Introduction
Statistics has become the bedrock of data analysis across various fields. Whether you’re navigating the tricky waters of psychology, deciphering business trends, or charting the course of health sciences, statistics is your trusty compass. And when it comes to statistical analysis, IBM SPSS stands out as a powerhouse tool. It simplifies complex data manipulation, making it accessible to both novices and seasoned statisticians.
Enter Andy Field, a statistical maestro whose book, “Discovering Statistics Using IBM SPSS”, is a beacon for learners. Field has a unique ability to transform daunting statistical concepts into digestible morsels, often laced with humor. His engaging style has won over countless students who once feared the mere mention of statistics.
With SPSS at your fingertips and Field’s guidance, you’re not just learning statistics; you’re embarking on an adventure filled with insights and revelations. This guide aims to equip you with the skills needed to harness the power of SPSS effectively. We will break down the essentials of SPSS, explore its myriad applications, and provide a roadmap for statistical analysis. So buckle up, because you’re in for a fun ride through the world of statistics!
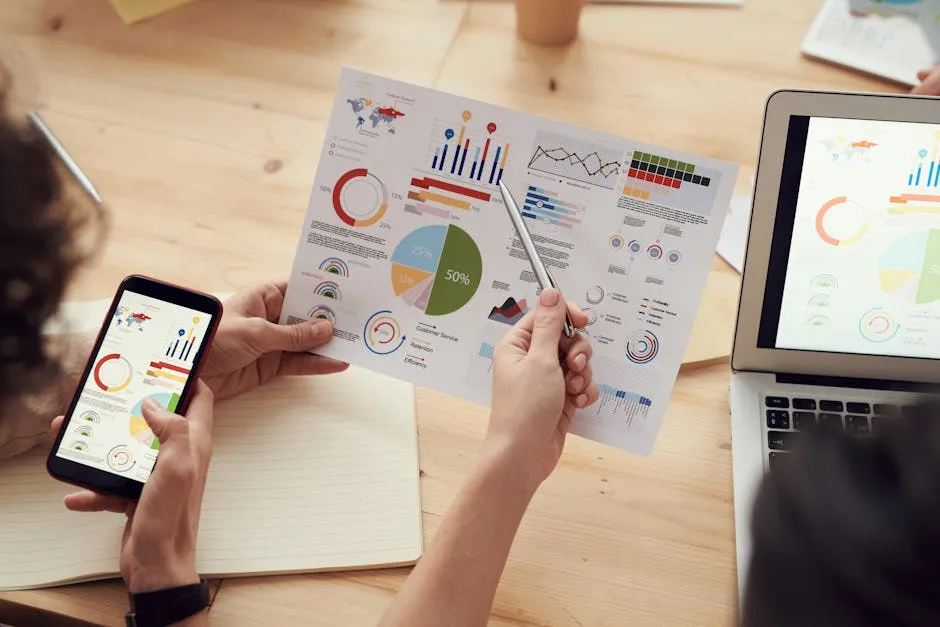
Understanding the Basics of SPSS
What is SPSS?
SPSS, or the Statistical Package for the Social Sciences, is a software suite designed for data management and statistical analysis. Initially created for social science research, SPSS has expanded its horizons, becoming a staple in various fields like psychology, business, and health sciences. It provides a comprehensive toolset for researchers to analyze data, generate reports, and visualize results.
In psychology, researchers utilize SPSS to assess the efficacy of treatments through statistical tests. Business analysts leverage it to interpret market trends, while health professionals employ SPSS to analyze clinical trial data. This versatility makes SPSS invaluable in today’s data-driven world, enabling users to turn raw data into actionable insights.
SPSS isn’t just a software; it’s a partner in your research journey, offering a user-friendly interface and a robust set of features to tackle a wide range of statistical tasks. Whether you’re crunching numbers or visualizing data, SPSS is your go-to resource for making sense of the chaos. As you familiarize yourself with SPSS, you’ll find it transforms the overwhelming into the manageable, making statistics not only accessible but also enjoyable.
Let’s dive deeper into why understanding statistics matters and how SPSS can be your best ally in this endeavor. If you’re looking for an accessible introduction to statistical concepts, consider getting Statistics for Dummies. It’s a great resource to build a solid foundation!
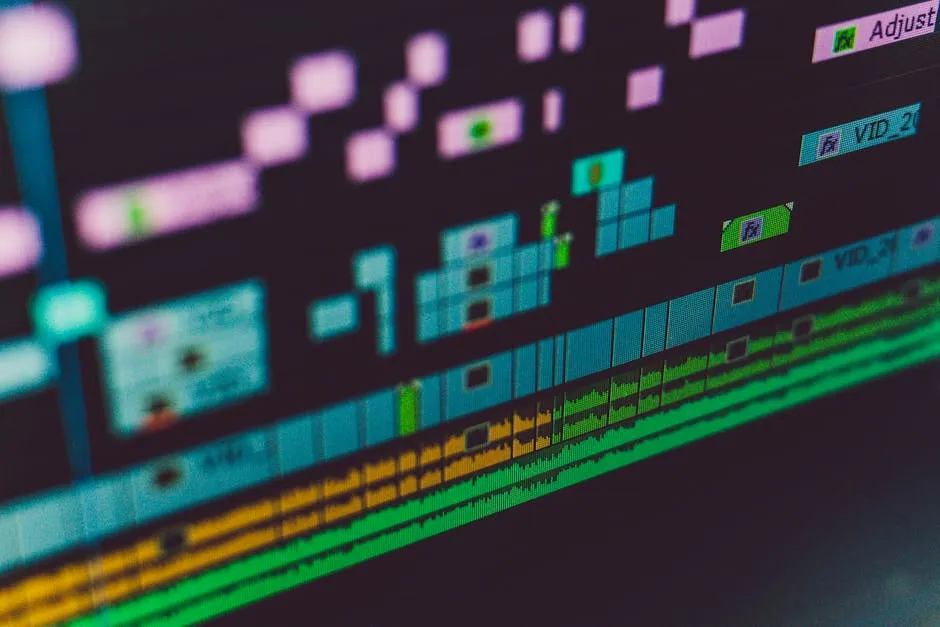
Importance of Statistics
Statistics are essential in research and decision-making. They help transform raw data into meaningful insights. By understanding statistics, you can make informed decisions based on evidence. This is crucial in fields like psychology, business, and health sciences.
Familiarizing yourself with common statistical terms is vital. Let’s break down a few key concepts:
- Mean: The average value in a dataset.
- Median: The middle value when data is sorted.
- Mode: The most frequently occurring value in a dataset.
- Standard Deviation: Measures how spread out the numbers are in a dataset.
- Hypothesis Testing: A method to determine if a statement about a population is true based on sample data.
Understanding these terms will give you a solid foundation. It’s like learning the alphabet before writing a novel. So, grab your metaphorical pen and prepare to write your statistical story! And if you’re curious about the deeper intricacies of data analysis, check out The Art of Statistics: Learning from Data. It’s a fantastic read!
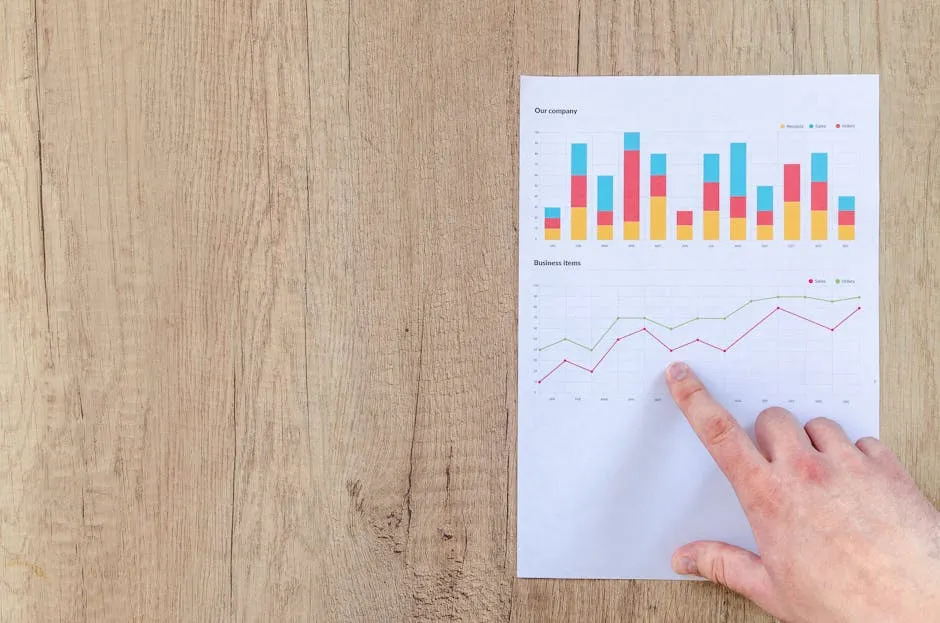
Navigating the SPSS Environment
Getting Started with SPSS
Installing SPSS is a breeze! Here’s a step-by-step guide to set you up:
- Download SPSS: Visit the IBM website or your institution’s portal to download SPSS. Choose the version compatible with your operating system.
- Installation Process: Open the downloaded file. Follow the on-screen instructions. You might need administrative rights.
- License Activation: If required, enter your license code. This usually comes with your purchase or institution subscription.
- Launch SPSS: Once installed, find the SPSS icon on your desktop or in your applications folder. Double-click to open.
Now that you’ve got SPSS up and running, let’s familiarize you with its user interface.
The Data Editor is your main workspace. Here, you can enter and view your data in a spreadsheet format. Each row represents a case, while columns represent variables.
Next is the Output Viewer. This is where SPSS displays results. Think of it as your statistical scoreboard. You can see tables, charts, and analysis results displayed neatly for review.
Finally, we have the Syntax Editor. This nifty tool allows you to write and execute commands in SPSS. If you’re feeling adventurous, you can automate repetitive tasks using syntax. It’s like having a personal assistant for your statistical needs!
With these components at your fingertips, you’re ready to conquer the SPSS environment. You won’t just be using software; you’ll be wielding a powerful statistical tool to turn data into knowledge! And if you’re looking for a comprehensive guide on using SPSS, don’t miss The Complete Guide to Data Analysis Using SPSS. It’s a must-have!
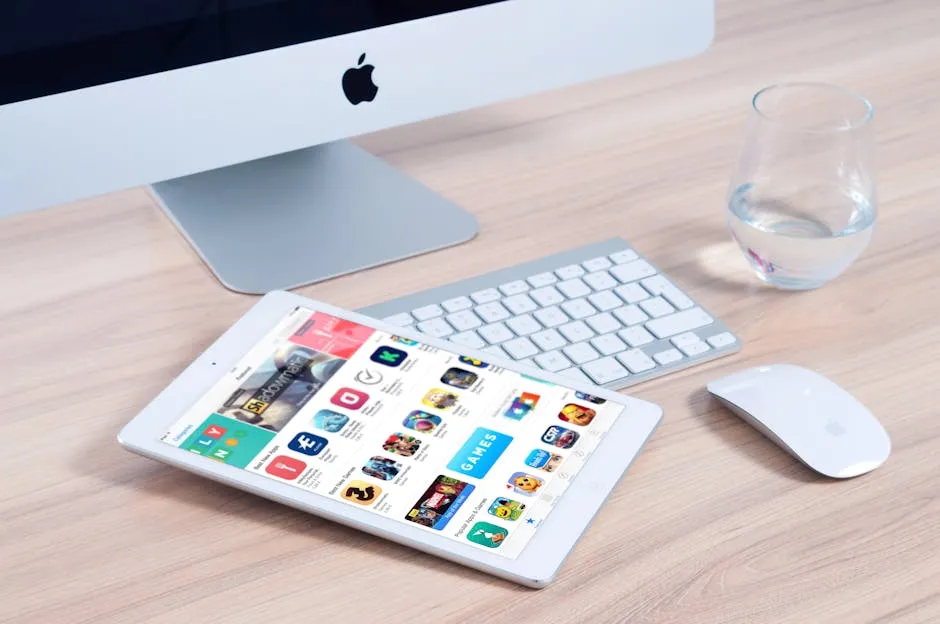
Data Entry and Management
Entering data into SPSS can feel like trying to solve a Rubik’s Cube blindfolded, but fear not! There are two primary methods for getting your data into SPSS: manual entry and importing from other formats.
Manual Entry: This method is straightforward. You can simply type your data directly into the Data Editor. Each row represents a different case, while each column is a variable. It’s like filling out a spreadsheet, but with a bit more statistical flair. However, be cautious! Manual entry can lead to errors. Double-check your data before you hit that save button.
Importing Data: If you’ve already collected your data in Excel or CSV formats, you can import it into SPSS. To do this, go to the “File” menu, select “Open,” then choose “Data.” From there, you can browse for your Excel or CSV file. SPSS will guide you through the process, asking questions to make sure everything aligns correctly. It’s like having a GPS for your data!
Now that your data is in SPSS, let’s talk about management techniques.
Inferential statistics is like a crystal ball for researchers. It allows you to make predictions and draw conclusions about a population based on a sample. This is crucial in hypothesis testing, where you’re basically playing detective. You formulate a hypothesis, gather evidence, and then determine whether to accept or reject it based on your data analysis. For a deeper understanding of hypothesis testing, refer to our Statistics Hypothesis Testing Cheat Sheet.
Hypothesis testing is fundamental in drawing conclusions from data. Explore our cheat sheet for more insights.
Key tests in inferential statistics include t-tests and ANOVA. A t-test is your go-to for comparing the means of two groups. For example, you might want to know if students who study with music perform differently than those who study in silence. SPSS makes this easy! Just click on “Analyze,” select “Compare Means,” and choose “Independent-Samples T Test.” Voilà! You’ll get results that show you whether the differences are statistically significant or just random noise.
ANOVA, or Analysis of Variance, steps in when you’re comparing three or more groups. Imagine you want to see if different teaching methods affect student performance. Instead of running multiple t-tests (which can get messy), ANOVA lets you do it all in one go. In SPSS, navigate to “Analyze,” select “General Linear Model,” then “Univariate.” After entering your variables, you’ll have your answer faster than you can say “statistically significant!”
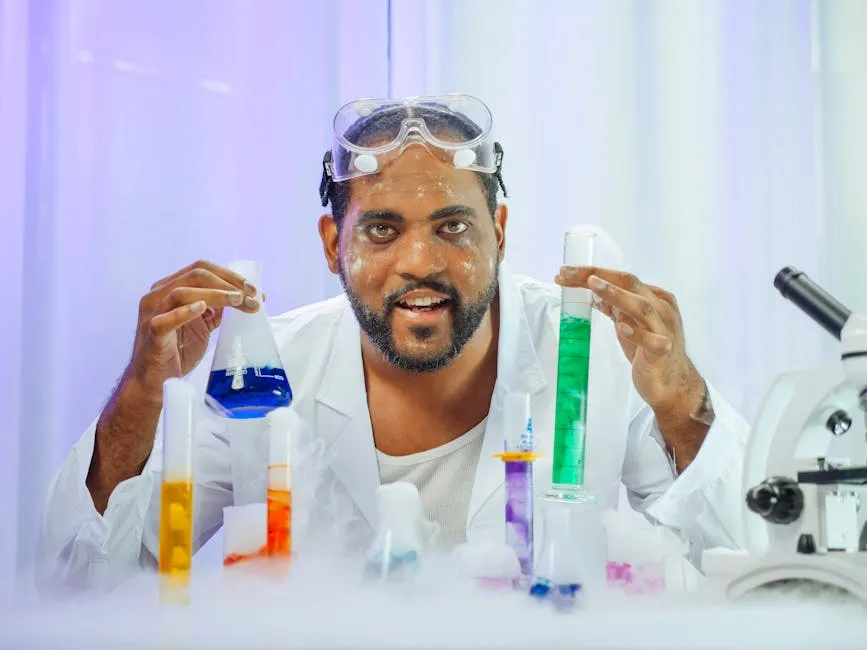
Regression Analysis
Regression analysis is like the magic wand of statistics. It helps you understand relationships among variables. Want to know how study hours affect exam scores? Regression has your back! It estimates the relationships, allowing you to make predictions. For those looking to deepen their understanding of regression techniques, consider grabbing Data Analysis Using Regression and Multilevel/Hierarchical Models. It’s a fantastic resource for advanced learners!
To conduct a simple regression analysis in SPSS, follow these steps:
- Open your data file in SPSS.
- Click on “Analyze,” then select “Regression,” and choose “Linear.”
- Move your dependent variable (e.g., exam scores) to the “Dependent” box and your independent variable (study hours) to the “Independent(s)” box.
- Hit “OK” and watch SPSS work its magic!
For multiple regression, it’s just as easy. Simply add more independent variables to the “Independent(s)” box. This allows you to examine how multiple factors impact your outcome. SPSS will provide you with a comprehensive output, including coefficients, R-squared values, and significance levels. It’s like having a statistical sidekick!
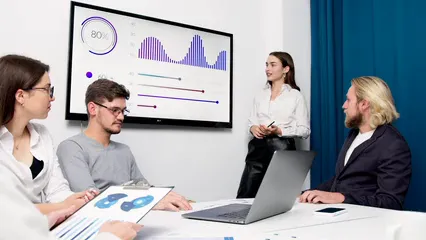
Advanced Statistical Techniques
Correlation and Causation
Correlation and causation are often mistaken for one another. Understanding the difference is essential in statistics. Correlation measures the relationship between two variables. For example, as ice cream sales go up, so do drowning incidents. But does eating ice cream cause drowning? Definitely not! They’re both influenced by warmer weather.
To run a correlation test in SPSS, follow this quick guide:
- Open your dataset.
- Click on “Analyze,” then select “Correlate,” and choose “Bivariate.”
- Move the variables you want to analyze into the box.
- Hit “OK,” and SPSS will provide you with correlation coefficients and significance levels.
This will help you understand how strongly variables are related. Just remember: correlation does not equal causation! Use this knowledge wisely, and you’ll be on your way to mastering advanced statistical techniques like a pro. If you’re interested in exploring statistical analysis further, Naked Statistics: Stripping the Dread from the Data is a highly recommended read!
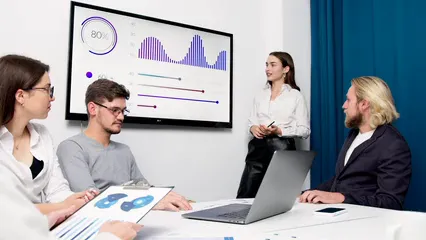
Non-parametric Tests
Non-parametric tests are your best friends when the data doesn’t fit the usual assumptions. They come into play when your data doesn’t follow a normal distribution. This is crucial because many standard tests rely on those assumptions. So, when in doubt, these tests can save the day!
One of the most common non-parametric tests is the Mann-Whitney U test. This test compares two independent groups to see if they differ in rank. For example, if you want to compare test scores between two different teaching methods, this is your go-to. Conducting this test in SPSS is a piece of cake:
- Navigate to Analyze.
- Select Nonparametric Tests.
- Choose Independent Samples.
- Move your grouping variable and the test variable into the respective boxes.
- Click OK, and voilà! SPSS will churn out your results faster than you can say “data analysis.”
Another heavy-hitter is the Kruskal-Wallis test. This one handles three or more groups. Think of it as the ANOVA for non-parametric data. For instance, if you want to assess customer satisfaction across different stores, this test does the trick. Here’s how to execute it in SPSS:
- Head to Analyze.
- Select Nonparametric Tests.
- Go for Independent Samples.
- Place your grouping variable in the “Groups” box and the test variable in the “Test Variable List” box.
- Hit OK, and SPSS will provide the results, allowing you to make sense of your data quickly.
Non-parametric tests are essential tools in your statistical toolkit. They allow you to analyze data that doesn’t conform to traditional requirements, opening up a world of possibilities for your research. And if you’re interested in diving deeper into biostatistics, consider The Essentials of Biostatistics for Physicians, Nurses, and Clinicians. It’s a fantastic resource!
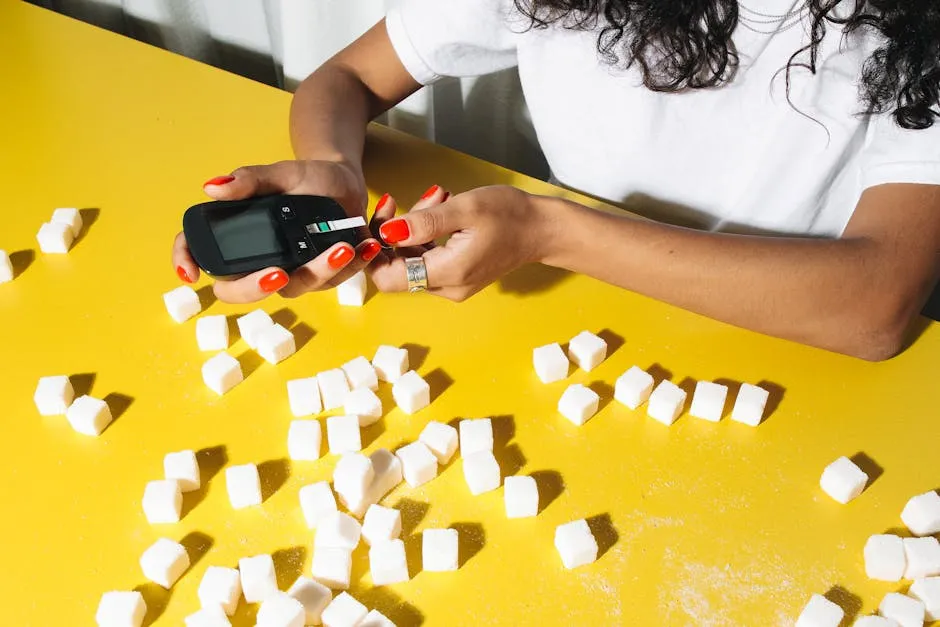
Multivariate Analysis
Ready to take your analysis up a notch? Multivariate analysis is where the magic happens. It involves examining multiple variables to understand their relationships and impacts. Two key techniques to consider are MANOVA and factor analysis.
MANOVA, or Multivariate Analysis of Variance, allows you to analyze multiple dependent variables simultaneously. It’s perfect for studies where you want to see how different factors influence multiple outcomes. For instance, in a study evaluating the effects of a new teaching method on student performance across several subjects, MANOVA shines. Here’s how to run it in SPSS:
- Click on Analyze.
- Choose General Linear Model and then Multivariate.
- Move your dependent variables into the “Dependent Variables” box and your independent variables into the “Fixed Factor(s)” box.
- Hit OK and watch the results unfold.
Now, let’s talk about factor analysis. This technique helps reduce data complexity by identifying underlying factors. It’s particularly useful when you have many variables and want to simplify your analysis. Imagine you’re researching customer preferences across various product features. Factor analysis can reveal which features cluster together, helping you focus your marketing efforts. Here’s how you can conduct it in SPSS:
- Go to Analyze.
- Select Dimension Reduction, then Factor.
- Move your variables into the “Variables” box.
- Click on Extraction, choose the method (like Principal Components), and specify how many factors to extract.
- Hit OK, and SPSS will present the factors, making your data more manageable.
Incorporating these advanced techniques not only enhances your analysis but also provides richer insights. Whether you’re diving into the depths of data with MANOVA or simplifying with factor analysis, SPSS is here to support your statistical journey! And for those interested in a broader view of data science, consider Data Science for Business: What You Need to Know About Data Mining and Data-Analytic Thinking. It’s a great addition to your library!
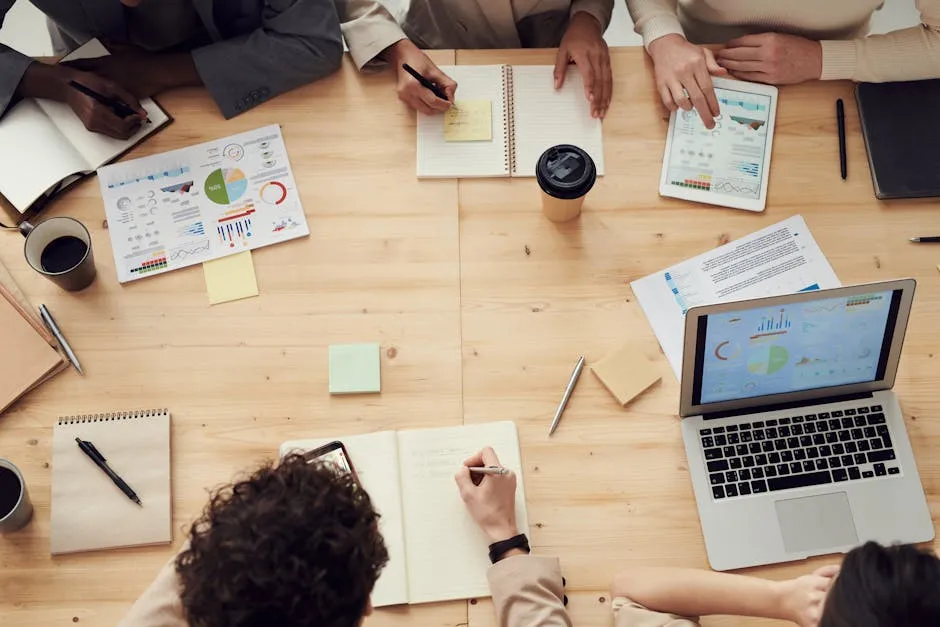
Please let us know what you think about our content by leaving a comment down below!
Thank you for reading till here 🙂
All images from Pexels
- Click on “Analyze.”
- Choose “Descriptive Statistics.”
- Select “Descriptives.”
- Move the variables you want to analyze into the “Variables” box.
- Hit “OK,” and voila! SPSS will generate a table with your results.
Common Descriptive Statistics:
- Mean: This is the average value. To find it, add up all values and divide by the total number of entries. It’s like sharing a pizza among friends!
- Median: The middle value when your data is sorted. If your dataset were a group of friends standing in line, the median would be the one right in the center. For more insights on median calculations, check out our post on Statistics Poland Median Salary 2024.
- Mode: The most frequently occurring value. It’s like finding the most popular snack at a party!
- Standard Deviation: This measures how spread out the numbers are. A low standard deviation means your data points are close to the mean, while a high standard deviation indicates a wider spread.
Understanding median is vital for analyzing data distributions effectively. Find out more about median salary trends here.
By understanding these basic concepts, you’re well on your way to making sense of your data. Descriptive statistics are your trusty sidekick, guiding you through the complexities of analysis. Armed with this knowledge, you can confidently tackle more advanced statistical techniques in SPSS! And if you’re looking for a comprehensive overview, consider The Elements of Statistical Learning: Data Mining, Inference, and Prediction. It’s an excellent reference!
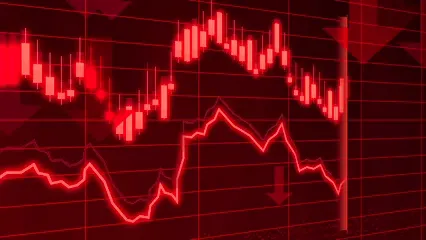
Inferential Statistics
Inferential statistics is like a crystal ball for researchers. It allows you to make predictions and draw conclusions about a population based on a sample. This is crucial in hypothesis testing, where you’re basically playing detective. You formulate a hypothesis, gather evidence, and then determine whether to accept or reject it based on your data analysis. For a deeper understanding of hypothesis testing, refer to our Statistics Hypothesis Testing Cheat Sheet.
Hypothesis testing is fundamental in drawing conclusions from data. Explore our cheat sheet for more insights.
Key tests in inferential statistics include t-tests and ANOVA. A t-test is your go-to for comparing the means of two groups. For example, you might want to know if students who study with music perform differently than those who study in silence. SPSS makes this easy! Just click on “Analyze,” select “Compare Means,” and choose “Independent-Samples T Test.” Voilà! You’ll get results that show you whether the differences are statistically significant or just random noise.
ANOVA, or Analysis of Variance, steps in when you’re comparing three or more groups. Imagine you want to see if different teaching methods affect student performance. Instead of running multiple t-tests (which can get messy), ANOVA lets you do it all in one go. In SPSS, navigate to “Analyze,” select “General Linear Model,” then “Univariate.” After entering your variables, you’ll have your answer faster than you can say “statistically significant!”
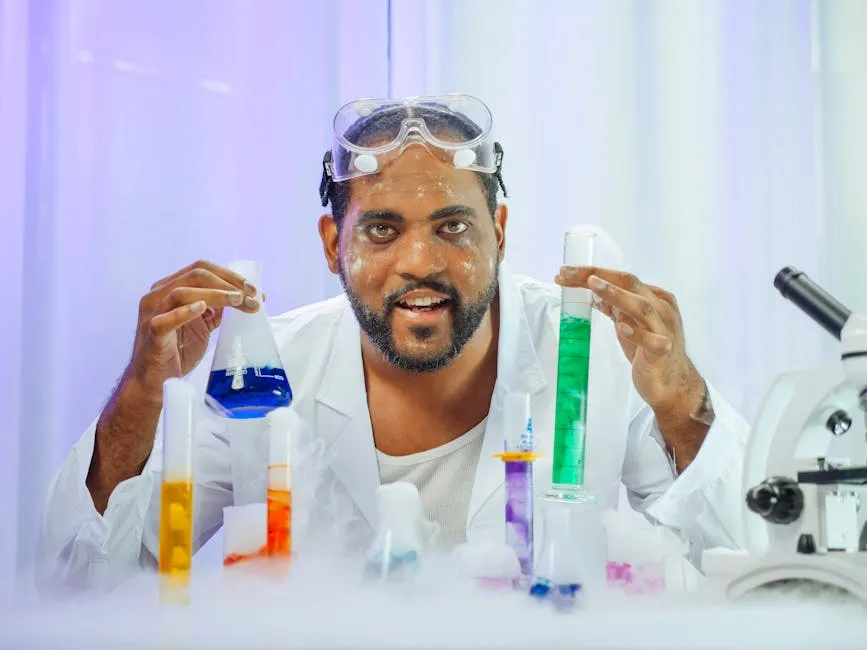
Regression Analysis
Regression analysis is like the magic wand of statistics. It helps you understand relationships among variables. Want to know how study hours affect exam scores? Regression has your back! It estimates the relationships, allowing you to make predictions. For those looking to deepen their understanding of regression techniques, consider grabbing Data Analysis Using Regression and Multilevel/Hierarchical Models. It’s a fantastic resource for advanced learners!
To conduct a simple regression analysis in SPSS, follow these steps:
- Open your data file in SPSS.
- Click on “Analyze,” then select “Regression,” and choose “Linear.”
- Move your dependent variable (e.g., exam scores) to the “Dependent” box and your independent variable (study hours) to the “Independent(s)” box.
- Hit “OK” and watch SPSS work its magic!
For multiple regression, it’s just as easy. Simply add more independent variables to the “Independent(s)” box. This allows you to examine how multiple factors impact your outcome. SPSS will provide you with a comprehensive output, including coefficients, R-squared values, and significance levels. It’s like having a statistical sidekick!
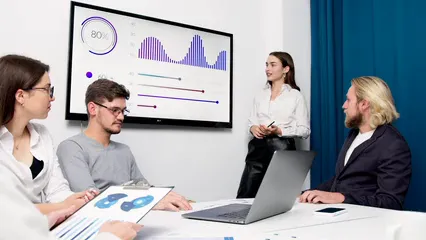
Advanced Statistical Techniques
Correlation and Causation
Correlation and causation are often mistaken for one another. Understanding the difference is essential in statistics. Correlation measures the relationship between two variables. For example, as ice cream sales go up, so do drowning incidents. But does eating ice cream cause drowning? Definitely not! They’re both influenced by warmer weather.
To run a correlation test in SPSS, follow this quick guide:
- Open your dataset.
- Click on “Analyze,” then select “Correlate,” and choose “Bivariate.”
- Move the variables you want to analyze into the box.
- Hit “OK,” and SPSS will provide you with correlation coefficients and significance levels.
This will help you understand how strongly variables are related. Just remember: correlation does not equal causation! Use this knowledge wisely, and you’ll be on your way to mastering advanced statistical techniques like a pro. If you’re interested in exploring statistical analysis further, Naked Statistics: Stripping the Dread from the Data is a highly recommended read!
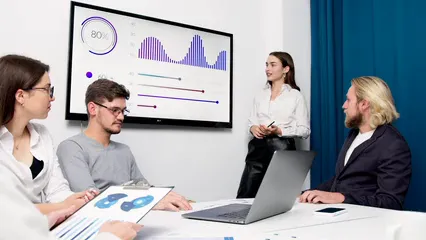
Non-parametric Tests
Non-parametric tests are your best friends when the data doesn’t fit the usual assumptions. They come into play when your data doesn’t follow a normal distribution. This is crucial because many standard tests rely on those assumptions. So, when in doubt, these tests can save the day!
One of the most common non-parametric tests is the Mann-Whitney U test. This test compares two independent groups to see if they differ in rank. For example, if you want to compare test scores between two different teaching methods, this is your go-to. Conducting this test in SPSS is a piece of cake:
- Navigate to Analyze.
- Select Nonparametric Tests.
- Choose Independent Samples.
- Move your grouping variable and the test variable into the respective boxes.
- Click OK, and voilà! SPSS will churn out your results faster than you can say “data analysis.”
Another heavy-hitter is the Kruskal-Wallis test. This one handles three or more groups. Think of it as the ANOVA for non-parametric data. For instance, if you want to assess customer satisfaction across different stores, this test does the trick. Here’s how to execute it in SPSS:
- Head to Analyze.
- Select Nonparametric Tests.
- Go for Independent Samples.
- Place your grouping variable in the “Groups” box and the test variable in the “Test Variable List” box.
- Hit OK, and SPSS will provide the results, allowing you to make sense of your data quickly.
Non-parametric tests are essential tools in your statistical toolkit. They allow you to analyze data that doesn’t conform to traditional requirements, opening up a world of possibilities for your research. And if you’re interested in diving deeper into biostatistics, consider The Essentials of Biostatistics for Physicians, Nurses, and Clinicians. It’s a fantastic resource!
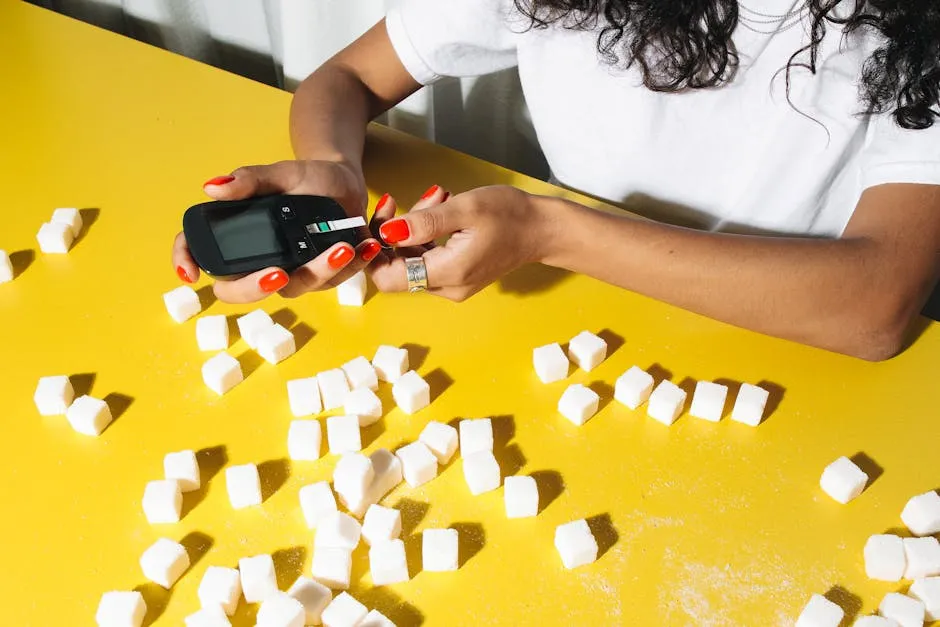
Multivariate Analysis
Ready to take your analysis up a notch? Multivariate analysis is where the magic happens. It involves examining multiple variables to understand their relationships and impacts. Two key techniques to consider are MANOVA and factor analysis.
MANOVA, or Multivariate Analysis of Variance, allows you to analyze multiple dependent variables simultaneously. It’s perfect for studies where you want to see how different factors influence multiple outcomes. For instance, in a study evaluating the effects of a new teaching method on student performance across several subjects, MANOVA shines. Here’s how to run it in SPSS:
- Click on Analyze.
- Choose General Linear Model and then Multivariate.
- Move your dependent variables into the “Dependent Variables” box and your independent variables into the “Fixed Factor(s)” box.
- Hit OK and watch the results unfold.
Now, let’s talk about factor analysis. This technique helps reduce data complexity by identifying underlying factors. It’s particularly useful when you have many variables and want to simplify your analysis. Imagine you’re researching customer preferences across various product features. Factor analysis can reveal which features cluster together, helping you focus your marketing efforts. Here’s how you can conduct it in SPSS:
- Go to Analyze.
- Select Dimension Reduction, then Factor.
- Move your variables into the “Variables” box.
- Click on Extraction, choose the method (like Principal Components), and specify how many factors to extract.
- Hit OK, and SPSS will present the factors, making your data more manageable.
Incorporating these advanced techniques not only enhances your analysis but also provides richer insights. Whether you’re diving into the depths of data with MANOVA or simplifying with factor analysis, SPSS is here to support your statistical journey! And for those interested in a broader view of data science, consider Data Science for Business: What You Need to Know About Data Mining and Data-Analytic Thinking. It’s a great addition to your library!
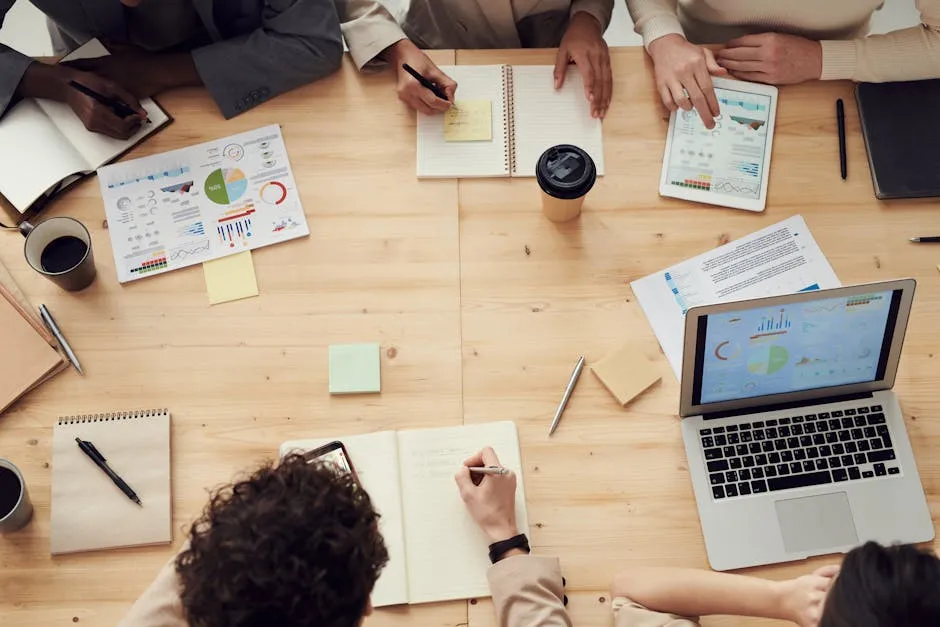
Please let us know what you think about our content by leaving a comment down below!
Thank you for reading till here 🙂
All images from Pexels
Data Cleaning: Think of this as tidying up your digital closet. Data cleaning involves checking for errors, missing values, or inconsistencies. SPSS offers tools to help you identify outliers and correct them. For example, you can use the “Descriptive Statistics” function to spot any rogue numbers that don’t belong. You can learn more about this process in our Descriptive Statistics in Manufacturing Plant post.
Data cleaning is crucial for accurate analysis and understanding how to use descriptive statistics can help identify outliers. Learn more about descriptive statistics here.
Data Transformation: Sometimes, you need your data to wear a different outfit. Data transformation allows you to alter your dataset for better analysis. This includes creating new variables, recoding values, or even aggregating data. SPSS provides several functions to assist with this, like “Compute Variable” and “Recode into Different Variables.”
By mastering data entry and management in SPSS, you set a strong foundation for your statistical analysis. It’s all about keeping your data neat, tidy, and ready for action! And for those looking to dive deeper into data mining concepts, check out Data Mining: Concepts and Techniques. It’s a great resource!

Core Statistical Concepts Covered in the Book
Descriptive Statistics
Descriptive statistics are the bread and butter of data analysis. They provide a summary of your dataset, giving you a clear picture of what’s happening in your data. Understanding how to calculate these statistics using SPSS is crucial, and Andy Field makes this as easy as pie.
Why Are Descriptive Statistics Important? They help you understand the central tendencies and variability within your data. It’s like taking a snapshot of your dataset, allowing you to identify patterns and trends quickly.
Calculating Descriptive Statistics in SPSS: To calculate descriptive statistics, you can use the “Descriptive Statistics” option in the “Analyze” menu. Here’s how:
- Click on “Analyze.”
- Choose “Descriptive Statistics.”
- Select “Descriptives.”
- Move the variables you want to analyze into the “Variables” box.
- Hit “OK,” and voila! SPSS will generate a table with your results.
Common Descriptive Statistics:
- Mean: This is the average value. To find it, add up all values and divide by the total number of entries. It’s like sharing a pizza among friends!
- Median: The middle value when your data is sorted. If your dataset were a group of friends standing in line, the median would be the one right in the center. For more insights on median calculations, check out our post on Statistics Poland Median Salary 2024.
- Mode: The most frequently occurring value. It’s like finding the most popular snack at a party!
- Standard Deviation: This measures how spread out the numbers are. A low standard deviation means your data points are close to the mean, while a high standard deviation indicates a wider spread.
Understanding median is vital for analyzing data distributions effectively. Find out more about median salary trends here.
By understanding these basic concepts, you’re well on your way to making sense of your data. Descriptive statistics are your trusty sidekick, guiding you through the complexities of analysis. Armed with this knowledge, you can confidently tackle more advanced statistical techniques in SPSS! And if you’re looking for a comprehensive overview, consider The Elements of Statistical Learning: Data Mining, Inference, and Prediction. It’s an excellent reference!
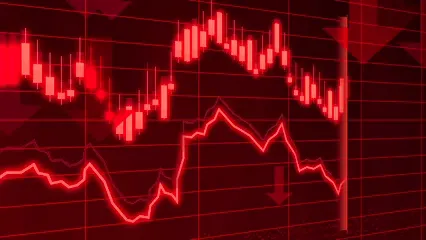
Inferential Statistics
Inferential statistics is like a crystal ball for researchers. It allows you to make predictions and draw conclusions about a population based on a sample. This is crucial in hypothesis testing, where you’re basically playing detective. You formulate a hypothesis, gather evidence, and then determine whether to accept or reject it based on your data analysis. For a deeper understanding of hypothesis testing, refer to our Statistics Hypothesis Testing Cheat Sheet.
Hypothesis testing is fundamental in drawing conclusions from data. Explore our cheat sheet for more insights.
Key tests in inferential statistics include t-tests and ANOVA. A t-test is your go-to for comparing the means of two groups. For example, you might want to know if students who study with music perform differently than those who study in silence. SPSS makes this easy! Just click on “Analyze,” select “Compare Means,” and choose “Independent-Samples T Test.” Voilà! You’ll get results that show you whether the differences are statistically significant or just random noise.
ANOVA, or Analysis of Variance, steps in when you’re comparing three or more groups. Imagine you want to see if different teaching methods affect student performance. Instead of running multiple t-tests (which can get messy), ANOVA lets you do it all in one go. In SPSS, navigate to “Analyze,” select “General Linear Model,” then “Univariate.” After entering your variables, you’ll have your answer faster than you can say “statistically significant!”
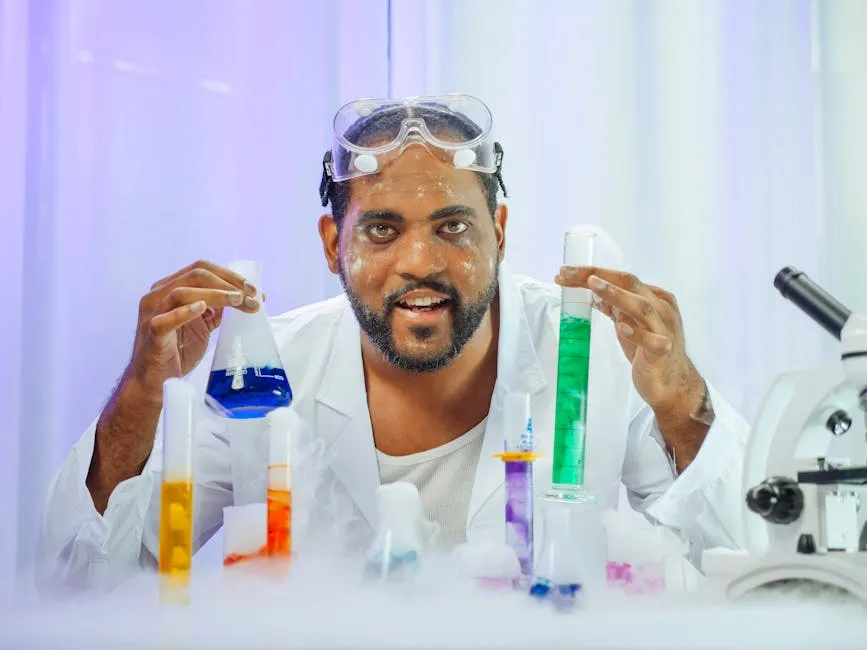
Regression Analysis
Regression analysis is like the magic wand of statistics. It helps you understand relationships among variables. Want to know how study hours affect exam scores? Regression has your back! It estimates the relationships, allowing you to make predictions. For those looking to deepen their understanding of regression techniques, consider grabbing Data Analysis Using Regression and Multilevel/Hierarchical Models. It’s a fantastic resource for advanced learners!
To conduct a simple regression analysis in SPSS, follow these steps:
- Open your data file in SPSS.
- Click on “Analyze,” then select “Regression,” and choose “Linear.”
- Move your dependent variable (e.g., exam scores) to the “Dependent” box and your independent variable (study hours) to the “Independent(s)” box.
- Hit “OK” and watch SPSS work its magic!
For multiple regression, it’s just as easy. Simply add more independent variables to the “Independent(s)” box. This allows you to examine how multiple factors impact your outcome. SPSS will provide you with a comprehensive output, including coefficients, R-squared values, and significance levels. It’s like having a statistical sidekick!
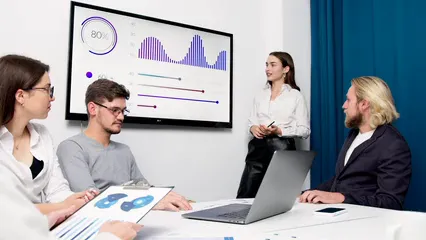
Advanced Statistical Techniques
Correlation and Causation
Correlation and causation are often mistaken for one another. Understanding the difference is essential in statistics. Correlation measures the relationship between two variables. For example, as ice cream sales go up, so do drowning incidents. But does eating ice cream cause drowning? Definitely not! They’re both influenced by warmer weather.
To run a correlation test in SPSS, follow this quick guide:
- Open your dataset.
- Click on “Analyze,” then select “Correlate,” and choose “Bivariate.”
- Move the variables you want to analyze into the box.
- Hit “OK,” and SPSS will provide you with correlation coefficients and significance levels.
This will help you understand how strongly variables are related. Just remember: correlation does not equal causation! Use this knowledge wisely, and you’ll be on your way to mastering advanced statistical techniques like a pro. If you’re interested in exploring statistical analysis further, Naked Statistics: Stripping the Dread from the Data is a highly recommended read!
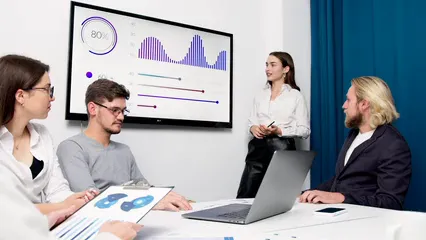
Non-parametric Tests
Non-parametric tests are your best friends when the data doesn’t fit the usual assumptions. They come into play when your data doesn’t follow a normal distribution. This is crucial because many standard tests rely on those assumptions. So, when in doubt, these tests can save the day!
One of the most common non-parametric tests is the Mann-Whitney U test. This test compares two independent groups to see if they differ in rank. For example, if you want to compare test scores between two different teaching methods, this is your go-to. Conducting this test in SPSS is a piece of cake:
- Navigate to Analyze.
- Select Nonparametric Tests.
- Choose Independent Samples.
- Move your grouping variable and the test variable into the respective boxes.
- Click OK, and voilà! SPSS will churn out your results faster than you can say “data analysis.”
Another heavy-hitter is the Kruskal-Wallis test. This one handles three or more groups. Think of it as the ANOVA for non-parametric data. For instance, if you want to assess customer satisfaction across different stores, this test does the trick. Here’s how to execute it in SPSS:
- Head to Analyze.
- Select Nonparametric Tests.
- Go for Independent Samples.
- Place your grouping variable in the “Groups” box and the test variable in the “Test Variable List” box.
- Hit OK, and SPSS will provide the results, allowing you to make sense of your data quickly.
Non-parametric tests are essential tools in your statistical toolkit. They allow you to analyze data that doesn’t conform to traditional requirements, opening up a world of possibilities for your research. And if you’re interested in diving deeper into biostatistics, consider The Essentials of Biostatistics for Physicians, Nurses, and Clinicians. It’s a fantastic resource!
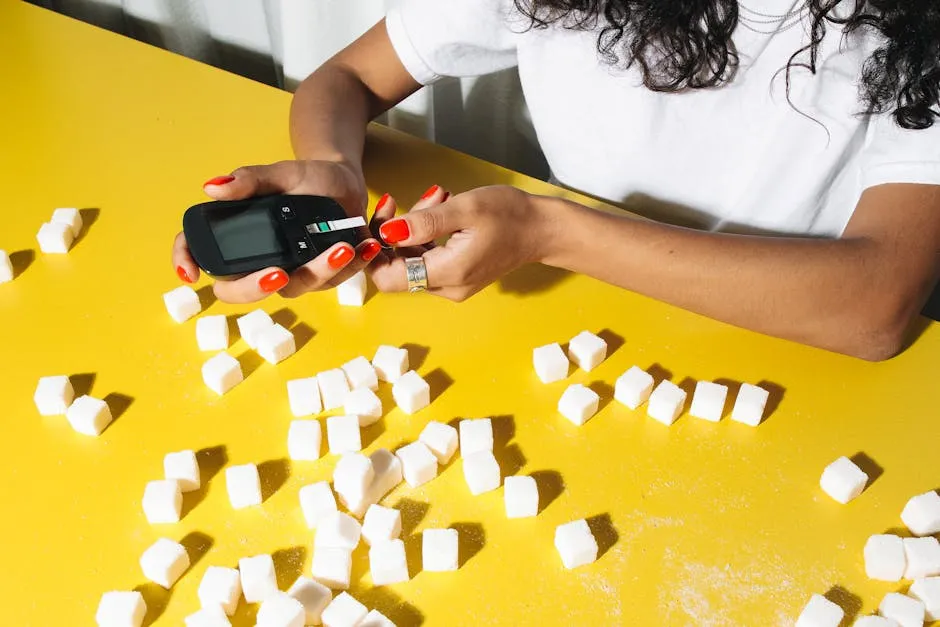
Multivariate Analysis
Ready to take your analysis up a notch? Multivariate analysis is where the magic happens. It involves examining multiple variables to understand their relationships and impacts. Two key techniques to consider are MANOVA and factor analysis.
MANOVA, or Multivariate Analysis of Variance, allows you to analyze multiple dependent variables simultaneously. It’s perfect for studies where you want to see how different factors influence multiple outcomes. For instance, in a study evaluating the effects of a new teaching method on student performance across several subjects, MANOVA shines. Here’s how to run it in SPSS:
- Click on Analyze.
- Choose General Linear Model and then Multivariate.
- Move your dependent variables into the “Dependent Variables” box and your independent variables into the “Fixed Factor(s)” box.
- Hit OK and watch the results unfold.
Now, let’s talk about factor analysis. This technique helps reduce data complexity by identifying underlying factors. It’s particularly useful when you have many variables and want to simplify your analysis. Imagine you’re researching customer preferences across various product features. Factor analysis can reveal which features cluster together, helping you focus your marketing efforts. Here’s how you can conduct it in SPSS:
- Go to Analyze.
- Select Dimension Reduction, then Factor.
- Move your variables into the “Variables” box.
- Click on Extraction, choose the method (like Principal Components), and specify how many factors to extract.
- Hit OK, and SPSS will present the factors, making your data more manageable.
Incorporating these advanced techniques not only enhances your analysis but also provides richer insights. Whether you’re diving into the depths of data with MANOVA or simplifying with factor analysis, SPSS is here to support your statistical journey! And for those interested in a broader view of data science, consider Data Science for Business: What You Need to Know About Data Mining and Data-Analytic Thinking. It’s a great addition to your library!
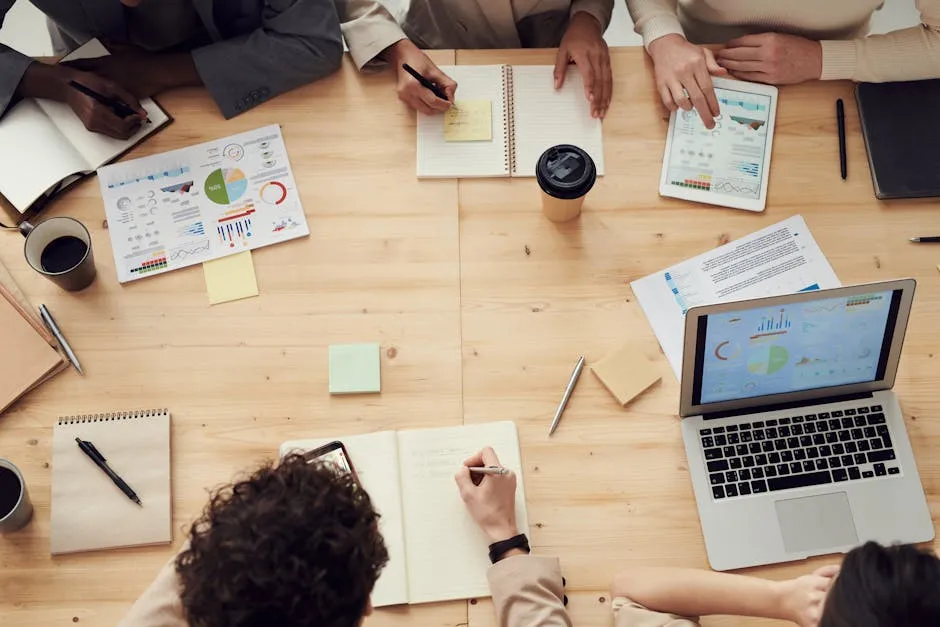
Please let us know what you think about our content by leaving a comment down below!
Thank you for reading till here 🙂
All images from Pexels