Introduction
Statistics is the unsung hero of our data-driven world. From helping businesses make informed decisions to guiding scientific research, statistics plays a pivotal role in various fields. This article aims to introduce essential statistics as a vital tool for understanding and analyzing data, regardless of your background. Whether you’re a student, a professional, or just a curious mind, grasping the basics of statistics can be immensely beneficial.
Why is statistics so important, you ask? Well, think of it this way: every day, we encounter data in multiple forms. Whether you’re checking the weather forecast, analyzing financial reports, or even scrolling through social media, statistics is at play. It helps us interpret information, identify trends, and make decisions based on evidence rather than gut feelings. A sound understanding of statistics empowers individuals to evaluate claims critically and discern facts from mere opinions.
In this guide, we’ll embark on a journey through the essential concepts of statistics. We’ll start by defining what statistics is and explore its significance in research and data analysis. Then, we’ll dive into the two main types of statistics: descriptive and inferential. Each section is designed to build your knowledge step by step, ensuring you leave with a clear understanding of the subject.
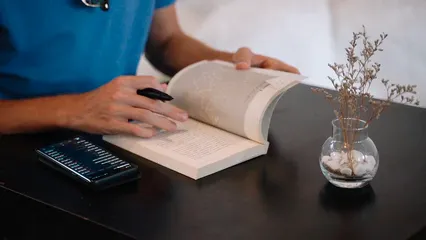
So, grab your favorite beverage, settle in, and let’s unravel the wonders of essential statistics together! And if you want to kickstart your journey, consider picking up “Statistics for Dummies” by Deborah J. Rumsey. It’s a great way to start understanding the basics!
Understanding Statistics
What is Statistics?
Statistics is the science of collecting, analyzing, and interpreting data. It transforms raw numbers into meaningful insights, guiding informed decisions across various sectors. In research, statistics helps summarize findings, validate hypotheses, and predict future trends. It’s like having a trusty sidekick that always provides clarity amidst chaos.
Statistics can be categorized into two main types: descriptive and inferential. Descriptive statistics focuses on summarizing and presenting data in an understandable way. Think of it as the spotlight shining on the data, highlighting key features and trends. It includes measures like the mean, median, mode, and standard deviation, which help describe the central tendency and variability of the data.
On the other hand, inferential statistics takes it a step further. It allows us to make predictions or generalizations about a larger population based on a sample of data. This type of statistics is crucial for hypothesis testing, where researchers determine the likelihood that their observations are due to chance. It involves concepts such as confidence intervals and p-values, which help assess the reliability of the conclusions drawn from the data.
Understanding these two branches of statistics is essential for anyone looking to navigate the world of data analysis effectively. With descriptive statistics, we gain insight into what the data tells us. With inferential statistics, we can make informed predictions and decisions based on that data. Together, they form the foundation of statistical reasoning, equipping us with the tools to interpret the vast amounts of information we encounter daily. For a deeper dive into this subject, check out “Naked Statistics: Stripping the Dread from the Data” by Charles Wheelan. It’s a witty read that makes statistics fun!
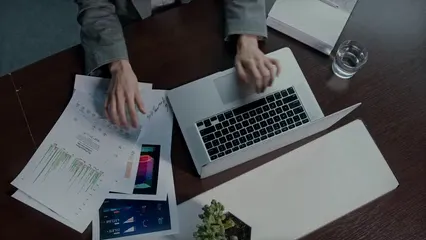
The Role of Statistics in Various Fields
Statistics is the backbone of various fields, working behind the scenes to influence decisions and strategies. In healthcare, for instance, it helps in analyzing clinical trial data to determine the effectiveness of new treatments. Doctors rely on statistical methods to understand patient outcomes and improve care.
In social sciences, statistics plays a crucial role in research. It helps researchers gather insights about human behavior, societal trends, and cultural phenomena. Surveys often use statistical sampling techniques to ensure that the data collected is representative of the larger population. For those looking to understand these methods more thoroughly, “The Art of Statistics: Learning from Data” by David Spiegelhalter is a fantastic resource.
Businesses utilize statistics to make data-driven decisions. From market research to sales forecasts, statistical analysis helps companies understand consumer behavior and optimize their strategies. For example, A/B testing allows businesses to compare two versions of a product or advertisement to see which performs better. You can learn more about statistical methods that are vital for such analyses.
Statistical methods are essential for businesses to analyze data effectively. statistical methods for finance professionals
Engineering also benefits from statistical methods, particularly in quality control and reliability testing. Engineers use statistical tools to analyze the performance of materials and design processes, ensuring safety and efficiency in their projects. If you’re interested in learning more about the importance of statistics in engineering, consider checking out “Practical Statistics for Data Scientists: 50 Essential Concepts” by Peter Bruce.
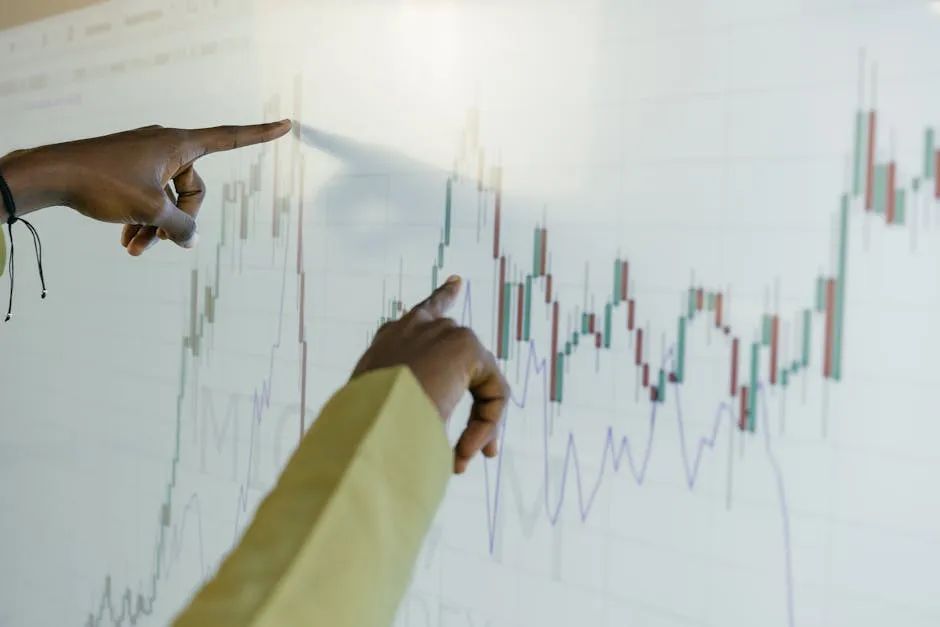
Real-world Examples
Consider a pharmaceutical company developing a new drug. They conduct randomized controlled trials, using statistics to analyze the results and ascertain whether the drug is effective compared to a placebo. The results guide regulatory approval and market entry.
In education, standardized testing is another example of applied statistics. Schools use data from tests to evaluate student performance and improve teaching methods. This data helps identify areas where students struggle, enabling educators to tailor their approaches. To understand the role of statistics in education further, check out “Statistics for Healthcare Professionals”.
Lastly, think about sports analytics. Teams analyze player performance data to make strategic decisions about game plans and player acquisitions. By utilizing statistical models, teams can gain a competitive edge in their performance. If you want to dive deeper into sports statistics, consider “Statistics Done Wrong” by Alex Reinhart for a humorous take on common statistical pitfalls.
Statistics is everywhere, influencing decisions and shaping outcomes across various fields. Understanding its role is essential for anyone looking to navigate our increasingly data-driven world.
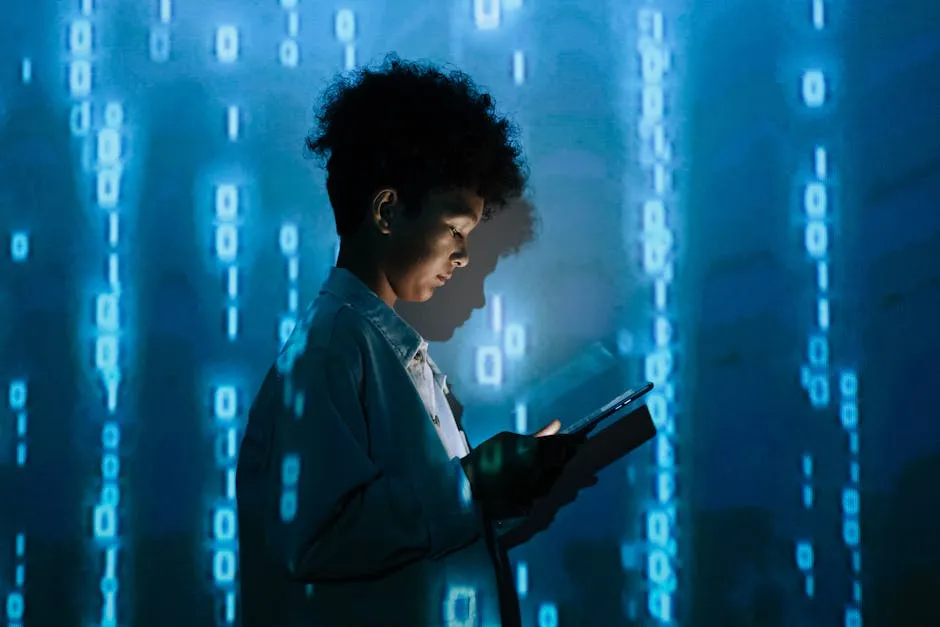
Inferential Statistics
Inferential statistics is a fascinating branch of statistics. It allows us to draw conclusions about a population based on a sample. Imagine you’re at a party and want to know how much fun everyone is having. Instead of asking every guest, you ask just a few. Using the responses, you can infer how the entire party feels. This ability is crucial in research and data analysis, where drawing accurate conclusions from limited data is essential. Understanding inferential statistics helps in making informed decisions in fields like healthcare, marketing, and social sciences. You can explore more about the problem with inferential statistics to get deeper insights.
Understanding inferential statistics is vital for making informed decisions based on sample data. the problem with inferential statistics

Hypothesis Testing
Hypothesis testing is like a courtroom drama, where we have two opposing sides: the null hypothesis (H0) and the alternative hypothesis (H1). The null hypothesis posits that there is no effect or no difference, while the alternative suggests otherwise. To determine which side has the stronger case, we set a significance level, commonly denoted as alpha (α), often set at 0.05. This means we are willing to accept a 5% chance of incorrectly rejecting the null hypothesis.
Next comes the p-value, which tells us how compatible our sample data is with the null hypothesis. If the p-value is lower than α, we reject H0, suggesting that our findings are statistically significant. Think of it as the moment the jury reaches a verdict, only this time, it’s based on data rather than drama!

Confidence Intervals
Confidence intervals (CIs) are like a safety net for estimates. They provide a range of values that likely contain the true population parameter. For instance, if a study gives a 95% CI of (10, 20) for the average height of a group, we can be 95% confident that the actual average height lies within this interval. It’s a way of saying, “We might not know the exact number, but we’re pretty sure it’s somewhere in here!” CIs offer valuable insights, especially when making predictions or planning. For a more comprehensive understanding of confidence intervals, consider reading “Statistical Power Analysis for the Behavioral Sciences” by Jacob Cohen.

Probability
Basic Concepts
Probability is the backbone of statistics. It quantifies uncertainty and helps us make informed decisions. Let’s say you flip a coin. The probability of it landing heads is 0.5, while the chance of tails is also 0.5. This probability shows us the likelihood of random events occurring. Understanding probabilities allows us to navigate randomness in everyday life, from predicting weather to betting on sports. If you’re interested in getting a solid foundation in probability, check out “Introduction to Probability” by Dimitri P. Bertsekas.
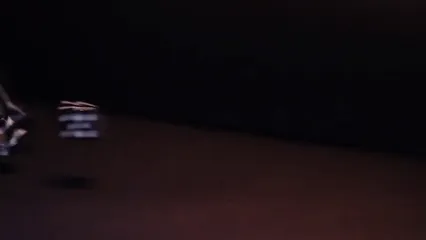
Probability Distributions
Probability distributions describe how probabilities are allocated across different outcomes. Three common types are normal, binomial, and Poisson distributions.
- Normal Distribution: This bell-shaped curve is ubiquitous in statistics. It represents data where most values cluster around a central mean, with fewer values as you move away. Think of heights in a population: most people are of average height, with fewer very tall or very short individuals.
- Binomial Distribution: This distribution applies to experiments with two possible outcomes, like flipping a coin. If you flip a coin 10 times, you can calculate the probability of getting a certain number of heads using the binomial distribution.
- Poisson Distribution: This distribution models the number of events happening in a fixed interval of time or space. For instance, it can help predict how many customers might arrive at a store in an hour.
Understanding these distributions allows us to analyze and interpret data effectively, providing a foundation for more complex statistical methods. If you want to explore these concepts in more depth, consider “The Complete Guide to Statistical Analysis”.

Key Concepts in Essential Statistics
Types of Data
Quantitative vs Qualitative
Data comes in various flavors, but it generally falls into two categories: quantitative and qualitative. Quantitative data is all about numbers. Think heights, weights, or test scores. It allows for mathematical operations like addition or averaging. On the flip side, qualitative data is descriptive. It includes categories, labels, or traits like colors, names, or preferences. While you can count qualitative data, you can’t perform calculations on it like you can with quantitative data. In a nutshell, if it’s a number, it’s quantitative; if it’s a word, it’s likely qualitative!
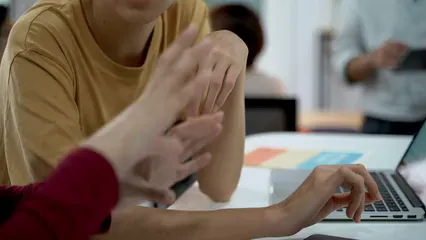
Data Scales
Now, let’s break down data scales: nominal, ordinal, interval, and ratio. Nominal scales are the simplest. They categorize data without any order—like your favorite ice cream flavors. Moving up, ordinal scales rank data but don’t specify the distance between ranks. For example, think of class rankings. Next, we have interval scales, which measure differences between values but lack a true zero point. Temperature is a classic example here. Lastly, ratio scales are the most informative. They possess all the characteristics of interval scales, plus a true zero. Height and weight fit in this category like a glove!
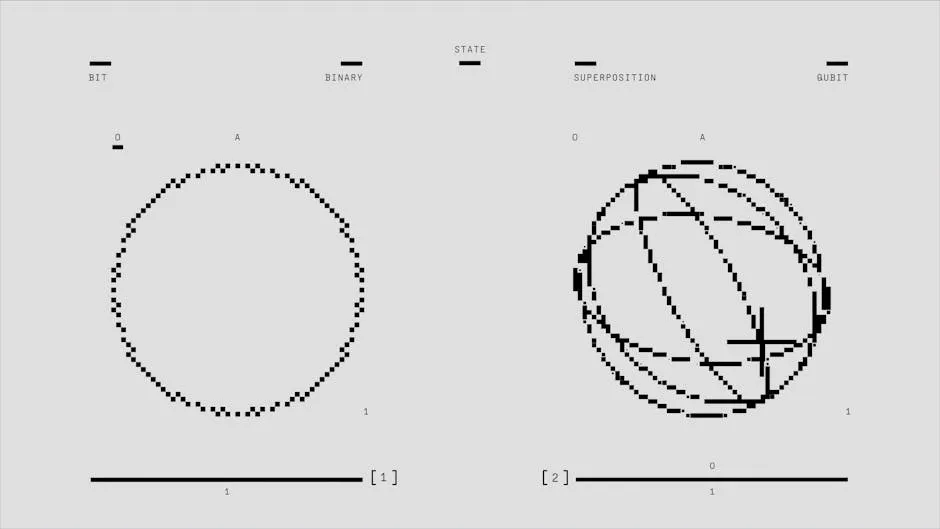
Methods of Data Collection
Surveys and Experiments
When it comes to collecting data, surveys, experiments, and observational studies are the stars of the show. Surveys gather information from a group through questionnaires or interviews. They’re super handy for capturing opinions or experiences. Experiments, on the other hand, involve manipulating variables to see their effects—like testing a new drug against a placebo. Observational studies let researchers watch subjects in their natural environment without interference. Each method has its strengths, and the choice often depends on the research question at hand. For a comprehensive look at these methods, consider “Discovering Statistics Using IBM SPSS Statistics” by Andy Field.
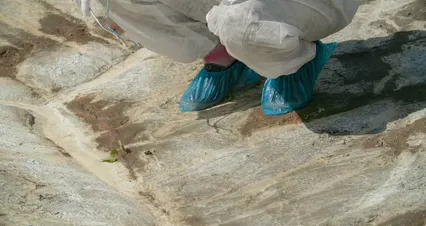
Sampling Techniques
Sampling techniques are essential in ensuring data is representative. Random sampling is the gold standard. It gives everyone an equal chance of being chosen, which reduces bias. Stratified sampling divides the population into subgroups, ensuring all are represented—imagine sampling students from different grades. Convenience sampling is the easy way out, selecting subjects that are readily available. While it’s simple, it often introduces bias. Each technique has its pros and cons, and understanding them helps in selecting the right approach for your study. If you’re curious about sampling methods, you might want to check out “Statistical Methods for the Social Sciences” by Alan Agresti.

Data Analysis Techniques
Data Visualization
Visualizing data is like giving it a new outfit—suddenly, it’s much more appealing! Charts, graphs, and plots help reveal trends and patterns that numbers alone can’t convey. A well-designed graph can make complex data digestible and engaging. Consider pie charts for showing proportions or line graphs for trends over time. The key takeaway? Visuals enhance understanding and retention, making them a must-have in data analysis. For more on data visualization techniques, you might explore “Excel Statistics: A Quick Guide” by Neil J. Salkind.
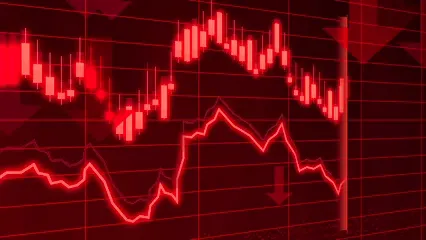
Statistical Software
In the realm of data analysis, statistical software is your trusty sidekick. Tools like SPSS, R, and Excel streamline the analysis process. SPSS is user-friendly and widely used in social sciences, while R offers powerful statistical computing capabilities, perfect for the more adventurous. Excel, meanwhile, is familiar to many and perfect for basic analyses and visualizations. Each software has its unique features, and choosing the right one can make your data analysis journey smoother and more efficient. If you’re keen to learn R, consider grabbing “The R Book” by Michael J. Crawley.

Common Statistical Tests
T-tests
Definition and Types
T-tests are statistical tests used to compare the means of different groups. They help determine if the observed differences are statistically significant. There are three main types of t-tests:
- One-Sample T-test: This test compares the mean of a single sample to a known value (like a population mean). For instance, if you want to check if the average height of your class is different from the average height of the population, you’d use this test.
- Independent T-test: This compares the means of two independent groups. Think of comparing test scores between two different classes. If you want to see if one class performed better than the other, the independent t-test comes into play.
- Paired Sample T-test: This test is used when you have two related groups. For example, measuring the weights of individuals before and after a diet plan. It helps identify if the diet had a significant impact on their weights.
T-tests are ideal when you have small sample sizes (typically under 30), and the data follows a normal distribution. Use a one-sample t-test when comparing a sample mean to a known value. An independent t-test is your go-to when comparing means from two different groups. If your data is paired, like before-and-after measurements, the paired sample t-test is the way to go. For further reading on t-tests, you might find “Statistical Methods for Research Workers” by Ronald A. Fisher beneficial.
ANOVA (Analysis of Variance)
Overview
ANOVA, short for Analysis of Variance, is a statistical method used to compare means among three or more groups. It helps determine if at least one group mean is different from the others. Imagine a researcher trying to test the effectiveness of three different teaching methods. ANOVA can reveal if one method stands out in terms of student performance. For a deeper understanding of ANOVA, check out “The Analysis of Variance” by R. L. Hogg.
Types of ANOVA
- One-Way ANOVA: This is used when comparing means across a single factor. For instance, if you want to compare scores from different teaching methods, one-way ANOVA can show if there’s a significant difference due to the method used.
- Two-Way ANOVA: This method examines the influence of two different factors on a dependent variable. For example, if you want to study how teaching method and study time together affect student scores, two-way ANOVA would be appropriate. It also reveals if there’s any interaction between the factors.
Regression Analysis
Introduction to Regression
Regression analysis is a powerful statistical method used for predicting outcomes based on one or more predictor variables. This method helps establish relationships between variables, making it essential for forecasting. For example, if you want to predict sales based on advertising spend, regression analysis can provide insights into how changes in advertising might affect sales. To get started with regression analysis, you might find “Elements of Statistical Learning” by Trevor Hastie quite useful.
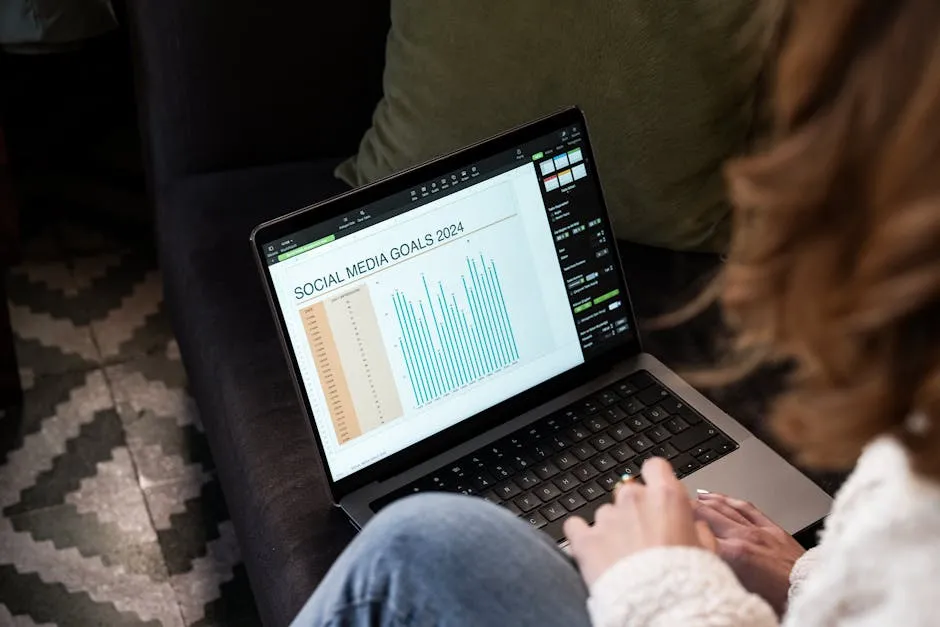
Linear vs Non-linear Regression
- Linear Regression: This is the simplest form, where the relationship between the variables is represented as a straight line. It’s like saying, “As advertising increases, sales also increase.” The equation takes the form of Y = a + bX, where Y is the predicted value, X is the predictor, and a and b are constants.
- Non-linear Regression: This type is used when the relationship between the variables is not linear. Think of a scenario where the effect of advertising on sales increases at a decreasing rate. Non-linear regression can capture this relationship, providing a more accurate model.
Practical Applications of Statistics
Case Studies
Healthcare
In healthcare, statistics plays a crucial role in clinical trials and epidemiological studies. For instance, when testing a new drug, researchers use statistical methods to analyze data from trials. This helps determine the drug’s efficacy and safety, guiding decisions on whether it should be approved for public use. Moreover, epidemiological studies rely on statistics to understand disease patterns and risk factors, ultimately aiding in public health interventions. To understand the significance of statistics in healthcare, you might find “The Essentials of Biostatistics for Physicians, Nurses, and Clinicians” by Michael R. Chernick enlightening.
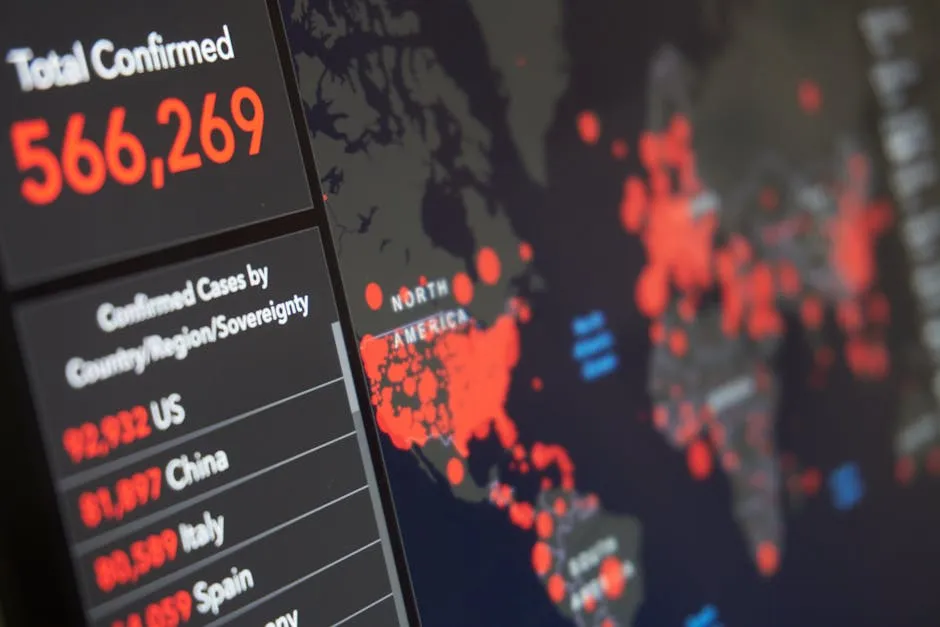
Business
Statistics is indispensable in business for market research and quality control. Companies utilize statistical analysis to understand consumer preferences and behaviors. For example, a business might conduct surveys and analyze the results to inform product development. Furthermore, quality control processes often use statistical methods to monitor production quality, ensuring products meet specified standards and minimizing defects. If you want to delve into business statistics, consider “Statistics for Business and Economics” by Paul Newbold.
Importance of Statistical Literacy
Critical Thinking
Understanding statistics enhances critical thinking and decision-making skills. It allows individuals to evaluate information critically and recognize biases or misinterpretations in data. In an age where misinformation is rampant, being statistically literate empowers people to discern facts from fiction. If you’re interested in honing your critical thinking skills through statistics, consider reading “Statistics in Plain English” by Timothy C. Urdan.

Data-Driven Decision Making
Making decisions based on data is crucial in various sectors. Businesses rely on statistical analysis to guide marketing strategies, product development, and customer service improvements. In healthcare, data-driven decisions can lead to improved patient outcomes and efficient resource allocation. Emphasizing the importance of statistical literacy is essential for fostering a culture of informed decision-making across fields. To further enhance your understanding of data-driven decisions, consider “How to Lie with Statistics” by Darrell Huff, which cleverly illustrates how statistics can be misused.
Please let us know what you think about our content by leaving a comment down below!
Thank you for reading till here 🙂
All images from Pexels