Introduction
Normality is crucial in statistical analysis. It helps ensure valid conclusions, especially in crop yield data. The Grubbs test is a robust method to assess normality and identify outliers. Accurate data analysis is vital for effective agricultural research and practices.
To further enhance your agricultural research, consider adding a Data Collection Tool for Agricultural Research. These tools can streamline your data gathering process, making it more efficient and reliable!
Summary and Overview
The Grubbs test, developed by Frank E. Grubbs in 1950, is widely used in agricultural studies. It assesses whether a dataset follows a normal distribution while detecting outliers. Normality testing is essential for crop yield analysis, as non-normal data can skew results. Detecting outliers helps improve assessments of crop yields, enabling better agricultural decisions.
Speaking of agricultural decisions, having a solid Agricultural Statistics Textbook can provide you with the theoretical foundations needed to interpret your data effectively.
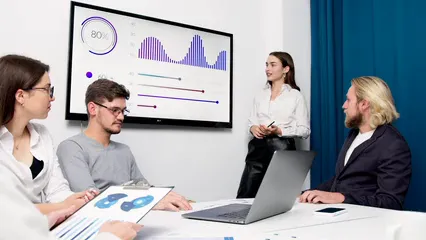
Understanding the Grubbs Test
What is the Grubbs Test?
The Grubbs test is a statistical procedure designed to identify outliers in a dataset assumed to be normally distributed. It works by comparing each data point to the sample mean. The test calculates a statistic based on the largest deviation from the mean. If the calculated statistic exceeds a critical value, the point is considered an outlier. This process allows researchers to refine their analyses and ensure data integrity. The Grubbs test is particularly effective when working with small sample sizes, making it ideal for agricultural research where data collection can be limited.
Before diving deeper, ensure your Statistical Methods for Agriculture Research are solid! This resource can guide you through the complexities of data analysis in agriculture.
Importance of Normality in Crop Yield Data
Normality is vital in statistical analyses and hypothesis testing. When crop yield data follows a normal distribution, it ensures the validity of statistical results. Many statistical methods assume normality, and violating this assumption can lead to inaccurate conclusions.
For instance, if crop yield data is not normally distributed, it may inflate the significance of results. Imagine analyzing crop yields from different fertilizers without checking normality. If the data is skewed, you might incorrectly conclude that one fertilizer significantly outperforms others.
Non-normal data can also mislead researchers. Consider a scenario where outliers exist in yield data. These outliers can distort averages and variances, leading to misguided agricultural recommendations. Thus, assessing normality is crucial for reliable analyses in agricultural studies, ensuring decisions are made based on accurate data interpretation.

Applying the Grubbs Test
Steps to Conduct the Grubbs Test
Applying the Grubbs test to crop yield data involves several clear steps. First, prepare your data by ensuring it’s clean and organized. Remove any irrelevant entries and check for missing values.
Next, visualize the data using histograms or box plots. This will help identify potential outliers and assess normality. Once you feel confident about the data’s structure, calculate the sample mean and standard deviation.
Now, execute the Grubbs test. Calculate the Grubbs test statistic, which is the maximum absolute deviation from the mean divided by the standard deviation. Compare this statistic to critical values from Grubbs’ distribution table. If your statistic exceeds the critical value, an outlier is present.
Finally, interpret the results. If an outlier is detected, consider whether to exclude it or analyze its impact on your results. Repeat the test if necessary, removing detected outliers iteratively until no more are found. This process ensures your crop yield analysis remains robust and reliable.
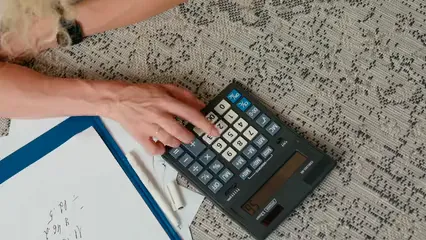
For your data visualization, you might want to explore Box Plot and Histogram Visualization Tools. These tools can provide clarity in your data presentation!
Hypotheses of the Grubbs Test
The Grubbs test involves two primary hypotheses. The null hypothesis (H0) states that there are no outliers in the dataset. Conversely, the alternative hypothesis (Ha) posits that at least one outlier exists.
Understanding these hypotheses is crucial, especially in the context of crop yield data. Validating the null hypothesis means you can confidently analyze your data without worrying about the influence of outliers. If you reject H0 in favor of Ha, it indicates that outliers may skew your results, prompting further investigation. This clarity aids in making informed agricultural decisions based on reliable data.
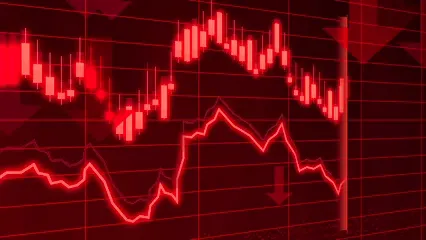
Assumptions of the Grubbs Test
The Grubbs test relies on several key assumptions for valid results. First, the data must be approximately normally distributed. This ensures that the test statistics hold true. Second, the data should be independent; each observation must not influence another. Third, the test is designed for univariate datasets. If your data has multiple variables, alternative methods should be considered.
Violating these assumptions can lead to misleading results in crop yield analysis. For instance, if the data is not normally distributed, the Grubbs test might falsely identify outliers or miss them altogether. This can skew the interpretation of crop performance and affect decision-making for farmers and researchers. Ensuring these assumptions are met is crucial for reliable analysis and effective agricultural practices.
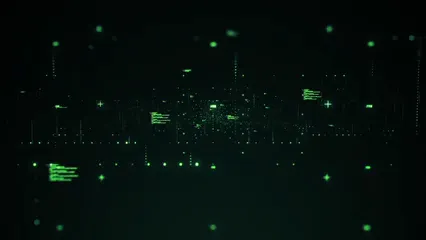
Interpreting Results
Understanding Test Statistics
The Grubbs test statistic calculates the largest deviation of a data point from the mean, expressed in terms of standard deviation. Specifically, it identifies how far the most extreme value is from the average of the dataset. The formula used is:
G = \frac{\max(|X_i - \bar{X}|)}{s}
Here, \bar{X} represents the sample mean, and s is the sample standard deviation. A higher Grubbs test statistic indicates a greater likelihood that an outlier exists.
When interpreting results, compare the calculated statistic to critical values from the Grubbs distribution table. If the statistic exceeds the critical value, you can reject the null hypothesis, suggesting the presence of an outlier. In the context of crop yield data, this means that certain yields may be significantly different from the norm, warranting further investigation.
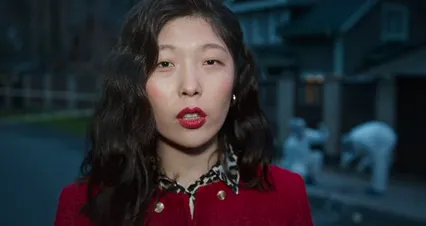
Handling Outliers Detected by the Grubbs Test
Once outliers are identified using the Grubbs test, it’s essential to handle them thoughtfully. One common strategy is to assess whether the outlier represents a genuine anomaly or results from data collection errors. If the outlier is valid, consider retaining it for further analysis, as it might provide valuable insights.
Alternatively, if the outlier is deemed erroneous, remove it and conduct the analysis again. Be cautious with exclusions, as removing too many data points can lead to biased results. Document the rationale behind your decisions, ensuring transparency in your analysis. This approach not only improves the integrity of your crop yield data but also enhances the reliability of any conclusions drawn from it.
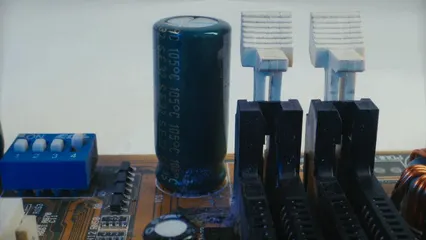
Best Practices for Implementing the Grubbs Test
Visualizing Data Before Testing
Visualizing crop yield data is crucial before applying the Grubbs test. Why? It helps you understand the data’s distribution and spot potential outliers. Using graphical methods like box plots and histograms allows you to see patterns clearly.
Box plots display median values and quartiles, highlighting potential outliers effectively. Histograms show the frequency distribution, giving insights into normality. By visualizing the data first, you enhance your analysis and ensure a more accurate application of the Grubbs test. You might want to consider a Crop Yield Analysis Handbook for deeper insights!

Complementary Tests for Normality
While the Grubbs test is powerful, it’s wise to use additional tests for normality. The Shapiro-Wilk test is a popular choice, helping to confirm your data’s distribution. This test assesses whether the data significantly deviates from a normal distribution.
Using both the Grubbs test and Shapiro-Wilk can provide a comprehensive understanding of your dataset. If both tests indicate normality, you can confidently proceed. Conversely, if one shows non-normality, consider transforming your data or using non-parametric methods. This dual approach ensures that your analysis of crop yield data is robust and reliable, leading to better agricultural decisions.
For effective data analysis, it is essential to follow tips for effective data analysis in economics and statistics.
Conclusion
In summary, the Grubbs test is an essential tool for assessing normality in crop yield data. Visualizing data before testing and using complementary tests like the Shapiro-Wilk can strengthen your analysis. Emphasizing statistical rigor is vital in agricultural research, ultimately enhancing productivity and sustainability. By applying these best practices, you can make informed decisions that positively impact crop yields and farming practices.
FAQs
What is the Grubbs test used for?
The Grubbs test is primarily used for outlier detection in statistical analysis. It helps identify data points that significantly deviate from the mean of a normally distributed dataset. In crop yield studies, this is crucial, as outliers can skew results and mislead conclusions. By applying the Grubbs test, researchers can enhance the reliability of their crop yield data, ensuring more accurate assessments and decisions.
How do I interpret the results of the Grubbs test?
Interpreting the results of the Grubbs test involves understanding test statistics and p-values. The test provides a statistic that represents the largest deviation from the mean in standard deviation units. If this statistic exceeds a critical value, the null hypothesis is rejected, indicating an outlier is present. A low p-value, typically below 0.05, also suggests statistical significance. In crop yield data, this means that certain yields are significantly different from the norm, warranting further investigation.
Can the Grubbs test be used for small sample sizes?
The Grubbs test has limitations concerning sample size. While it can be effective, it’s not ideal for very small datasets, typically those with six or fewer observations. In such cases, the test may inaccurately label most points as outliers. For small sample sizes, researchers should consider alternative methods or combine the Grubbs test with other statistical techniques to ensure valid conclusions in crop yield analysis.
What should I do if my data is not normally distributed?
If your data does not meet normality assumptions, consider alternative approaches. Transforming the data using logarithmic or square root transformations can help achieve normality. Alternatively, you might opt for non-parametric tests that do not assume a normal distribution, such as the Wilcoxon rank-sum test. These methods ensure that your analysis remains robust and valid, allowing for accurate assessments of crop yield data.
Is there software available to perform the Grubbs test?
Yes, several statistical software packages can perform the Grubbs test. Popular options include R, Python (with libraries like SciPy), and specific statistical tools like SPSS and Minitab. These software solutions streamline the testing process, making it easier for researchers to apply the Grubbs test to their crop yield data and interpret the results efficiently.
For a comprehensive understanding of agricultural practices, consider exploring a Sustainable Farming Practices Book. It’s a great resource to keep your farming methods eco-friendly and productive!
Please let us know what you think about our content by leaving a comment down below!
Thank you for reading till here 🙂
All images from Pexels