Introduction
Statistics is everywhere! From tracking the weather to predicting election outcomes, it plays a vital role in our daily lives. Understanding statistics helps us make informed decisions, whether it’s choosing the best investment or analyzing health data. It’s not just a subject for math geeks anymore; it’s for everyone!
Enter Excel, the superhero of data analysis. Excel makes statistical analysis accessible to students and professionals alike. Its user-friendly interface and powerful functions allow anyone to crunch numbers without needing a PhD in statistics. Whether you’re a student tackling your first stats class or a professional needing to analyze data, Excel has got your back.
This article aims to guide you through the world of elementary statistics using Excel. You’ll learn essential statistical concepts, how to apply them using Excel, and pick up some handy tips along the way. We’ll include practical examples to help solidify your understanding, ensuring that you’re well-equipped to tackle any statistical challenge. So, grab your Excel spreadsheet and let’s get started!
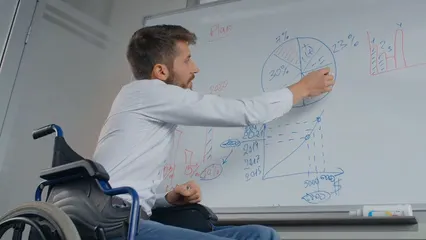
Understanding Elementary Statistics
What is Statistics?
Statistics is the science of collecting, analyzing, interpreting, and presenting data. It’s like a detective that helps us uncover hidden patterns and insights within the numbers. In decision-making, statistics provides a framework to understand uncertainty and make predictions based on empirical evidence.
There are two main branches of statistics: descriptive and inferential statistics. Descriptive statistics summarize data sets through numbers and visual representations, making it easier to understand overall trends. Think of it as the warm-up act before the main event. Inferential statistics, on the other hand, takes things further. It allows us to make predictions or generalizations about a population based on a sample. This is where things heat up!
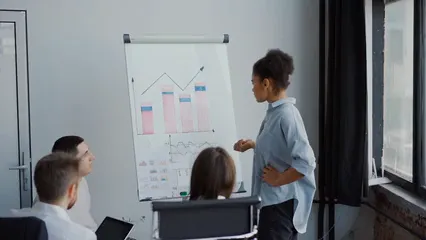
Why Use Excel for Statistics?
Using Excel for statistical analysis is like having a Swiss Army knife in your back pocket. It’s accessible, easy to use, and packed with powerful functions. Excel is widely adopted in both academic and professional settings, making it a go-to tool for many.
One of the biggest advantages of Excel is its accessibility. You don’t need to be a statistics wizard to use it. With its intuitive interface and built-in functions, you can perform complex statistical calculations with just a few clicks. Plus, Excel allows you to visualize data through charts and graphs, making your findings more digestible.
For a practical example, let’s say we want to test if a new teaching method improves student performance. Our null hypothesis (H0) could state that the new method has no effect, while the alternative hypothesis (H1) would assert that it does improve performance. Using Excel, we can conduct a t-test to analyze the data. The Excel function T.TEST(array1, array2, tails, type)
will help us determine if the difference in means is statistically significant. For more on hypothesis testing, check out this statistics hypothesis testing cheat sheet.
Understanding hypothesis testing is crucial for making data-driven decisions. Learn more about hypothesis testing techniques here.
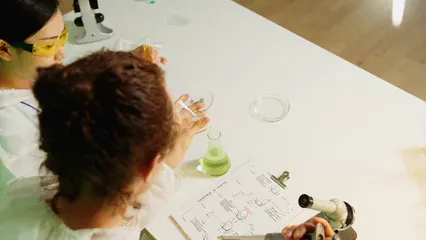
Confidence Intervals
Confidence intervals provide a range of values that likely contain the true population parameter. They offer a measure of uncertainty about our estimates. For example, if we calculate a 95% confidence interval for a mean, it means we are 95% confident that the true mean falls within this range.
Calculating confidence intervals in Excel is straightforward. Use the following steps:
1. Calculate the mean using the AVERAGE(range)
function.
2. Determine the standard deviation with STDEV.S(range)
for a sample.
3. Use the formula for the confidence interval:
– Lower limit: =mean - (t_value * (std_dev / SQRT(n)))
– Upper limit: =mean + (t_value * (std_dev / SQRT(n)))
Here, t_value
is obtained from the t-distribution table based on your desired confidence level and sample size, while n
is the sample size. Excel’s built-in functions make this process seamless, providing valuable insights into your data.
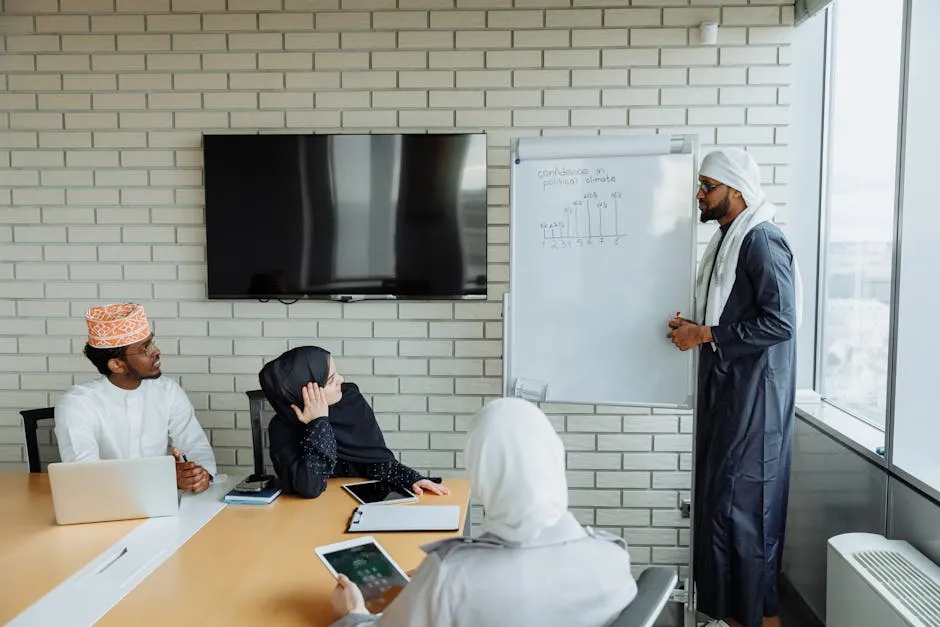
Regression Analysis in Excel
Introduction to Regression
Regression analysis is a powerful statistical method used to understand relationships between variables. It helps us predict one variable based on another, making it a favorite among analysts.
The two primary types of regression are linear regression and multiple regression. Linear regression examines the relationship between two variables, often visualized with a straight line. For instance, predicting sales based on advertising spend is a common linear regression application.
On the other hand, multiple regression involves two or more independent variables predicting a single dependent variable. Imagine predicting house prices based on location, size, and age. It provides a more comprehensive analysis by considering multiple factors simultaneously. Understanding these concepts is essential for effectively using Excel for data analysis.
With its built-in functions and chart capabilities, Excel simplifies regression analysis, making it accessible to anyone willing to learn. Whether you’re a student or a professional, mastering these techniques can significantly enhance your analytical skills.
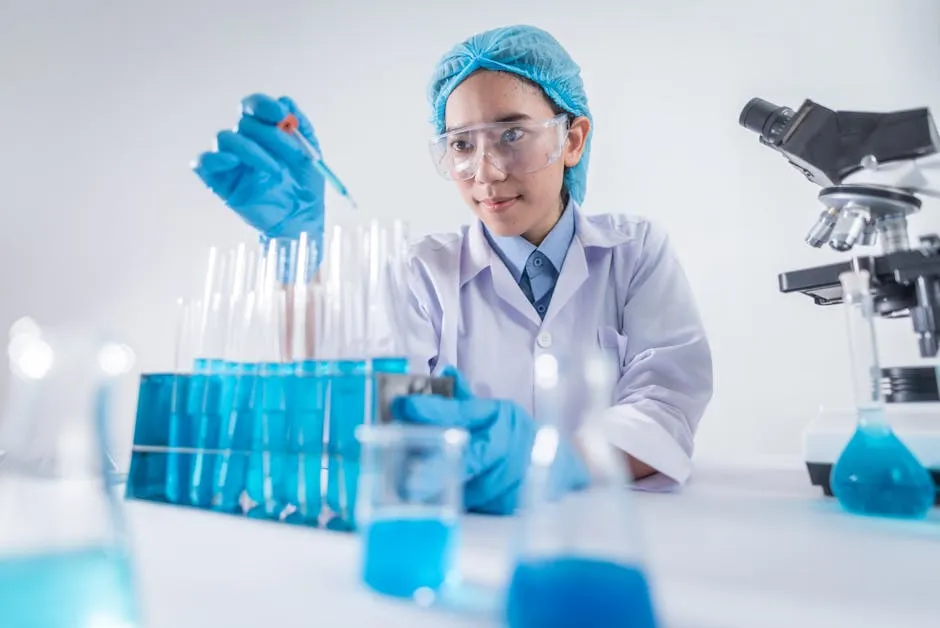
Performing Linear Regression in Excel
Linear regression helps us understand relationships between variables. It’s like matching socks—finding how one variable affects another. Let’s walk through the steps to conduct linear regression in Excel.
1. Prepare Your Data: Organize your data into two columns in Excel. One column represents the independent variable (X), and the other represents the dependent variable (Y). For example, let’s say you have sales data based on advertising spend.
| Advertising Spend (X) | Sales (Y) |
|———————–|———–|
| 100 | 200 |
| 200 | 300 |
| 300 | 400 |
| 400 | 500 |
| 500 | 600 |
2. Select Your Data: Highlight the two columns of data.
3. Insert a Scatter Plot: Go to the “Insert” tab, click on “Scatter,” and select “Scatter with Straight Lines.” This visualizes your data points.
4. Add a Trendline: Click on any data point in the chart. Right-click and select “Add Trendline.” Choose “Linear” from the options.
5. Display Equation: In the Trendline options, check “Display Equation on chart” and “Display R-squared value on chart.” The equation shows the line’s formula, while R-squared indicates how well the data fits the model.
6. Interpret the Output: The trendline visually represents the relationship. The equation helps predict sales based on advertising spend. If your equation is Y = 1X + 100, for every dollar spent on advertising, sales increase by one dollar!
This straightforward process turns Excel into a powerful tool for regression analysis. Now you can make predictions based on your data—no crystal ball required!
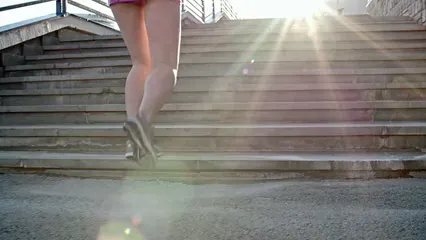
Interpreting Regression Outputs
Interpreting regression outputs is crucial for understanding your data model. Let’s break down the key components.
– Coefficients: These numbers represent the relationship between each independent variable and the dependent variable. For example, in the equation Y = 1X + 100, the coefficient of X (1) indicates that for every unit increase in X, Y increases by one unit.
– R-squared: This value ranges from 0 to 1. It tells you the proportion of variance in the dependent variable explained by the independent variable(s). An R-squared of 0.8 means 80% of the variance in sales is explained by advertising spend. The closer to 1, the better the model fits the data.
– P-values: These values help determine the significance of your coefficients. A low p-value (typically < 0.05) indicates that the relationship is statistically significant. If your p-value for the advertising coefficient is 0.01, it means there’s only a 1% chance that the relationship is due to random chance.
Understanding these outputs allows you to make informed decisions based on your analysis. Armed with this knowledge, you can confidently present your findings and make data-driven predictions!
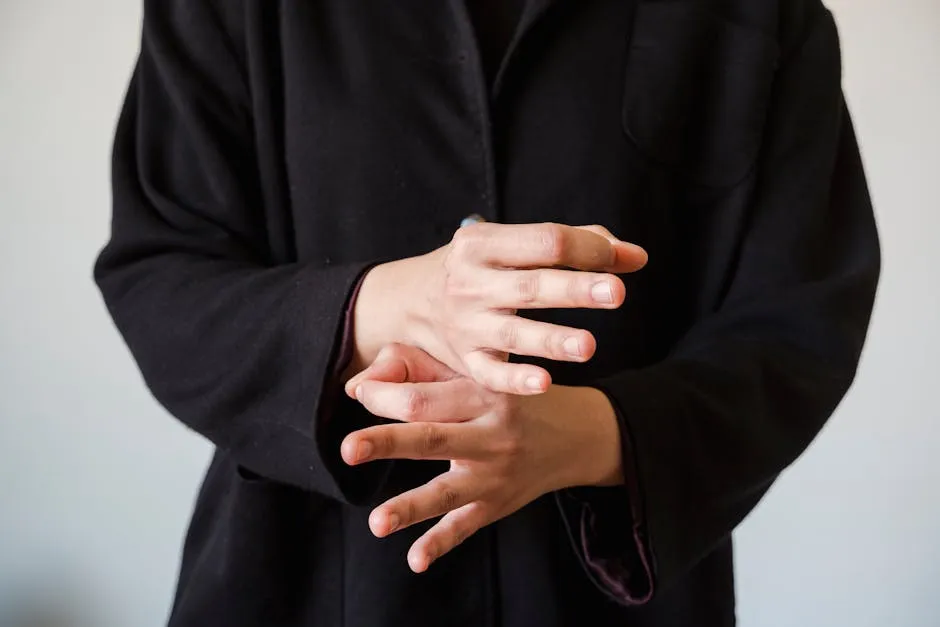
For those looking to enhance their data analysis skills further, I recommend Elementary Statistics: Picturing the World. It’s a fantastic read that will help you visualize and understand statistical concepts better.
Please let us know what you think about our content by leaving a comment down below!
Thank you for reading till here 🙂
All images from Pexels
In a world where data is king, mastering Excel for statistical analysis gives you a competitive edge. Whether you’re a student preparing for exams or a professional analyzing market trends, Excel helps transform raw data into actionable insights. So why not harness its power? Your future self will thank you!
If you want to dive deeper into Excel, consider picking up a copy of Excel 2021 for Dummies. It’s a fantastic resource that will guide you through the ins and outs of Excel, making your statistical journey even smoother!
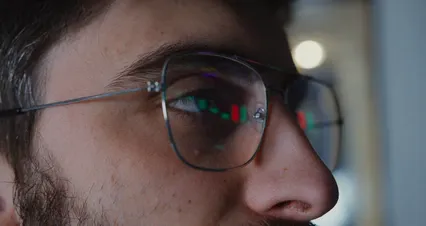
Getting Started with Excel for Statistics
Basic Excel Features for Statistics
Excel is a treasure chest for anyone dabbling in statistics. Its features transform mundane data into insightful revelations. First off, let’s talk about formulas and functions. These mathematical wizards allow you to perform calculations effortlessly. Need to find an average? Use the AVERAGE function. Want to count occurrences? The COUNT function is your friend.
But wait, there’s more! Excel’s built-in statistical functions are like a buffet of options. Functions such as MEDIAN, MODE, and STDEV.P let you calculate central tendency and variability without breaking a sweat.
Charts and graphs? Oh, they’re the icing on the statistical cake! Visualize your data with pie charts, bar graphs, or scatter plots. A picture is worth a thousand numbers, right? They make your findings pop and help convey complex information in a digestible format.
Now, let’s not forget about data organization. A little structure goes a long way. Use clear headers for your columns, and keep your data types consistent. Excel loves a good table format! You can even use filters to sift through large data sets effortlessly. Remember, tidy data is happy data!
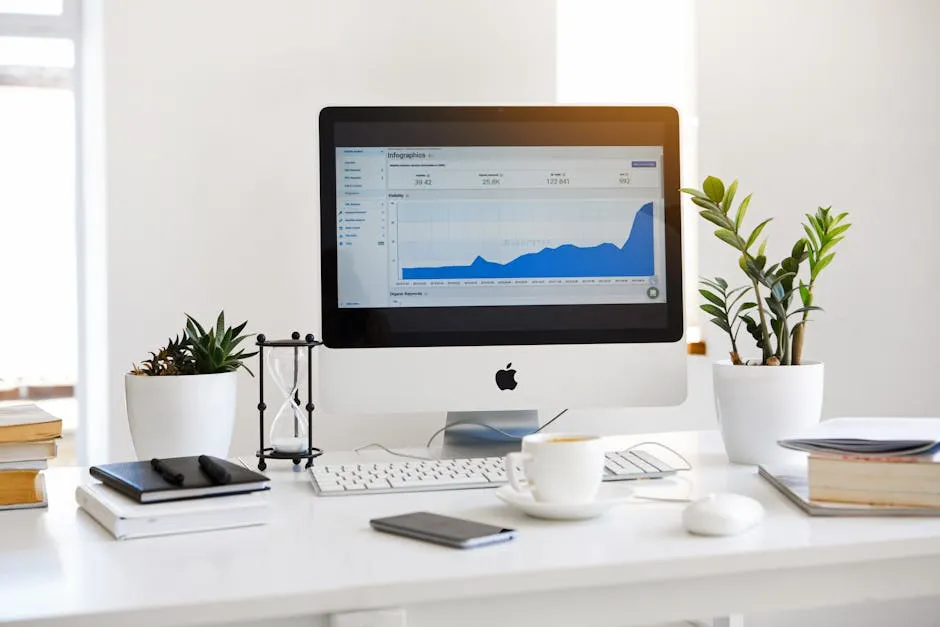
Setting Up Your Data
Setting up your data correctly is like laying a strong foundation for a house. If it’s shaky, everything else crumbles. Start by inputting your data neatly in columns. Each column should represent a variable, while each row corresponds to an observation.
Consistent formatting is crucial. Keep dates in one format and numbers as numbers. Avoid mixing text with numbers. This ensures Excel recognizes your data correctly and performs calculations without hiccups.
Clean data is paramount. Any errors or missing values can skew your results. Before jumping into analysis, double-check for typos or out-of-range values. Use conditional formatting to highlight any anomalies. If something looks fishy, it probably is!
Finally, always label your data clearly. A good label saves you from scratching your head when you revisit the spreadsheet later. Clarity is key!
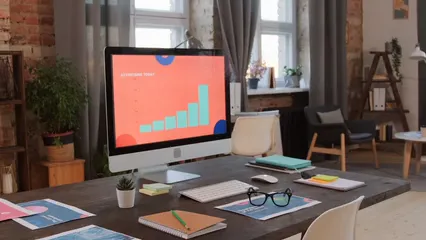
Descriptive Statistics in Excel
Measures of Central Tendency
Mean, Median, and Mode
Let’s dive into the heart of statistics: measures of central tendency! These measures give us insights into where our data points cluster.
Mean is the average of your data. To calculate it in Excel, use the AVERAGE function. Enter =AVERAGE(range)
in a cell, replacing range
with the actual range of your data. Voila! You have your mean.
Next up, we have the Median. This is the middle value, which is particularly useful when your data is skewed. To find the median in Excel, simply type =MEDIAN(range)
in a cell. Excel will do the heavy lifting for you!
Then comes the Mode, the most frequently occurring value in your dataset. Sometimes, there’s more than one mode (hello, bimodal!). To find it, use =MODE(range)
. Excel will reveal the champion number that appears most often.
These three measures together provide a comprehensive view of your dataset. They help you understand not just where your data sits, but also how it behaves. So, whether you’re averaging test scores or analyzing customer feedback, knowing how to quickly calculate these measures in Excel is a game changer!
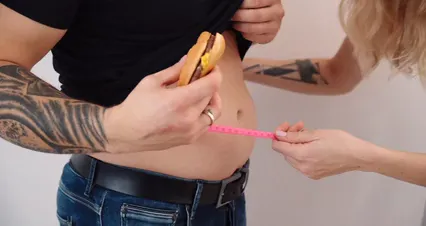
Measures of Dispersion
Range, Variance, and Standard Deviation
When we’re talking about data, it’s not just about the average. We need to understand how spread out our data is. This is where measures of dispersion come into play. Let’s break down the range, variance, and standard deviation.
Range is the simplest of the three. It measures how far apart the highest and lowest values are. To calculate the range, simply subtract the smallest value from the largest. For instance, if your dataset is [5, 10, 15], the range is 15 – 5 = 10. Easy peasy!
Variance takes it up a notch. It tells us how much the values in a dataset vary from the mean. A high variance means the numbers are spread out; a low variance means they’re close. To calculate variance in Excel, you can use the function VAR.P(range)
for the entire population or VAR.S(range)
for a sample. For example, if your data is in cells A1 to A3, enter =VAR.P(A1:A3)
to get the population variance.
Now, let’s get to standard deviation. This measure shows how much individual data points deviate from the mean, on average. It’s like variance’s cooler sibling, expressed in the same units as the data. To calculate standard deviation in Excel, use STDEV.P(range)
for the population or STDEV.S(range)
for a sample. Following the earlier example with data in A1 to A3, you would type =STDEV.P(A1:A3)
to find the population standard deviation.
Understanding these measures helps you get a clearer picture of your data. You can see not just where the average lies, but how diverse your data really is.
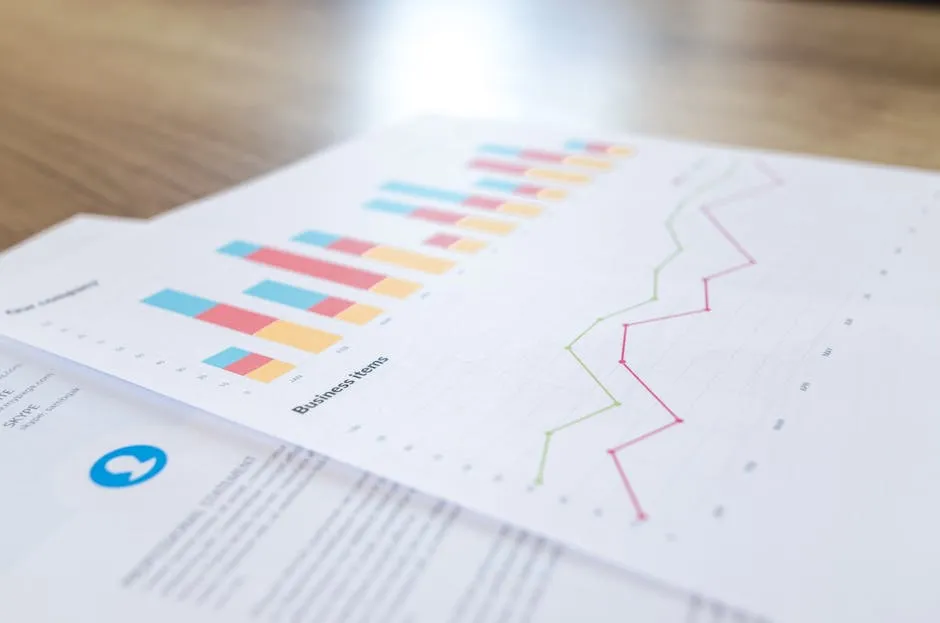
Data Visualization Techniques
Creating Graphs and Charts
Visualizing data is not just decoration; it’s essential! Charts and graphs make complex data understandable. They reveal trends, outliers, and patterns that numbers alone can’t convey.
Histograms are perfect for showing frequency distributions. To create one in Excel, follow these steps: 1. Select your data. 2. Click on the “Insert” tab. 3. Choose “Histogram” from the charts section. Excel will generate a histogram that displays how data is distributed across different ranges.
Box plots provide a visual summary of data through its quartiles. They highlight the median, upper, and lower quartiles, making it easy to spot outliers. To create a box plot in Excel, you can use the “Insert” tab, select “Box and Whisker” under the charts section, and voilà! Your box plot is ready to go.
Scatter plots are fantastic for showing relationships between two variables. To make one: 1. Highlight your data. 2. Navigate to the “Insert” tab. 3. Select “Scatter” from the charts section. This will illustrate how two datasets relate, helping you identify correlations or trends.
By utilizing these visual tools, you complement your statistical analysis. They not only make your findings more engaging but also enhance understanding. Remember, a well-placed chart can tell a story that numbers alone cannot!
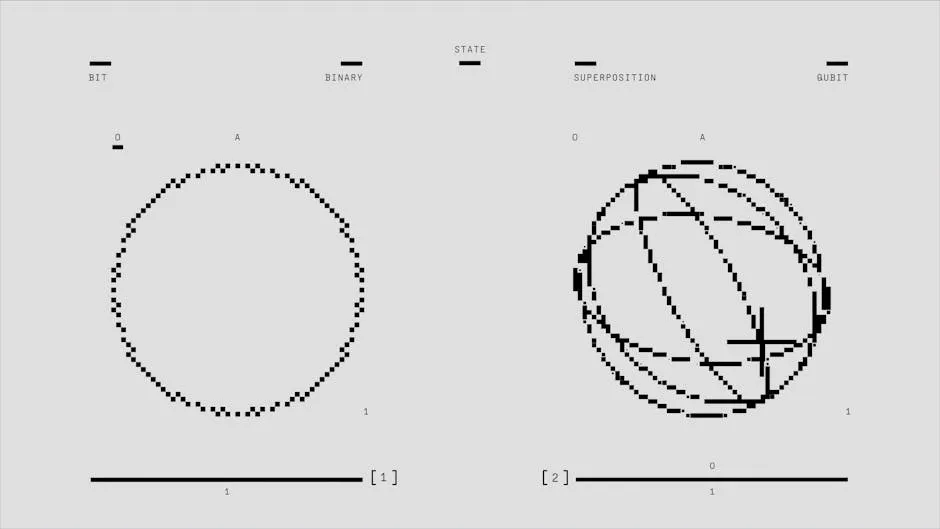
Hypothesis Testing
Steps in Hypothesis Testing
Hypothesis testing is a statistical method used to make decisions based on data. It starts with defining two competing statements: the null hypothesis (H0) and the alternative hypothesis (H1). The null hypothesis suggests no effect or no difference, while the alternative proposes a change or difference.
Next, we assess the risks associated with our decisions. This involves understanding Type I and Type II errors. A Type I error occurs when we mistakenly reject the null hypothesis, claiming a difference exists when it doesn’t. Conversely, a Type II error happens when we fail to reject the null hypothesis, missing a real effect.
For a practical example, let’s say we want to test if a new teaching method improves student performance. Our null hypothesis (H0) could state that the new method has no effect, while the alternative hypothesis (H1) would assert that it does improve performance. Using Excel, we can conduct a t-test to analyze the data. The Excel function T.TEST(array1, array2, tails, type)
will help us determine if the difference in means is statistically significant. For more on hypothesis testing, check out this statistics hypothesis testing cheat sheet.
Understanding hypothesis testing is crucial for making data-driven decisions. Learn more about hypothesis testing techniques here.
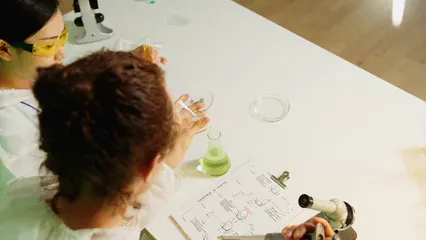
Confidence Intervals
Confidence intervals provide a range of values that likely contain the true population parameter. They offer a measure of uncertainty about our estimates. For example, if we calculate a 95% confidence interval for a mean, it means we are 95% confident that the true mean falls within this range.
Calculating confidence intervals in Excel is straightforward. Use the following steps:
1. Calculate the mean using the AVERAGE(range)
function.
2. Determine the standard deviation with STDEV.S(range)
for a sample.
3. Use the formula for the confidence interval:
– Lower limit: =mean - (t_value * (std_dev / SQRT(n)))
– Upper limit: =mean + (t_value * (std_dev / SQRT(n)))
Here, t_value
is obtained from the t-distribution table based on your desired confidence level and sample size, while n
is the sample size. Excel’s built-in functions make this process seamless, providing valuable insights into your data.
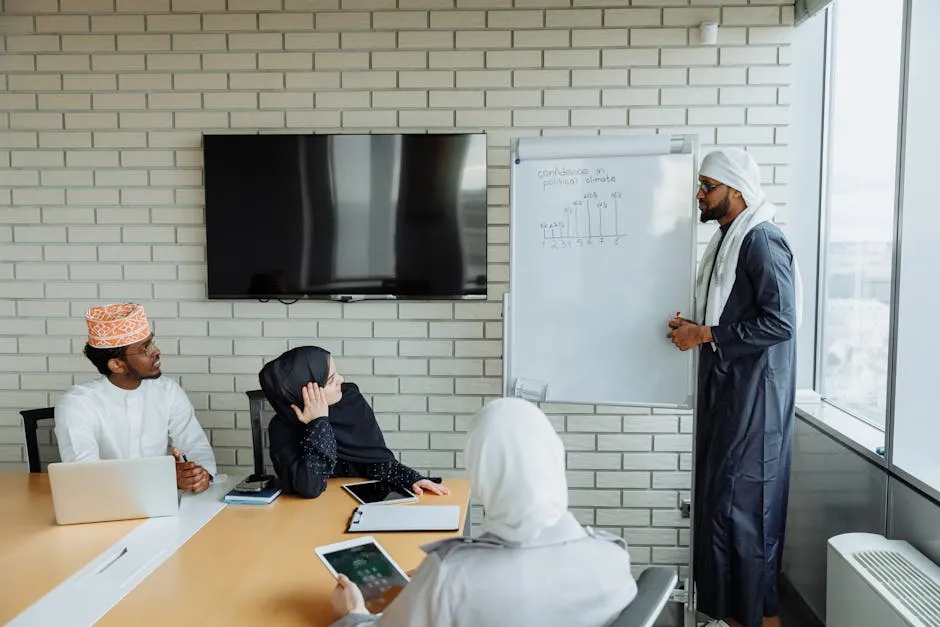
Regression Analysis in Excel
Introduction to Regression
Regression analysis is a powerful statistical method used to understand relationships between variables. It helps us predict one variable based on another, making it a favorite among analysts.
The two primary types of regression are linear regression and multiple regression. Linear regression examines the relationship between two variables, often visualized with a straight line. For instance, predicting sales based on advertising spend is a common linear regression application.
On the other hand, multiple regression involves two or more independent variables predicting a single dependent variable. Imagine predicting house prices based on location, size, and age. It provides a more comprehensive analysis by considering multiple factors simultaneously. Understanding these concepts is essential for effectively using Excel for data analysis.
With its built-in functions and chart capabilities, Excel simplifies regression analysis, making it accessible to anyone willing to learn. Whether you’re a student or a professional, mastering these techniques can significantly enhance your analytical skills.
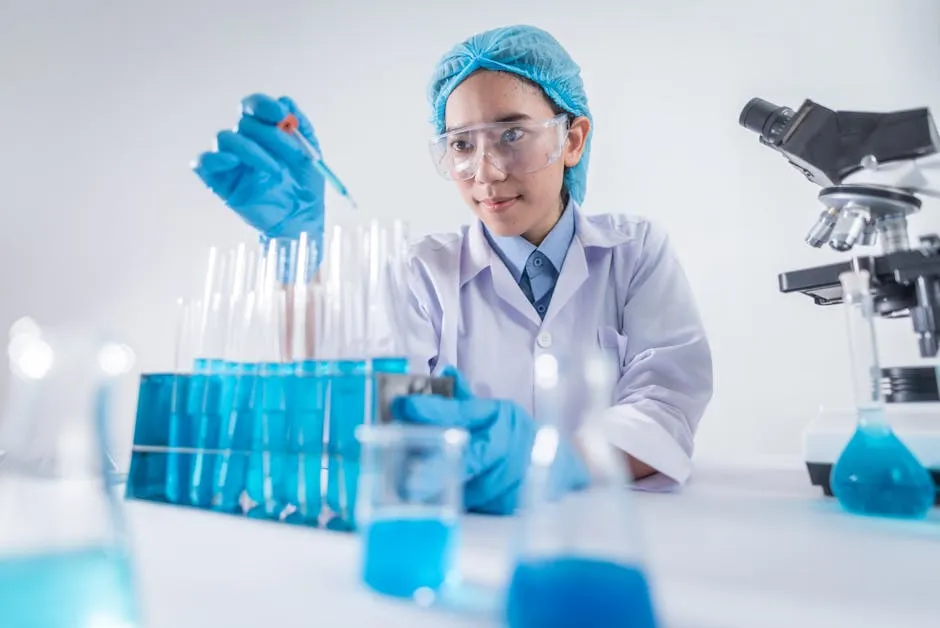
Performing Linear Regression in Excel
Linear regression helps us understand relationships between variables. It’s like matching socks—finding how one variable affects another. Let’s walk through the steps to conduct linear regression in Excel.
1. Prepare Your Data: Organize your data into two columns in Excel. One column represents the independent variable (X), and the other represents the dependent variable (Y). For example, let’s say you have sales data based on advertising spend.
| Advertising Spend (X) | Sales (Y) |
|———————–|———–|
| 100 | 200 |
| 200 | 300 |
| 300 | 400 |
| 400 | 500 |
| 500 | 600 |
2. Select Your Data: Highlight the two columns of data.
3. Insert a Scatter Plot: Go to the “Insert” tab, click on “Scatter,” and select “Scatter with Straight Lines.” This visualizes your data points.
4. Add a Trendline: Click on any data point in the chart. Right-click and select “Add Trendline.” Choose “Linear” from the options.
5. Display Equation: In the Trendline options, check “Display Equation on chart” and “Display R-squared value on chart.” The equation shows the line’s formula, while R-squared indicates how well the data fits the model.
6. Interpret the Output: The trendline visually represents the relationship. The equation helps predict sales based on advertising spend. If your equation is Y = 1X + 100, for every dollar spent on advertising, sales increase by one dollar!
This straightforward process turns Excel into a powerful tool for regression analysis. Now you can make predictions based on your data—no crystal ball required!
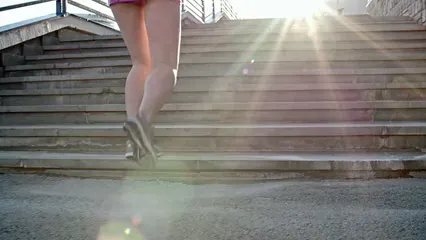
Interpreting Regression Outputs
Interpreting regression outputs is crucial for understanding your data model. Let’s break down the key components.
– Coefficients: These numbers represent the relationship between each independent variable and the dependent variable. For example, in the equation Y = 1X + 100, the coefficient of X (1) indicates that for every unit increase in X, Y increases by one unit.
– R-squared: This value ranges from 0 to 1. It tells you the proportion of variance in the dependent variable explained by the independent variable(s). An R-squared of 0.8 means 80% of the variance in sales is explained by advertising spend. The closer to 1, the better the model fits the data.
– P-values: These values help determine the significance of your coefficients. A low p-value (typically < 0.05) indicates that the relationship is statistically significant. If your p-value for the advertising coefficient is 0.01, it means there’s only a 1% chance that the relationship is due to random chance.
Understanding these outputs allows you to make informed decisions based on your analysis. Armed with this knowledge, you can confidently present your findings and make data-driven predictions!
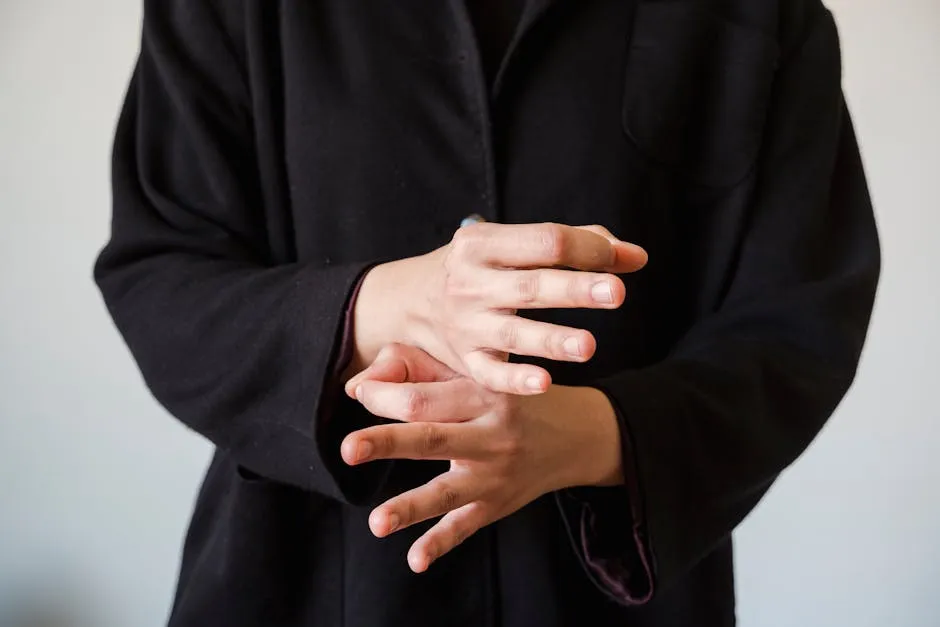
For those looking to enhance their data analysis skills further, I recommend Elementary Statistics: Picturing the World. It’s a fantastic read that will help you visualize and understand statistical concepts better.
Please let us know what you think about our content by leaving a comment down below!
Thank you for reading till here 🙂
All images from Pexels