Introduction
Matched pairs statistics is a powerful tool in the realm of statistical analysis. It’s like having a secret weapon that allows researchers to control for confounding variables, ensuring the results of their studies are as accurate as possible. Imagine testing a new diet or treatment; matched pairs help ensure the participants are comparable, eliminating biases that could skew the results.
So, what exactly are matched pairs? Simply put, they are collections of pairs of observations that are linked in some meaningful way. This could be the same subjects measured before and after a treatment or different subjects paired based on similar characteristics. This design is particularly useful in studies with small sample sizes, where random assignment alone might not create balanced groups.
Matched pairs are fundamental in various scenarios, such as clinical trials, educational assessments, and psychological experiments. For instance, if a study measures the effectiveness of a new medication, researchers might compare the blood pressure of the same patients before and after treatment. This ensures that any changes observed are due to the medication and not other external factors.
In this comprehensive guide, we’ll break down the intricacies of matched pairs statistics. We’ll start with a clear definition of matched pairs and their characteristics. Following that, we’ll explore real-world examples to illustrate their application. Then, we’ll dive into hypothesis testing specific to matched pairs, ensuring you understand how to conduct and interpret these tests. Next, we’ll discuss key assumptions, common pitfalls to avoid, and the advantages and disadvantages of this design. Finally, we’ll explore how matched pairs are used across different fields, including healthcare, psychology, and business.
Get ready to unlock the potential of matched pairs statistics and elevate your data analysis skills to new heights!

What are Matched Pairs?
Definition of Matched Pairs
Matched pairs, sometimes referred to as paired samples, are groups of observations that are intentionally linked to control for variability. In a matched pairs design, researchers pair subjects based on specific characteristics or measurements. For example, in a study assessing the impact of exercise on weight loss, each participant’s weight could be measured before and after the intervention. This is an ideal scenario for matched pairs because it allows for direct comparison within the same subject.
The importance of matched pairs lies in their ability to control for confounding variables. By matching participants, researchers can reduce the noise in their data. This means that the only significant difference in the outcomes is likely due to the treatment or intervention being tested. For instance, if one group receives a new educational program while a matched group follows the traditional curriculum, any differences in performance can be attributed to the educational method rather than differences in prior knowledge or learning environments.
Matched pairs are particularly valuable when dealing with limited sample sizes. In these cases, matching can help ensure that even small groups maintain comparable characteristics, thus enhancing the reliability of the results. By controlling for confounding variables, matched pairs help researchers draw more accurate conclusions about cause and effect relationships.
In summary, matched pairs design is essential for any study aiming to produce reliable, valid results. By minimizing variability and controlling for external influences, researchers can gain deeper insights into the effects of interventions or treatments, making this a cornerstone of effective statistical analysis.

Examples of Matched Pairs
Matched pairs statistics shine brightly in the spotlight of real-world scenarios. They serve as a powerful tool in various fields, particularly in studies that aim to measure change over time. Let’s take a closer look at some common examples that illustrate the magic of matched pairs.
One classic example is the before-and-after studies. Imagine a group of participants trying out a new skincare regimen. Researchers might measure their skin’s moisture levels before the regimen begins, and then again four weeks later. By pairing these measurements, the researchers can directly assess the impact of the product on the same individuals. This approach minimizes variability and allows for a clearer picture of effectiveness. To enhance your skincare journey, consider the Before and After: A Skincare Journey. It’s a perfect companion for anyone wanting to see real results!
Another prominent domain is medical trials. In a clinical study evaluating a new medication, participants may be measured on a specific health outcome before and after the treatment. For instance, researchers might analyze blood pressure readings of patients before starting the medication and then again after six weeks. The paired approach ensures that any observed changes in blood pressure can be attributed to the medication rather than other external factors. For those interested in pain management, check out The Hypnotist’s Handbook: A Guide to Pain Management for insights on managing discomfort effectively.
Matched pairs are also frequently used in educational assessments. Consider a scenario where students take a test at the beginning of a semester and then again at the end. By pairing those scores, educators can evaluate the effectiveness of teaching methods and identify areas for improvement. For additional insights into educational psychology, Statistical Methods for Psychology provides a wealth of information.
In experimental designs, matched pairs help control for confounding variables. For example, when studying the effectiveness of a new diet, researchers might pair participants based on age, gender, and baseline health metrics. One member of each pair could follow the new diet, while the other continues with their usual eating habits. By analyzing the differences in outcomes, researchers can draw more accurate conclusions about the diet’s effectiveness. If you’re looking to dive deeper into statistical analysis, The Art of Data Analysis: A Guide for Beginners is an excellent resource.

Overview of Hypothesis Testing
Hypothesis testing is the backbone of statistical analysis, and when it comes to matched pairs, it becomes even more crucial. This process allows researchers to make inferences about populations based on sample data.
In matched pairs, we focus on two key hypotheses: the null hypothesis and the alternative hypothesis. The null hypothesis (H0) typically states that there is no difference between the paired samples. In contrast, the alternative hypothesis (Ha) posits that a significant difference exists. For example, in a study assessing the impact of a new weight-loss program, the null hypothesis may suggest that the mean weight before and after the program is the same, while the alternative hypothesis would argue that there is a difference in mean weight. If you want to enhance your understanding of weight loss strategies, consider The Complete Guide to Weight Loss.
The beauty of hypothesis testing in matched pairs lies in its ability to account for individual variability. By examining the differences between paired observations, we can utilize statistical tests—most commonly the paired t-test. This test evaluates whether the mean of the differences is significantly different from zero, providing a robust framework for decision-making.
For the test to be valid, certain assumptions must be met. The paired differences should ideally follow a normal distribution, especially when the sample size is small. If the assumptions hold, researchers can confidently use the t-distribution to calculate the test statistic and corresponding p-value.
Through hypothesis testing, matched pairs statistics not only validate the effectiveness of interventions but also guide future research directions. By rejecting or failing to reject the null hypothesis, researchers contribute valuable insights to their respective fields, paving the way for evidence-based practices and informed decision-making.
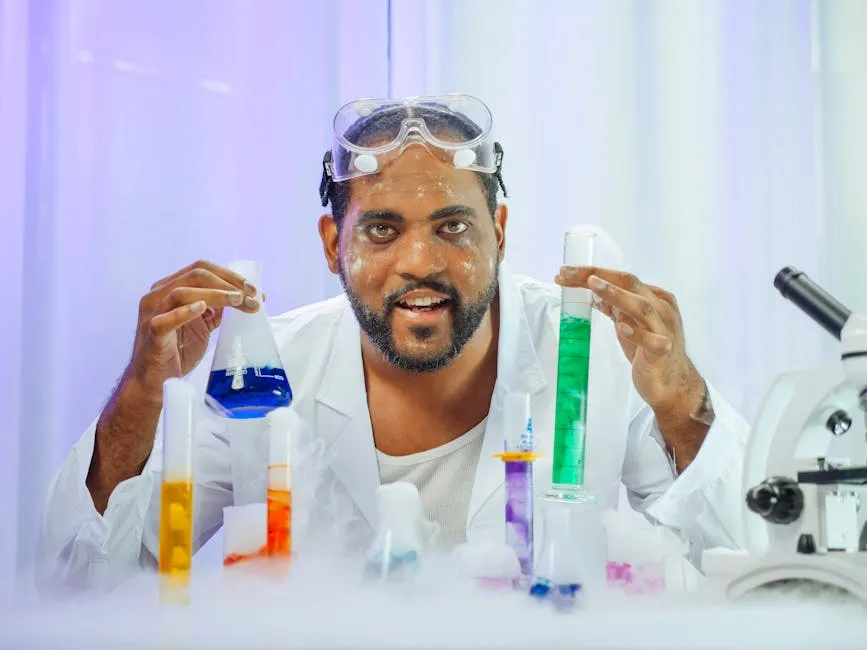
Conducting a Hypothesis Test
Conducting hypothesis tests for matched pairs involves several steps. Let’s break it down into a step-by-step process to ensure clarity and precision.
1. Collecting Paired Data
Start by gathering data in pairs. This data should come from the same subjects or closely matched subjects. For instance, if evaluating a weight-loss program, measure each participant’s weight before and after the program. This pairing helps control for individual variability.
2. Calculating Differences
Next, calculate the differences between the paired measurements. This is done by subtracting the “before” value from the “after” value for each pair. For instance, if a participant weighed 150 pounds before and 145 pounds after, the difference is -5 pounds. This difference forms the core of your analysis.
3. Determining the Mean and Standard Deviation of Differences
With your differences calculated, find the mean of these differences. This mean provides insight into the average change across all pairs. Additionally, compute the standard deviation of the differences to understand the variability. A low standard deviation suggests the changes are similar across subjects, while a high standard deviation indicates more variability. If you’re keen on tracking your fitness progress, a Fitness Tracker Watch could be the perfect addition to your routine!
4. Choosing the Appropriate Statistical Test
For matched pairs, the paired t-test is typically the go-to statistical test. This test evaluates whether the mean of the differences is significantly different from zero. Ensure that the assumptions for the paired t-test are met—namely, that the differences are approximately normally distributed.
5. Calculating the Test Statistic and p-value
Finally, calculate the test statistic using the formula:
\[ t = \frac{\bar{x}_d – \mu_d}{\left(\frac{s_d}{\sqrt{n}}\right)} \]
where \(\bar{x}_d\) is the mean of the differences, \(\mu_d\) is the hypothesized population mean difference (usually 0), \(s_d\) is the standard deviation of the differences, and \(n\) is the number of pairs. Once you have the t-value, use a t-distribution table or software to find the p-value. This p-value shows the probability of observing your results under the null hypothesis.
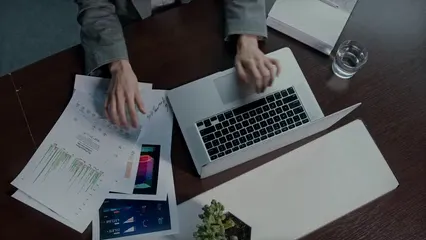
Example: Effectiveness of Hypnotism in Reducing Pain
Now, let’s consider a real-world application of the paired t-test, focusing on the effectiveness of hypnotism for pain reduction.
In a study conducted with randomly selected subjects, researchers measured pain levels before and after hypnosis using a scale where lower scores indicated less pain. Here’s the data collected:
Subject | Before | After |
---|---|---|
A | 6.6 | 6.8 |
B | 6.5 | 2.4 |
C | 9.0 | 7.4 |
D | 10.3 | 8.5 |
E | 11.3 | 8.1 |
F | 8.1 | 6.1 |
G | 6.3 | 3.4 |
H | 11.6 | 2.0 |
Calculating Differences
Subtract the “before” scores from the “after” scores to get the differences:
Subject | Difference |
---|---|
A | 0.2 |
B | -4.1 |
C | -1.6 |
D | -1.8 |
E | -3.2 |
F | -2.0 |
G | -2.9 |
H | -9.6 |
These differences yield a new dataset: {0.2, -4.1, -1.6, -1.8, -3.2, -2.0, -2.9, -9.6}.
Mean and Standard Deviation of Differences
Calculating the mean of the differences yields \(\bar{x}_d = -3.13\) and the standard deviation \(s_d = 2.91\).
Hypotheses
– Null Hypothesis (H0): \(\mu_d \geq 0\) (no improvement)
– Alternative Hypothesis (Ha): \(\mu_d < 0\) (improvement)
Test Statistic and p-value Calculation
Using the test statistic formula:
\[ t = \frac{-3.13 – 0}{\left(\frac{2.91}{\sqrt{8}}\right)} \approx -3.036 \]
With 7 degrees of freedom, the p-value calculated is approximately 0.0095.
Decision
At a significance level of \(\alpha = 0.05\), since \(p < \alpha\), we reject the null hypothesis. This indicates sufficient evidence to conclude that hypnotism effectively reduces pain levels on average. If you’re curious about more ways to manage pain, consider The Complete Guide to Hypnosis for Pain Relief.

Assumptions and Considerations
Key Assumptions
When it comes to matched pairs statistics, we’re dealing with a few crucial assumptions that can make or break your analysis. Let’s unravel these assumptions to ensure your results are as valid as a perfectly brewed cup of coffee!
1. Normality of Differences
First up, we have the assumption of normality. The differences between paired samples should ideally follow a normal distribution. This means that if you were to plot these differences on a graph, they should form a nice bell curve. If your sample size is small (typically less than 30), you’ll want to check this condition closely. If the data doesn’t meet the normality assumption, it might be worth considering a non-parametric test like the Wilcoxon signed-rank test. After all, nobody wants to serve a half-baked analysis!
2. Dependence of Samples
Next, we have the dependence of samples. In matched pairs design, each participant in one group is linked to a specific participant in another group. Think of it as pairing socks—each sock has a mate! This dependency is essential because it helps control for confounding variables that might skew your results. If you treat the samples as independent when they are not, you might just end up comparing apples to oranges.
3. Random Sampling
Lastly, we need to ensure that the samples are drawn randomly. Random sampling helps eliminate bias. If your pairs are not randomly selected, you might as well be flipping a coin when making conclusions! Random selection ensures that every participant has an equal chance of being chosen, which increases the generalizability of your results.
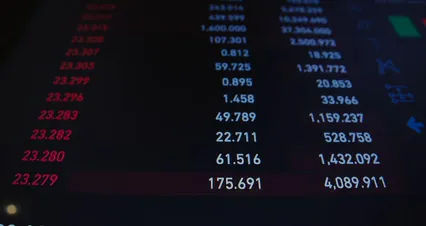
Common Pitfalls
Now that we’ve covered the assumptions, let’s look at some common pitfalls when conducting matched pairs analysis. Avoiding these missteps will help you maintain the integrity of your study.
1. Improper Matching
One of the biggest mistakes researchers make is failing to match participants appropriately. If you match individuals based on irrelevant characteristics, the analysis will be flawed. For example, pairing participants based solely on their favorite pizza topping instead of relevant health metrics could lead to misleading conclusions. Always ensure that the matching criteria are genuinely related to the outcome you’re measuring.
2. Ignoring Assumptions
Another common blunder is ignoring the assumptions we just discussed. Researchers sometimes jump into analysis without verifying normality or the dependency of their samples. This oversight can lead to invalid conclusions. Always check your assumptions—after all, good researchers are like great chefs; they don’t skip the prep work!
3. Inadequate Sample Size
Let’s not forget about sample size. A small sample may not provide enough power to detect a significant effect, especially if the effects are subtle. On the flip side, a sample that’s too large can lead to statistically significant results that lack practical significance. Striking the right balance is key!
4. Failing to Analyze Differences
Lastly, it’s crucial to focus on the differences between paired observations. Sometimes researchers get so caught up in the original measurements that they forget to compute and analyze the differences. Focusing on the wrong data is like trying to read a book without turning the pages—frustrating and unproductive!
Strategies to Avoid Pitfalls
To steer clear of these pitfalls, take a moment to plan your study carefully. Ensure you have a solid matching strategy in place, verify the assumptions, and be mindful of your sample size. Regularly consult statistical resources or collaborate with a statistician for guidance. Remember, it’s always better to ask for directions than to get lost in the woods!
By adhering to these assumptions and avoiding common pitfalls, your matched pairs analysis will be on the right track, yielding valid results that can confidently drive your conclusions. Happy analyzing!

Advantages and Disadvantages of Matched Pairs Design
Advantages
Matched pairs design has gained popularity in research due to its significant advantages. One of the most notable benefits is increased statistical power. By reducing variability between groups, researchers can detect differences more easily. Think of it as trying to find a needle in a haystack—less hay means a better chance of spotting that needle!
Another key advantage is better control of confounding variables. In matched pairs, participants are paired based on shared characteristics. This ensures that any differences observed in outcomes can be more confidently attributed to the treatment rather than other factors. It’s akin to cooking a gourmet meal with the right ingredients—precision leads to delicious results!
Additionally, matched pairs design is incredibly useful in scenarios with limited sample sizes. In such cases, researchers can still achieve statistically meaningful results without needing a large pool of participants. This can be particularly beneficial in fields like medicine, where recruiting enough participants can be challenging. By matching participants, researchers can ensure their data is robust and reliable, even with fewer subjects. If you’re interested in learning more about practical statistics, Practical Statistics for Data Scientists is a great resource.
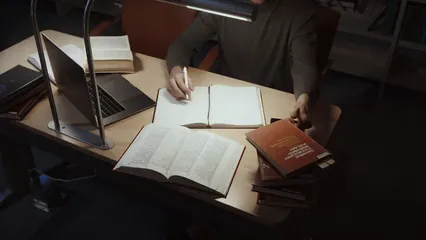
For more insights on enhancing your data analysis skills, check out our tips for effective data analysis in economics and statistics.
Disadvantages
However, matched pairs design is not without its challenges. One significant disadvantage is the complexity involved in matching participants. Finding suitable pairs that share essential characteristics can be time-consuming and sometimes impractical. Imagine trying to find the perfect dance partner—sometimes it’s just easier to go solo!
Another drawback is the potential for loss of data if one participant drops out. In a matched pairs study, if one individual from a pair withdraws, the entire pair may need to be removed from the analysis. This can lead to a significant reduction in sample size, impacting the study’s power and reliability. It’s like having a two-part puzzle where one piece goes missing—good luck completing that picture!
Lastly, matched pairs design may limit generalizability. Since participants are matched based on specific characteristics, the findings may not be applicable to a broader population. While this design is excellent for specific studies, it might not always translate well to different groups or settings. It’s essential to be cautious when drawing conclusions from such tailored data; after all, one size does not fit all!
In summary, while matched pairs design offers numerous advantages, researchers must navigate its complexities and limitations. Understanding these factors is crucial for making informed decisions about whether this design suits their research objectives. Also, if you’re looking for a good read on the psychology behind pain, consider The Psychology of Pain: Understanding Your Body.

Please let us know what you think about our content by leaving a comment down below!
Thank you for reading till here 🙂
If you’re looking for a detailed guide on hypothesis testing, check out our statistics hypothesis testing cheat sheet.
All images from Pexels