Introduction
Statistics play a crucial role in our lives. From science to business, it’s everywhere! Whether you’re analyzing data for a marketing campaign or interpreting research findings, statistics are the backbone of informed decision-making. You might wonder, “Why is statistics so important?” Well, it helps us make sense of numbers, identify trends, and draw conclusions from data.
But let’s face it, statistics can sometimes feel like a foreign language. Enter the statistics cheat sheet! This handy tool simplifies complex concepts. It serves as a quick reference, making it easier to grasp essential statistical principles. Imagine having a mini guide in your pocket during exams or data analysis sessions.
In this article, we aim to provide a comprehensive overview of key statistics concepts and their applications. Plus, we’ll offer downloadable PDF resources to help you on your journey. So, whether you’re a student, professional, or just a curious mind, you’ll find something useful here. Get ready to boost your statistical prowess and navigate the world of data with confidence!
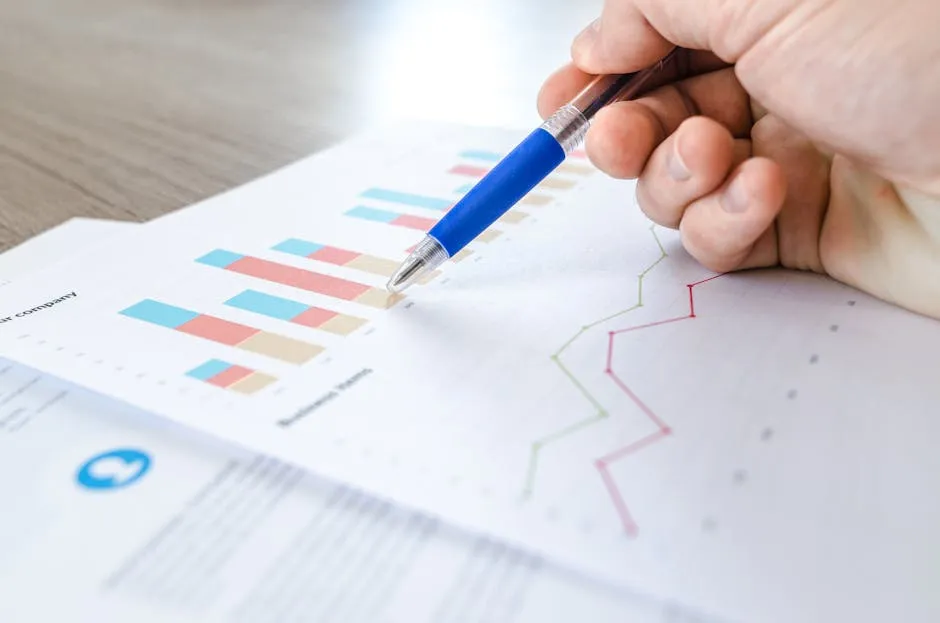
Understanding Statistics
What is Statistics?
Statistics is the science of collecting, analyzing, and interpreting data. It helps us understand patterns and relationships within numerical information. Why is it significant? Because it allows us to make informed decisions based on evidence rather than guesswork.
Statistics finds applications across various disciplines. In science, researchers use statistical methods to validate hypotheses and draw conclusions from experiments. In business, analytics drive marketing strategies and improve customer experience. Economists rely on statistics to forecast economic trends, while social scientists use it to study human behavior.
In the realm of healthcare, statistics are vital for clinical trials, helping determine the efficacy of treatments. Even in sports, statistics help teams analyze performance and develop strategies. The significance of statistics is clear: it’s a universal language for making sense of complex data.
Understanding statistics is essential for anyone working with data. It empowers individuals to interpret findings, validate claims, and ultimately enhance decision-making processes. Whether you’re a seasoned data analyst or a casual learner, grasping the basics of statistics will undoubtedly enrich your understanding of the world. So, let’s dive into the various types of statistics and their applications!
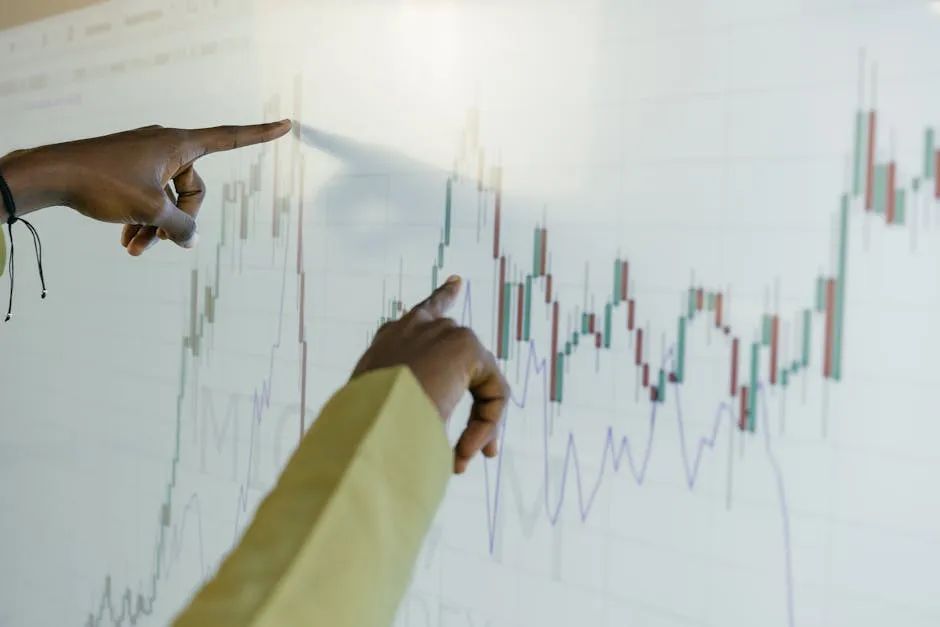
Types of Statistics
Statistics can be categorized into two main types: descriptive and inferential. Each serves a distinct purpose in analyzing data and drawing conclusions.
Descriptive Statistics
Descriptive statistics provide a summary of the data at hand. Think of it as the “CliffNotes” version of a long novel. Its primary goal is to describe and summarize the features of a dataset. It helps us organize and simplify large volumes of data into understandable formats.
Common examples include:
- Mean: This is the average of all values. You calculate it by adding all numbers and dividing by their count. For instance, if you have test scores of 70, 80, and 90, the mean is (70 + 80 + 90) / 3 = 80. Learn more about mean and its significance in statistics.
- Median: This is the middle value when numbers are sorted in order. For example, in the set 3, 5, 7, and 9, the median is 6. If the numbers are even, like 3, 5, and 7, the median is the average of the two middle values (5 and 7), resulting in 6. Explore median statistics in Poland for 2024.
- Mode: This refers to the most frequently occurring value in a dataset. If you have the numbers 1, 2, 2, 3, and 4, the mode is 2, since it appears most often. Learn more about mode and its applications.
Understanding the concept of mean is essential for statistical analysis. what does mean identically distributed in statistics
Median is a crucial measure of central tendency that provides insights into data distribution. statistics poland median salary 2024
The mode is an important statistical measure that can highlight trends in data. statsmodels residuals statistics
Descriptive statistics help researchers and analysts get a quick grasp of the data’s characteristics without diving into complex analyses. If you’re looking to dive deeper into statistical concepts, consider picking up “Naked Statistics: Stripping the Dread from the Data” by Charles Wheelan. It’s a delightful read that makes statistics approachable and entertaining!
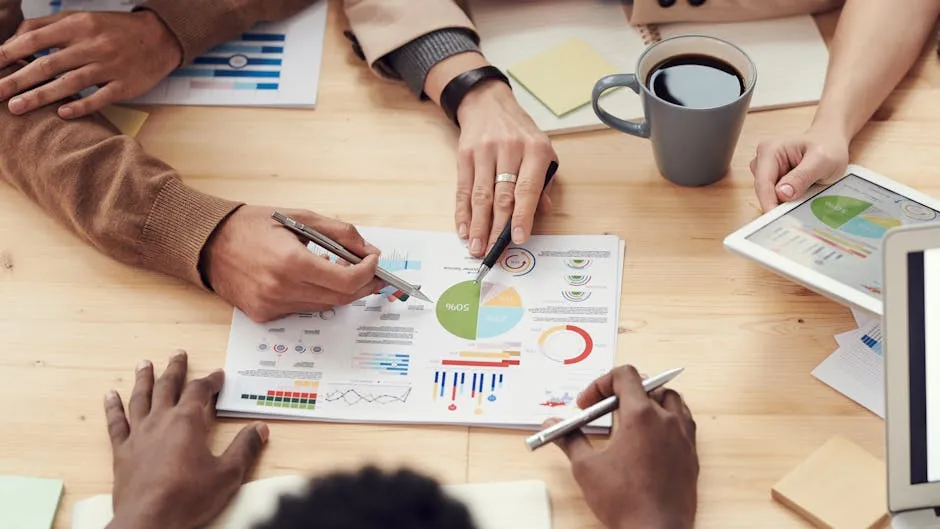
Inferential Statistics
Inferential statistics take things a step further. They allow us to make predictions or generalizations about a larger population based on a smaller sample. Imagine tossing a coin 10 times and concluding that it will likely land heads up in future tosses. That’s inferential statistics at work!
This type of statistics is crucial for research and surveys. For example, if a company surveys 1,000 consumers about a new product, they can infer how the entire customer base might react based on this sample.
Another common application is in clinical trials, where researchers test a new drug on a small group. They then use inferential statistics to predict how the drug will perform in the general population.
In summary, both descriptive and inferential statistics are vital. Descriptive statistics summarize data, while inferential statistics allow us to make broader conclusions based on that data. Together, they form the backbone of data analysis, enabling informed decision-making across various fields.
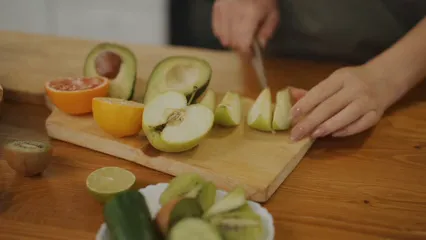
Measure of Dispersion
Range
The range is the simplest measure of dispersion. It gives you the difference between the highest and lowest values in a dataset. Think of it as the “stretch” of your data.
For example, if you have test scores of 55, 68, 74, 88, and 95, the range is calculated as follows:
Range = Highest Score – Lowest Score
Range = 95 – 55 = 40
So, the range of these test scores is 40. It’s like saying, “Hey, the scores vary by 40 points!” Easy, right?
However, the range can be a bit misleading. Why? Because it only considers extremes and ignores the values in between. If you have outliers, they can skew your range. Like when your friend brings home a 100 on an exam, and suddenly, the average score seems way off.
For a more comprehensive approach, consider the graphing calculator. It’s a handy tool for visualizing data and performing complex calculations that can help you understand your statistics better!
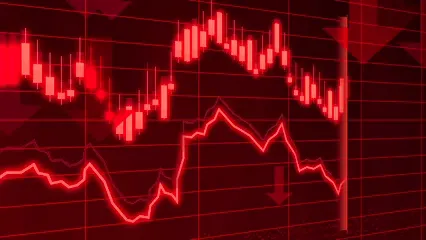
Variance and Standard Deviation
Variance and standard deviation are the dynamic duo of statistics. They help you understand how much data varies from the mean.
Variance measures the average squared deviation from the mean. The formula is:
Variance (σ²) = Σ (X – μ)² / N
Where:
– X represents each value in the dataset.
– μ is the mean of the dataset.
– N is the number of observations.
Let’s say we have the numbers: 2, 4, 4, 4, 5, 5, 7, 9. The mean (μ) is 5. The variance is calculated as:
Variance = [(2-5)² + (4-5)² + (4-5)² + (4-5)² + (5-5)² + (5-5)² + (7-5)² + (9-5)²] / 8
Variance = [9 + 1 + 1 + 1 + 0 + 0 + 4 + 16] / 8
Variance = 32 / 8 = 4
Now, the standard deviation is simply the square root of the variance, providing a more intuitive measure of spread:
Standard Deviation (σ) = √Variance
Standard Deviation = √4 = 2
This means, on average, your data points deviate by 2 units from the mean. Standard deviation is super helpful in data analysis. It tells you how consistent your data is. A low standard deviation means your data points are close to the mean, while a high one indicates they’re spread out.
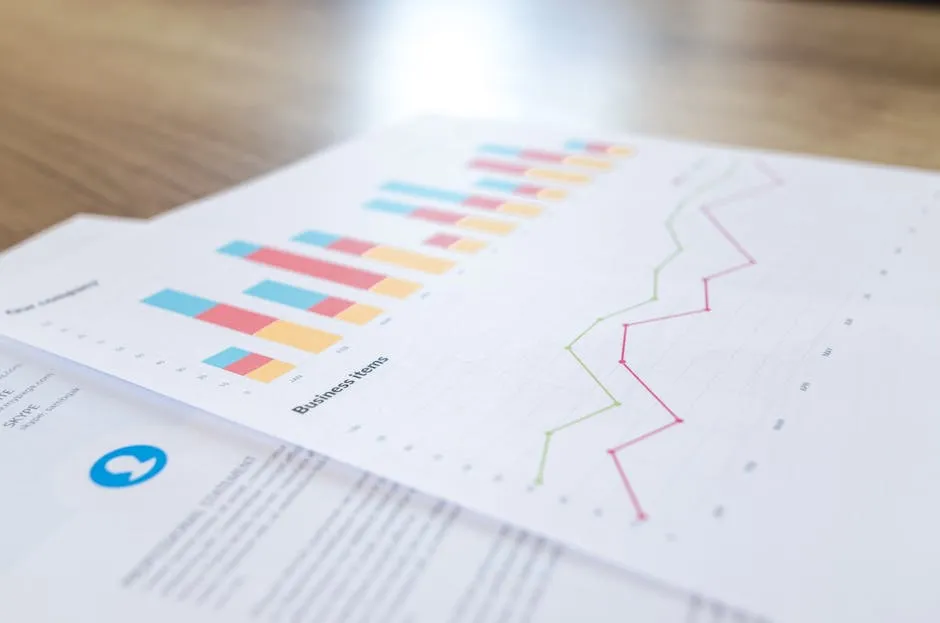
Interquartile Range (IQR)
The Interquartile Range (IQR) is another important measure of dispersion. It shows you the range of the middle 50% of your data. This makes it resistant to outliers, giving a clearer picture of data distribution.
To calculate IQR, follow these steps:
- Order your data: For example, consider the dataset: 1, 3, 5, 7, 9, 11, 13, 15.
- Find Q1 and Q3:
– Q1 (the first quartile) is the median of the first half of the dataset. In our case, Q1 is 4.
– Q3 (the third quartile) is the median of the second half. Here, Q3 is 12. - Calculate IQR:
IQR = Q3 – Q1
IQR = 12 – 4 = 8
This tells you that the middle 50% of the data lies within a range of 8 units. The IQR is particularly useful in identifying outliers. If a value falls below Q1 – 1.5*IQR or above Q3 + 1.5*IQR, it’s considered an outlier. It’s a great way to keep your data tidy and focused!
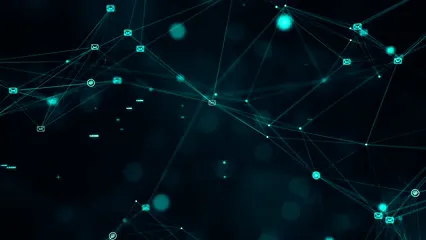
Measure of Shape
Skewness
Skewness is a measure of the asymmetry of a data distribution. It tells you whether your data leans towards the left or right side of the mean.
A positively skewed distribution has a long tail on the right side. This means there are a few high values pulling the average up. Think of income distributions where a handful of people earn way more than everyone else.
Conversely, a negatively skewed distribution has a long tail on the left side. This indicates that most data points are high, but a few low values drag the average down.
Understanding skewness helps in data analysis, as it can affect statistical tests and interpretations. If your data is skewed, you might want to consider using median and IQR instead of mean and standard deviation.
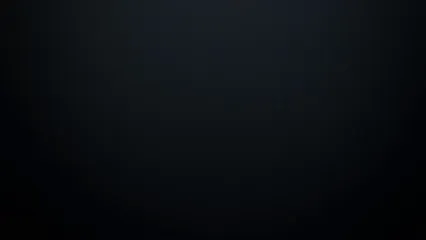
Kurtosis
Kurtosis measures the “tailedness” of a distribution. It helps you understand the shape of the data’s distribution compared to a normal distribution.
There are three types of kurtosis:
- Mesokurtic: This is the baseline, resembling a normal distribution. Its kurtosis value is 3.
- Leptokurtic: These distributions have fatter tails and sharper peaks. This means more data is concentrated around the mean, with a higher likelihood of extreme values. The kurtosis score is greater than 3.
- Platykurtic: In this case, the distribution has thinner tails and a flatter peak. Here, data is spread out more evenly, producing a kurtosis score less than 3.
Kurtosis is significant because it can indicate the risk of outliers in your data. Higher kurtosis implies a higher risk of extreme values, which could impact your analyses. Understanding these shapes allows you to better interpret your data and apply the correct statistical methods.
And there you have it! An engaging yet detailed look into measures of dispersion and shape. Understanding these concepts will make you a more informed data analyst, ready to tackle any dataset that comes your way!
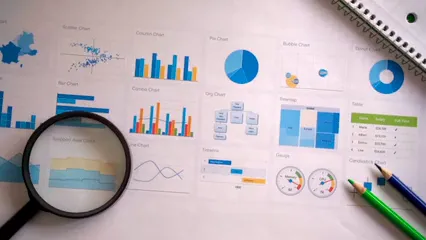
Probability Theory
Basic Concepts
Probability theory is the mathematical framework for quantifying uncertainty. To grasp this, let’s start with some key terms.
Sample Space: This is the set of all possible outcomes of a random experiment. Think of it as a buffet of potential results waiting for you to sample. For example, when flipping a coin, the sample space consists of two outcomes: heads (H) and tails (T).
Events: An event is a subset of the sample space. It can be as simple as getting heads when flipping that coin, or more complex, like rolling an even number on a six-sided die.
Outcomes: These are the specific results of an experiment. In our coin flip, getting heads is one outcome, while tails is another.
Now that we’ve defined our terms, let’s jump into some fundamental probability rules.
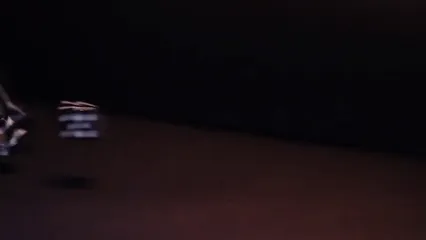
Addition Rule
This rule helps us calculate the probability of the occurrence of at least one of several events. For instance, if you want to know the probability of rolling a 2 or a 4 on a die, you simply sum the probabilities of each event:
P(2 or 4) = P(2) + P(4)
If the events are mutually exclusive (they can’t happen at the same time), this works perfectly. However, if they can occur together, you need to subtract the probability of their intersection.
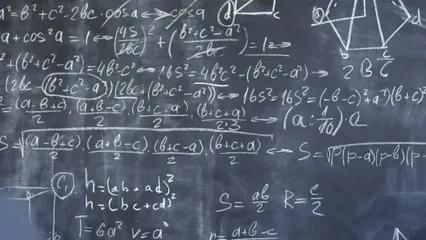
Multiplication Rule
This rule applies when determining the probability of two events happening together. If you want to know the chance of rolling a 3 and then flipping heads, you multiply the probabilities of each independent event:
P(3 and H) = P(3) × P(H)
It’s vital to note that this rule only applies to independent events, meaning the occurrence of one does not affect the other.
In summary, understanding these basic concepts is essential for grasping the later complexities in probability theory. These concepts not only make sense of numerical information but also empower decision-making based on statistical evidence.
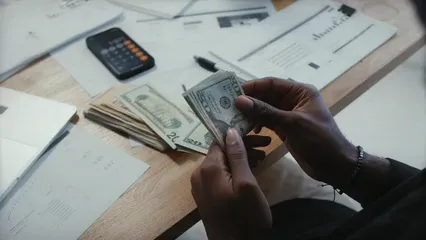
Probability Distributions
Normal Distribution
The normal distribution, often dubbed the “bell curve,” is a cornerstone of probability theory. Its curve is symmetric, centering on the mean. What makes it special? Many natural phenomena, from heights to test scores, tend to follow this distribution.
Characteristics of the normal distribution include:
- Symmetry: The left side mirrors the right side.
- Mean, Median, and Mode: These measures of central tendency are all equal.
- 68-95-99.7 Rule: About 68% of data falls within one standard deviation from the mean. About 95% falls within two, and nearly all (99.7%) falls within three.
Applications of the normal distribution span various fields, including psychology, finance, and quality control. It aids in understanding variability and making predictions.
For those looking to master the intricacies of statistics, consider reading “The Art of Statistics: Learning from Data” by David Spiegelhalter. It’s a fantastic resource that demystifies statistical concepts!

Other Distributions
Besides the normal distribution, there are other important probability distributions to consider.
Binomial Distribution
This distribution models the number of successes in a fixed number of independent trials. For example, if you flip a coin ten times, you might want to know the probability of getting exactly three heads. It’s defined by two parameters: the number of trials (n) and the probability of success (p).
The formula for calculating binomial probabilities is:
P(X=k) = \(\binom{n}{k} p^k (1-p)^{n-k}\)
where \(\binom{n}{k}\) is a binomial coefficient.
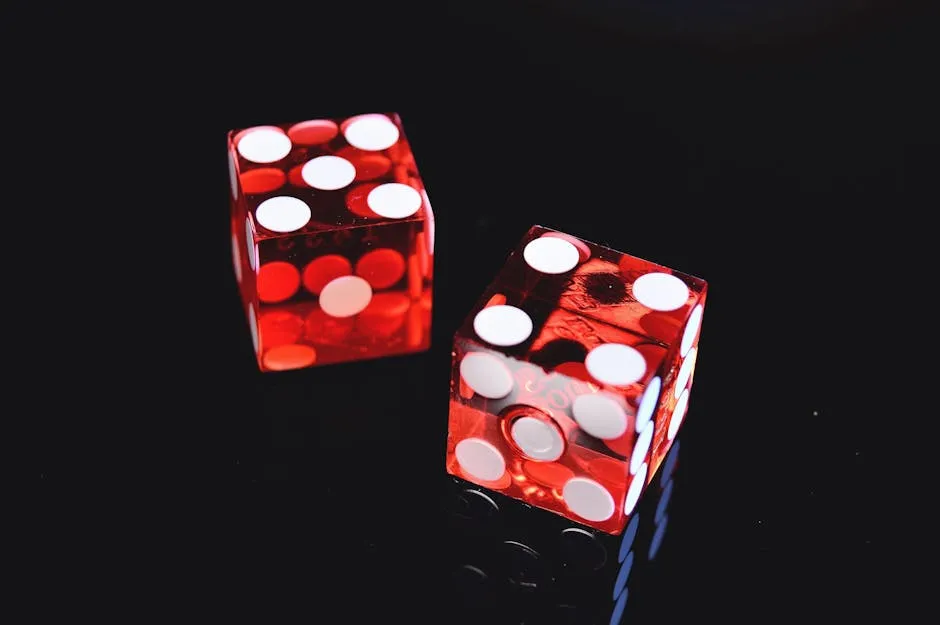
Poisson Distribution
This distribution applies to rare events in a given interval of time or space. For instance, it can model the number of cars passing through a toll booth in an hour. The formula is:
P(X=k) = \(\frac{\lambda^k e^{-\lambda}}{k!}\)
where \(\lambda\) is the average rate of occurrence.
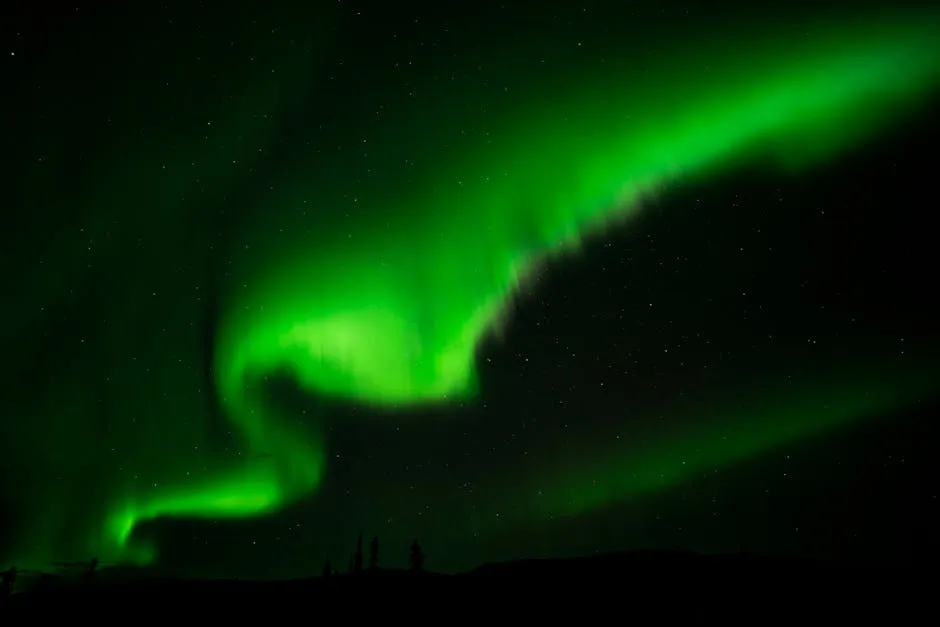
Uniform Distribution
In this distribution, every outcome is equally likely. Imagine rolling a fair die; each number from 1 to 6 has the same chance of appearing. The probability density function is flat, indicating that all outcomes are equally probable.
These distributions offer valuable insights into various scenarios. Understanding them is crucial for data analysis, enabling analysts to interpret results and draw conclusions effectively. Whether you’re predicting sales, analyzing survey results, or conducting experiments, these distributions are your trusty tools in the statistics toolbox.
Practical Applications of Statistics
A/B Testing
A/B testing, also known as split testing, is a powerful method used to compare two versions of something. Think of it as a friendly competition between two ideas. Marketers and product developers leverage A/B testing to determine which version performs better.
How does it work? You take two variations—let’s call them A and B. Each variation gets presented to a segment of your audience. You then analyze which version achieves better results based on specific metrics—like click-through rates or conversion rates.
Why is A/B testing so crucial? In the fast-paced world of marketing, making informed decisions can save time and resources. Instead of guessing what works, you can rely on data to guide your choices. This is especially valuable in product development, where testing features can lead to enhancements that users actually want.
Imagine launching a new website. You might want to test different headlines or images. By running an A/B test, you can see which elements resonate more with your visitors. This data-driven approach leads to higher user engagement and potentially increased sales.
In summary, A/B testing is about making decisions based on evidence rather than intuition. It’s an essential tool in the marketer’s toolkit, enabling the optimization of campaigns and products by directly observing user behavior.

Regression Analysis
Regression analysis is a statistical method that examines relationships between variables. It helps determine how the value of one variable changes when another variable is altered. This method is key in predicting outcomes and understanding dependencies.
At its core, regression answers the question: “How does variable Y change when variable X changes?” For example, if you’re a marketer, you might want to know how advertising expenditure influences sales. A regression analysis can provide insights into this relationship.
There are two primary types of regression: simple and multiple.
- Simple Regression involves two variables: one independent and one dependent. For instance, if you’re analyzing how temperature impacts ice cream sales, your independent variable is temperature, while sales are the dependent variable. The formula for a simple regression model looks like this:
y = α + βx + e
Here, y is the predicted variable, x is the explanatory variable, and e represents the error term.
- Multiple Regression takes it a step further, involving multiple independent variables. For example, if you want to predict sales based on temperature, advertising spend, and day of the week, you’d use multiple regression. The model can be represented as:
y = α + β1x1 + β2x2 + … + βnxn + e
This allows for a more nuanced understanding of how different factors impact an outcome.
Regression analysis is essential in various fields, from economics to healthcare. In finance, it can predict stock prices based on economic indicators. In healthcare, it might model how different treatments affect patient outcomes.
To summarize, regression analysis is a fundamental tool for understanding relationships between variables. It empowers decision-makers by providing insights that can lead to better strategies and outcomes in marketing, product development, and beyond. For more in-depth knowledge, consider exploring “How to Measure Anything: Finding the Value of ‘Intangibles’ in Business” by Douglas W. Hubbard. It’s a great resource to understand the practical application of statistical analysis!
Please let us know what you think about our content by leaving a comment down below!
Thank you for reading till here 🙂
All images from Pexels