Introduction
StatCrunch is a robust statistical software tool. It provides an intuitive platform for a variety of statistical analyses. One of its key features is hypothesis testing, which helps users make informed decisions based on data. If you’re looking for a reliable statistical software, check out StatCrunch Software!
Understanding test statistics is crucial. They serve as the backbone of hypothesis testing, allowing researchers to evaluate claims about populations. When you grasp test statistics, you empower yourself to make data-driven choices that can change the course of a study or project.
This article will thoroughly explore test statistics using StatCrunch. We’ll cover step-by-step instructions, practical examples, and best practices. By the end, you’ll be well-equipped to tackle statistical analysis with confidence and a bit of flair!

Understanding Test Statistics
What is a Test Statistic?
A test statistic is a standardized value. It arises from sample data during hypothesis testing. Essentially, it helps determine whether to reject or fail to reject the null hypothesis.
When you conduct a hypothesis test, you compare the test statistic to a critical value. If your test statistic exceeds the critical value, it’s time to bid farewell to the null hypothesis. It’s like a dramatic exit in a play—goodbye, old friend!
Types of Test Statistics
There are several types of test statistics, each suited for different scenarios. Let’s break them down:
- Z-Test: This is your go-to for large sample sizes (n > 30) or when the population variance is known. Imagine you’re checking if a new teaching method boosts test scores. Use the z-test to compare means.
- T-Test: Perfect for small sample sizes (n < 30) or unknown population variances, this test helps you compare sample means. For instance, if you’re curious whether two different diets affect weight loss differently, the t-test is your friend.
- Chi-Square Test: This one deals with categorical data. Use it when you want to see if two variables are independent. Picture a survey about favorite ice cream flavors among different age groups—this test helps you understand if age influences flavor choice.
Each type of test statistic has its unique application, so choosing wisely is key! By using the right test, you set the stage for accurate and meaningful results.
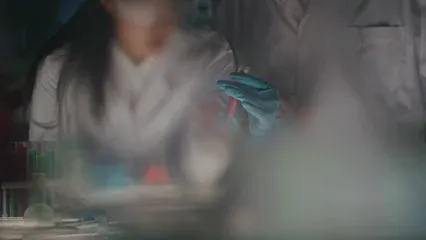
Performing Hypothesis Tests Using StatCrunch
One-Sample Tests
One-Sample Z-Test
A one-sample z-test is your best buddy when you have a large sample size (n > 30) or know the population variance. Think of it as your trusty sidekick when comparing a sample mean to a known population mean!
When to Use: Use the z-test when the sample size is large, and the population standard deviation is known. For instance, if you want to check if a new drug lowers blood pressure more effectively than a known mean, this is your go-to test.
Step-by-Step Instructions:
- Open StatCrunch: Click the box next to your dataset and select “Open in StatCrunch.”
- Select the Z-Test: Navigate to “Stat,” then “T Stats” (yes, it’s a bit cheeky here) and select “One Sample.”
- Enter Data: Choose “with summary” if you have the mean, standard deviation, and sample size. Input your values. For example:
- Sample Mean (X̄): 80
- Population Mean (μ): 75
- Standard Deviation (σ): 10
- Sample Size (n): 50
- Set Hypotheses: Fill in your null hypothesis (H0: μ = 75) and alternative hypothesis (H1: μ ≠ 75).
- Compute: Hit that compute button! StatCrunch will churn out a test statistic and a p-value.
- Interpret Results: If your p-value is less than your alpha level (commonly 0.05), you reject the null hypothesis. In our example, if the p-value is 0.03, it suggests the sample mean is significantly different.
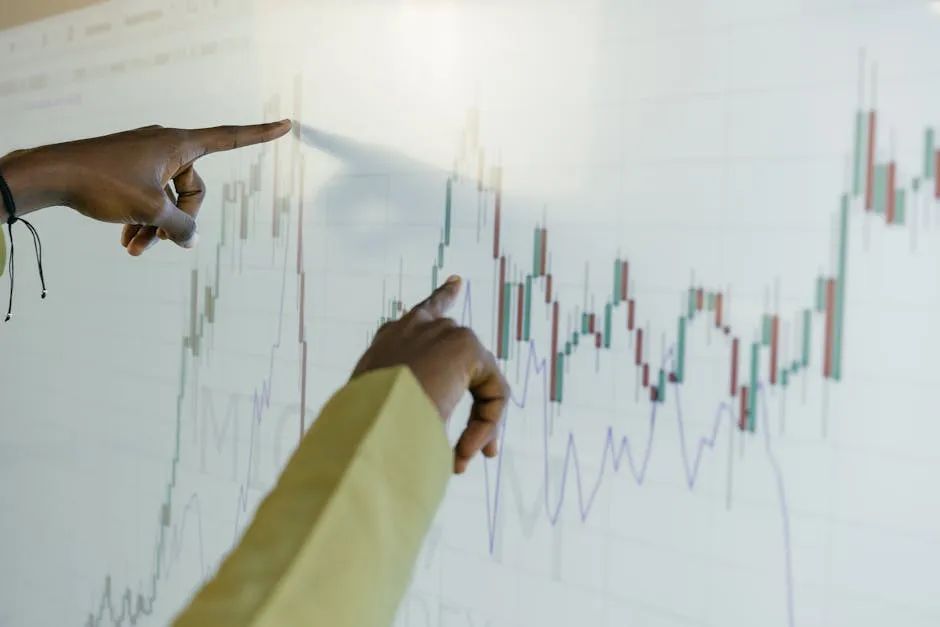
One-Sample T-Test
The one-sample t-test shines when you have a small sample size (n < 30) or don’t know the population standard deviation. It’s like your favorite sweater on a chilly day—comforting and reliable!
Conditions for Use: Use this test when your sample size is small and the population standard deviation is unknown. For instance, if you’re testing the average number of hours students sleep and only surveyed 15 students, the t-test is your friend.
Step-by-Step Instructions:
- Open StatCrunch: Just like before, click the box next to your dataset and select “Open in StatCrunch.”
- Choose the T-Test: Go to “Stat,” then select “T Stats” and “One Sample.”
- Input Summary Data: Click on “with summary” and fill in your stats:
- Sample Mean (X̄): 6.5
- Sample Standard Deviation (s): 1.5
- Sample Size (n): 15
- Set Hypotheses: Enter your null hypothesis (H0: μ = 7) and alternative hypothesis (H1: μ ≠ 7).
- Compute: Click that compute button. StatCrunch will give you the test statistic and p-value.
- Interpret Results: If the p-value is less than your alpha level, you reject H0. For example, a p-value of 0.04 suggests that the average sleep hours are significantly different from 7 hours.

Two-Sample Tests
Two-Sample T-Test
Two-sample t-tests are your go-to when comparing the means of two independent groups. Think of it as a friendly competition between two teams!
Independent vs. Paired Samples: Independent samples occur when the groups are not related. For example, comparing test scores between two different classrooms. Paired samples are used when the same subjects are tested under different conditions, like measuring blood pressure before and after treatment.
Step-by-Step Guide:
- Open StatCrunch: Click the box next to your dataset and choose “Open in StatCrunch.”
- Select the T-Test: Go to “Stat,” select “T Stats,” and then pick “Two Sample.”
- Choose Your Data: For independent samples, select “with summary.” Enter your data:
- Group 1: Mean = 78, SD = 10, n = 30
- Group 2: Mean = 85, SD = 12, n = 35
- Set Hypotheses: Fill in H0 (μ1 = μ2) and H1 (μ1 ≠ μ2).
- Compute: Click compute! StatCrunch will provide the test statistic and p-value.
- Interpret the Results: If the p-value is less than 0.05, there’s a significant difference between the groups. For example, if the p-value is 0.02, you can conclude that the average test scores differ significantly.

Two-Sample Proportion Test
This test is perfect for comparing proportions between two independent groups! Imagine a taste test between two different brands of ice cream. Which one is preferred?
When to Use: Use this test when you want to compare the proportions of a characteristic in two groups. For example, comparing the proportion of students who prefer online learning versus in-person classes.
Instructions:
- Open StatCrunch: Click the box next to your dataset and select “Open in StatCrunch.”
- Choose the Proportion Test: Navigate to “Stat,” then “Proportion Stats,” and select “Two Sample.”
- Enter Your Data: Input the number of successes and sample sizes for both groups:
- Group 1: Successes = 40, n = 100
- Group 2: Successes = 60, n = 120
- Set Hypotheses: Fill in H0 (p1 = p2) and H1 (p1 ≠ p2).
- Compute: Click that compute button! StatCrunch will give you the test statistic and p-value.
- Interpret Results: If your p-value is below 0.05, there’s a significant difference in proportions. For instance, if the p-value is 0.01, that means one group significantly prefers their learning method over the other.
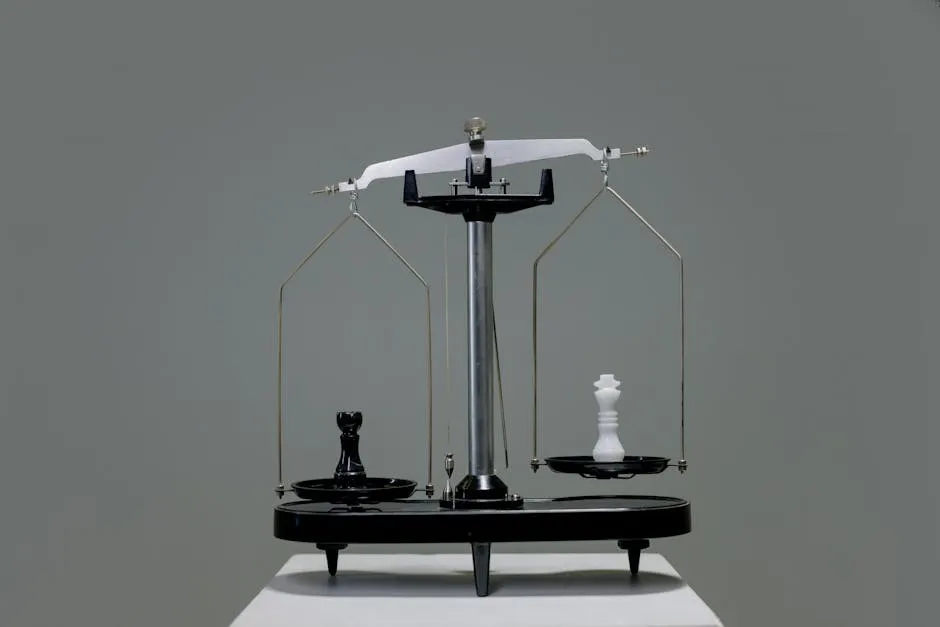
Chi-Square Tests
Chi-Square Goodness of Fit Test
This test checks if a sample distribution matches a theoretical distribution. Think of it as determining if a die is fair!
When to Use: Use the chi-square goodness of fit test when you want to see if observed frequencies match expected frequencies. For example, testing if the number of votes across different candidates is evenly distributed.
Step-by-Step Guide:
- Open StatCrunch: Click the box next to your dataset and select “Open in StatCrunch.”
- Choose the Chi-Square Test: Go to “Stat,” then “Goodness of Fit,” and select “Chi-Square.”
- Input Frequencies: Enter your observed and expected frequencies. For instance:
- Observed: 30, 20, 25, 25
- Expected: 25, 25, 25, 25
- Compute: Click compute! StatCrunch will provide the test statistic and p-value.
- Interpret Results: If your p-value is less than 0.05, the observed distribution differs significantly from expected. A p-value of 0.03 indicates a significant difference.
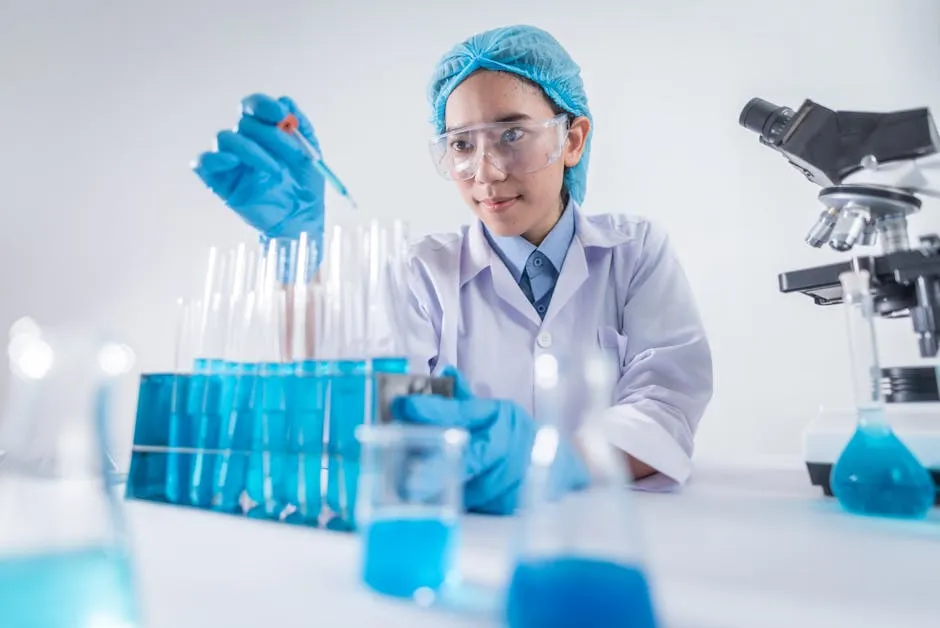
Chi-Square Test of Independence
This test assesses whether two categorical variables are independent. Imagine analyzing if there’s a link between gender and preferred ice cream flavor.
When to Use: Use this test when you want to explore the relationship between categorical variables. For example, checking if students’ major influences their preference for online or in-person classes.
Instructions:
- Open StatCrunch: Click the box next to your dataset and select “Open in StatCrunch.”
- Select the Chi-Square Test: Navigate to “Stat,” then “Tables,” and choose “Contingency.”
- Input Your Data: Create a contingency table with your counts. For example:
| Flavor | Male | Female | |-------------|------|--------| | Vanilla | 20 | 15 | | Chocolate | 10 | 25 |
- Compute: Click compute! StatCrunch calculates the chi-square statistic and p-value.
- Interpret Results: If the p-value is less than 0.05, the variables are dependent. A p-value of 0.04 suggests a significant association between gender and flavor preference.
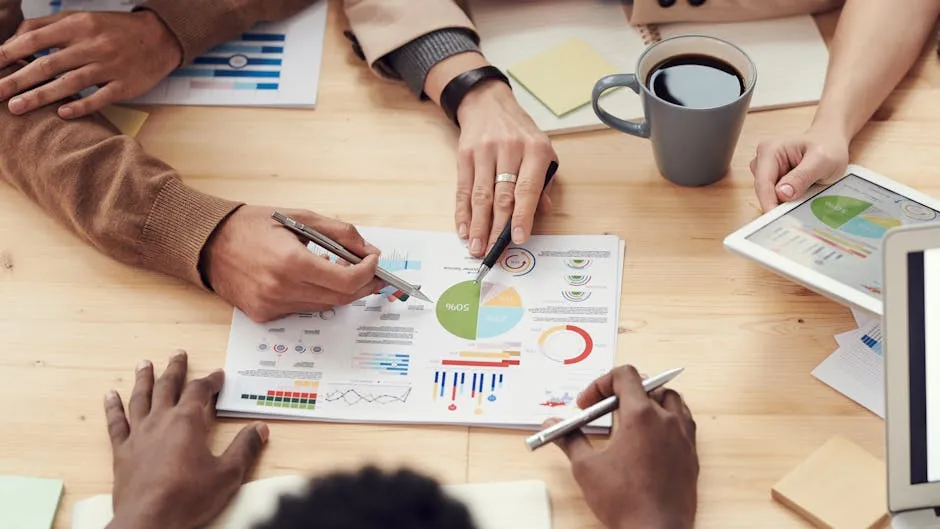
Now you’re all set to perform hypothesis tests using StatCrunch! With these step-by-step guides, you’ll confidently navigate the statistical seas. Happy testing!
Interpreting Results
Understanding Output
Once you’ve performed a hypothesis test in StatCrunch, it’s time to make sense of the output. Think of the results as your trusty map after a long trek—essential for finding your way!
First up, let’s talk about the p-value. This little gem tells you how likely you’d see your results if the null hypothesis were true. A low p-value (typically less than 0.05) suggests that your results are statistically significant. It’s like finding out your favorite pizza joint has a secret sauce—definitely worth noting!
Next, we have the test statistic. This value indicates how far your sample statistic is from the null hypothesis. If it’s a z-test or a t-test, higher absolute values mean stronger evidence against the null hypothesis. Imagine you’re watching a talent show; the more spectacular the performance, the more likely they’ll win!
Lastly, there’s the confidence interval. This range estimates where the true population parameter lies. For example, if your confidence interval for a mean is (5, 10), you can say, “I’m 95% confident the true mean is between 5 and 10.” It’s like peeking through a window to catch a glimpse of what’s going on inside!
By breaking down these components, you can start to grasp what the data is telling you. Each piece is crucial for making informed decisions.
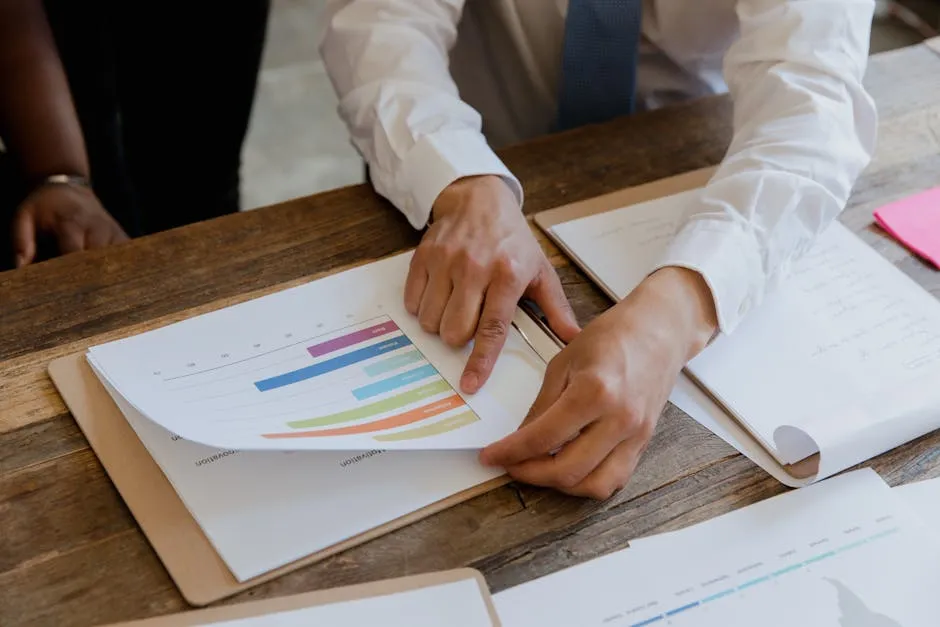
Making Decisions
Now that you’ve deciphered the output, it’s time to make some decisions. Start with the p-value. If it’s below your alpha level (commonly set at 0.05), you can confidently reject the null hypothesis. It’s like saying, “Aha! There’s something interesting happening here!”
But don’t just stop there! Consider your confidence intervals as well. If they don’t include the null hypothesis value, that’s further evidence to reject it. For instance, if you’re testing whether a new teaching method is more effective and your confidence interval for the difference in means doesn’t include zero, you’ve got a winner!
When communicating your findings, clarity is essential. Use straightforward language, and avoid jargon—unless it’s a statistical party, then feel free to show off a bit! Always explain what the p-value means in the context of your study. For example, “Our p-value of 0.03 indicates that the observed difference in test scores is statistically significant.” This helps others understand the importance of your results.
Moreover, visuals can enhance comprehension. Consider using graphs or charts to illustrate your findings. A well-placed bar chart can transform dry statistics into an engaging story!
In summary, make decisions based on not just the p-value but also the context of your data. Explain findings clearly, and support them with visual aids when possible. With these strategies, you’ll communicate your results effectively and keep your audience engaged!
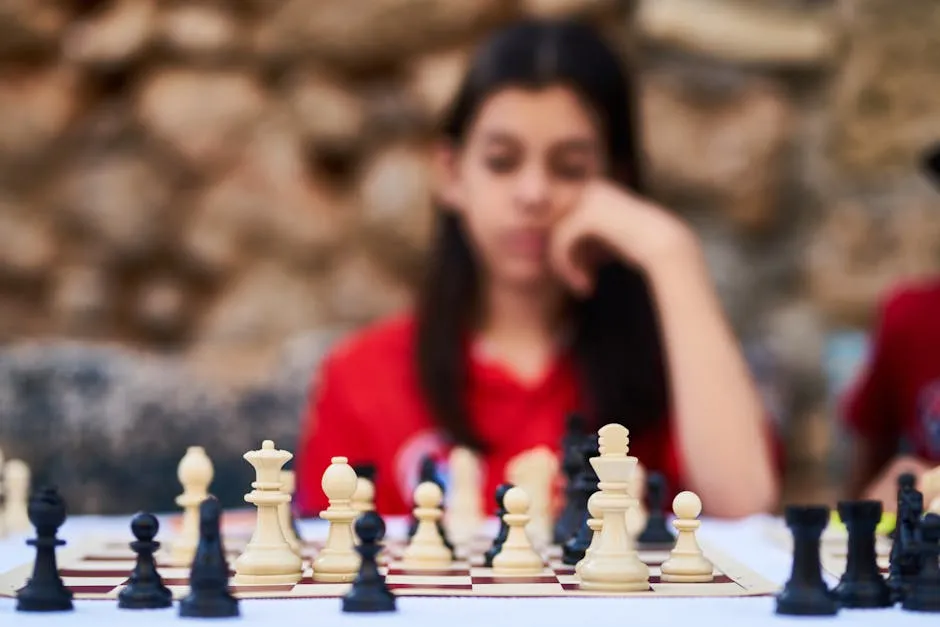
Best Practices and Common Mistakes
Using StatCrunch effectively requires a few best practices. First, always double-check your data before running analyses. A small typo can lead to big misunderstandings. Just like you wouldn’t bake without measuring your ingredients, don’t run tests without verifying your data!
Next, familiarize yourself with the assumptions behind each test. For example, both the t-test and z-test have specific requirements regarding sample size and distribution. Understanding these assumptions ensures you choose the right test, leading to more reliable results.
Now, let’s chat about common mistakes. A frequent blunder is misinterpreting the p-value. Remember, a p-value does not measure the probability that the null hypothesis is true. It merely indicates the likelihood of observing the data if the null is correct. Keeping this straight can save you from a statistical faux pas!
Another common error is neglecting the confidence intervals. Focusing solely on p-values can lead to incomplete conclusions. Always examine confidence intervals to provide a fuller picture of your results.
Finally, avoid the temptation to cherry-pick results. Presenting only the significant findings while ignoring non-significant results can mislead your audience. Transparency fosters trust and integrity in your research.
Implementing these best practices and avoiding common pitfalls will help you navigate the statistical landscape of StatCrunch with confidence and clarity. Happy analyzing!

Conclusion
Understanding test statistics is crucial for data analysis. Test statistics help us evaluate hypotheses and make informed decisions. StatCrunch simplifies this process, making statistical analyses more accessible. With its user-friendly interface, you can perform various tests without feeling lost in the statistical jungle.
StatCrunch allows you to conduct z-tests, t-tests, chi-square tests, and more with just a few clicks. It transforms complex calculations into simple steps, enabling you to focus on interpreting the results. This ease of use is vital for students and professionals alike. They can now harness the power of statistics without needing a Ph.D. in mathematics!
Practicing with StatCrunch is key. The more you use it, the more confident you’ll become. Try different statistical tests on various datasets. Experimenting with real data will help solidify your understanding. Each practice session brings you closer to mastering statistics. You’ll soon navigate through the tests like a pro!
So, don’t hesitate! Open StatCrunch, upload some data, and start testing those hypotheses. With practice, you’ll gain proficiency in data analysis. Before you know it, you’ll be interpreting results and making data-driven decisions like a seasoned statistician. Dive into the world of statistics with StatCrunch and watch your analytical skills flourish!
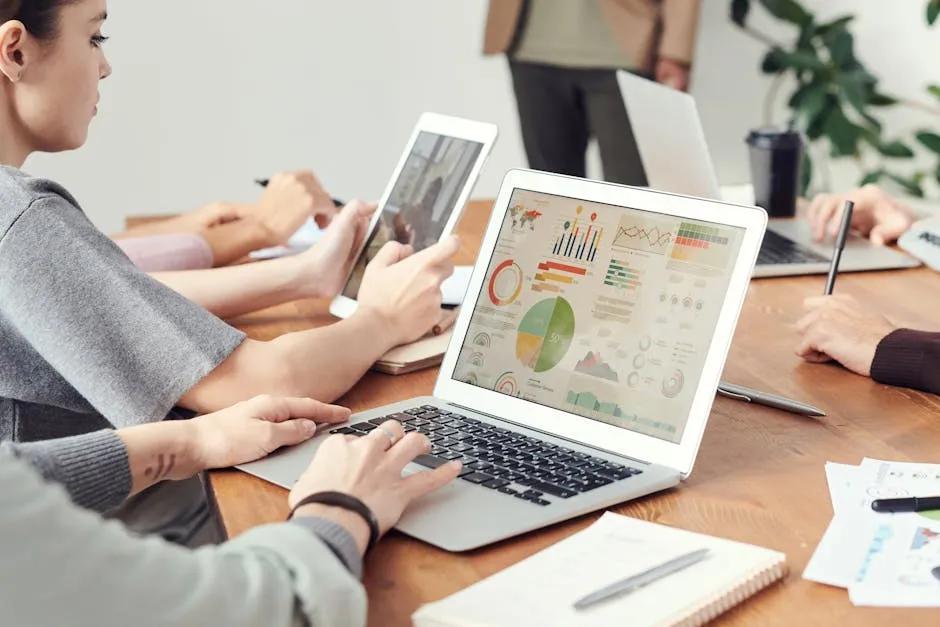
FAQs
What is the difference between a z-test and a t-test?
A z-test is used for larger sample sizes (n > 30) or when the population variance is known. It compares sample means to a population mean. A t-test, on the other hand, is ideal for smaller sample sizes (n < 30) and unknown population variances. It also compares sample means but adjusts for the smaller sample size.
When should I use a chi-square test?
You should use a chi-square test when analyzing categorical data. It helps determine if there’s a significant association between two variables. For example, if you want to assess whether gender influences preferred ice cream flavors, a chi-square test is perfect for the job.
How do I interpret p-values?
P-values indicate the probability of observing your data if the null hypothesis is true. A low p-value (typically less than 0.05) suggests strong evidence against the null hypothesis, meaning you may reject it. Conversely, a high p-value indicates insufficient evidence to reject the null hypothesis.
Can I perform regression analysis in StatCrunch?
Absolutely! StatCrunch allows you to perform regression analysis seamlessly. You can explore relationships between variables and generate regression equations. Just click on “Stat,” then “Regression,” and choose “Simple Linear” to get started.
Is StatCrunch suitable for large datasets?
Yes, StatCrunch is well-equipped to handle large datasets. It efficiently processes data, allowing you to perform complex analyses without lag. Whether you’re dealing with hundreds or thousands of rows, StatCrunch ensures a smooth experience for your statistical needs.
Please let us know what you think about our content by leaving a comment down below!
Thank you for reading till here 🙂
For a detailed overview of hypothesis testing, check out this statistics hypothesis testing cheat sheet.
To understand the various test statistics, you can refer to the test statistics cheat sheet.
Learn how to effectively analyze data with the tips for effective data analysis in economics and statistics.
For a deeper understanding of confidence intervals, see how to calculate test statistic for confidence interval ti84.
To distinguish between practical significance and statistical significance, refer to practical significance versus statistical significance.
If you’re looking to dive deeper into statistics, consider picking up “Statistics for Dummies” by Deborah J. Rumsey. This book breaks down complex concepts into digestible bites, perfect for beginners and experts alike!
All images from Pexels