Introduction
Welcome to the world of parametric statistics! This fascinating realm is like a well-organized library—each book (data point) has its rightful place, making research a delightful journey. In social sciences, parametric statistics play a crucial role in analyzing data and drawing conclusions. However, before you start unleashing your statistical prowess, it’s essential to grasp the underlying assumptions.
Ah, assumptions! Those sneaky little gremlins that can ruin a perfectly good analysis if ignored. Picture this: you’re on a treasure hunt, armed with a map that leads to a fortune. But alas, you forget to check whether the map is accurate. The result? You end up digging in your neighbor’s garden, unearthing nothing but weeds. Ignoring parametric assumptions can lead to invalid conclusions and misleading results, much like that unfortunate dig.
So, why should you care? Understanding these assumptions ensures your findings are credible and reliable. In social sciences, where human behavior and societal trends are analyzed, the stakes are high. Misleading results can lead to misguided policies or flawed theories. Imagine basing a social program on faulty data—yikes!
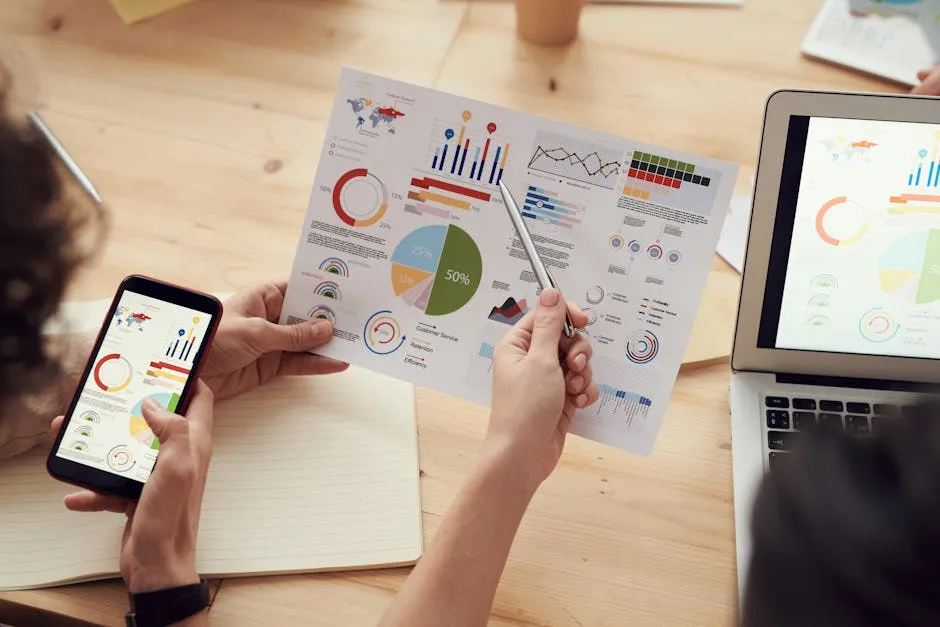
If you want to dive deeper into the world of statistics, consider picking up “The Art of Statistics: Learning from Data” by David Spiegelhalter. This book is a treasure trove of knowledge that will guide you through the intricacies of data analysis with a sprinkle of humor!
In this section, we’ll explore the significance of parametric statistics assumptions, helping researchers navigate their way to sound conclusions. Buckle up, because the journey through data analysis is about to get enlightening!
Summary
In this blog post, we will unpack the essential guidelines for parametric statistics assumptions, a topic often overlooked by researchers. By adhering to these principles, you can elevate the credibility of your research findings. We’ll discuss how proper application of these guidelines can lead to more accurate conclusions and enhance the overall quality of your studies.
Curious about what’s coming up? We’ll cover a variety of statistical tests, including t-tests, ANOVA, and correlation analyses. Each of these tests has specific scenarios where they shine. For instance, when comparing the means of two groups, a t-test is your go-to tool. ANOVA is perfect for examining differences across three or more groups. And if you’re interested in relationships between variables, correlation analysis will be your trusty companion.
By the end of this article, you’ll understand the importance of adhering to parametric assumptions. You’ll also discover practical examples that illustrate how these statistical tests can be applied in real-world social research. So, let’s dive into the nitty-gritty of parametric statistics and unlock the secrets to robust data analysis!
For a deeper understanding of statistical analysis, check out this summary statistics table.
Understanding Parametric Statistics
What Are Parametric Statistics?
Parametric statistics are a set of statistical techniques that make specific assumptions about the data. These methods rely on the idea that the data follows a particular distribution, often a normal distribution. If your data meets these conditions, you can use these techniques to draw powerful conclusions about your sample and, by extension, your population.
One of the significant advantages of using parametric methods is increased statistical power. Think of power as your analysis’s ability to detect a true effect when it exists. When the assumptions hold true, parametric tests provide more informative results than their nonparametric counterparts. They utilize parameters like means and standard deviations, allowing for nuanced interpretation of data.
In social sciences, where human behavior is often unpredictable, being able to rely on the power of parametric statistics can be a game changer. So, understanding the assumptions behind these methods isn’t just a checkbox on your research to-do list; it’s a vital component of producing high-quality, credible research.
If you’re looking for a comprehensive introduction to statistical concepts, you might enjoy “Statistics for People Who (Think They) Hate Statistics” by Neil J. Salkind. This book makes statistics approachable and fun, perfect for those who are just starting out!

In the following sections, we will break down the key assumptions necessary for parametric statistics. From normality to homogeneity of variance, we’ll explore what each assumption entails and why they are essential for valid statistical analysis. Prepare yourself for a deep dive into the world of assumptions—your research will thank you later!
Key Assumptions in Parametric Statistics
Understanding the key assumptions in parametric statistics is essential for researchers in social sciences. These assumptions ensure that data analysis yields valid and reliable results. Let’s break down each assumption and its significance, along with practical methods for assessment.
Normality
Normality refers to the distribution of data following a bell-shaped curve, known as the normal distribution. This assumption is crucial because many parametric tests rely on it. If your data isn’t normally distributed, your results might resemble a game of darts—somewhere close, but rarely hitting the bullseye.
To assess normality, researchers often use the Shapiro-Wilk test. This test evaluates whether the sample data deviates from normality. A significant result (p < 0.05) suggests that the data is not normally distributed. Visualization tools like histograms or Q-Q plots can also help. If your data points align along a straight diagonal line in a Q-Q plot, you’re golden. If they scatter like confetti, it’s time to reconsider your analysis!
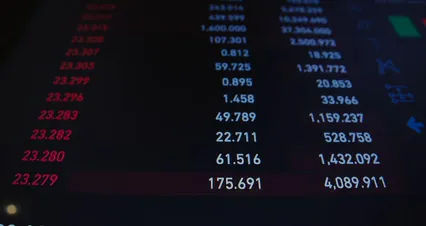
Independence
Independence is the next key player in the assumptions game. It means that the observations in your data set are not related to one another. Think of it like a game of chess; each piece moves independently, creating unique strategies. If you have paired samples—such as pre- and post-test scores from the same participants—you risk violating this assumption.
Common scenarios where independence might be compromised include nested data (like students within classrooms) or repeated measures. To address this, consider using statistical methods that account for dependencies, such as multilevel modeling or paired t-tests.
Need a reliable resource to help you navigate these concepts? Check out “Discovering Statistics Using IBM SPSS Statistics” by Andy Field. This book is a fantastic guide for anyone looking to master SPSS and statistical analysis!
Homogeneity of Variance
Homogeneity of variance, or homoscedasticity, is the assumption that different groups in your analysis have similar variances. Imagine trying to compare the height of giraffes and turtles—clearly, they have different variances! In parametric tests, unequal variances can lead to inflated Type I errors, skewing your findings.
To assess this assumption, researchers often employ Levene’s test. A non-significant result (p > 0.05) indicates that variances are equal across groups. If this assumption is violated, consider using methods like Welch’s ANOVA, which is robust to heteroscedasticity.
If you want a deeper dive into statistical methods, grab a copy of “Practical Statistics for Data Scientists: 50 Essential Concepts” by Peter Bruce and Andrew Bruce. It’s a reliable resource for understanding essential statistical concepts in data science!
By ensuring that these key assumptions—normality, independence, and homogeneity of variance—are met, researchers can confidently apply parametric statistical methods. This diligence leads to more accurate interpretations and ultimately, better-informed conclusions in social science research.

ANOVA (Analysis of Variance)
Assumptions
ANOVA, short for Analysis of Variance, is a powerful statistical tool. But, like a superhero, it has some sidekicks—its assumptions—that need to be met for it to work its magic. Let’s break down the critical assumptions of ANOVA:
- Normality: The first assumption is that the data in each group should be approximately normally distributed. Think of it as each group needing to fit that classic bell curve. If you plot your data and it resembles a rollercoaster instead, you might need to rethink your approach. You can assess normality using tests like the Shapiro-Wilk. If your results come back significant (p < 0.05), it might be time to change course.
- Homogeneity of Variance: The second assumption is that the variances among the groups should be equal. In simpler terms, if your groups were in a race, they should all be running at about the same speed. You wouldn’t want one group sprinting while another is crawling, right? Levene’s test is a handy tool to check this. A non-significant result (p > 0.05) means your variances are just fine.
- Independence: Lastly, the observations should be independent of one another. This means that the score of one participant shouldn’t influence another’s. It’s like at a dinner party; if one guest starts telling jokes, it shouldn’t affect the punchline delivery of another. If you have paired or matched samples, consider using a different test.
Meeting these assumptions is essential for ANOVA to deliver valid results. Ignoring them can lead to conclusions that are as solid as a house of cards.
For those looking to explore more about statistical analysis and its applications, check out “Statistical Methods for the Social Sciences” by Alan Agresti and Barbara Finlay. This book is perfect for social science researchers seeking to deepen their understanding of statistical methods.
Practical Examples
ANOVA shines brightest in various social science scenarios. Here are a couple of practical applications:
- Comparing Educational Methods: Imagine a researcher investigating different teaching methods’ effectiveness on student performance. They could use ANOVA to compare the test scores of students taught using traditional lectures, interactive discussions, and online modules. By analyzing the scores across these three groups, the researcher can determine if one method significantly outperforms the others.
- Evaluating Therapy Outcomes: Consider a study evaluating the effectiveness of three different therapy approaches for treating anxiety. A researcher could collect data on anxiety levels before and after treatment for each group. Using ANOVA, they can assess whether one therapy leads to greater reductions in anxiety compared to the others.
These examples illustrate ANOVA’s versatility in social science research, helping to clarify the impacts of various interventions or conditions on outcomes. With a proper understanding of its assumptions, researchers can confidently apply ANOVA to generate insights that matter.
Pearson Correlation
Overview
The Pearson correlation coefficient is like that trusty sidekick who measures the strength and direction of a linear relationship between two continuous variables. If you’re curious about how two variables dance together—whether they move in sync or completely out of step—Pearson’s your go-to. This coefficient ranges from -1 to +1. A +1 indicates a perfect positive correlation (as one variable increases, so does the other), while -1 signifies a perfect negative correlation (as one variable increases, the other decreases). A value around 0? Well, that’s the sign of two variables not sharing much of a relationship at all.
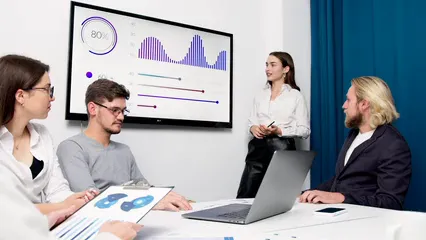
Assumptions
Before you start calculating your correlation, a few assumptions must be met to ensure your results are reliable:
- Linearity: There should be a linear relationship between the two variables. If you graph them and it resembles a straight line (or close to it), you’re good to go. If it curves like a banana, then Pearson may not be your best friend.
- Normality: Both variables should follow a normal distribution. This means if you plot the data points for each variable, they should form that lovely bell curve. Normality can be assessed with tests like the Shapiro-Wilk or visually through histograms.
- Homoscedasticity: This fancy term means that the spread of scores around the regression line should be consistent across all levels of the independent variable. If the spread varies wildly at different levels, you might want to look into transforming your data or using a different analysis technique.
By ensuring these assumptions hold true, you can confidently interpret your correlation results. And if you’re interested in exploring more about this topic, consider “Statistics: A Very Short Introduction” by David Spiegelhalter. This book offers a concise overview of statistical concepts and is perfect for quick reference!
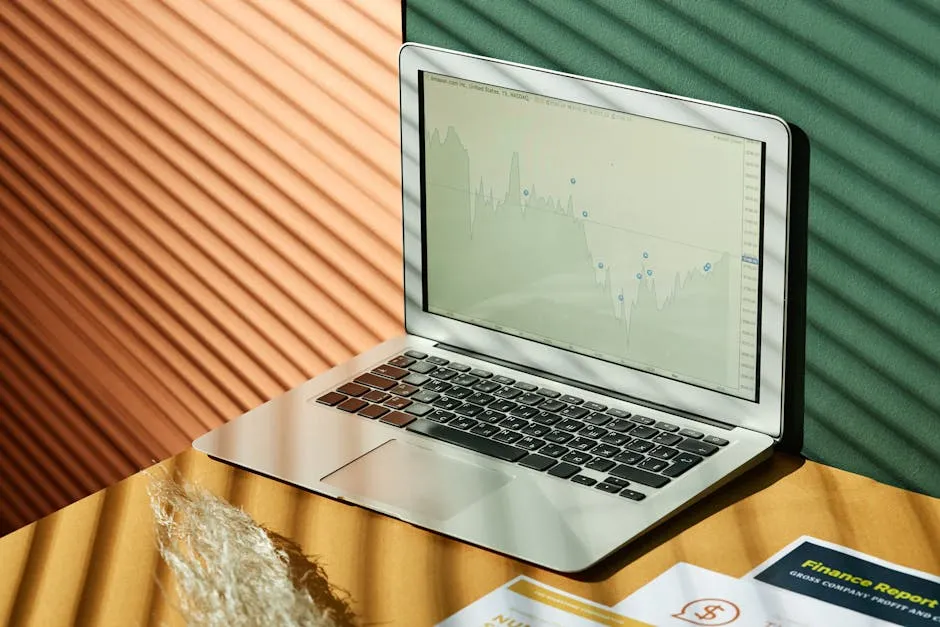
Practical Examples
The Pearson correlation can be applied in various social research contexts:
- Studying Income and Education: A researcher might examine the relationship between years of education and income levels. By calculating the Pearson correlation, they can determine if higher education correlates with increased income, providing valuable insights into societal trends.
- Analyzing Stress and Sleep: Consider a study exploring the connection between stress levels and hours of sleep. Researchers could gather data on individuals’ stress scores and the number of hours they sleep. A Pearson correlation analysis would reveal whether more stress relates to fewer hours of sleep, helping to inform mental health interventions.
These practical examples highlight the Pearson correlation’s utility in social research, allowing researchers to quantify relationships and draw meaningful conclusions about the variables at play.

When to Use Nonparametric Alternatives
Overview of Nonparametric Tests
Nonparametric tests are like the underdogs of the statistical world. They come to the rescue when parametric assumptions are violated, offering a pathway for analysis when the data doesn’t play nicely. These tests don’t require the data to follow any specific distribution, making them ideal for skewed data or smaller sample sizes. Think of them as the flexible friends who adapt to any situation, ensuring researchers can still uncover insights from their data.
Nonparametric tests, such as the Mann-Whitney U test or the Kruskal-Wallis test, are particularly useful when dealing with ordinal data or when the sample sizes are small. They allow researchers to draw conclusions without being constrained by the rigid assumptions of parametric tests. In the social sciences, where data can often be messy and complicated, these tests provide a reliable alternative.
Understanding when to use nonparametric alternatives is crucial. If your data fails the normality test, has unequal variances, or consists of ordinal measurements, it’s time to switch gears. Embracing the flexibility of nonparametric methods can lead to valuable insights, ensuring your research stands strong even in the face of data challenges.
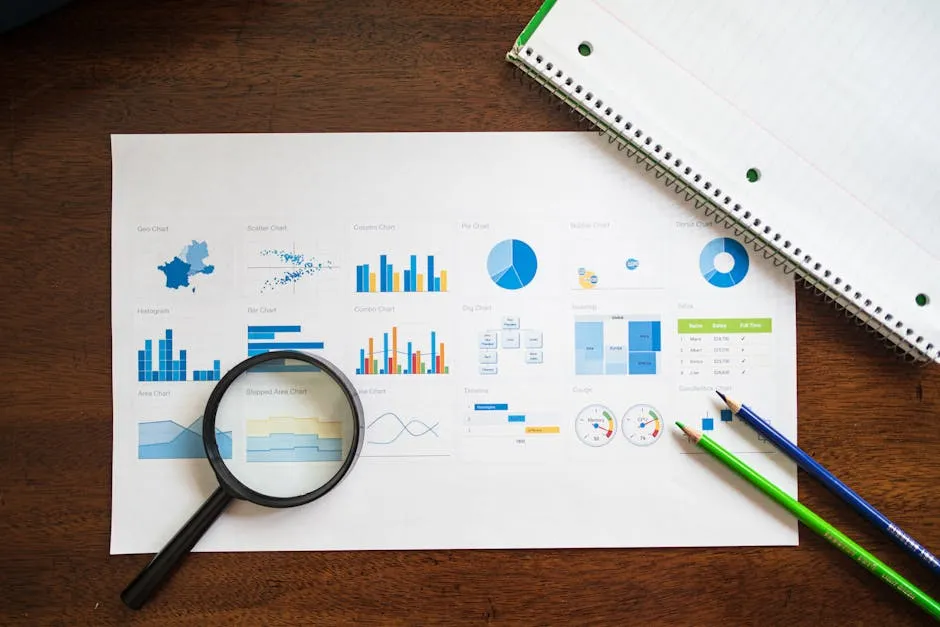
Techniques for Testing Assumptions
Assessing Normality
Normality is a cornerstone of parametric statistics. If your data isn’t normal, you might as well try to fit a square peg in a round hole. Fortunately, several methods can help you determine if your data is following the expected bell curve.
First up, histogram analysis! By creating a histogram, you can visualize the distribution of your data. If it resembles that classic bell shape, you’re likely in the clear. But if it looks more like a roller coaster, it’s time to rethink your strategy.
Next, let’s chat about Q-Q plots. This nifty tool compares your data’s quantiles against the quantiles of a normal distribution. If your points lie along the diagonal line, congratulations! Your data is behaving. If they scatter about like confetti, you might have a normality problem on your hands.
Finally, consider the Shapiro-Wilk test. This statistical test evaluates whether your sample comes from a normally distributed population. A significant result (p < 0.05) indicates departure from normality, while a non-significant result suggests you’re good to go. Just remember, a single test doesn’t tell the whole story—combine methods for a comprehensive assessment!
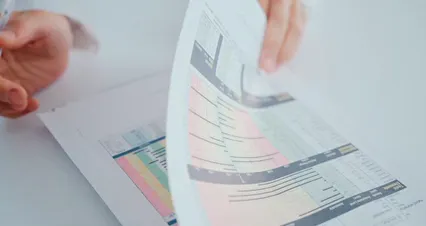
Evaluating Homogeneity of Variance
Homogeneity of variance is another key assumption for parametric tests. It states that the variances across different groups should be comparable. Think of it as ensuring that all your contestants are on equal footing before the big race. If one group is sprinting while another is crawling, your results could be skewed.
To assess this assumption, Levene’s test is your best friend. This test evaluates whether the variances are equal across groups. A non-significant result (p > 0.05) indicates that the variances are homogenous, allowing you to proceed with confidence.
If you find that your data violates this assumption, don’t despair! There are strategies to address it. One option is to use a robust statistical method, like Welch’s ANOVA. This test is less sensitive to unequal variances, giving you a valid alternative. Alternatively, consider transforming your data—sometimes a little log or square root can go a long way in stabilizing variances.
And if you’re looking for a solid resource on statistical quality control, consider “Statistical Quality Control: A Modern Introduction” by Douglas C. Montgomery. This book covers essential quality control techniques and is invaluable for researchers working in this field.

Importance of Independence
Independence is the unsung hero of statistical assumptions. It ensures that each observation in your dataset is independent of the others. If one participant’s response influences another’s, you risk violating this assumption. It’s like a game of dominoes—one fall can send the whole set tumbling.
To ensure independence, carefully design your study. Random sampling is crucial. Avoid scenarios where participants are paired or nested within groups unless you’re using the appropriate statistical methods. For example, in a crossover trial, consider using paired t-tests to account for the inherent dependencies.
If you’re dealing with nested data—like students within classrooms—multilevel modeling might be your ticket to independence nirvana. This technique accounts for the hierarchy in your data, ensuring that your analyses provide accurate insights. Keep independence at the forefront of your research design, and your results will thank you!
By rigorously testing these assumptions, researchers can confidently apply parametric methods, leading to credible and actionable insights in social sciences.

Conclusion
In the vast landscape of social sciences research, adhering to parametric statistics assumptions is not just a recommendation—it’s a necessity! Ignoring these guidelines is like trying to bake a soufflé without following the recipe. The result? A flat, unappetizing mess that leaves researchers questioning their methods and conclusions.
Understanding these assumptions—normality, independence, and homogeneity of variance—ensures that the statistical tests you apply yield valid and reliable results. Without this understanding, researchers risk drawing inaccurate conclusions that can misinform practices, policies, and theories. Imagine basing a critical social policy on flawed data! No one wants to be the researcher who unintentionally leads a charge down the wrong path.
Moreover, testing these assumptions rigorously before applying parametric methods is essential. This is akin to checking your parachute before jumping out of an airplane. You wouldn’t want to leap into data analysis without ensuring that your statistical methods are grounded in sound assumptions. So, take the time to assess your data. Use tests like the Shapiro-Wilk for normality and Levene’s test for homogeneity of variance. These steps will bolster the credibility of your research and enhance the quality of your findings.
Ultimately, the importance of following these guidelines cannot be overstated. A solid grasp of parametric statistics assumptions empowers researchers to confidently interpret their data and contribute meaningful insights to the social sciences. So, roll up your sleeves, test those assumptions, and let your research shine!

FAQs
What are the main assumptions of parametric statistics?
Parametric statistics rely on three key assumptions: normality, independence, and homogeneity of variance. Normality means that the data should follow a normal distribution. Independence ensures that observations are not related to one another. Finally, homogeneity of variance states that the variances across different groups should be roughly equal. Meeting these assumptions is crucial for valid statistical analysis.
Why is normality important in parametric tests?
Normality is vital because many parametric tests assume that the data follows a normal distribution. When this assumption holds true, the tests are more powerful, meaning they are better at detecting true effects. If the data is not normal, it can lead to incorrect conclusions, making it essential to assess this assumption before proceeding with analysis.
Can I use parametric tests with small sample sizes?
Using parametric tests with small sample sizes can be tricky. While the methods can still be applied, they become less reliable if the sample does not meet normality and homogeneity of variance assumptions. In such cases, researchers might want to consider nonparametric alternatives, which do not require these assumptions and are better suited for smaller datasets.
What should I do if my data does not meet parametric assumptions?
If your data fails to meet the assumptions necessary for parametric tests, don’t panic! You have alternatives. Consider using nonparametric tests like the Mann-Whitney U test or the Kruskal-Wallis test. These tests do not rely on the same assumptions and can provide valid insights from your data, ensuring that your research remains robust despite the challenges.
Please let us know what you think about our content by leaving a comment down below!
Thank you for reading till here 🙂
All images from Pexels