Introduction
The world of statistics is like a vibrant marketplace where various models vie for attention, each promising to reveal hidden truths about data. Comparing the methodologies of the Department of Statistics Singapore (DOS) with global standards is intriguing. In this statistical showdown, we’ll uncover insights, best practices, and nuances that set these models apart.
Statistics is the backbone of informed decision-making. In Singapore, the Department of Statistics takes a meticulous approach, ensuring data integrity and relevance. Globally, diverse statistical models exist, shaped by different cultures, needs, and technological advancements.
One might wonder: How do these different approaches impact the results we rely on? Are Singapore’s models more robust, or do international standards offer greater flexibility?
The DOS focuses on producing reliable data across various sectors. Their emphasis on local context allows them to address specific national issues effectively. Conversely, global standards aim to create universally applicable methodologies, ensuring comparability across borders.
As we navigate this statistical landscape, we’ll explore key aspects such as the types of models employed, the rigor of data collection methods, and the real-world applications of these statistical techniques. Buckle up because this journey promises to be as enlightening as it is entertaining!
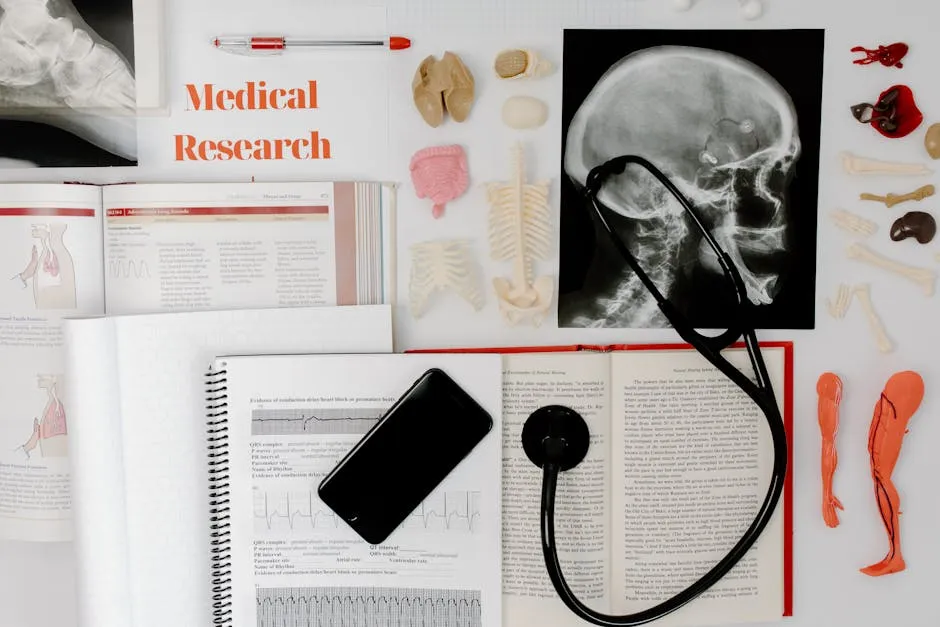
For a deeper understanding of statistical learning processes, refer to this introduction to statistical learning with Python.
If you’re keen on diving deeper into statistical methodologies, consider grabbing a copy of Poisson Regression Model Book. It’s a must-read for anyone looking to understand count data better—perfect for those who have ever wondered why there are so many accidents on their commute!
Summary of Key Points
In this article, we will explore the following key points:
- Overview of Statistical Models: A brief introduction to the significance of statistical models in data analysis.
- Department of Statistics Singapore: An in-depth look at the methodologies and practices adopted by Singapore’s statistical body.
- Global Standards: Understanding international statistical standards and how they compare to local approaches.
- Model Comparisons: A detailed analysis of different statistical models used in Singapore versus global practices, including strengths and weaknesses.
- Real-world Applications: Insights into how these models are applied in various sectors, from healthcare to finance.
- Best Practices: Recommendations for statisticians and data analysts when choosing models.
- Future Trends: Emerging trends in statistical modeling that could impact both local and global practices.
Through this exploration, readers will gain a clear understanding of how local and global statistical models function, their impacts on data-driven decisions, and best practices for future applications. With plenty of real-world examples and a sprinkle of humor, this post aims not just to inform but to engage and entertain!
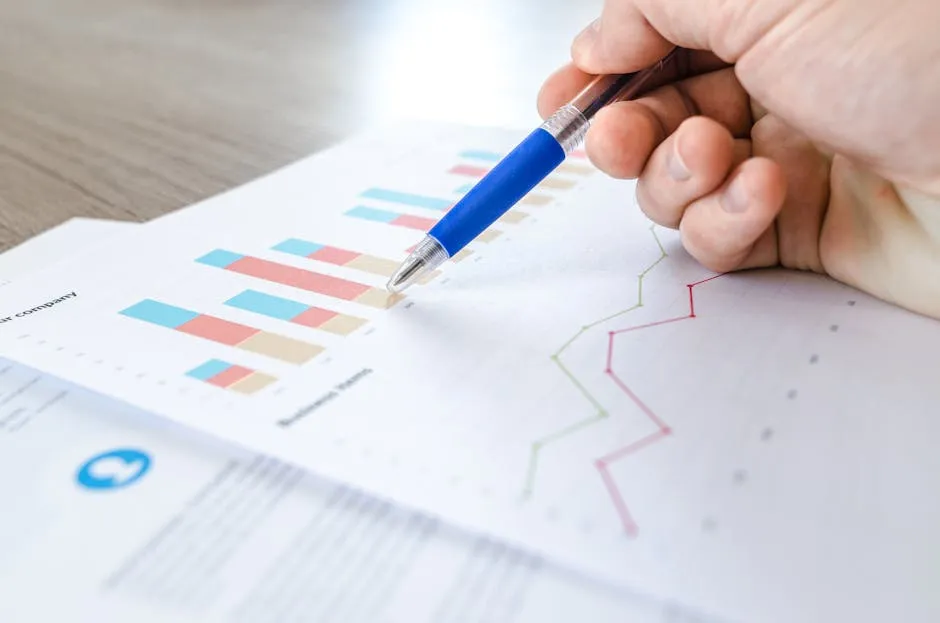
Department of Statistics Singapore
Mission and Vision
The Department of Statistics Singapore (DOS) aims to deliver reliable statistical information to support national development. Their mission revolves around providing high-quality data that informs policymakers. They envision a society where evidence-based decisions lead to sustainable growth and development. With a strong focus on accuracy and relevance, DOS plays a crucial role in shaping Singapore’s data landscape.
Statistical Models Used
Data Collection and Analysis Techniques
DOS employs various data collection methods to ensure comprehensive and accurate datasets. Surveys, for instance, provide critical insights into societal trends and economic conditions. Through national surveys like the Singapore Census and Household Income Study, DOS gathers vital information that informs policy decisions.
Data mining techniques are also utilized to extract valuable patterns from large datasets. This process involves filtering through vast amounts of data to identify trends that might not be immediately apparent. By leveraging advanced analytics, DOS enhances its ability to provide timely insights for policymakers.
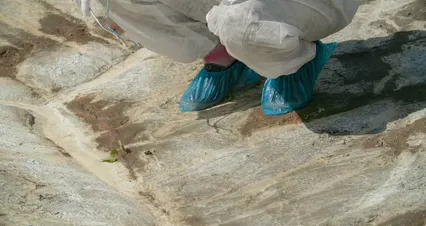
Key Models
DOS relies on several statistical models to analyze the data collected. One prominent model is Poisson regression, which is particularly effective for modeling count data—think of incidences like reported traffic accidents or hospital admissions. This model helps quantify the relationship between various factors and the frequency of certain events.
Time series analysis is another vital model used by DOS. This technique examines data points collected or recorded at specific time intervals. It’s crucial for forecasting economic indicators like GDP growth or inflation rates. By analyzing trends over time, DOS can predict future outcomes, allowing for proactive policy-making. If you’re interested in mastering this skill, check out the Time Series Analysis Book for a comprehensive guide.
For insights on using time series analysis effectively, check out this article on forecasting trends in manufacturing with time series analysis.

Case Studies
One striking case study involves the use of statistical models in Singapore’s housing policy. By utilizing Poisson regression, DOS assessed the impact of various factors on public housing demand. The model revealed significant correlations between income levels and housing needs, which directly influenced policy adjustments.
Another impressive application of time series analysis occurred during the COVID-19 pandemic. DOS used this model to forecast healthcare resource requirements, helping the government allocate resources efficiently. The timely insights prompted by these models significantly contributed to managing the crisis effectively.
In another instance, DOS applied data mining techniques in analyzing employment trends. By sifting through employment data, they identified emerging sectors that required workforce development. This analysis informed educational policies, ensuring the workforce remained relevant in a rapidly changing economic landscape.
These case studies illustrate how the Department of Statistics Singapore employs advanced statistical models to inform policy-making. The melding of rigorous data collection and analytical techniques enables DOS to provide actionable insights that ultimately benefit society. As the organization continues to evolve, it remains committed to using innovative statistical approaches to meet the challenges of tomorrow.

Comparing Models: Singapore vs. Global Standards
Strengths and Weaknesses
When comparing statistical models from the Department of Statistics Singapore (DOS) to global standards, it’s essential to highlight both their strengths and weaknesses.
DOS employs a robust set of models tailored for local needs. For instance, their use of Poisson regression excels in handling count data, making it invaluable for areas like traffic accident analysis. However, a limitation arises when they encounter overdispersed data, where the Negative Binomial model often outperforms it.
On the global stage, models like those promoted by the OECD emphasize flexibility and adaptability. Their emphasis on methods that incorporate international standards allows for better comparability across nations. However, this generalization can sometimes overlook local nuances, which may lead to less effective outcomes in specific contexts.
In summary, Singapore’s models shine in local specificity, while global standards excel in adaptability but may lack local relevance.

Data Quality and Accessibility
Data quality and accessibility play a critical role in the accuracy and application of statistical models. The DOS prides itself on high-quality data collection methods, utilizing national surveys and rigorous data validation processes. This ensures that the datasets are not only comprehensive but also reliable.
On the other hand, global standards often rely on data sharing among countries. While this can enhance the availability of diverse datasets, it can also lead to inconsistencies. For example, countries may have different definitions or methods for data collection, impacting the comparability of results.
Moreover, accessibility can be a double-edged sword. While open data initiatives promote transparency, they also raise concerns about data privacy and security. As countries move towards more open data policies, finding the right balance between accessibility and confidentiality becomes paramount.
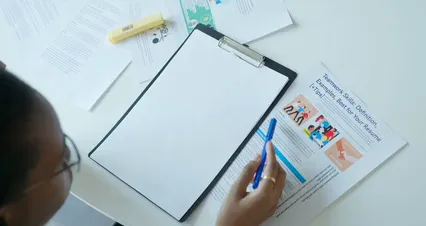
Cultural and Contextual Factors
Cultural and contextual factors significantly influence the choice and interpretation of statistical models. In Singapore, the approach to statistical modeling is deeply rooted in the nation’s unique socio-economic landscape. For instance, models addressing housing demand take into account local income levels and demographic trends, making them particularly relevant.
Conversely, global standards often adopt a one-size-fits-all approach. This can lead to models that may not resonate with local realities. For example, a model designed for predicting health outcomes in a high-income country may not apply well in a developing nation where healthcare access and cultural practices differ vastly.
Incorporating local context is crucial. Models that consider cultural factors can better reflect the complexities of human behavior. This, in turn, leads to more accurate predictions and effective policy outcomes.
Ultimately, the interplay between local and global contexts shapes the effectiveness of statistical models. By acknowledging and integrating these differences, statisticians can craft models that are not just statistically sound but also culturally relevant.
As we conclude this comparison, it’s clear that both the Department of Statistics Singapore and global standards have their unique advantages and challenges. Understanding these subtleties helps in selecting the right statistical approach for a given situation, ensuring that data-driven decisions are both informed and impactful.

Best Practices for Choosing Statistical Models
Selecting the right statistical model can feel like picking the perfect outfit for a first date—exciting yet daunting! The right model can lead to insightful conclusions, while the wrong one might leave you feeling embarrassed. Here’s how to ensure you choose wisely.
Model Selection Criteria
When choosing a statistical model, several factors come into play. First, consider your data type. Are you dealing with continuous, categorical, or count data? Each type calls for different modeling techniques. For instance, linear regression works wonders for continuous data, while logistic regression is your go-to for binary outcomes.
Next, think about your analysis goal. Are you aiming for prediction, explanation, or hypothesis testing? Your objectives will guide your choice. For instance, if you’re predicting future trends, time series models like ARIMA are fantastic. However, if you seek to understand relationships, regression models are more suitable.
Lastly, assess the data quality. High-quality data—clean, accurate, and relevant—leads to better model performance. If your data is full of holes or inaccuracies, even the fanciest model won’t save the day. To enhance your knowledge on this, consider checking out Data Mining Techniques Book to learn how to clean and analyze your data effectively.

Integration of Local and Global Practices
Combining local and global statistical practices can work wonders. The Department of Statistics Singapore excels in tailoring models to local contexts. However, global methodologies often incorporate best practices that enhance robustness. For example, adopting the OECD‘s guidelines on data collection can improve the quality of local datasets.
To blend these approaches, consider starting with a global model and adapt it to your local context. This way, you benefit from proven methodologies while addressing specific local needs. Collaborating with international experts can also provide fresh insights and enhance your analytical capabilities.

Continuous Learning
In the fast-evolving field of statistics, continuous learning is crucial. Keeping up with advancements in statistical modeling is like staying trendy in fashion. New techniques, tools, and algorithms emerge regularly, often reshaping the landscape.
Participate in workshops, webinars, and conferences to absorb new ideas. Online platforms like Coursera and edX offer courses on the latest statistical methods. Engaging with professional communities can also keep you informed about current trends and best practices. To further enhance your skills, consider the Statistics for Data Science Book that offers comprehensive insights into applying statistical concepts in data science.
In summary, selecting the right statistical model involves understanding your data, aligning with your analytical goals, and ensuring high data quality. By integrating local and global practices and committing to continuous learning, you’ll be well-equipped to navigate the intricate world of statistical modeling, ultimately leading to better data-driven decisions.

Future Trends in Statistical Modeling
Emerging Technologies
The rise of artificial intelligence (AI) and machine learning (ML) is transforming statistical modeling. These technologies enhance traditional models by automating complex analyses and uncovering patterns that were previously hidden. For example, machine learning algorithms can adapt quickly to new data, improving predictive accuracy. As these technologies continue to evolve, statisticians must embrace them to stay competitive.
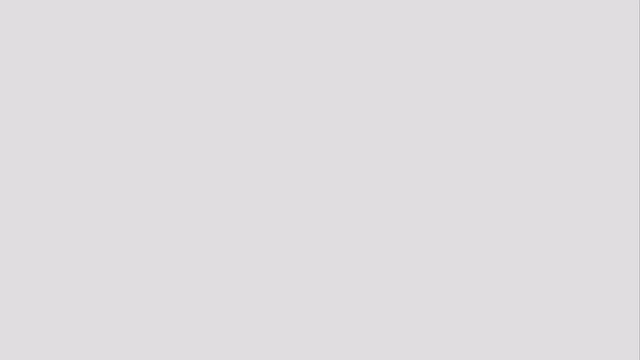
Global Collaboration
Sharing best practices across borders is becoming increasingly important. Global collaboration fosters innovation and knowledge exchange. By learning from the experiences of others, statisticians can refine their methods and improve their models. Initiatives like the OECD‘s Statistical Data and Metadata eXchange promote international cooperation in statistical practices, ensuring that countries benefit from each other’s expertise.
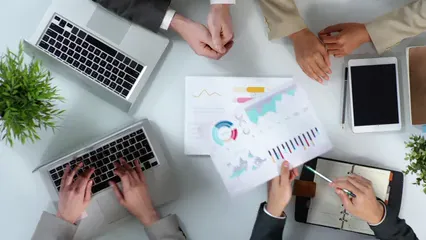
The Role of Open Data
Open data initiatives are revolutionizing statistical modeling. By making data widely accessible, researchers can analyze larger datasets, leading to more robust models. Open data encourages transparency and allows for independent verification of results. Countries that adopt open data policies can enhance their statistical modeling efforts, driving better decision-making and policy formulation. If you’re keen on understanding more about open data, consider reading Open Data Handbook for a comprehensive overview.
As we look to the future, emerging technologies, global collaboration, and open data will shape the evolution of statistical modeling. By staying ahead of these trends, statisticians can create more effective models that address complex problems, ultimately leading to more informed decisions and better outcomes across various sectors.

FAQs
What are the main differences between Singaporean and global statistical models?
Singaporean models often prioritize local context and specific societal needs. They utilize techniques like Poisson regression for count data and time series analysis for economic forecasting. In contrast, global models, such as those from the OECD, focus on comparability and adaptability across different nations, promoting methodologies that can be applied universally.
Why is it important to compare statistical models?
Comparing models fosters innovation and improvement. By understanding the strengths and weaknesses of various approaches, statisticians can adopt best practices, refine methodologies, and ultimately enhance the accuracy and effectiveness of their analyses. This process leads to better insights and informed decision-making.
How can I choose the right statistical model for my data?
Start by evaluating the type of data you have. Is it continuous, categorical, or count data? Next, consider your analysis goals—are you predicting outcomes or testing relationships? Finally, ensure the data quality is high. A well-defined framework can guide you in selecting the most suitable model for your specific needs.
What role does data quality play in statistical modeling?
High-quality data is crucial for accurate model outcomes. Flawed or incomplete data can lead to misleading results and poor decision-making. Ensuring that data is clean, relevant, and comprehensive enhances the reliability of statistical analyses, ultimately fostering trust in the insights derived from them.
Are there any common pitfalls in statistical modeling to avoid?
Yes! Frequent mistakes include using inappropriate models for the data type, neglecting data quality, and failing to validate results. Overlooking these factors can lead to incorrect conclusions. Always verify assumptions, validate models against real-world scenarios, and remain cautious about drawing conclusions from inadequate data.
Please let us know what you think about our content by leaving a comment down below!
Thank you for reading till here 🙂
All images from Pexels