s characteristics and test hypotheses.
Hypothesis testing is all about making claims. For example, a researcher might want to know if a new drug is more effective than the existing one. They would set up a null hypothesis (the drug isn’t effective) and an alternative hypothesis (the drug is effective). By analyzing the sample data, they can determine whether to reject or accept the null hypothesis, guiding their conclusions based on statistical evidence.
In summary, understanding statistics equips you with the tools to navigate data intelligently. From summarizing datasets to making predictions, statistics is essential for anyone looking to make informed decisions in an increasingly data-centric world.
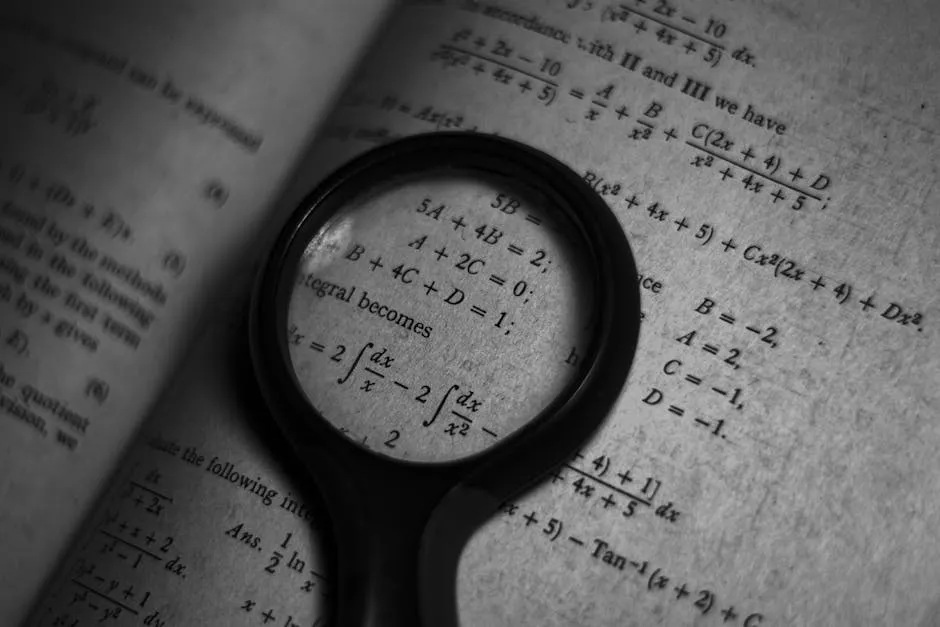
Data Collection Methods
Sampling Techniques
Sampling is the backbone of statistical research. It involves selecting a subset of individuals from a larger population to infer conclusions about that population. Imagine trying to taste-test every flavor at an ice cream shop—overwhelming, right? That’s why we sample!
There are various sampling methods, each with its strengths and weaknesses. Let’s break down a few popular techniques:
- Random Sampling: Everyone has an equal chance of being chosen. This method helps eliminate bias. Think of it as drawing names from a hat. It’s fair and straightforward.
- Stratified Sampling: Here, you divide the population into subgroups, or strata, based on shared characteristics. Then, you randomly sample from each stratum. Want to ensure you have a mix of ages in your ice cream taste test? Stratified sampling can help!
- Systematic Sampling: This technique involves selecting every nth individual from a list. For example, if you pick every 5th person on a roster, you’re using systematic sampling. It’s simple, but you must ensure the list isn’t biased!
- Cluster Sampling: In this method, you divide the population into clusters, often geographically, and randomly select entire clusters for your sample. Think of it like choosing entire neighborhoods for a survey instead of individuals. Learn more about cluster sampling techniques.
Representative sampling is crucial for accurate results. If your sample doesn’t reflect the population, your conclusions could be way off. Imagine conducting a survey on ice cream preferences but only sampling from a winter sports event—would you get accurate insights? Probably not!
Experimental vs. Observational Studies
When collecting data, researchers often choose between experimental and observational studies. Both have their unique flair.
Experimental Studies involve manipulating one variable to see its effect on another. Picture a scientist testing a new ice cream flavor by changing the amount of sugar. They control the variables to isolate the effect of sugar on flavor. This method allows for strong cause-and-effect conclusions.
On the flip side, Observational Studies gather data without manipulation. Researchers observe subjects in their natural environment. Imagine watching people choose their favorite ice cream in a park without interfering. While this method is less controlled, it captures real-life behaviors.
The key difference? Experimental studies aim to establish causation, while observational studies identify correlations. Use experimental designs when you want to test a hypothesis with control. Opt for observational studies when exploring subjects in their natural state.
Data Quality and Bias
Data quality is a non-negotiable aspect of statistical research. Poor quality data can lead to misleading conclusions faster than you can say “chocolate chip cookie dough.” One of the biggest challenges in data collection is bias.
Bias can creep in through various avenues. Selection bias occurs when the sample doesn’t accurately represent the population. For example, surveying only ice cream lovers at a festival might skew your results.
Measurement bias happens when the tools or methods used to collect data are flawed. Imagine if your ice cream thermometer always reads five degrees too high. Your temperature data would be off, leading to incorrect conclusions about how heat affects melting.
To mitigate bias, ensure your sampling methods are robust. Use random sampling when possible, and double-check your data collection tools for accuracy. Transparency in your methods also helps others assess the reliability of your findings.
In summary, maintaining data quality is essential for trustworthy statistics. By employing effective sampling techniques, understanding the differences between study types, and guarding against biases, researchers can collect meaningful data that leads to insightful conclusions. With good data, the ice cream of knowledge becomes even sweeter!
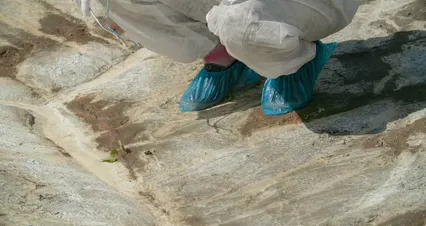
Statistical Analysis Techniques
Statistics is the art of turning numbers into stories! It helps us make sense of our world through various analytical techniques. Here, we’ll cover three main types of statistical analysis: descriptive statistics, inferential statistics, and advanced statistical techniques. Grab your favorite snack; this is going to be fun!
Descriptive Statistics
Descriptive statistics help us summarize and present data in an understandable way. Instead of drowning in numbers, we can use these techniques to communicate what the data really means.
First up, let’s talk about methods for summarizing data. You might have heard of measures of central tendency, which include the mean, median, and mode. The mean is your average Joe, the median is the middle value, and the mode is the life of the party—the most frequently occurring value. These measures give us a quick snapshot of our data.
Next, we have measures of dispersion, such as range, variance, and standard deviation. The range tells us the difference between the highest and lowest values, while variance and standard deviation reveal how spread out our data points are. Understanding these concepts is like knowing where all your socks go after laundry—important for keeping your sanity!
Now, let’s not forget visual representations! Graphs, charts, and tables act like the spice of life. A well-made bar graph can turn a boring dataset into a visual feast, while pie charts can make your data feel like a delicious slice of cake. Histograms, line charts, and scatter plots also play vital roles in illustrating relationships and trends within the data. With these visual tools, even your grandma can grasp complex information at a glance!
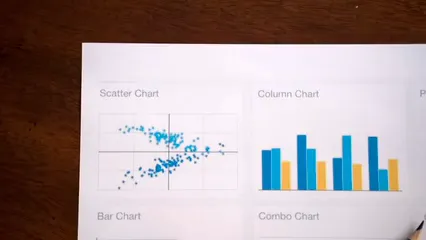
Inferential Statistics
Now that we’ve painted a pretty picture of our data, it’s time to infer. Inferential statistics allows us to make predictions about a population based on a sample. It’s like taking a bite of cake and predicting how the whole cake will taste!
One of the key concepts in inferential statistics is the confidence interval. This fancy term refers to a range of values that likely contain the true population parameter. For example, if we estimate that 60% of people prefer chocolate ice cream, our confidence interval might be between 55% and 65%. This means we’re pretty sure our estimate is accurate, but we leave a little wiggle room for uncertainty. Learn how to calculate test statistics for confidence intervals.
Next, we have p-values. These little numbers tell us the likelihood that our observed results happened due to random chance. If our p-value is less than 0.05, we usually reject the null hypothesis, which states that there is no effect or difference. In simpler terms, a low p-value indicates that our findings are statistically significant—yay, science!
Common statistical tests include t-tests, ANOVA, and chi-squared tests. T-tests help compare means between two groups, like chocolate vs. vanilla lovers. ANOVA (Analysis of Variance) takes it up a notch by comparing means across three or more groups. Chi-squared tests help us explore relationships between categorical variables, like whether people prefer chocolate over vanilla based on their age group.
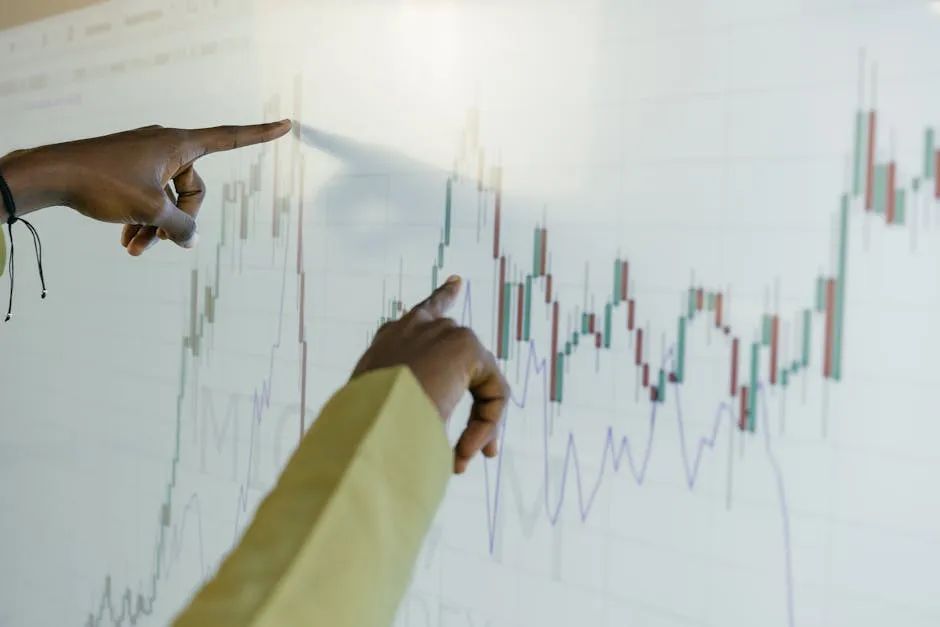
Advanced Statistical Techniques
As we move to advanced statistical techniques, we’re entering the realm of deeper analysis. Get ready for regression analysis and correlation!
Regression analysis allows us to understand relationships between variables. For instance, we can examine how ice cream sales correlate with temperature. By creating a regression model, we can make predictions based on independent variables. If the temperature rises, we can predict an increase in ice cream sales. Sweet!
Correlation, on the other hand, measures the strength and direction of a relationship between two variables. A positive correlation means that as one variable increases, so does the other. A negative correlation means they move in opposite directions. Correlation coefficients range from -1 to 1. A perfect positive correlation is 1, while a perfect negative correlation is -1. A correlation of 0 indicates no relationship. So, if you find that ice cream sales and umbrella sales have a correlation of -0.8, you might want to rethink your marketing strategy!
Lastly, let’s touch on Bayesian methods. These techniques provide a different approach to statistics by incorporating prior knowledge into the analysis. In Bayesian statistics, we update our beliefs based on new evidence. This approach is particularly useful in situations where prior data is available, helping refine our estimates and predictions.
In conclusion, understanding statistical analysis techniques is crucial for making informed decisions based on data. Descriptive statistics give us clarity, inferential statistics help us draw conclusions, and advanced techniques deepen our insights. So, let’s embrace the power of statistics and let the numbers tell their stories!

Applications of Statistics
Statistics is not just a collection of numbers; it’s the secret sauce that helps various industries make informed decisions. Let’s take a closer look at how statistics plays a pivotal role in business, healthcare, and social sciences.
Statistics in Business
In the business world, statistics is like having a crystal ball. Companies use data analysis to understand market trends, customer preferences, and competitive landscapes. This information is crucial for making smart decisions. Explore applied statistics in business and economics.
Market research relies heavily on statistical methods. Companies conduct surveys to gather customer feedback, using techniques like random sampling to ensure representative results. For instance, a beverage company might survey consumers to determine their flavor preferences before launching a new product. By analyzing this data, they can confidently tailor their marketing strategies.
Data-driven companies like Amazon and Netflix are prime examples of statistical success. Amazon uses complex algorithms to analyze customer behavior, recommending products based on previous purchases. Similarly, Netflix employs statistics to understand viewing patterns, enabling them to create tailored content that keeps subscribers engaged. These companies have mastered the art of using statistics for strategic advantage.
In summary, statistics empower businesses to make informed choices, optimize operations, and enhance customer satisfaction. It transforms raw data into actionable insights, proving that numbers can indeed tell fascinating stories!
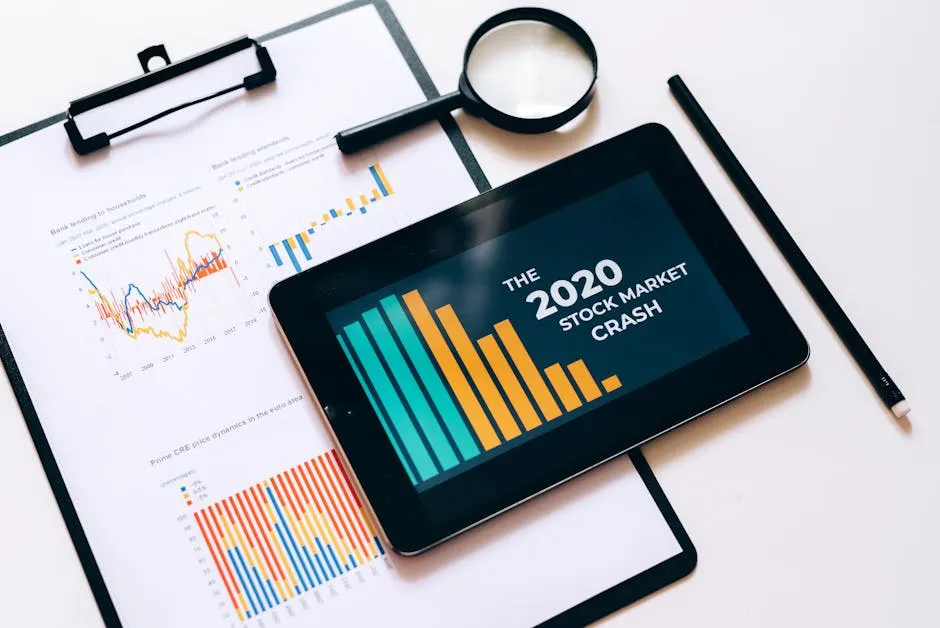
Statistics in Healthcare
Statistics is the unsung hero of healthcare. It plays a crucial role in clinical trials and public health studies, ensuring that treatments and interventions are both effective and safe.
In clinical trials, researchers use statistical methods to analyze the efficacy of new drugs. They compare data from participants receiving the treatment against those receiving a placebo. This not only helps determine whether a drug works but also evaluates potential side effects. For example, the COVID-19 vaccine trials utilized rigorous statistical analysis to ensure that the vaccines were both effective and safe for public use.
Moreover, public health studies depend on statistics to track disease outbreaks and assess intervention effectiveness. For instance, during a flu season, health organizations analyze statistics on infection rates to understand how the virus spreads. This information is vital for implementing strategies to protect communities.
Statistical analysis has also led to improved patient outcomes. By studying large datasets, healthcare providers can identify trends in patient care, highlight areas for improvement, and make data-informed decisions. For example, data analysis has shown that early detection of certain diseases significantly increases survival rates.
In essence, statistics in healthcare is about saving lives through informed decision-making. It underpins the scientific framework that guides treatment protocols and public health policies.
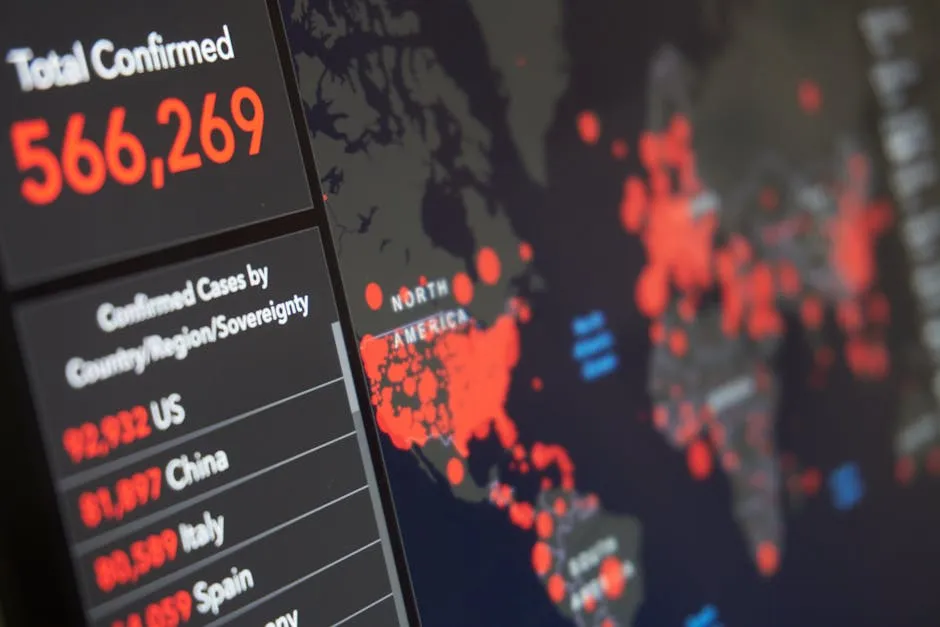
Statistics in Social Sciences
Statistics is the backbone of research in social sciences, including sociology, psychology, and economics. It provides the tools needed to analyze and understand complex social phenomena.
In sociology, researchers employ statistical methods to explore patterns of behavior within populations. For instance, they might conduct surveys to study the impact of education on income levels. By analyzing the data, they can identify correlations and trends, offering insights into societal issues.
Psychologists use statistics to test hypotheses about human behavior. Through experiments and observational studies, they gather data to better understand cognitive processes, emotions, and social interactions. For example, researchers might analyze the effects of various therapeutic approaches on mental health outcomes, using statistical tests to assess their effectiveness.
Economists rely on statistics to analyze market trends and consumer behavior. They use econometric models to interpret data on employment rates, inflation, and GDP growth. This statistical analysis is crucial for formulating economic policies that can stimulate growth and manage inflation.
The importance of data analysis in social sciences cannot be overstated. It helps researchers uncover societal trends, understand human behavior, and develop evidence-based policies. Statistics provides the foundation for informed discussions about pressing social issues, ensuring that decisions are driven by data, not just opinions.

Common Pitfalls and Misuse of Statistics
Statistics, while powerful, can also be misused. Understanding these common pitfalls is essential for anyone engaging with data.
One prevalent issue is the misinterpretation of data. Numbers can be misleading if taken out of context or presented without proper explanation. For example, a study might show that “70% of consumers prefer Product A.” Without additional information on the sample size or demographics, this statistic lacks meaning. Always consider the context and methodology behind the numbers!
Another common mistake is overgeneralization. Just because a trend appears in one dataset doesn’t mean it applies universally. For instance, a study on a small group cannot be extrapolated to the entire population. This can lead to faulty conclusions and misguided decisions.
Maintaining statistical literacy is crucial to avoid misinformation. In today’s data-rich world, it’s easy to be swayed by catchy headlines or cherry-picked statistics. Understanding the fundamentals of statistics allows individuals to critically evaluate claims and make informed decisions.
In summary, while statistics can illuminate truths, they can also obscure them if misused. By fostering a deeper understanding of statistical principles, we can navigate the complex landscape of data more effectively.
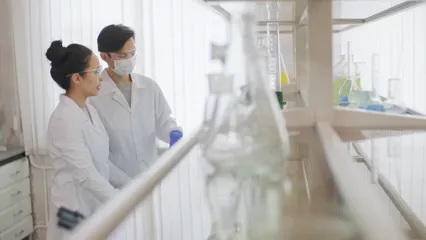
If you’re interested in further reading, check out this Statistics for Dummies guide. It’s a great way to brush up on your statistical knowledge in a fun and engaging manner!
Please let us know what you think about our content by leaving a comment down below!
Thank you for reading till here 🙂
All images from Pexels