Introduction
Matched pairs design is a statistical technique that allows researchers to compare two treatments while controlling for variables that could skew results. Imagine you’re testing a new diet. You want to ensure that the only difference between participants is the diet itself. That’s where matched pairs come in. By pairing participants based on specific characteristics like age, gender, or baseline health metrics, researchers can effectively isolate the treatment’s impact.
This design is especially valuable in experimental research. It ensures that each participant pair is as similar as possible, minimizing the influence of extraneous variables. This method is widely used in fields such as medicine, psychology, and education. For instance, in clinical trials, patients may be matched based on their health status to evaluate a new medication’s effectiveness. Similarly, in educational settings, students might be paired by academic performance to test different teaching strategies.
In the following sections, we will unpack the intricacies of matched pairs design. We’ll define it in detail, explore its key characteristics, and provide real-world examples to illustrate its application. Additionally, we will discuss the statistical analysis methods commonly used with matched pairs design and highlight its advantages, disadvantages, and best practices for implementation. By the end of this guide, you’ll have a comprehensive understanding of matched pairs design and its significance in statistical research.

What is Matched Pairs Design?
Matched pairs design, also referred to as paired samples design, is an experimental approach where participants are grouped into pairs based on specific characteristics. These characteristics can include age, gender, baseline measurements, or other relevant factors. The goal is to create pairs that are as similar as possible, allowing researchers to minimize variability and enhance the accuracy of their results.
In a typical matched pairs study, one participant from each pair is randomly assigned to the treatment group, while the other is assigned to the control group. This randomization within pairs helps ensure that any differences observed in outcomes can be attributed to the treatment rather than pre-existing differences between participants.
Key characteristics of matched pairs design include:
- Control of Confounding Variables: By matching participants on important characteristics, researchers can control for confounding variables that could influence outcomes. For example, if studying a new drug, matching participants on age and health status can help isolate the drug’s effects.
- Increased Statistical Power: Matched pairs design can enhance the statistical power of a study. This means researchers can detect smaller effects with greater confidence because the variability between pairs is minimized.
- Applications in Various Fields: Matched pairs design is widely used in clinical trials, educational research, and psychological studies. In clinical trials, participants might be matched based on age and health status to evaluate a new drug’s effectiveness against a placebo. In education, students could be paired based on test scores to compare the impact of different teaching methods.
For example, consider a study investigating the effectiveness of a new weight loss program. Researchers might match participants based on age, gender, and body mass index (BMI). One participant from each pair follows the new program, while the other follows a standard program. By comparing the results, researchers can determine the program’s effectiveness while controlling for individual differences.
In summary, matched pairs design is a powerful statistical tool that enhances the reliability of experimental findings. By carefully pairing participants based on relevant characteristics, researchers can make more accurate comparisons between treatments and draw valid conclusions about their effects.

The Structure of a Matched Pairs Design
Identifying Matching Variables
When researchers set out to conduct a matched pairs design, the first crucial step is identifying relevant characteristics for matching participants. These characteristics can vary but often include age, gender, baseline measurements, and other demographic factors that could impact the outcome of the study. Imagine you’re organizing a cooking competition. You wouldn’t want a novice chef competing against a culinary school graduate without controlling for skill levels. Similarly, in matched pairs design, researchers seek to create a level playing field.
Matching is essential because it helps control for confounding variables—those pesky influences that can skew results. By ensuring that participants are alike in key characteristics, researchers can be more confident that any observed differences in outcomes stem from the treatment itself rather than some underlying difference. For instance, if you’re testing a new medication, pairing participants based on age and health status ensures that the results reflect the drug’s effectiveness, not the participants’ varying health conditions.
The importance of matching cannot be overstated. It enhances the validity of the study by reducing the noise from extraneous variables. Good matching leads to more accurate comparisons between treatment groups. It’s like tuning a musical instrument; everything sounds better when the notes are in harmony.

Creating Matched Pairs
Once matching variables are identified, it’s time to form the matched pairs. This process involves several steps to ensure that each pair is appropriately matched before treatment assignment. First, researchers collect data on the identified variables. Next, they use this data to create pairs of participants who share similar characteristics. Think of it as finding a partner for a dance. You want to match the right partners based on their dance styles, so they move in sync.
After pairing participants, the next step is random assignment. This means one participant from each pair is randomly assigned to the treatment group, while the other is assigned to the control group. Randomization within pairs is critical; it helps eliminate bias and ensures that the treatment’s effects can be accurately assessed. It’s like flipping a coin to decide who gets the last slice of pizza—fair and square!
Let’s illustrate this with a case study. Imagine a study investigating the efficacy of a new antihypertensive drug. Researchers recruit 100 participants and match them based on age, gender, and baseline blood pressure. Each matched pair consists of two participants: one receives the drug, and the other receives a placebo. By comparing the blood pressure readings after treatment, researchers can ascertain whether the drug effectively lowers blood pressure while controlling for individual differences.
In summary, the process of creating matched pairs is foundational to the success of matched pairs design. By carefully identifying matching variables and ensuring fair treatment assignment, researchers can conduct studies that yield reliable and valid results. Whether it’s a clinical trial or a psychological experiment, matched pairs design allows for meaningful comparisons, leading to insights that can make a difference in real-world applications.

Common Statistical Tests
Paired t-Test
The paired t-test is a statistical method used to analyze data from matched pairs. Imagine you want to see if a new study technique improves test scores. You’d match students based on factors like age or prior performance. One half uses the new technique, while the other half continues with the old one.
The formula for the paired t-test is:
t = \frac{\bar{d}}{s_d / \sqrt{n}}
Where:
- \bar{d} is the mean of the differences between pairs.
- s_d is the standard deviation of those differences.
- n is the number of pairs.
Interpreting the results is straightforward. A significant t-value (usually compared to a critical value) indicates a true difference in effectiveness between the two techniques. If the p-value is less than your alpha level (commonly 0.05), you can confidently say the new technique made a difference.
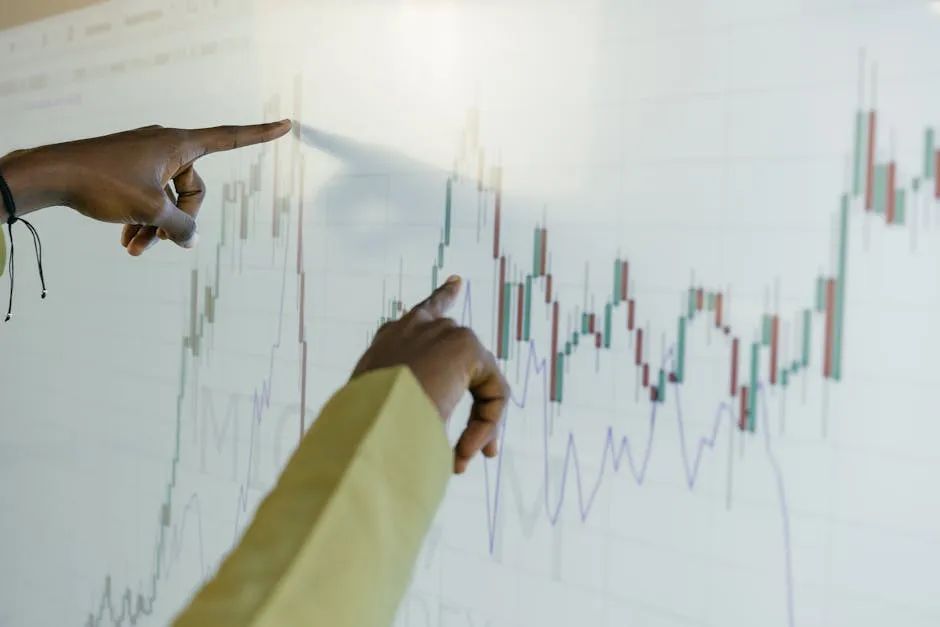
Non-Parametric Tests
Sometimes, data doesn’t meet the assumptions needed for a paired t-test. That’s where non-parametric tests come in handy! The Wilcoxon signed-rank test is a popular alternative. It’s great when you have small sample sizes or non-normally distributed data.
You’d use the Wilcoxon test when you want to compare the median differences between pairs rather than means. It ranks the absolute differences and assesses whether the ranks of positive and negative differences differ significantly. This method allows you to draw reliable conclusions even when data misbehaves.
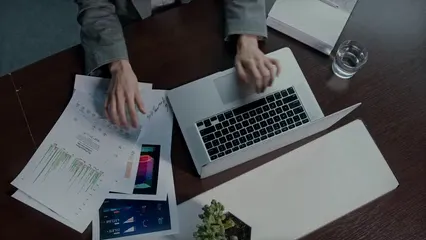
Interpreting Results
Example Calculations
Let’s say you conducted a study on a new reading program. You measured the reading scores of ten students before and after the program. Here are the scores:
Student | Before | After |
---|---|---|
1 | 75 | 85 |
2 | 80 | 90 |
3 | 65 | 70 |
4 | 90 | 95 |
5 | 70 | 80 |
6 | 60 | 65 |
7 | 85 | 90 |
8 | 78 | 88 |
9 | 82 | 84 |
10 | 76 | 80 |
Calculate the differences:
Student | Difference (After – Before) |
---|---|
1 | 10 |
2 | 10 |
3 | 5 |
4 | 5 |
5 | 10 |
6 | 5 |
7 | 5 |
8 | 10 |
9 | 2 |
10 | 4 |
The mean difference is:
\bar{d} = \frac{10 + 10 + 5 + 5 + 10 + 5 + 5 + 10 + 2 + 4}{10} = 6.6
Using the paired t-test formula, you can calculate the t-value and compare it against critical values to determine significance.
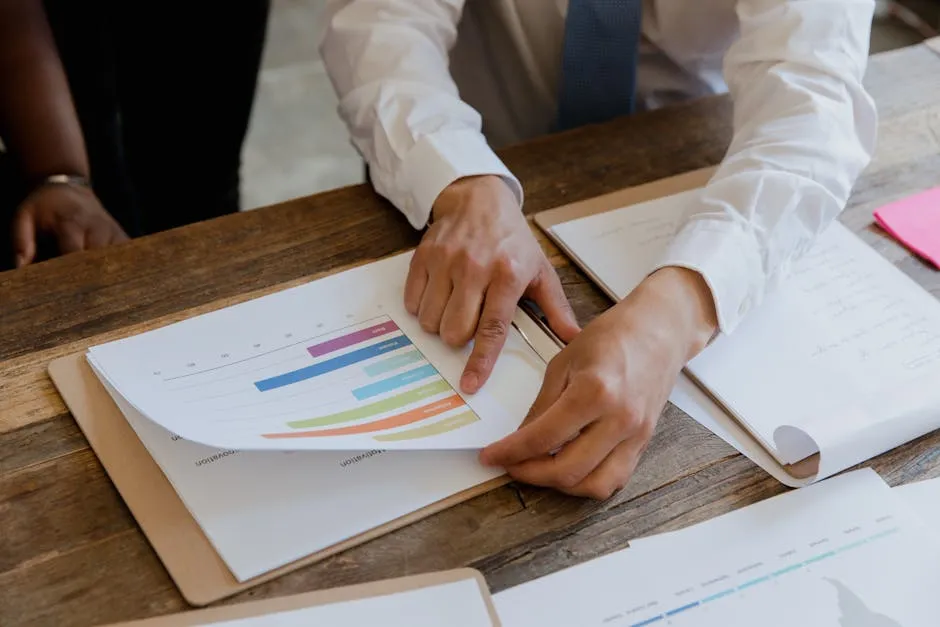
Advantages of Matched Pairs Design
Increased Statistical Power
Matched pairs design significantly boosts statistical power. By pairing participants based on key characteristics, researchers reduce variability between groups. This design allows researchers to detect smaller treatment effects more reliably. Picture a detective with a magnifying glass—matched pairs allow you to zoom in on subtle differences that might otherwise go unnoticed.

Reduction of Variability
One of the standout benefits of matched pairs design is its ability to minimize variability. By controlling for confounding factors, researchers ensure that the only differences between the groups stem from the treatments themselves. For example, in a clinical trial, matching patients by age and health status ensures that the observed effects are due to the drug, not variations in patient backgrounds.
Now, speaking of cooking up something great, if you’re looking to enhance your culinary skills, consider grabbing The Science of Cooking: Every Question Answered to Perfect Your Cooking. This book is like having a personal chef guiding you through the kitchen. With its insights, you’ll turn even the simplest meals into gourmet experiences!

Applicability in Small Sample Sizes
Matched pairs design shines when researchers deal with limited sample sizes. When participant numbers dwindle, random assignment alone can lead to imbalanced groups. By implementing a matched pairs approach, researchers maximize the data they gather from each participant, ensuring more robust findings even with fewer subjects. It’s a clever workaround that optimizes the potential of every participant, much like making a gourmet meal from a handful of pantry staples.
Speaking of maximizing potential, if you’re prepping meals for one, why not check out Cooking for One: 100 Delicious Recipes for One Person? It’s packed with recipes that are perfectly portioned, so you won’t be left with tons of leftovers!
In summary, matched pairs design enhances the reliability and validity of experimental findings. By controlling for variability and improving statistical power, this design is a go-to choice for researchers aiming for precision in their studies.

Disadvantages and Limitations of Matched Pairs Design
Challenges in Matching
Finding suitable matches can be a daunting task. Researchers often face difficulties in identifying pairs that share critical characteristics. Imagine trying to find identical socks in a messy drawer—it’s not as easy as it sounds! The more variables you want to match on, the harder it becomes. With multiple characteristics like age, gender, and health status, the pool of potential participants shrinks. This limitation can lead to an inability to form enough matched pairs, which may jeopardize the study’s validity. If researchers can’t find appropriate matches, they may have to compromise on matching criteria, leading to potential biases that could skew results.
Moreover, if the matching process is too rigid, it may result in losing valuable data. Picture a clinical trial where only those who fit the exact criteria are included. This can lead to a sample that is not representative of the broader population, limiting the generalizability of the findings. Therefore, while matching is essential for controlling confounding variables, the challenges associated with it can significantly impact the study’s execution and integrity.

Dropout Issues
Dropout is another significant concern in matched pairs design. If one participant in a matched pair decides to withdraw from the study, their partner must also be removed. This is akin to a two-for-one deal that no one asked for! Losing one participant means losing valuable data from both, which can lead to a reduction in statistical power.
Imagine a study with 50 matched pairs. If five participants drop out, you’re left with 45 pairs and a potential loss of insights. Such data loss can not only affect the study’s conclusions but may also skew the results if the dropouts are systematically different from those who remain. This situation poses a risk of bias and undermines the reliability of the findings. Researchers must, therefore, have robust strategies for participant retention to minimize dropout rates and ensure data integrity.
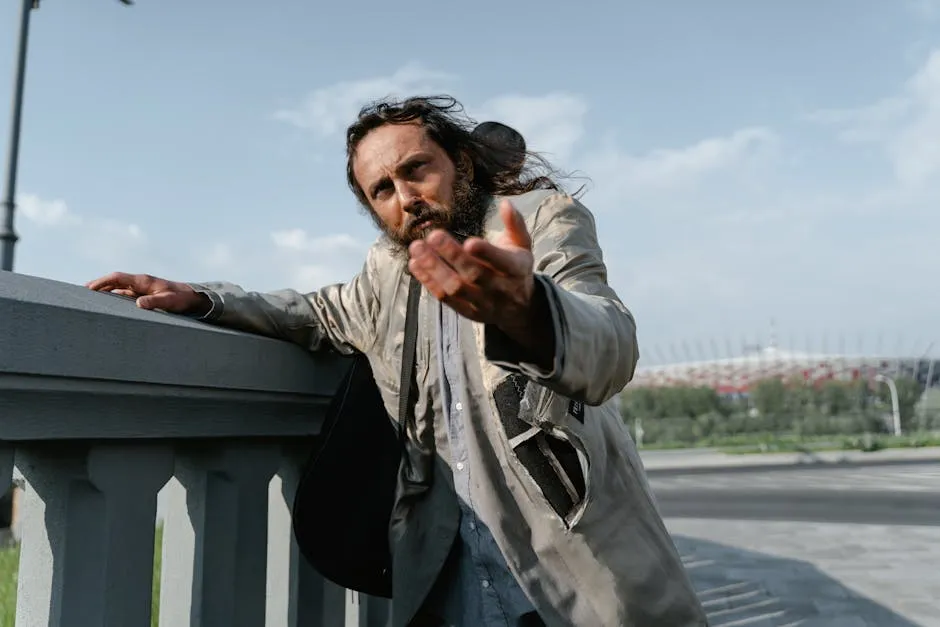
Potential for Residual Confounding
Even with careful matching, the lurking specter of confounding variables can still influence results. While matched pairs design aims to control for known confounders, it doesn’t guarantee that all variables are accounted for. For instance, if you’re studying the effectiveness of a new treatment, participants might be matched based on age and gender. However, if another variable, like socioeconomic status, is not considered, it could still impact the outcomes.
This residual confounding can lead to erroneous conclusions. It’s like trying to bake a cake and forgetting to measure the flour—your final product won’t turn out as expected. Therefore, while matched pairs design is a powerful tool for reducing variability, it’s vital for researchers to recognize its limitations. They must remain vigilant about potential confounders that could seep into the results and affect the study’s validity.
In summary, matched pairs design comes with its set of challenges. From the difficulties of finding suitable matches to the risks of dropout and unresolved confounding variables, researchers must navigate these hurdles carefully. Understanding these limitations can help in designing robust studies that yield valuable insights without falling prey to potential biases.

Best Practices for Implementing Matched Pairs Design
Planning and Preparation
Planning is the backbone of a successful matched pairs study. First, identify the variables you want to control. This could be age, gender, or any other relevant characteristic. Think of it as setting up a chessboard—every piece needs to be in its right place for a fair game. Once you’ve identified the matching variables, the next step is determining your sample size. A larger sample size often leads to more reliable results, but it also requires more resources. Balancing these factors is crucial, much like finding the perfect recipe for your favorite dish. You want enough ingredients to ensure it tastes good but not so many that it becomes overwhelming.
If you’re looking to make meal prep easier, consider investing in an Instant Pot Duo 7-in-1 Electric Pressure Cooker. This gadget can save you time and effort in the kitchen, allowing you to whip up delicious meals with minimal hassle!
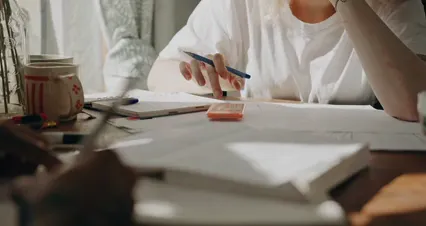
Data Collection and Management
When collecting data, consistency is key. Use standardized methods to gather information across all participants. This uniformity helps minimize errors. Imagine trying to compare apples to oranges; it doesn’t work well! Ensure that your data management system is robust. This means keeping track of who belongs to which pair and maintaining accurate records of their outcomes. It’s like running a well-organized library—every book (or in this case, participant) should be easy to find and track. Use statistical software to analyze the data efficiently, ensuring you can spot trends and insights quickly. After all, nobody wants to sift through piles of paperwork when they could be analyzing juicy data!

Statistical Analysis Planning
Before diving into the data, take a moment to plan your statistical analysis. This pre-planning is essential. Decide which statistical methods you will use based on the type of data collected. Common choices include the paired t-test and Wilcoxon signed-rank test. Each method has its strengths and weaknesses, so choose wisely! This choice is like picking the right tool for a job; using a hammer for a screw will only lead to frustration. Additionally, think about how you will interpret the results. Establish criteria for significance before you look at the data. This approach helps avoid biases that could cloud your conclusions. It’s similar to setting the rules before starting a game to ensure fair play.
Conclusion
Summary of Key Points
Throughout this guide, we explored the intricacies of matched pairs design in statistics. We began with an understanding of what matched pairs design entails, emphasizing its role in controlling variables and minimizing biases in experimental research. Key insights included the importance of identifying matching variables, creating well-defined pairs, and ensuring rigorous data collection and management practices. We highlighted the benefits of increased statistical power and reduced variability, which ultimately lead to more accurate and reliable results.
Moreover, we discussed the challenges researchers might face, such as difficulties in matching participants and the risk of dropout, which can undermine the integrity of the study. Best practices for implementing matched pairs design were outlined, focusing on careful planning, systematic data management, and thorough statistical analysis planning. By adhering to these principles, researchers can maximize the effectiveness of their studies.
Final Thoughts
Matched pairs design holds significant importance in enhancing the quality and accuracy of experimental research outcomes. By thoughtfully pairing participants based on relevant characteristics, researchers can better isolate the effects of treatments, leading to clearer conclusions. This design is particularly valuable in fields with limited sample sizes, where conventional methods may falter. As we move forward in an era increasingly reliant on data-driven decisions, mastering matched pairs design will empower researchers to produce reliable, impactful findings that can inform real-world applications and improve various domains, from healthcare to education.
FAQs
What is a matched pairs design?
A matched pairs design is an experimental approach that pairs participants based on shared characteristics. One participant in each pair receives a treatment while the other serves as a control, allowing for direct comparisons.
What are the advantages of using matched pairs design?
Matched pairs design offers several benefits, including increased statistical power, reduced variability, and the ability to control for confounding variables. This makes results more reliable and valid.
What are some common applications of matched pairs design?
This design is widely used in fields like medicine to evaluate treatments, psychology to assess behavioral interventions, and education to compare teaching methods. It helps provide clear insights into treatment effectiveness.
How do researchers handle participant dropouts in matched pairs design?
If a participant drops out, their paired counterpart must also be excluded to maintain the integrity of the study. To mitigate data loss, researchers can implement retention strategies and closely monitor participant engagement.
Can matched pairs design be used with more than two treatments?
While matched pairs design typically involves two treatments, researchers can adapt it by creating multiple matched pairs for each treatment condition or utilizing factorial designs to include additional treatments.
Please let us know what you think about our content by leaving a comment down below!
Thank you for reading till here 🙂
All images from Pexels