Introduction
In our fast-paced, data-driven era, big data applications are changing the game. They’ve transformed how we analyze and interpret extensive information. With this evolution comes a shift in statistical methods, particularly in parametric statistics.
Traditional assumptions, such as normality and equal variance, have long been the pillars of parametric analysis. But as technology advances, so do these foundational beliefs. The digital landscape is brimming with complex data that doesn’t always fit neatly into these classical assumptions.
New trends are emerging that adapt these assumptions to better suit the unique challenges posed by big data. These include accommodating non-normal distributions, leveraging machine learning, and utilizing advanced computational techniques.
As we venture deeper into this intriguing subject, prepare to uncover the nuances shaping the future of statistical analysis in the big data era. We’ll explore how these trends not only enhance our methodologies but also pave the way for more accurate and insightful data interpretations.
Let’s get ready to rethink what we know about parametric statistics and see how these advancements are revolutionizing our analytical capabilities. The future is here, and it’s time to embrace the changes that big data brings to the table.
Summary
The world of big data is in a constant state of flux, prompting a necessary reevaluation of traditional parametric statistical assumptions. As we delve into this dynamic topic, we’ll highlight key emerging trends that are reshaping the landscape of parametric statistics.
One significant trend is the adaptation of assumptions to handle non-normal data distributions. More data is being generated than ever, and much of it defies the classic bell curve. Techniques for testing normality and addressing violations are gaining traction, allowing statisticians to analyze data in a more nuanced way.
Additionally, for those looking to deepen their understanding of data science methodologies, “Data Science for Business” by Foster Provost is a fantastic resource. This book demystifies data mining and analytical thinking, helping you to leverage data effectively in your business decisions.
Another crucial trend is the integration of machine learning techniques. These methods are not just for data mining anymore; they are becoming essential for informing and refining statistical analyses. We will showcase case studies that exemplify this successful marriage of machine learning and traditional statistics.
Additionally, advanced data visualization techniques are taking center stage. In big data contexts, visualizing data distributions can reveal insights that raw numbers simply can’t convey. Effective visualization methods enhance our ability to understand complex datasets. For example, to master data visualization, consider “Data Visualization: A Practical Introduction” by Kieran Healy. This book provides clear guidance on creating effective visualizations that can help you tell compelling stories with your data.
As we progress through this summary, we’ll discuss the implications of these trends for practitioners and researchers alike. The need for continuous learning and adaptation in statistical practices is paramount. By the conclusion, readers will gain a comprehensive understanding of how emerging trends in parametric statistics assumptions are transforming big data applications, leading to more accurate insights and informed decision-making.
In this evolving landscape, the importance of flexibility, robustness, and innovative thinking cannot be overstated. The future of statistical analysis is bright, and it’s time to embrace these exciting developments.
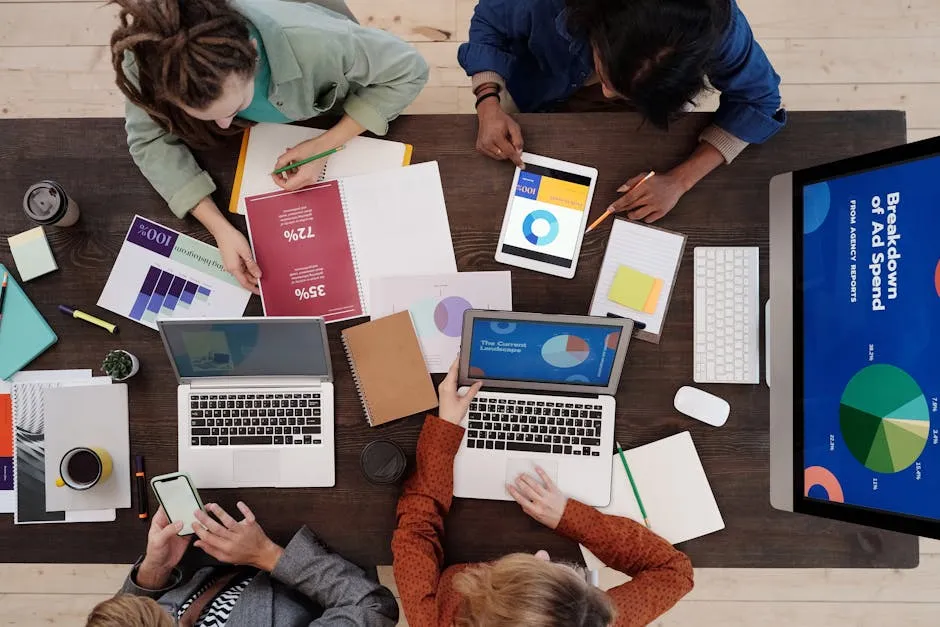
Theoretical Foundations of Parametric Statistics
Definition and Importance of Parametric Statistics
Parametric statistics refers to statistical techniques that make specific assumptions about the underlying distribution of data. These methods typically require data to follow a known distribution, such as the normal distribution. Common examples include the t-test and analysis of variance (ANOVA).
The importance of parametric statistics lies in its power and efficiency. When assumptions hold true, parametric tests provide precise estimates of population parameters. They also tend to have greater statistical power, meaning they are more likely to detect true effects compared to non-parametric methods. This is critical in hypothesis testing and inference, as accurate conclusions rely on well-founded statistical models. Thus, understanding these methods is essential for researchers and analysts in various fields. If you’re looking for a solid introduction to statistics, check out “Statistics for Dummies” by Deborah J. Rumsey. It’s a great way to get your feet wet without feeling overwhelmed.

Traditional Assumptions of Parametric Tests
Parametric tests are built upon several core assumptions that must be met for the results to be valid. These assumptions include:
- Normality: Data should be normally distributed. This is especially crucial when sample sizes are small. For larger samples, the Central Limit Theorem suggests that the means of samples will approximate normality, even if the data itself does not. However, checking for normality remains a best practice.
- Equal Variance: Also known as homoscedasticity, this assumption states that the variance within each group being compared should be approximately equal. This can be visually assessed using boxplots or confirmed with tests like Levene’s test.
- Independence: Observations in each group must be independent of each other. This assumption is fundamental to ensure that the statistical tests can produce valid results. Data collected through random sampling methods typically meets this criterion.
- No Outliers: Parametric tests assume that extreme outliers do not exist in the data. Outliers can disproportionately affect the results, leading to misleading conclusions. Visualization techniques, such as boxplots, can help identify and assess the influence of outliers.
These assumptions are essential for the statistical validity of parametric tests. If any of these conditions are violated, it can lead to inflated Type I error rates or underpowered tests, ultimately hindering the reliability of the findings. Thus, it’s crucial for researchers to assess these assumptions before conducting parametric analyses to ensure sound conclusions are drawn from their data.
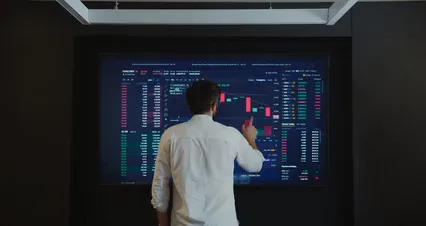
Emerging Trends in Parametric Assumptions
Non-Normal Data Handling
The rise of big data has brought a significant challenge: non-normal data. As the volume and complexity of data increase, so does the likelihood that it won’t conform to traditional distribution assumptions. This shift has prompted statisticians to rethink how they approach parametric statistics.
To address the growing prevalence of non-normal data, several techniques have emerged. Firstly, statisticians can test for normality using methods like the Shapiro-Wilk test. If data does not meet the normality assumption, researchers can employ transformations, such as logarithmic or square root transformations, to stabilize variance and make the data more normal-like.
Alternatively, robust statistical methods have gained traction. These methods are designed to be less sensitive to violations of assumptions, providing reliable results even when data deviate from normality. If you’re interested in deepening your understanding of these robust techniques, consider “Practical Statistics for Data Scientists” by Peter Bruce. This book covers essential concepts and techniques that every data scientist should know.
Additionally, machine learning approaches are increasingly being integrated into traditional parametric frameworks. Machine learning algorithms can handle non-normal distributions effectively, allowing for a blend of statistical rigor and the flexibility needed for complex datasets. This integration is particularly valuable as it allows for the use of parametric methods in scenarios where traditional assumptions are not met.
In summary, the landscape of parametric statistics is evolving to accommodate the realities of big data. By embracing new methods and techniques, statisticians can ensure their analyses remain relevant and insightful in a data-rich world.
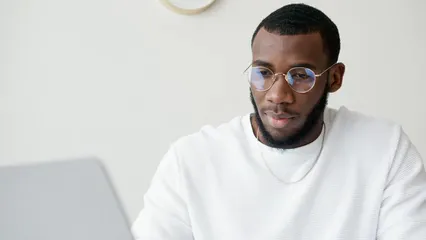
Machine Learning Integration
Machine learning is shaking up the world of parametric statistics. It’s not just a trendy buzzword; it’s transforming how we view data assumptions. Traditional statistical methods often require strict adherence to normality and equal variance. But machine learning is like that friend who brings a new perspective to the table. It offers tools that adapt to the data, allowing statisticians to use techniques that were once deemed too rigid.
Take healthcare analytics, for instance. Researchers are applying machine learning to predict patient outcomes based on vast datasets. A notable case study involved using machine learning models to analyze patient records. The result? Enhanced accuracy in identifying at-risk patients. This integration of machine learning with parametric statistics is not just theoretical; it’s practical and impactful, leading to better healthcare decisions. If you’re interested in learning more about machine learning, check out “Machine Learning Yearning” by Andrew Ng. This book is a must-read for anyone looking to understand the nuances of machine learning.
In finance, machine learning models analyze stock market trends, often revealing patterns that traditional statistics might miss. By blending machine learning with parametric tests, analysts improve their predictions, leading to more informed investment strategies. Such case studies showcase the successful marriage of these two powerful methodologies, proving that when statistics and machine learning unite, remarkable insights arise.
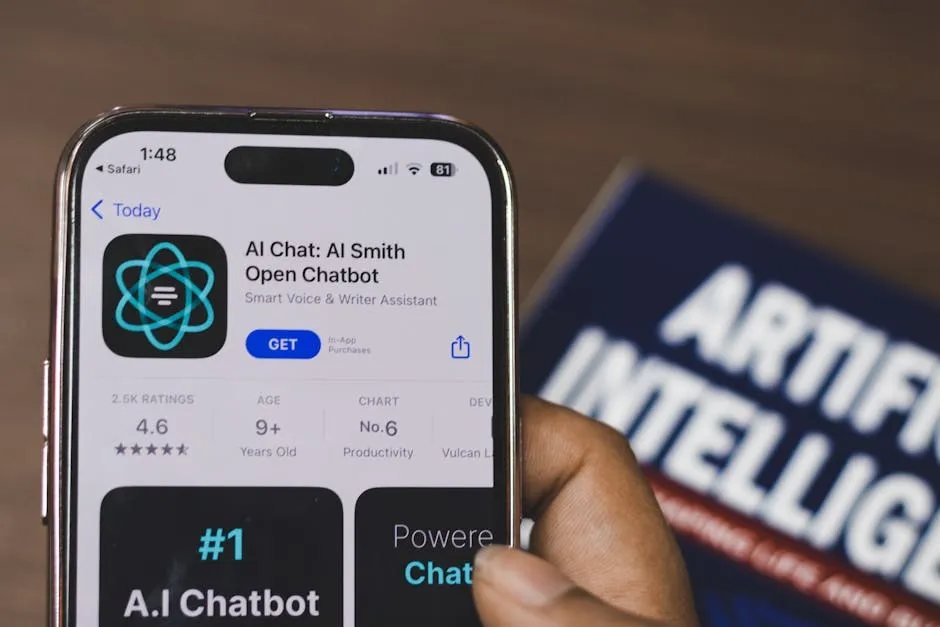
Data Visualization Techniques
Data visualization is the unsung hero in the realm of big data. Without it, understanding complex data distributions would feel like trying to read hieroglyphics. The importance of visualization lies in its ability to transform raw data into intuitive formats, making it accessible to everyone, from data scientists to decision-makers.
Effective visualization techniques help reveal underlying patterns and trends. For example, heatmaps can illustrate correlations, while boxplots can highlight outliers and distributions. In big data contexts, tools like Tableau and Power BI are game-changers. They empower users to create interactive dashboards that provide real-time insights, making data comprehensible and actionable. If you’re a beginner looking to get started with Tableau, consider “Tableau Desktop 2021: A Beginner’s Guide”. This guide will walk you through the basics to help you create stunning visualizations.
Moreover, the integration of advanced visualization tools allows statisticians to convey complex statistical findings with clarity. Imagine presenting a complicated dataset through a few well-placed graphics rather than pages of numbers. It’s not just about aesthetics; it’s about communication. Visualization helps bridge the gap between statistical analysis and practical application, ensuring that insights lead to informed decisions.
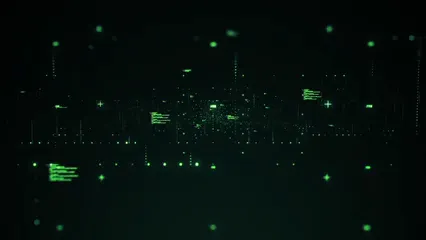
Advanced Computational Techniques
Role of Big Data Technologies
Big data technologies are the superheroes of statistical analysis. They are the backbone behind the scenes, enabling researchers to tackle the enormous datasets that have become commonplace. Tools like Hadoop and Spark are not just fancy jargon; they play a crucial role in making sense of big data.
Hadoop allows for distributed storage and processing, making it possible to analyze vast amounts of data across multiple systems. This means statisticians can run complex computations without the fear of crashing their systems. Spark, on the other hand, accelerates data processing tasks, allowing real-time analytics. Imagine being able to analyze data as it’s generated—that’s the power of Spark.
These technologies facilitate the handling of large datasets and complex computations, making it easier for researchers to validate or challenge parametric assumptions. For example, when analyzing patient data from numerous hospitals, traditional methods would struggle to process such volumes. However, big data technologies streamline this process, allowing for robust statistical analysis even with non-normal data distributions.
Moreover, the scalability of these technologies means that as data grows, so too does the capacity to analyze it. The result? More reliable insights that can drive significant changes in fields like healthcare, finance, and beyond. The synergy between big data technologies and parametric statistics is essential for navigating the complexities of modern data landscapes, making advanced computational techniques a cornerstone of effective analysis.

Simulation and Bootstrapping Methods
When it comes to assessing parametric assumptions, simulation techniques offer a goldmine of possibilities. These methods provide a robust framework for evaluating how well data meets the necessary assumptions for parametric tests. By simulating data under various conditions, researchers can observe the behavior of statistical tests in situations that mimic real-world complexities. For example, using Monte Carlo simulations, analysts can generate thousands of datasets to see how often a statistical test might yield a false positive when assumptions are violated. This approach not only enhances understanding but also informs better decision-making in the context of data analysis.
Bootstrapping, on the other hand, is like having a magic wand for making inferences without strictly adhering to traditional assumptions. This resampling method allows researchers to create ‘new’ datasets by repeatedly sampling from the original data. By doing this, they can estimate the sampling distribution of almost any statistic. This is particularly useful when dealing with small sample sizes or when the data does not meet normality assumptions. If you’re interested in learning more about the bootstrapping technique, the book “Data Science from Scratch” by Joel Grus offers insights into these techniques and more.
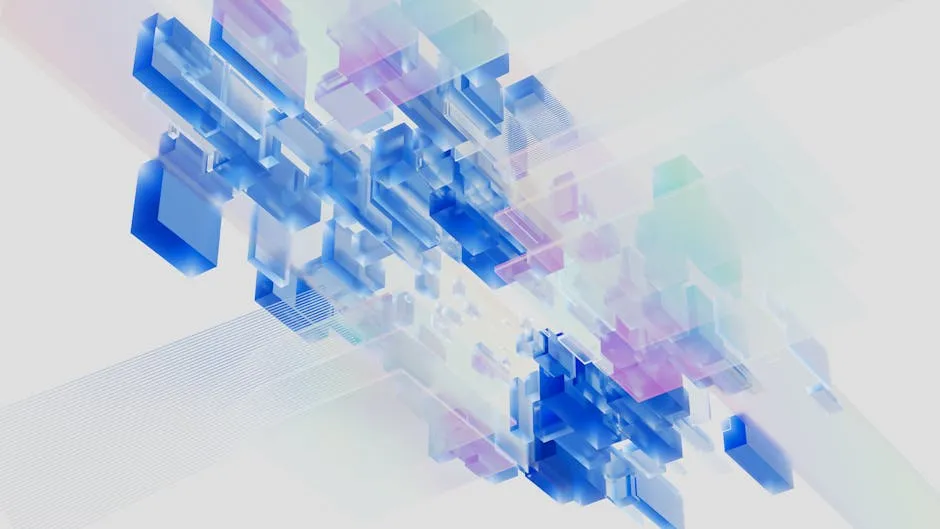
Practical Applications in Various Industries
Case Study: Healthcare
Emerging trends in parametric assumptions are making waves in healthcare analytics. With the vast amounts of data generated from electronic health records and clinical trials, traditional statistical methods often fall short. Enter advanced parametric techniques that adapt to the nuances of healthcare data.
For example, a recent study used flexible parametric models to analyze patient outcomes after surgery. By accommodating non-normal data distributions, researchers identified critical factors influencing recovery rates. This led to a targeted approach in post-operative care, resulting in a 20% reduction in recovery time for patients. Another instance involves predictive modeling for chronic disease management. By integrating machine learning with parametric methods, healthcare providers can now predict flare-ups in conditions like asthma or diabetes, enhancing proactive care and improving patient outcomes significantly.
These advancements underscore the importance of modern statistical methods in healthcare. As data continues to grow, the ability to adapt parametric assumptions will play a crucial role in driving improvements in patient care and clinical decision-making. If you’re keen on delving deeper into healthcare data analytics, “Deep Learning” by Ian Goodfellow provides a comprehensive look at how deep learning techniques can be applied in healthcare.

Case Study: Finance
In finance, the application of flexible parametric assumptions is reshaping the landscape of risk assessment and investment strategies. Traditional models often assume normal distribution of asset returns, which can lead to severe miscalculations, especially during market volatility.
A notable case involved a hedge fund that adopted advanced parametric techniques to model asset returns. By relaxing the assumption of normality and incorporating fat-tailed distributions, the fund improved its risk assessment models. This adjustment enabled them to forecast potential losses more accurately during economic downturns, resulting in a 15% increase in returns over a year compared to their previous models. Explore statistical methods for finance professionals.
This adjustment enabled them to forecast potential losses more accurately during economic downturns. Explore statistical methods for finance professionals.
Moreover, financial analysts are leveraging machine learning alongside parametric methods to enhance portfolio optimization. By analyzing historical data with advanced statistical techniques, they can identify patterns that inform better investment choices. This combination of flexible parametric assumptions and machine learning not only improves risk management strategies but also enhances overall portfolio performance.
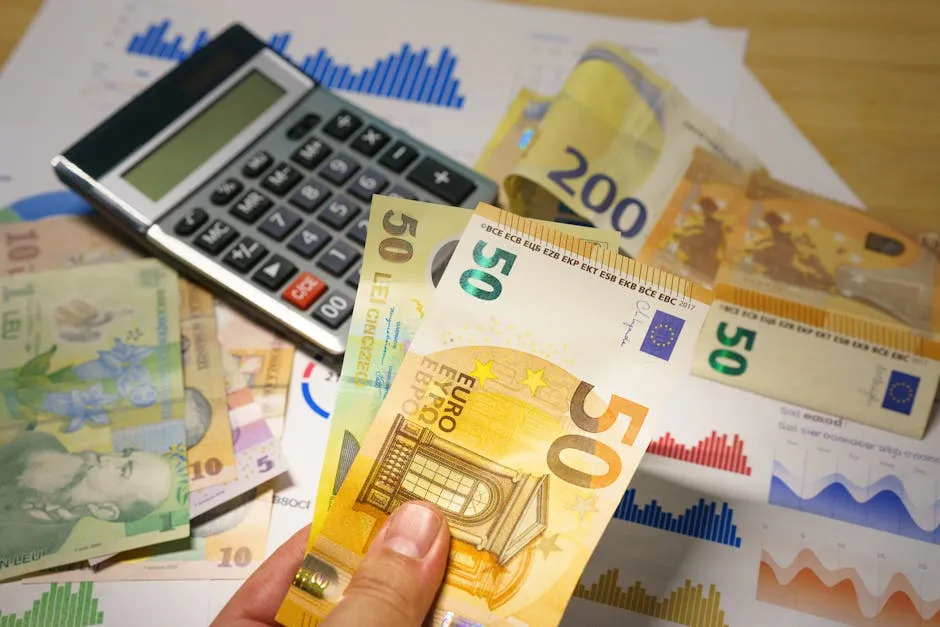
Case Study: Social Sciences
The social sciences are not immune to the transformative effects of emerging trends in parametric assumptions. Researchers are increasingly adapting their statistical methodologies to better align with the multifaceted nature of social data. Traditional assumptions often fail to capture the rich complexity of human behavior, leading to oversimplified conclusions.
A case study in sociology highlighted the use of advanced parametric methods to analyze survey data on community engagement. By relaxing the normality assumption, researchers could better understand the factors influencing participation in local governance. The results revealed unexpected insights, such as the role of social media in mobilizing younger demographics. This knowledge has informed community leaders on how to engage diverse populations effectively. Discover Agresti’s statistical methods for the social sciences.
This knowledge has informed community leaders on how to engage diverse populations effectively. Discover Agresti’s statistical methods for the social sciences.
Furthermore, policymakers are utilizing these advanced statistical methods to evaluate the effectiveness of social programs. By tailoring parametric assumptions to fit real-world data, they can make more informed decisions that enhance the societal impact of their initiatives.
In summary, the applications of emerging trends in parametric assumptions span various industries. From healthcare to finance and social sciences, the ability to adapt statistical methodologies to fit the complexities of big data is proving invaluable. As we continue to advance in the age of data, embracing these trends will be essential for generating meaningful insights and driving progress across sectors.

Conclusion
As we navigate the complexities of big data, it is essential to acknowledge the emerging trends reshaping parametric statistics assumptions. These trends not only enhance the robustness of statistical analyses but also offer new avenues for insights across various domains. Embracing these changes will empower researchers and practitioners to extract meaningful information from data, ultimately leading to better decisions and outcomes. The future of statistical analysis lies in flexibility, adaptability, and a willingness to innovate.
The shift toward accommodating non-normal data distributions is paramount. Traditional methods often falter when faced with the intricacies of real-world data. Emerging techniques, such as robust statistical methods and machine learning integration, address these challenges head-on. By utilizing these advanced tools, researchers can make powerful predictions and draw actionable insights.
In addition, the role of data visualization cannot be overstated. It serves as a bridge between complex data and clear insights, ensuring that findings are communicated effectively. This is especially crucial in fields like healthcare and social sciences, where understanding data can lead to significant improvements in policy and practice.
Ultimately, the evolution of parametric statistics in the age of big data is a testament to the field’s resilience and adaptability. As we continue to embrace new methodologies, we open doors to previously unimaginable insights. This transformation will not only enhance our understanding of various domains but also facilitate more informed decision-making, paving the way for a brighter future driven by data-informed strategies.
FAQs
What are parametric statistics?
Parametric statistics are techniques that assume data follows specific distributions. They rely on parameters like mean and variance to make inferences about populations.
Why are traditional assumptions being challenged?
Traditional assumptions are being challenged due to the evolving nature of data, which often does not conform to expected distributions. This requires flexible methodologies that can accommodate a wider variety of data types.
How does machine learning affect parametric statistics?
Machine learning enhances parametric statistics by providing tools that can handle complex data structures. This integration allows for refined analyses and improved predictive capabilities.
What are some practical applications of these trends?
Practical applications span multiple industries, such as healthcare analytics, where advanced statistical methods lead to better patient outcomes, and finance, where risk assessments improve investment strategies. These examples showcase the real-world implications of emerging trends in statistics.
Please let us know what you think about our content by leaving a comment down below!
Thank you for reading till here 🙂
All images from Pexels