Introduction
What is a Case Study? A case study is a detailed examination of a specific instance, often involving a company or a particular event. In the realm of business and statistics, case studies provide insights into successful strategies and methodologies. They bridge the gap between theory and practice, allowing businesses to learn from real-world applications. The significance of case studies lies in their ability to contextualize data. They illustrate how concepts translate into actual outcomes, demonstrating the practical implications of various strategies. For businesses, understanding these applications can lead to smarter decision-making. Purpose of the Article This article aims to explore the realm of statistical case studies within companies. We will showcase various methodologies employed by organizations and the outcomes of their analyses. By examining these cases, businesses can glean insights into effective strategies and the potential benefits of rigorous statistical analysis. Importance of Statistical Analysis in Case Studies Statistical analysis serves as a backbone in case studies, enhancing their reliability and validity. By incorporating quantitative data, organizations can substantiate their findings and make informed decisions. This analysis not only supports conclusions but also aids in identifying patterns and trends that may not be immediately apparent. With robust statistical methodologies, companies can confidently advocate for their strategies and initiatives, ensuring that decisions are driven by data rather than assumptions. In this context, understanding various statistical methods is essential for effective analysis.For those looking to dive deeper into data science methodologies, consider picking up a copy of R Programming for Data Science by Hadley Wickham. This book unravels the complexities of R, making it easier to understand and apply statistical methods effectively.
Understanding statistical methods enhances the reliability of case studies. Statistical methods for finance professionals 2024
Understanding Case Studies in Business
What Makes a Good Case Study?
Key Components A compelling case study consists of several essential components. First, background information sets the stage, offering context about the company and the challenges faced. Next, a clear problem statement outlines the issues at hand, followed by a comprehensive methodology detailing the approach taken. The results section highlights the outcomes, and the conclusion synthesizes the findings into actionable insights. Types of Case Studies There are various types of case studies, each serving a unique purpose. Illustrative case studies provide descriptive insights, while exploratory studies help identify hypotheses for further investigation. Cumulative case studies compile information from multiple sources, enhancing generalizability. Finally, critical instance case studies focus on unique situations, providing depth rather than breadth.The Role of Statistical Methods in Case Studies
Complementarity of Qualitative and Quantitative Research Combining qualitative and quantitative methods enriches the insights derived from case studies. While qualitative research provides depth and context, quantitative analysis offers measurable evidence. This fusion enables more comprehensive conclusions, as it captures both the nuances of human experience and the rigor of statistical validation. Examples of Statistical Methods Used Several statistical methods play a pivotal role in case studies. Regression analysis helps identify relationships between variables, while hypothesis testing assesses the validity of assumptions. Descriptive statistics summarize data, providing a snapshot of key trends and patterns. By employing these methods, organizations can extract meaningful insights that inform their strategic decisions.For anyone interested in a practical guide to data analysis, grab a copy of Data Analysis with R: A Hands-On Guide. This resource will empower you to conduct your own analyses and draw insightful conclusions.
In summary, case studies are invaluable in the business landscape. They provide a framework for understanding complex situations through empirical evidence. Statistical analysis enhances these studies, ensuring that the insights gleaned are both reliable and applicable. As businesses continue to navigate a data-driven world, harnessing the power of case studies and statistical methods will be crucial for sustained success.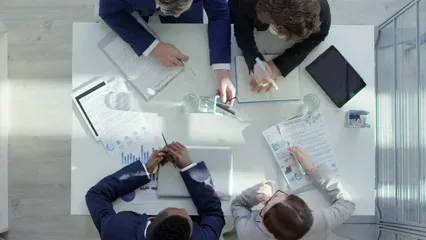
Case Study Examples from Various Industries
1. E-commerce: Amazon’s Data-Driven Decision Making
Background Amazon started as a humble online bookstore. Fast forward to today, it reigns supreme in the e-commerce sector. With over 150 million users worldwide, Amazon’s growth is nothing short of phenomenal. Its expansion into various sectors like Amazon Prime and AWS showcases its commitment to innovation and customer satisfaction. Statistical Techniques Used Amazon employs a robust data analytics system for personalized recommendations. Utilizing collaborative filtering, it analyzes user profiles and browsing behaviors. This method is not just a gimmick; it significantly drives sales. In fact, a staggering 35% of Amazon’s annual revenue stems from their recommendation system. That’s not just a number—it’s a testament to the impact of data-driven strategies. Outcomes The results speak volumes. By tailoring the shopping experience, Amazon has seen a remarkable boost in customer satisfaction and loyalty. Shoppers are not just buying products; they are enjoying a personalized journey. This data-driven approach not only enhances user experience but also solidifies Amazon’s position as a top player in online retail.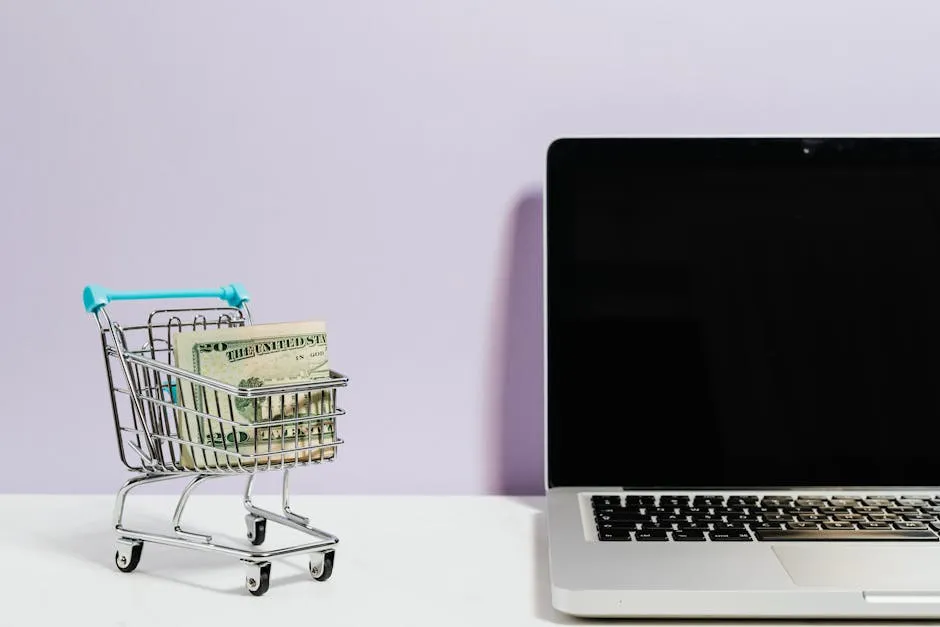
2. Healthcare: Predictive Analytics at Kaiser Permanente
Background Kaiser Permanente operates under a unique healthcare model that emphasizes prevention and care integration. With millions of members across the United States, it’s crucial for them to optimize patient outcomes while managing costs. Statistical Techniques Used Kaiser Permanente utilizes predictive modeling to assess patient outcomes. By analyzing historical data, they can identify high-risk patients who may require additional support. This proactive approach allows healthcare providers to intervene before issues escalate, improving overall patient care. Outcomes Thanks to predictive analytics, Kaiser Permanente has achieved a significant reduction in hospital readmissions. This not only enhances patient care but also reduces healthcare costs. The focus on preventive measures has resulted in a healthier patient population, demonstrating the transformative power of data in healthcare.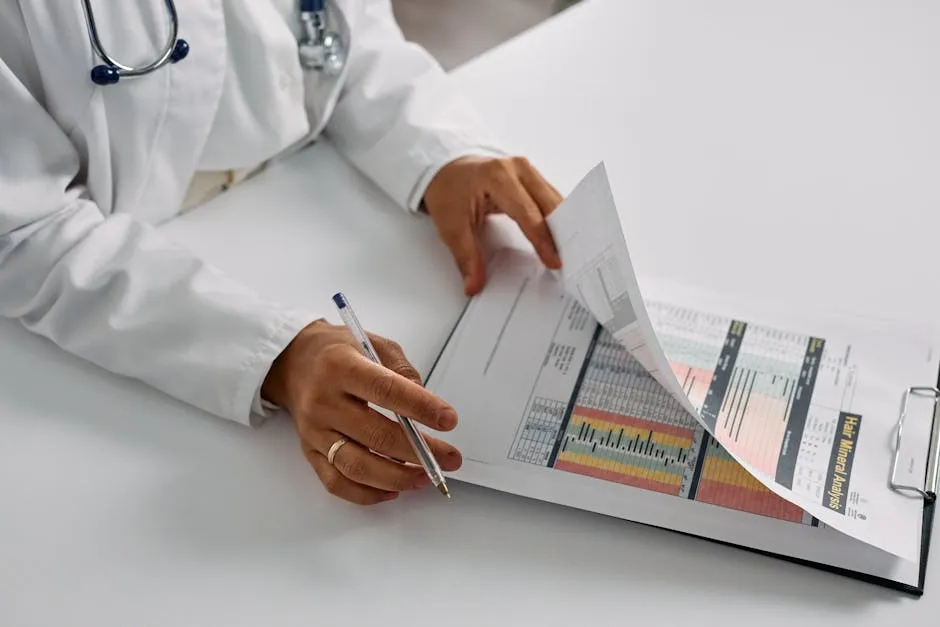
3. Finance: Fraud Detection at PayPal
Background PayPal is a global leader in online payments, facilitating millions of transactions daily. With such a high volume of transactions, ensuring security is paramount. Statistical Techniques Used PayPal employs advanced machine learning algorithms for fraud detection. These algorithms sift through transaction data in real time, identifying patterns that may indicate fraudulent activity. By continuously learning from new data, the system adapts to evolving threats. Outcomes The impact of this data strategy is profound. PayPal has seen an increase in fraud detection rates, significantly reducing financial losses. By safeguarding user transactions, PayPal not only protects its revenue but also enhances customer trust. This success story underscores the critical role of data analytics in the finance sector.
4. Marketing: Target’s Predictive Analytics for Consumer Behavior
Background Target is a powerhouse in the retail world, known for its savvy marketing strategies. The company has mastered the art of understanding consumer behavior. Target employs a mix of traditional advertising and innovative technology to connect with shoppers. Their approach goes beyond simple sales tactics; it’s about creating a personalized shopping experience. They leverage data analytics to anticipate customer needs and preferences, ensuring shoppers feel like Target knows them personally. This ability to connect is what keeps them ahead in the fiercely competitive retail landscape. Statistical Techniques Used At the heart of Target’s marketing success lies predictive analytics, particularly market basket analysis and customer segmentation. Market basket analysis helps Target understand the purchasing habits of its customers. By analyzing data on which products are frequently bought together, Target can optimize product placement and promotions. For example, if customers often buy diapers and beer simultaneously, Target can place these items closer together or create bundles to increase sales. Customer segmentation is another vital technique. Target collects data on demographics, shopping behavior, and preferences to categorize its customers into distinct groups. Each segment receives tailored marketing messages and promotions. This personalization enhances customer engagement, making shoppers feel valued and understood. Outcomes The outcomes of Target’s predictive analytics are impressive. By effectively targeting their marketing efforts, they have seen a significant increase in sales. The company reported a notable lift in sales for products that were promoted based on insights gained from their analytics. Moreover, customer loyalty has improved, with shoppers returning more frequently due to the tailored experiences they receive. Data-driven decisions allow Target to allocate marketing resources efficiently, maximizing their return on investment.
5. Manufacturing: Toyota’s Lean Manufacturing
Background Toyota is synonymous with quality and innovation in the automotive industry. The company has long been a leader in manufacturing efficiency, thanks to its pioneering lean manufacturing philosophy. This approach emphasizes eliminating waste while maximizing productivity. Toyota’s commitment to continuous improvement has defined its manufacturing processes, allowing it to produce vehicles that meet high standards without unnecessary costs. Statistical Techniques Used Toyota employs statistical process control (SPC) and quality assurance methodologies to maintain its manufacturing excellence. SPC involves using statistical methods to monitor and control production processes. By analyzing data from various stages of production, Toyota can quickly identify and address any deviations from quality standards. This proactive approach reduces defects and ensures that every vehicle meets the company’s stringent quality criteria. Quality assurance is another integral aspect of Toyota’s manufacturing strategy. The company utilizes rigorous testing and measurement techniques to assess product quality at every stage. By collecting and analyzing data on defects, Toyota can implement corrective actions swiftly. This focus on quality not only enhances customer satisfaction but also reinforces Toyota’s reputation for reliability. Outcomes The outcomes of Toyota’s lean manufacturing practices are remarkable. The company has achieved significant reductions in waste and production costs. By optimizing processes, Toyota has increased operational efficiency and enhanced its ability to respond to market demands. This has resulted in shorter lead times, allowing the company to bring new models to market faster than its competitors. Furthermore, the emphasis on quality has led to higher customer satisfaction. Toyota consistently ranks among the top automotive brands for reliability and safety, which translates into strong sales and brand loyalty. The integration of statistical techniques into its manufacturing processes demonstrates how data-driven decisions can lead to substantial improvements in both efficiency and product quality.
Analyzing Your Data
Statistical Tools and Software
When it comes to analyzing data from case studies, the right tools make all the difference. Several statistical software packages shine in this area, each with unique strengths. R is a powerhouse for statistical analysis. It’s open-source, meaning it’s free and has a vibrant community. With countless packages, R excels at complex analyses, making it a favorite among statisticians. Python is another heavyweight, especially with libraries like Pandas and NumPy. Its versatility extends beyond statistics, making it a go-to for data manipulation and machine learning. If you’re comfortable coding, Python is your friend. And for those looking for a solid introduction, Python Crash Course: A Hands-On, Project-Based Introduction to Programming by Eric Matthes is a fantastic resource. SPSS offers a user-friendly interface for those who prefer point-and-click. It’s widely used in social sciences and is great for performing statistical tests without needing to write code. Finally, Excel is the unsung hero. While it may not match the capabilities of R or Python, it’s accessible and familiar. Users can perform basic statistical analyses, create charts, and manipulate data efficiently. For many, Excel is the first step into the world of data analysis. If you want to get started with Excel, Excel 2021 for Dummies is a perfect guide.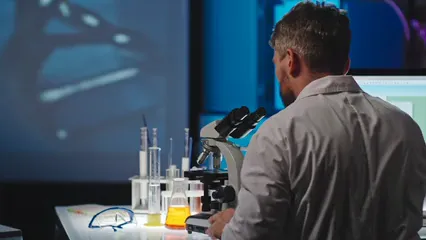
Interpreting Results
Once the data is crunched, interpreting the results is where the magic happens. But how do you draw meaningful conclusions from the numbers? Start by looking for patterns. Ask yourself questions: What do the trends suggest? Are there any surprising findings? Understanding the story behind the data is crucial. It’s not just about the statistics; it’s about what those statistics mean for your business. Next, consider the context. Numbers can be misleading without proper context. Compare your findings with industry benchmarks or past performance to gauge their significance. Are you performing better or worse than expected? Lastly, don’t shy away from visualizing your results. Graphs and charts can transform complex data into digestible insights. They not only make your findings clear but also engage your audience. Remember, a picture is worth a thousand words, especially in data interpretation!Presenting Your Findings
Structure and Clarity
When it’s time to present your findings, organization is key. A well-structured presentation helps convey your message effectively. Begin with an introduction that outlines the purpose of your analysis. What questions did you set out to answer? Follow with a clear methodology section. Explain how you gathered and analyzed your data. This transparency builds trust and credibility. Next, present your results clearly. Use bullet points for key findings and keep technical jargon to a minimum. Your goal is to ensure your audience understands the insights without feeling overwhelmed. Finally, conclude with actionable recommendations. What should the business do with these insights? Offer clear next steps based on your analysis to guide decision-making.Visual Aids
Visual aids are your best friends when presenting data. Charts, graphs, and tables can enhance understanding and retention. Choose the right type of visual for your data. Bar charts are great for comparing categories, while line graphs effectively show trends over time. Pie charts can illustrate proportions, but use them sparingly—too many segments can confuse. Tables are useful for presenting raw data but should be clear and concise. Avoid cluttering them with excessive information. Remember, each visual should serve a purpose, enhancing your message rather than distracting from it. Incorporating visuals can transform a dry presentation into an engaging narrative, making it easier for your audience to grasp complex information. So, don’t just tell your audience the data; show them!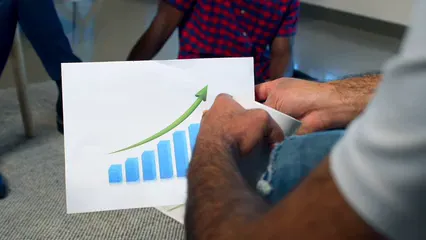
Conclusion
In the realm of business, case studies combined with statistical analysis create powerful insights. They help organizations understand their performance and identify areas for improvement. Case studies provide a narrative, contextualizing data in real-world scenarios. They illustrate how businesses apply strategies and the outcomes that follow. Statistical analysis, on the other hand, offers the rigor and quantifiable results necessary to validate those narratives. As we recap the importance of these tools, it’s clear they are not just academic exercises. They have real implications for business strategy and decision-making. Businesses that leverage these methodologies can navigate challenges more effectively, making informed choices that drive growth. Looking ahead, the role of data analytics will continue to evolve. With advancements in technology, businesses will have access to even more sophisticated tools and methodologies. This will enhance their ability to analyze data and derive actionable insights. Ultimately, embracing case studies and statistical analysis is not just about keeping up with trends. It’s about positioning your organization for success in an increasingly data-driven world. By investing in these practices, businesses can unlock new opportunities and achieve sustainable growth.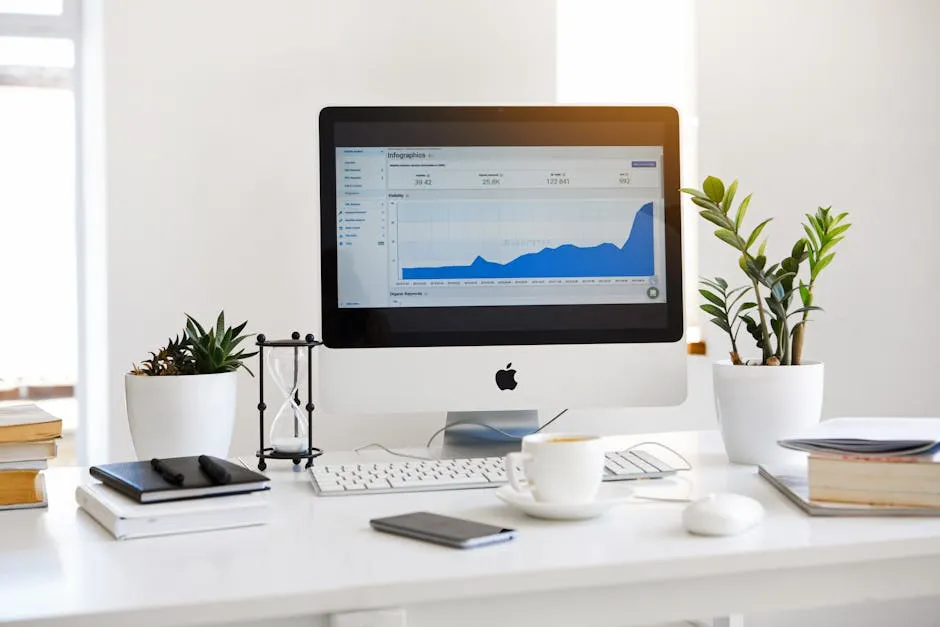
Final Thoughts
In today’s data-driven world, case studies are essential tools for strategic decision-making. They provide a detailed examination of real-world scenarios, allowing businesses to learn from both successes and failures. By analyzing these studies, companies can uncover valuable insights that guide their strategies and improve outcomes. Leverage the power of case studies to inform your decisions. They reveal best practices and innovative approaches that can enhance your business operations. Whether you’re a small startup or a large corporation, case studies offer a wealth of knowledge that can help you navigate challenges and seize opportunities. Remember, the key to successful implementation lies in understanding the context of each case study. Adapt the lessons learned to fit your unique situation. By doing so, you can create a more informed and effective strategy that drives growth and success.FAQs
What is the primary purpose of a case study?
The main purpose of a case study is to provide in-depth insights into a specific instance, event, or organization. They help illustrate complex concepts in a real-world context, allowing businesses to learn from actual experiences and outcomes.
How can statistical analysis improve the validity of a case study?
Statistical analysis enhances the validity of a case study by providing quantitative evidence that supports findings. By applying statistical methods, researchers can identify patterns, test hypotheses, and ensure that conclusions are based on solid data rather than assumptions.
What industries benefit most from case studies?
Case studies are beneficial across various industries, including healthcare, finance, marketing, and education. They help organizations understand best practices, assess performance, and make informed decisions tailored to their specific challenges and goals.
What are some common pitfalls to avoid in case study research?
Common pitfalls include focusing too narrowly on a single case, failing to collect diverse data, and neglecting to consider the broader context. Additionally, researchers should avoid confirmation bias, ensuring that they analyze data objectively and consider all relevant factors.
How can small businesses implement case study methodologies?
Small businesses can implement case study methodologies by starting with a specific challenge they face. They should gather data through interviews, surveys, or observations, analyze the findings, and document their insights. Sharing these learnings can help other small businesses benefit from their experiences, creating a community of shared knowledge.
All images from Pexels