Introduction
Statistics play a crucial role in manufacturing. It helps companies analyze data, improve processes, and maintain quality control. Without statistics, making informed decisions would be like trying to navigate a maze blindfolded.
Descriptive statistics summarize and describe the main features of a dataset. They provide insights into what has happened in a manufacturing process. For example, calculating the average production time or the most common defect can help manufacturers understand their operations. To dive deeper into this subject, consider checking out Statistics for Business and Economics by Paul Newbold.
On the other hand, inferential statistics take things a step further. They allow manufacturers to make predictions and generalizations about larger populations based on sample data. Imagine you have a batch of products. Instead of inspecting every item, you can test a sample and infer the quality of the entire batch.
Understanding both descriptive and inferential statistics is essential for data-driven decision-making in manufacturing. This knowledge helps in identifying trends, making forecasts, and implementing improvements. Want to get a solid grasp of these concepts? Check out The Art of Statistics: Learning from Data by David Spiegelhalter.
In this article, we’ll explore the key concepts of statistics in manufacturing. We will break down the differences between descriptive and inferential statistics, their applications, and how they can enhance manufacturing processes. Buckle up; it’s going to be a data-filled ride!
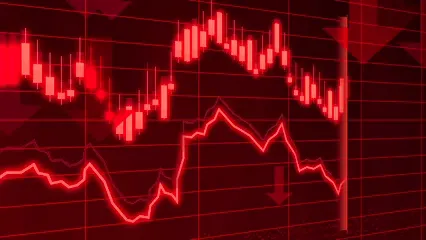
Understanding Statistics in Manufacturing
What Are Statistics?
Statistics is the science of collecting, analyzing, interpreting, and presenting data. It plays a vital role across various fields, especially manufacturing. By turning raw data into meaningful insights, statistics helps organizations streamline operations and boost efficiency.
In manufacturing, statistics help in process improvement and quality control. For instance, quality control teams use statistical methods to monitor production processes. They analyze data to identify defects and inefficiencies, paving the way for corrective actions. For a comprehensive guide on these methods, consider picking up Statistical Methods for Quality Improvement by Thomas P. Ryan.
When a plant adopts statistical methods, it can significantly enhance its operational performance. Think of it as having a crystal ball that reveals patterns and trends within the chaos of numbers.

Importance of Data Analytics in Manufacturing
In the modern manufacturing landscape, data analytics has become indispensable. Companies now rely on data-driven decisions to stay competitive. With the right statistical tools, manufacturers can optimize processes, reduce waste, and improve product quality. If you’re looking to enhance your data skills, check out Data Science for Business by Foster Provost.
Data analytics empowers manufacturers to make informed choices. For example, analyzing production data can reveal bottlenecks in the assembly line. By addressing these issues, companies can increase efficiency and reduce costs.
Let’s not forget about real-world examples! A major automobile manufacturer used data analytics to monitor assembly line performance. By implementing changes based on statistical insights, they reduced production time by 20%. This not only saved costs but also improved customer satisfaction.
In conclusion, understanding statistics is essential for effective manufacturing processes. With a solid grasp of descriptive and inferential statistics, manufacturers can harness the power of data analytics. This knowledge translates into better decision-making, increased efficiency, and ultimately, a healthier bottom line.
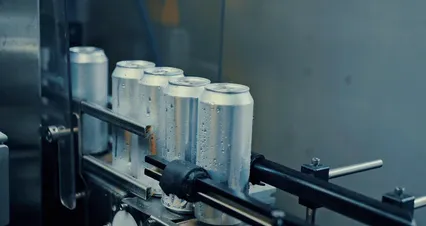
Descriptive Statistics in Manufacturing
Definition and Purpose
Descriptive statistics play a vital role in manufacturing. They summarize large datasets, showcasing essential features and trends. Think of descriptive statistics as the first few chapters of a gripping novel, setting the stage for what comes next. They provide a snapshot of the data, aiding manufacturers in understanding their processes without diving into complex statistical methods.
In manufacturing, these statistics help managers and engineers gauge performance, quality, and efficiency. By summarizing data, they reveal patterns that inform decision-making. For example, if a company tracks production times, descriptive statistics can highlight average times and identify outliers, such as unusually long production runs. This clarity allows companies to pinpoint issues and streamline operations effectively.
Understanding the role of descriptive statistics is crucial in manufacturing. For a deeper dive, check out this article on descriptive statistics in manufacturing plant. You might also find the insights in Data Visualization: A Practical Introduction by Kieran Healy beneficial for visualizing your data.
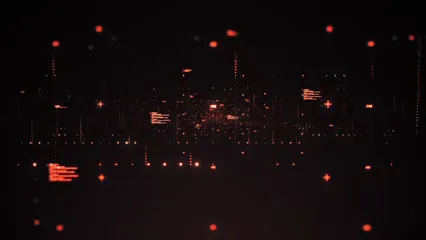
Key Components of Descriptive Statistics
Measures of Central Tendency
Measures of central tendency include mean, median, and mode. These metrics are crucial for understanding the average performance in manufacturing settings.
- Mean: This is the average value of a dataset. For instance, if a factory records production times of 20, 22, 21, 19, and 30 minutes, the mean production time would be (20+22+21+19+30)/5 = 22 minutes. This tells managers about the overall efficiency of their production line.
- Median: This value represents the middle point of a sorted dataset. Using the same production times (19, 20, 21, 22, and 30), the median would be 21 minutes. The median is particularly useful when data includes outliers, as it provides a more accurate reflection of typical performance.
- Mode: The mode indicates the most frequently occurring value. In a manufacturing context, if the most common defect reported is a “scratch,” understanding this statistic helps direct quality control efforts where they are needed most.
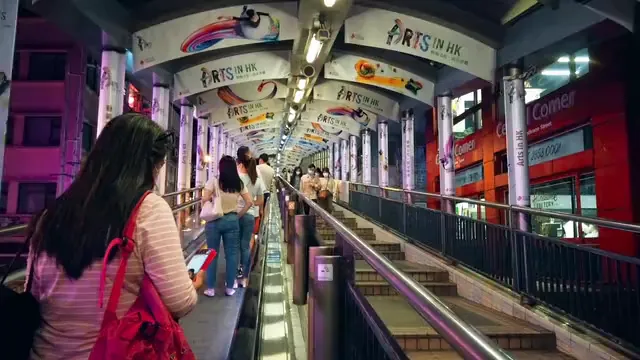
Measures of Dispersion
Measures of dispersion assess the variability in manufacturing data. They answer questions like, “How much do our production times vary?” Understanding this variability is key to maintaining quality and efficiency.
- Range: This measure indicates the difference between the maximum and minimum values. If the highest production time is 30 minutes and the lowest is 19 minutes, the range is 11 minutes. A large range may signal inconsistencies that need addressing.
- Variance: This metric shows how far each data point is from the mean. A high variance indicates that production times are widely spread out, which can lead to inefficiencies.
- Standard Deviation: This is the square root of variance and provides insight into how much individual production times deviate from the mean. A small standard deviation means most production times are close to the average, while a large standard deviation indicates inconsistency.
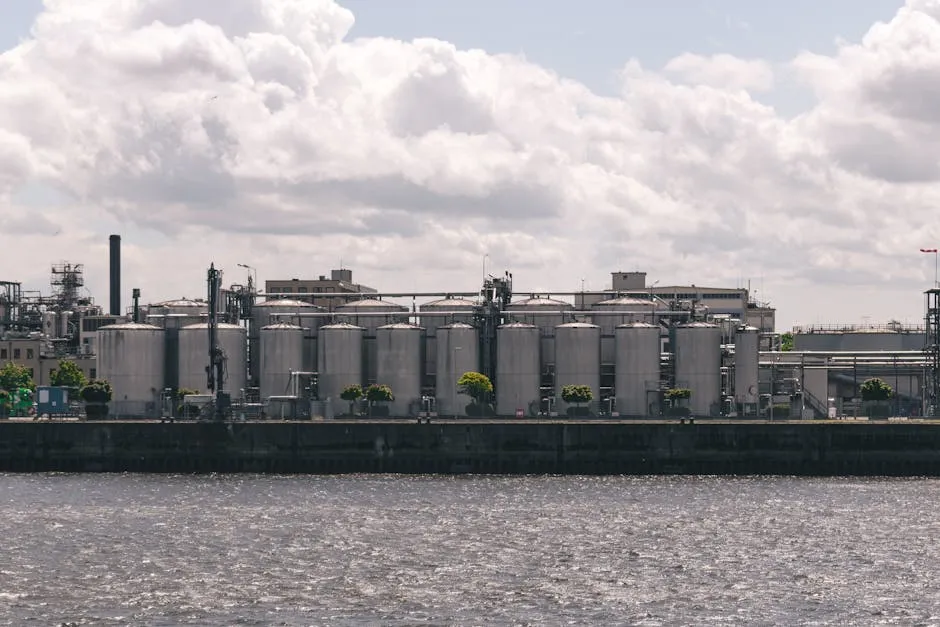
Graphical Representations
Visual data representation is essential for communicating findings in manufacturing. Charts and graphs make it easier for stakeholders to interpret large amounts of data quickly.
- Control Charts: These are vital for monitoring manufacturing processes over time. They display data points in relation to control limits, indicating whether the process remains stable or requires intervention. For a deeper understanding, check out Control Charts: Tools for Continuous Improvement by Dale H. Besterfield.
- Pareto Charts: These charts help focus on the most significant factors affecting production quality. By displaying data in descending order, they illustrate which defects should be prioritized for quality improvements.
- Histograms: These visual representations of data distribution allow manufacturers to see variations in production times or defect rates. They provide a clear overview of the frequency of different outcomes, making trends easy to spot.
In summary, descriptive statistics serve as the foundation for data analysis in manufacturing. By summarizing data through measures of central tendency and dispersion, and employing graphical representations, manufacturers gain critical insights into their processes. This understanding empowers them to enhance efficiency, maintain quality, and drive decision-making. As they say, “A picture is worth a thousand words,” and in manufacturing, a well-constructed graph can be worth its weight in gold!
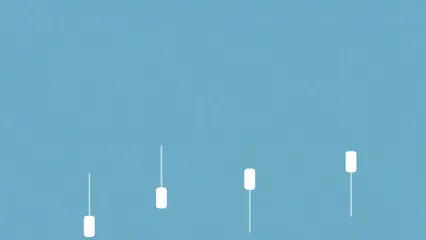
Inferential Statistics in Manufacturing
Definition and Purpose
Inferential statistics is all about making educated guesses. It uses sample data to make predictions and draw conclusions about larger populations. In manufacturing, this approach is invaluable. Why? Because inspecting every single product is often impractical, and that’s where inferential statistics steps in like a superhero. It allows manufacturers to infer quality, efficiency, and performance without needing to check each item.
Imagine you want to know the average lifespan of a light bulb produced in your factory. Instead of testing every bulb, you can take a representative sample, perform some calculations, and infer the average lifespan for all bulbs produced. It’s like having a crystal ball that helps you understand your entire production without the headache of examining every piece. If you’re keen on mastering these techniques, grab a copy of The Elements of Statistical Learning by Trevor Hastie.
For insights into the challenges faced with inferential statistics, read more about the problem with inferential statistics.
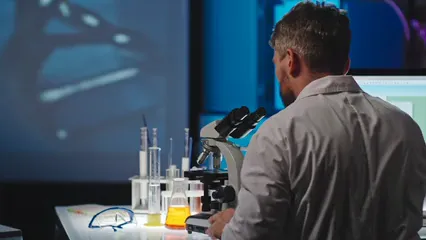
Key Techniques in Inferential Statistics
Hypothesis Testing
Hypothesis testing is like a game of “guess who” but with numbers. It involves making an assumption about a population parameter and testing whether that assumption is true. In manufacturing, you might test the effectiveness of a new production method. Let’s say you think a new assembly line will reduce defects. You can set up a null hypothesis stating that the new method does not reduce defects compared to the old one. You then collect data, analyze it, and see if you can reject that null hypothesis. If the data shows a significant reduction in defects, you’ve got a winner.
For example, a toy manufacturer might want to determine if a new molding process decreases the number of defects. By sampling units from both the old and new methods, they can conduct hypothesis testing to decide if the new method is indeed superior. If you’re interested in further exploring this method, consider reading Practical Statistics for Data Scientists by Peter Bruce.
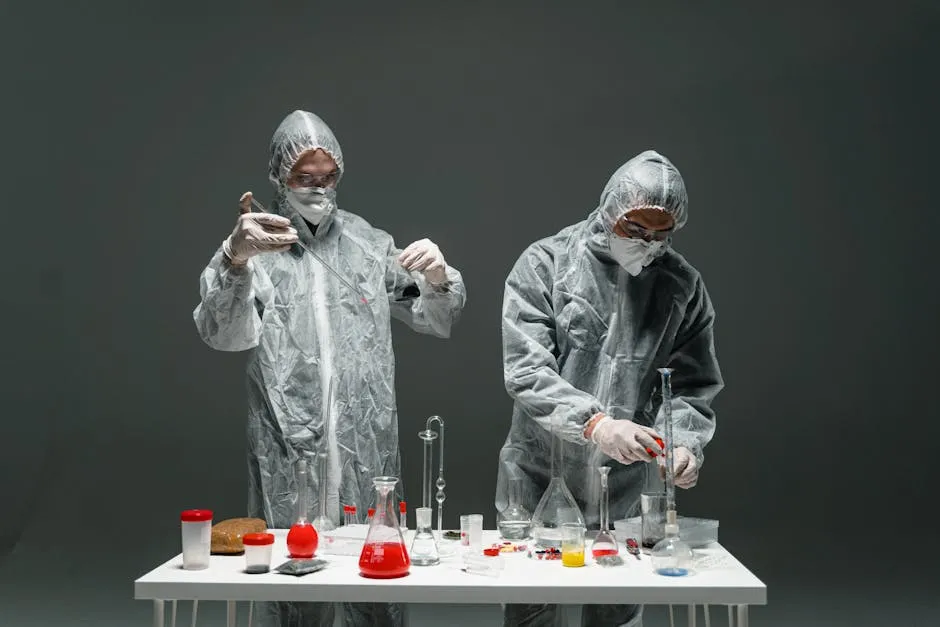
Confidence Intervals
Confidence intervals are like safety nets. They provide a range of values that likely contain the true population parameter. For instance, if you’re estimating the average defect rate in your production, a confidence interval will give you a range (like 2% to 4% defects) along with a confidence level (say, 95%). This means you can be 95% sure that the true defect rate lies within that interval.
In manufacturing, confidence intervals help in quality control. If the interval for defect rates is deemed acceptable, you can confidently proceed without major adjustments. If it’s too high, it’s time to investigate. For an in-depth understanding of these concepts, look into Data Analysis Using Regression and Multilevel/Hierarchical Models by Andrew Gelman.

Regression Analysis
Regression analysis is like matchmaking for variables. It examines the relationship between different factors and helps predict outcomes. In manufacturing, you might want to know how changes in temperature during production affect product durability. By using regression analysis, you can model this relationship, allowing you to predict how a 5-degree increase in temperature might impact durability.
For instance, if a manufacturer discovers that lower humidity levels lead to higher product quality, they can use regression analysis to quantify that relationship. This could lead to better control measures in the manufacturing process, reducing defects and improving overall performance. To enhance your skills in this area, consider checking out The Data Warehouse Toolkit: The Definitive Guide to Dimensional Modeling by Ralph Kimball.
In summary, inferential statistics are crucial for making informed decisions in manufacturing. By applying techniques like hypothesis testing, confidence intervals, and regression analysis, manufacturers can derive meaningful insights from sample data. This not only enhances product quality but also optimizes processes and resource allocation. So, the next time you hear “inferential statistics,” think of it as the secret ingredient to manufacturing success!
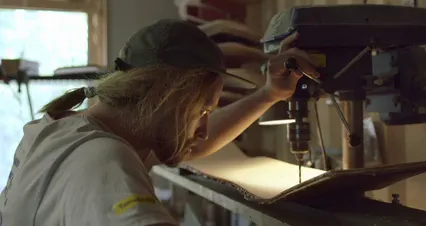
Applications in Manufacturing
In manufacturing, inferential statistics shine like a diamond in the rough. They’re the secret sauce behind process improvement and decision-making, turning raw data into actionable insights. Let’s peek behind the curtain with a couple of case studies that illustrate this.
Take, for example, a well-known automotive manufacturer. They faced a recurring defect in one of their car models. Instead of inspecting every vehicle—imagine the chaos!—they used inferential statistics. By sampling a few units from each production batch, they could identify the defect rate and determine if it was within acceptable limits. The result? A targeted approach to quality control that saved time and reduced costs.
Another case comes from a consumer electronics company struggling to manage their resources effectively. They implemented inferential statistics to analyze production data. By examining samples of completed products, they could accurately predict the demand for various components. This led to smarter resource allocation, minimizing waste and optimizing inventory levels. If you’re curious about the future of data analytics, check out Manufacturing Data Analytics: A Practical Guide by Brian J. Smith.
These real-world applications show how inferential statistics not only enhance process improvement but also bolster risk management. By making educated guesses based on sample data, manufacturers can foresee potential pitfalls before they become costly problems.
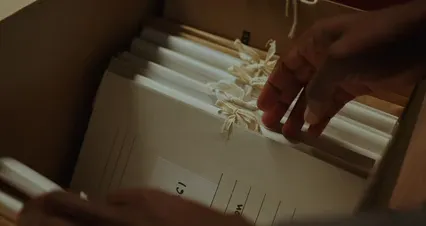
Key Differences Between Descriptive and Inferential Statistics
Comparison Table
| Aspect | Descriptive Statistics | Inferential Statistics |
|——————————|——————————————————–|———————————————————-|
| **Purpose** | Summarizes and describes features of a dataset | Makes inferences and predictions about a population |
| **Scope** | Focuses on specific sample data | Extends findings to a larger population |
| **Objective** | Describes characteristics of the data | Generalizes findings from sample to population |
| **Examples** | Measures of central tendency, dispersion, graphical representations | Hypothesis testing, regression analysis, confidence intervals |
| **Data Analysis** | Provides a summary and visualization of data | Draws conclusions, tests hypotheses, and makes predictions |
| **Population Representation** | Represents features within the sample only | Represents features of the larger population |
| **Statistical Techniques** | Mean, median, mode, range, variance, standard deviation | Hypothesis testing, regression analysis, confidence intervals |
| **Goal** | To provide insights into the characteristics of a dataset | To make predictions or draw conclusions about a population |
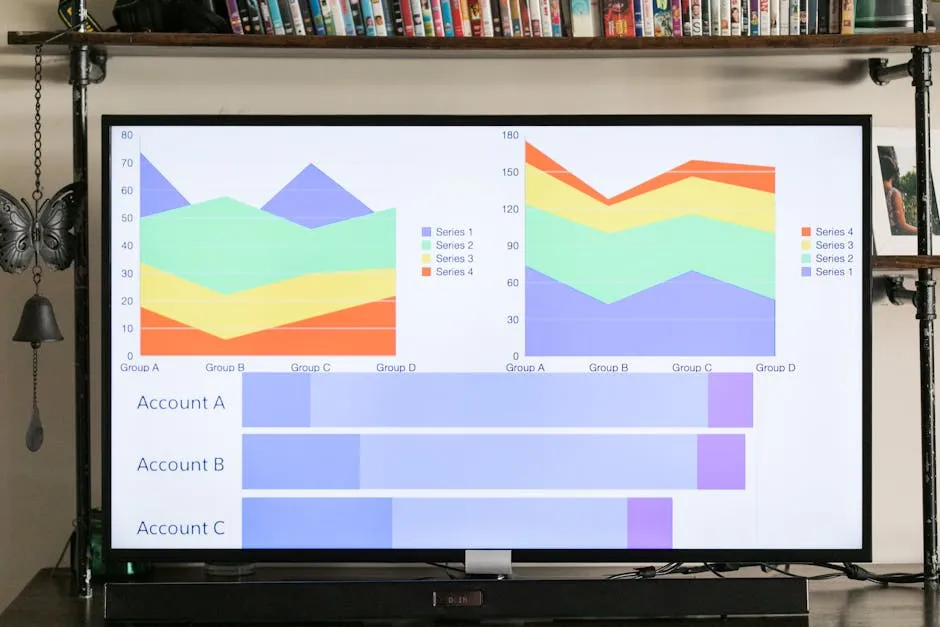
Practical Implications
Understanding the differences between descriptive and inferential statistics can greatly impact manufacturing processes. Descriptive statistics serve as a solid foundation, offering concrete insights into current operations. They help managers monitor performance, identify trends, and detect anomalies. However, relying solely on descriptive statistics can lead to stagnation. Without the predictive power of inferential statistics, manufacturers may miss opportunities for improvement.
Inferential statistics, on the other hand, empower manufacturers to make informed decisions. By analyzing sample data, they can anticipate future trends, optimize processes, and allocate resources more effectively. This proactive approach can lead to enhanced productivity and reduced operational risks. If you’re looking to boost your analytics skills, consider reading The Complete Guide to Business Analytics by David W. Smith.
In summary, while descriptive statistics provide clarity on what has already occurred, inferential statistics open the door to possibilities, guiding manufacturers towards a more efficient and profitable future.
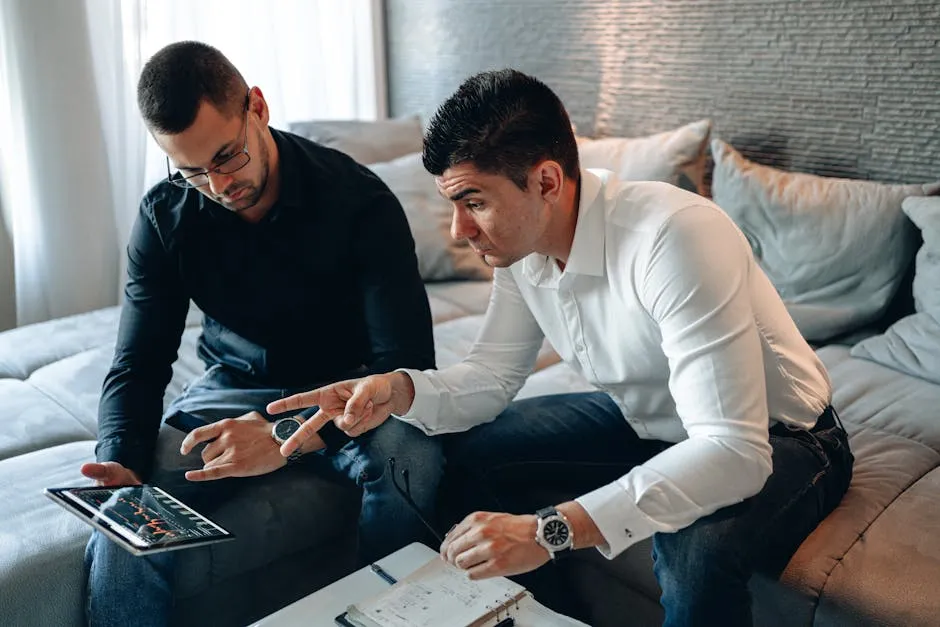
Best Practices for Using Statistics in Manufacturing
When it comes to effectively utilizing descriptive and inferential statistics in manufacturing, a few best practices can help turn data into gold.
- Understand Your Data: Before diving into analysis, take the time to understand the nature of your data. What variables are involved? What patterns are you looking for? This foundational knowledge will guide your statistical approach.
- Choose the Right Tools: Various tools can help streamline your statistical analysis. Excel is great for basic calculations. For advanced analyses, consider using R or Python, which offer robust libraries for statistical computing. If you’re keen on Python, grab a copy of Python for Data Analysis by Wes McKinney.
- Visualize Your Data: A picture is worth a thousand words, and in statistics, it’s no different. Use charts and graphs to represent data visually. Tools like Tableau or Power BI can help make sense of complex datasets.
- Train Your Team: Ensure your staff is well-trained in statistical methods. This investment in education will pay dividends in the long run, enabling your team to make data-driven decisions.
- Regularly Review and Update Analysis: Manufacturing is a dynamic field. Regularly review your statistical methods and update them as needed. New trends, technologies, or market changes can influence data interpretation.
By adhering to these best practices, manufacturers can harness the power of statistics effectively, leading to improved processes and better decision-making. Remember, the goal is to transform data into actionable insights that propel your business forward.
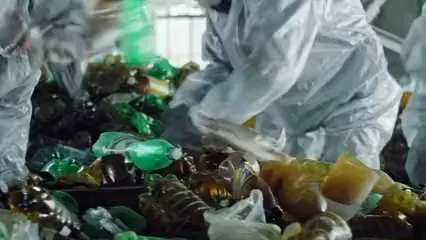
Importance of Training Staff in Statistical Methods
In the fast-paced world of manufacturing, data is king! However, raw data is only as good as the people who can interpret it. That’s where training comes in. Educating your staff in statistical methods is essential for harnessing the full potential of data analysis.
Imagine a production line where every worker is equipped with the skills to analyze data. They can spot trends, identify inefficiencies, and suggest improvements. This not only boosts confidence but also empowers employees to take ownership of their work. It’s like giving them a superhero cape, transforming them from mere operators to data-driven decision-makers!
Moreover, statistical training enhances problem-solving abilities. With a solid understanding of descriptive and inferential statistics, employees can tackle issues head-on. Whether it’s quality control or process optimization, statistical methods provide the tools to make informed choices. They start to see data not just as numbers but as clues to a more efficient operation. For a great resource on this topic, check out The Lean Six Sigma Pocket Toolbook by Michael L. George.
Let’s not forget about collaboration! When everyone speaks the same statistical language, teamwork flourishes. Employees from various departments can share insights and contribute to a culture of continuous improvement. Just think of the innovation that could spring forth when everyone is on the same page!
Investing in training also yields significant returns. Research shows that companies with well-trained staff are more productive and efficient. They can reduce waste and improve product quality, leading to happier customers. Who doesn’t want that?
In conclusion, training your staff in statistical methods is vital. It not only empowers them but also enhances overall company performance. So, let’s roll up those sleeves and get everyone on board with the power of statistics!
Conclusion
Understanding both descriptive and inferential statistics is crucial for the manufacturing sector. These statistical methods provide the foundation for data-driven decision-making. Descriptive statistics allow manufacturers to summarize and analyze past performance. On the other hand, inferential statistics enable predictions and generalizations about future outcomes.
When manufacturers leverage these statistical techniques, they can significantly improve efficiency and quality. For instance, by utilizing descriptive statistics, a company can identify trends in production times and defect rates. This insight leads to targeted interventions that optimize processes. Meanwhile, inferential statistics empower manufacturers to make informed predictions based on sample data, reducing risks associated with large-scale changes. For a comprehensive overview, consider reading Data Analysis for Business Decisions by John A. Miller.
Manufacturers must invest in data analytics training and tools. Equipping employees with statistical knowledge fosters a data-driven culture. Employees can confidently analyze data, leading to continuous improvements. Moreover, utilizing modern statistical software enhances the accuracy and efficiency of analyses.
In summary, understanding statistics is not just an academic exercise. It’s a practical necessity for manufacturers aiming to thrive in a competitive landscape. By investing in training and tools, companies can ensure their workforce is ready to harness the power of data. So, take action now! Enhance your operations and elevate your business by diving into the world of statistics.
FAQs
Please let us know what you think about our content by leaving a comment down below!
Thank you for reading till here 🙂
All images from Pexels