Understanding Contingency Analysis Statistics
A contingency table, often termed a crosstab or two-way table, is your go-to tool in statistics for visualizing the relationship between two categorical variables. Picture it as a grid: rows represent one variable while columns represent another. Each cell in the table indicates the frequency of observations corresponding to the variable combinations.
Imagine you’re studying the relationship between coffee preference (Espresso or Latte) and time of day (Morning or Evening). Your contingency table would neatly display how many people prefer each coffee type during the specified times.
Key terms in this context include marginal totals and cell values. Marginal totals are the sums of the rows and columns. They give you a sneak peek into the overall distribution of each variable independently. Cell values, on the other hand, represent the frequency of each combination of variables.
By organizing data this way, you can easily spot patterns, trends, and associations. This method shines in survey research, market analysis, and social sciences, where categorical data reigns supreme.
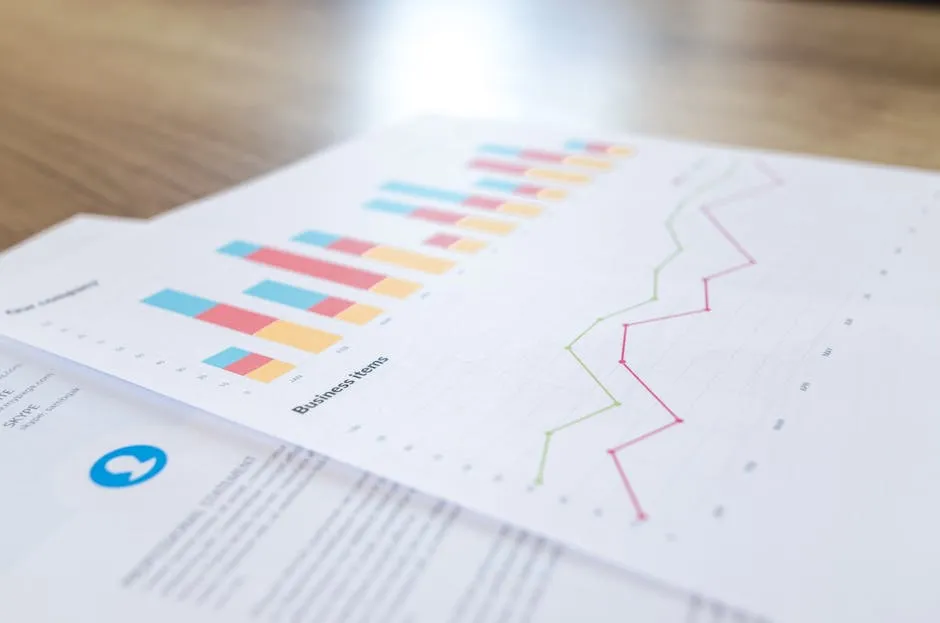
And speaking of data analysis, if you want to delve deeper into the world of statistics, grab a copy of Contingency Table Statistics Book. It’s like having a personal tutor guiding you through the intricacies of categorical data analysis!
Understanding how to utilize contingency tables can greatly enhance your data analysis skills. contingency table statistics
The Anatomy of a Contingency Table
Let’s break down the elements of a contingency table. Imagine a simple table comparing pet ownership (Dog, Cat) and household type (Apartment, House).
– Rows and Columns: The rows represent one category (e.g., Pet Type) while the columns represent another (e.g., Household Type). This layout allows you to analyze how each category interacts.
– Cell Counts: These numbers are the stars of the show! Each cell tells you how many observations belong to that specific combination of categories. For instance, if you find that 10 households with dogs live in apartments, that’s a key insight.
– Marginal Distributions: Want to know the total number of households with dogs? Look at the marginal totals! They provide the sums of each row and column. For instance, you may find that out of 100 households, 40 have dogs, and 60 have cats.
– Conditional Distributions: These distributions tell you how one variable behaves when you fix the other variable. For example, if you focus only on apartments, you can see the distribution of pet types specifically within that group.
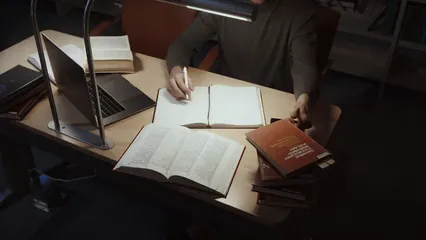
These elements make contingency tables powerful tools for data analysis. They allow you to not just observe but also interpret the relationships between your variables, making sense of complex datasets in a digestible format.
By mastering contingency tables, you unlock the potential to understand intricate data relationships and make informed decisions based on statistical evidence. So, whether you’re analyzing consumer behavior or studying social trends, these tables will be your trusty allies in the quest for insights!
Types of Contingency Tables
Two-way vs. Multi-way Tables
Contingency tables come in various shapes and sizes, mainly two-way and multi-way tables. Let’s unravel the differences, shall we?
Two-way tables are the simplest variety. They display the relationship between two categorical variables. Picture a classic school project where students are grouped by favorite fruit (Apples, Oranges) and snack preference (Chips, Nuts). Each cell in the table tells you how many students prefer a specific fruit with a particular snack. Simple, right?
Now, imagine we crank up the complexity. Multi-way tables take this to another level by including three or more variables. Think of a multi-way table analyzing age groups, gender, and favorite fruit. Now you have a delightful matrix that can show preferences across multiple categories!
But why stop there? Researchers use multi-way tables to explore intricate relationships in various fields. For example, in public health, a multi-way table might analyze how exercise frequency correlates with age, gender, and health conditions. This helps professionals pinpoint trends and target interventions effectively.
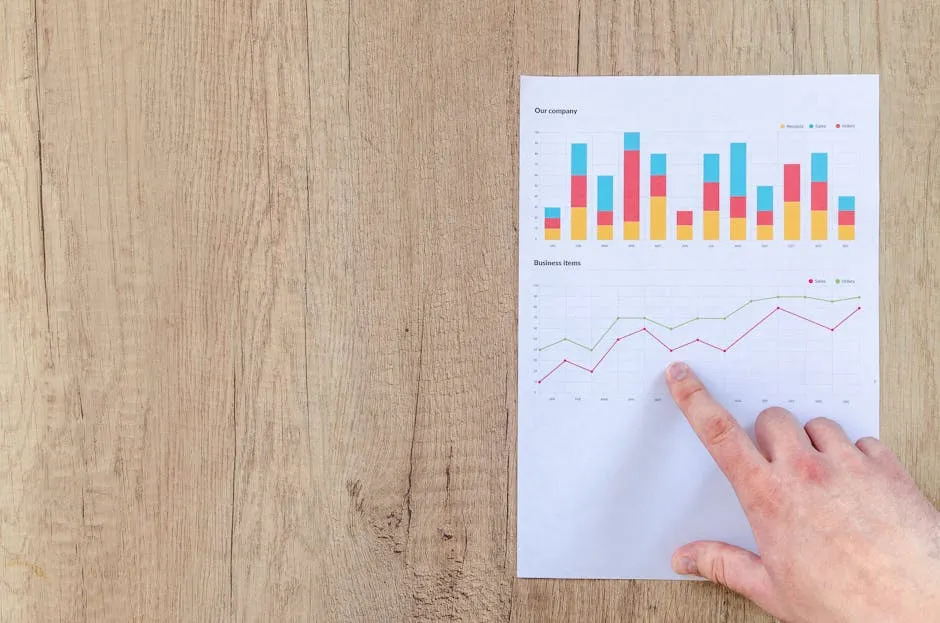
In essence, two-way tables provide straightforward insights, while multi-way tables offer a treasure trove of data relationships. Whether you’re conducting market research or public health analysis, understanding these tables enhances your analytical skills. So, which table will you use for your next data adventure?
Analyzing Contingency Tables
Constructing a Contingency Table
Step-by-Step Guide
Creating a contingency table from raw data is like assembling a jigsaw puzzle. Follow these steps, and you’ll have a masterpiece in no time!
1. Gather Your Data: Start with a dataset that includes two categorical variables. For instance, let’s say you want to analyze survey responses about pet ownership based on age groups.
2. Organize Your Variables: Identify your variables. In our case, they are “Pet Type” (Dog, Cat) and “Age Group” (Under 30, 30-50, Over 50).
3. Create Rows and Columns: Draw a grid. The rows will represent one variable (e.g., Pet Type), while the columns represent the other variable (e.g., Age Group).
4. Fill in the Data: Count how many respondents fall into each category combination. For example, if 15 people under 30 own dogs, you’ll place that number in the corresponding cell.
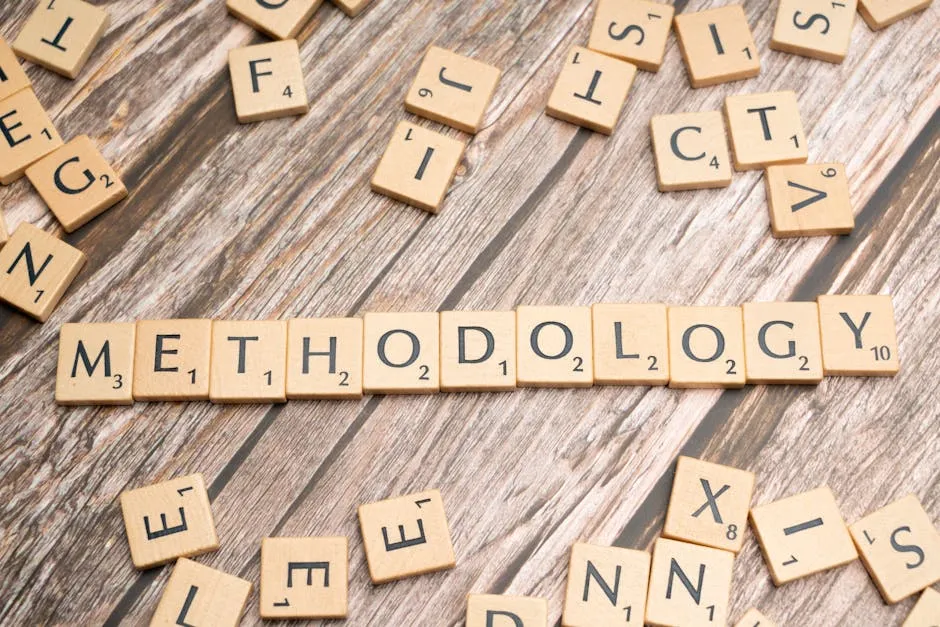
5. Calculate Marginal Totals: Sum up the counts for each row and column. This helps you see the overall distribution of responses.
6. Double-check for Accuracy: Review your entries. Accuracy is key! Mistakes can lead to misinterpretations.
7. Analyze Your Table: Once your table is complete, you can analyze the relationships between the variables. Ask questions like, “Do younger people prefer dogs over cats?”
By following these steps, you’ll ensure your contingency table is an accurate reflection of your dataset. Now go ahead, build your table, and uncover the hidden stories behind your data!
And while you’re at it, why not enhance your analytical skills with a Excel for Data Analysis Book? It’s the perfect companion to help you crunch those numbers like a pro!
Calculating Probabilities Using Contingency Tables
Joint, Marginal, and Conditional Probabilities
Probabilities are the icing on the cake when analyzing contingency tables. Let’s break down the three main types: joint, marginal, and conditional probabilities.
Joint Probability refers to the likelihood of two events occurring together. For example, if you have a table showing pet ownership by age group, the joint probability of someone being under 30 and owning a dog can be calculated by dividing the count in that cell by the total number of respondents.
Marginal Probability looks at the likelihood of a single event without considering any other variables. To find the marginal probability of owning a dog, sum up all the counts in the dog row and divide by the total number of respondents. This shows you the overall preference for dogs, regardless of age.
Conditional Probability focuses on the probability of an event given that another event has already occurred. For instance, if you want to know the probability of owning a dog given that the respondent is under 30, divide the joint probability of being under 30 and owning a dog by the marginal probability of being under 30.
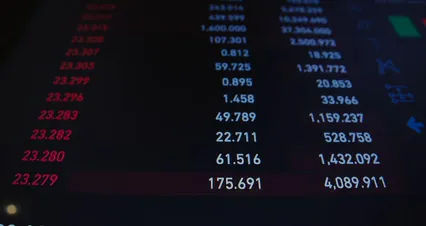
Let’s consider a real-world example. Suppose your table reveals that 20 out of 100 respondents under 30 own dogs. Your calculations would look something like this:
– Joint Probability (Under 30 and Dog) = 20/100 = 0.2
– Marginal Probability (Dog) = (20 + other dog owners) / 100
– Conditional Probability (Dog | Under 30) = 20/ (total under 30 respondents)
By mastering these probabilities, you can uncover significant insights and trends from your contingency tables. So, roll up your sleeves and get calculating!
Statistical Tests for Independence
Chi-Square Test
The Chi-Square test is your trusty sidekick when assessing relationships between categorical variables in contingency tables. It’s like examining the dance between variables to see if they’re truly in sync or just moving independently.
To perform a Chi-Square test, follow these steps:
1. Set Up Your Hypotheses: Start with a null hypothesis (H0) that states there’s no association between the variables. The alternative hypothesis (H1) suggests an association exists.
2. Calculate Expected Frequencies: For each cell in your table, compute the expected frequency using the formula: (Row Total * Column Total) / Grand Total. This gives you the number of observations you would expect if there were no association.
3. Compute the Chi-Square Statistic: Use the formula:
\[ \chi^2 = \sum \frac{(O – E)^2}{E} \]
Here, O represents the observed frequency, and E is the expected frequency. Sum this for all cells.
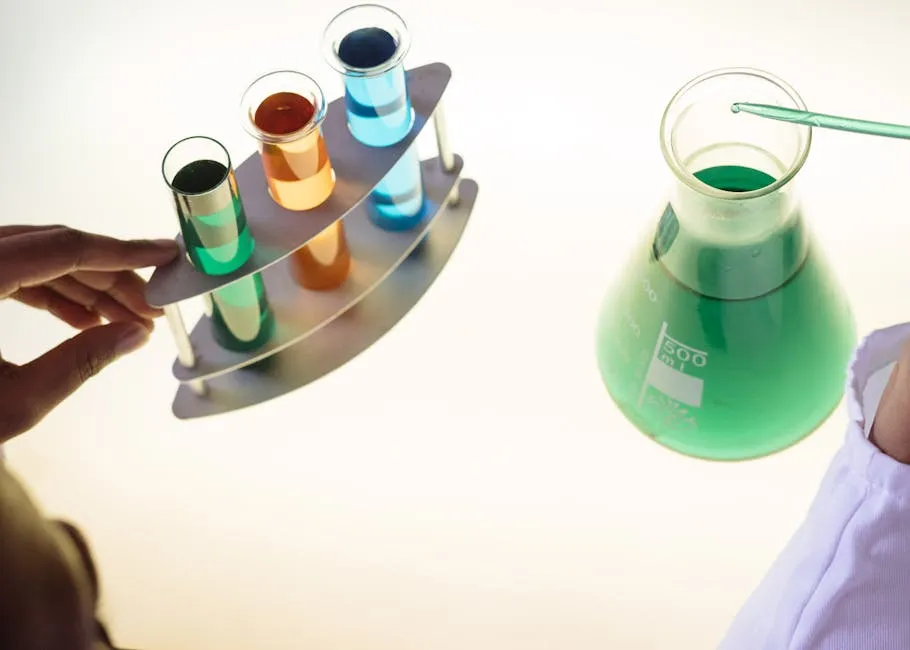
4. Determine Degrees of Freedom: Calculate this as (number of rows – 1) * (number of columns – 1). This helps you find the critical value for your test.
5. Find the P-value: Using a Chi-Square distribution table, compare your calculated statistic to the critical value. The P-value indicates whether the result is statistically significant.
6. Interpret Results: A significant result (typically P < 0.05) means you reject the null hypothesis, suggesting an association between the variables. If not, the variables are likely independent.
By following these steps, you’ll master the Chi-Square test and uncover the hidden relationships in your data. Remember, statistical significance doesn’t imply causation, so keep your detective hat on and interpret your results wisely!
Measures of Association
Understanding Association Strength
When it comes to analyzing categorical data, understanding the strength of association between variables is crucial. In this realm, several measures stand out: the Odds Ratio, Phi Coefficient, and Cramér’s V. Let’s break them down one by one.
Odds Ratio (OR)
The Odds Ratio is a favorite among statisticians. It helps to compare the odds of a certain outcome occurring in one group versus another. To calculate it, you need a 2×2 contingency table. For instance, imagine you’re studying the effect of a new diet on weight loss among men and women.
| | Weight Loss | No Weight Loss | Total |
|—————-|————-|—————-|——-|
| Men | 30 | 70 | 100 |
| Women | 50 | 50 | 100 |
Here, the Odds for men losing weight is 30/70, while for women, it’s 50/50. The Odds Ratio can be computed as:
\[ \text{OR} = \frac{\text{Odds (Men)}}{\text{Odds (Women)}} = \frac{30/70}{50/50} = \frac{30 \times 50}{70 \times 50} = \frac{30}{70} \approx 0.43 \]
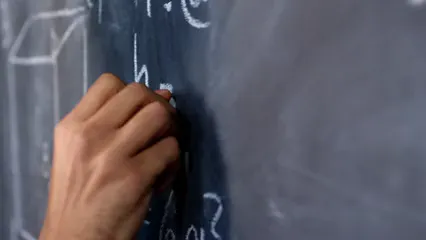
An OR of less than 1 suggests that men are less likely to lose weight compared to women. An OR of 1 indicates no difference, while more than 1 means men fare better in weight loss.
Phi Coefficient (φ)
Next up is the Phi Coefficient, which is perfect for 2×2 tables. It measures the degree of association between two binary variables. The formula is:
\[ \phi = \sqrt{\frac{\chi^2}{N}} \]
Where \( \chi^2 \) is the chi-squared statistic from the contingency table and \( N \) is the total number of observations.
Let’s say the chi-squared value calculated from the previous table is 10. The total number of observations is 200. Plugging these into the formula gives:
\[ \phi = \sqrt{\frac{10}{200}} = \sqrt{0.05} \approx 0.224 \]
A Phi coefficient of 0.224 indicates a moderate association; values range from -1 to 1, where 0 means no association and 1 or -1 indicates a perfect positive or negative association, respectively.
Cramér’s V
For tables larger than 2×2, Cramér’s V is your go-to measure. It assesses the strength of association between two nominal variables. The formula is:
\[ V = \sqrt{\frac{\chi^2}{N \cdot (k – 1)}} \]
Here, \( k \) is the smaller of the number of rows or columns. If we take the previous example, suppose you find \( \chi^2 \) to be 15 and you have a 2×2 table (so \( k = 2 \)):
\[ V = \sqrt{\frac{15}{200 \cdot (2 – 1)}} = \sqrt{\frac{15}{200}} = \sqrt{0.075} \approx 0.273 \]
Cramér’s V values range from 0 to 1. A higher value suggests a stronger association. In our scenario, a value of 0.273 indicates a moderate relationship between the diet and weight loss across genders.
These measures—Odds Ratio, Phi Coefficient, and Cramér’s V—serve as essential tools for interpreting the strength of associations in categorical data analysis. By applying these calculations, you’re empowered to make more informed conclusions about the relationships in your data!
Social Science Research
Analyzing Relationships in Public Surveys
When researchers dive into public surveys, they often encounter a sea of categorical data. Here, contingency tables become their trusty lifebuoys. By laying out survey results in a clear grid format, these tables allow researchers to interpret relationships between variables effectively.
Imagine a survey exploring the connection between education level and voting preference. A contingency table might reveal how many college graduates prefer one political party over another. This visual format makes it easy to spot trends and patterns that might otherwise slip through the cracks.
But how do researchers actually use these tables? They start by populating the rows and columns with variables of interest. In our example, one axis could represent education levels (high school, undergraduate, graduate), while the other displays voting preferences (Party A, Party B). Each cell in the table then reflects the number of respondents fitting that specific combination.
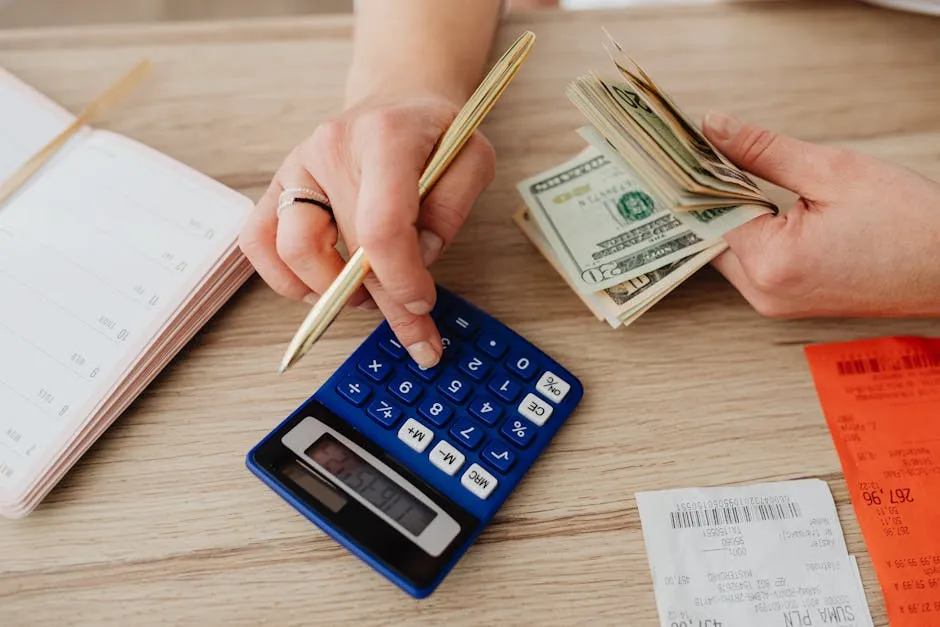
Once the table is filled, researchers can calculate marginal totals. These totals summarize the overall counts for each category, providing insights at a glance. For example, a researcher might discover that a higher percentage of college graduates support Party A than those with just a high school diploma.
Next comes the fun part: statistical analysis. By applying chi-square tests to the data in the contingency table, researchers can assess whether the observed relationships are statistically significant. A significant result implies that education and voting preference are linked, while a non-significant result suggests independence between the two variables.
This analysis isn’t just academic—it’s a powerful tool for understanding societal trends. Public policy makers, for example, can use these insights to tailor initiatives that cater to specific educational demographics.
Moreover, the ability to visualize complex relationships in a straightforward manner makes contingency tables invaluable. They allow for easy comparisons, revealing nuances in public opinion that can shape future decisions.
In summary, contingency tables serve as a vital instrument in social science research. They simplify the process of interpreting categorical data and provide solid statistical backing for conclusions drawn from public surveys. By leveraging these tables, researchers can uncover insights that drive discussions around education, politics, and much more.
And if you’re a pet owner looking to understand your furry friends better, check out this Pet Care Book. It’s a great resource for ensuring your pets live their best lives!
For those interested in higher education and its significance in social science research, consider exploring a master of science in applied statistics for social science research.
Conclusion
Contingency analysis holds immense value in statistics, bridging gaps across various fields. Whether you’re analyzing consumer behavior, public opinion, or health data, these techniques illuminate hidden patterns. We encourage you to implement contingency analysis in your own projects. You might just uncover insights that transform your understanding of your data!
And speaking of transforming your data experience, if you want to dive into the world of data science, check out Data Science for Dummies Book. It’s a great starting point for anyone looking to get their feet wet in the vast ocean of data!
FAQs
What is the main purpose of a contingency table?
To summarize the relationship between two categorical variables, allowing for easy analysis of their association.
How do you interpret the results of a chi-square test?
A significant result (typically a P-value < 0.05) indicates a relationship between the variables, while a non-significant result suggests independence.
Can contingency tables be used for more than two variables?
Yes, multi-way contingency tables can analyze the relationship between three or more categorical variables. Think of it as expanding your party guest list! If you’re tracking preferences based on age, gender, and favorite drink, a multi-way table is your best friend.
What are some common pitfalls when using contingency analysis?
Contingency analysis sounds fancy, but it has its traps! Here are three common pitfalls to watch out for: 1. Misinterpreting correlation as causation: Just because two variables are related doesn’t mean one causes the other. 2. Overlooking sample size considerations: Small sample sizes can lead to misleading results. 3. Neglecting to check assumptions for statistical tests: Every statistical test has assumptions. Avoiding these pitfalls ensures your analysis is robust and credible.
How can I visualize the data from a contingency table?
Visualizing data from a contingency table can transform numbers into eye-catching graphics! Here are two effective methods: 1. Bar Charts: Perfect for displaying the counts or proportions of each category. 2. Mosaic Plots: Show the relative sizes of different categories with rectangles, making it easy to see which demographic prefers which drink at a glance.
Please let us know what you think about our content by leaving a comment down below!
Thank you for reading till here 🙂
All images from Pexels