Introduction
In the vast universe of research and analysis, the distinction between statistics and numerical data is crucial. Understanding this difference can save you from making flawed conclusions. Did you know that misinterpreting statistics can lead to decisions that resemble a dog chasing its tail? Not productive, right?
Statistics and numerical data serve different purposes. Numerical data refers to raw facts, like numbers and measurements, while statistics involves interpreting this data to draw insights. This article aims to clarify how a statistic is different from numerical data, providing clear definitions and practical implications for various fields.
By the end of this piece, you’ll have a solid grasp of these concepts, ensuring you make informed decisions based on accurate interpretations. So, let’s jump into the numbers and uncover the magic behind data and statistics!

Understanding Data and Statistics
What is Data?
Data consists of raw facts and figures collected for analysis. Think of it as the ingredients in a recipe—useful, but not very tasty on its own. Data can take multiple forms, primarily categorized into qualitative and quantitative types. Qualitative data is descriptive, like colors or names, while quantitative data consists of numbers that can be measured.
For example, test scores in a classroom represent numerical data. They reveal how well students performed, but without context or analysis, they don’t tell the whole story.
Now, if you want to dive deeper into understanding data, consider picking up a copy of Statistics for Dummies. This book breaks down complex concepts into easy-to-understand terms, making it an excellent resource for beginners.
What are Statistics?
Definition: Statistics is the science of collecting, analyzing, interpreting, and presenting data. Imagine it as the detective work of numbers! Statistics helps turn jumbled facts into coherent narratives.
Role of Statistics: Statistics play a crucial role in making sense of data. They help us draw inferences and make predictions based on patterns we observe. Think of statistics as a trusty compass, guiding us through the dense forest of raw data. Without statistics, we’d be lost in a maze of numbers, unsure of our next step!
If you’re curious about how to measure the value of seemingly intangible things in business, you might find How to Measure Anything: Finding the Value of “Intangibles” in Business a fascinating read!
Key Differences Between Data and Statistics
The differences between data and statistics boil down to two main aspects: nature and purpose.
1. Nature: Data is raw and unprocessed—think of it as uncut diamonds. Statistics, on the other hand, is refined information, polished to reveal insights.
2. Purpose: Data serves as the input for statistical analysis. Statistics summarize and interpret that data. In a nutshell, while data provides the foundation, statistics build the structure of understanding.
In summary, recognizing how a statistic is different from numerical data is essential for making sense of the information. Whether you’re a student, a business analyst, or just a curious soul, mastering this difference can enhance your decision-making skills significantly.
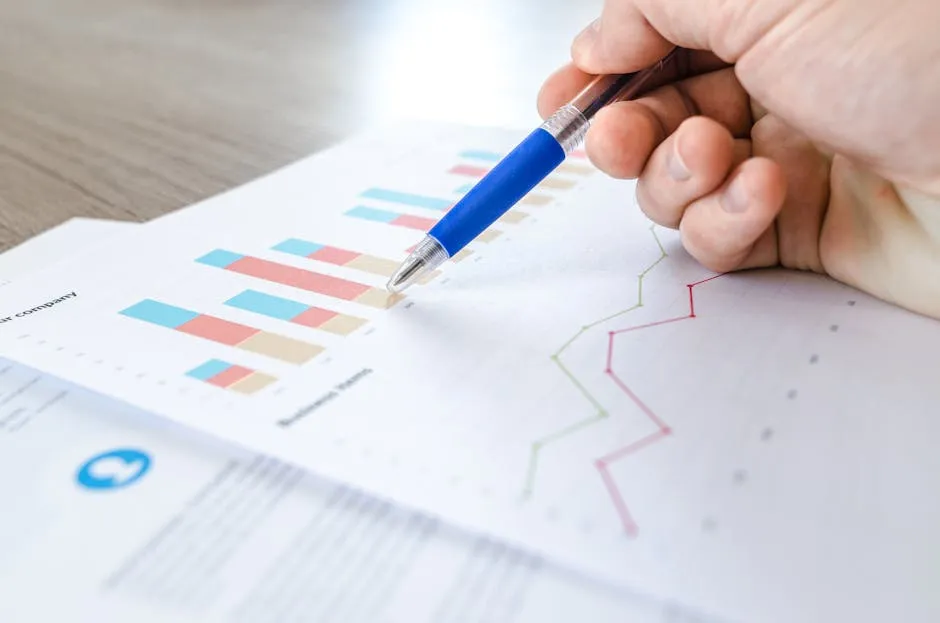
Importance of Numerical Data in Statistics
Role in Analysis
Numerical data is the backbone of statistical analysis. Without it, statistics would be like a ship without a sail—lost at sea! At its core, numerical data provides the raw information needed for calculations and comparisons. Think of it as the fuel that powers the analytical engine.
Statistical calculations help us make sense of the world. We use numerical data to find averages, variances, and trends. For example, businesses rely on sales figures to forecast future performance. In healthcare, patient data can reveal patterns in disease outbreaks. Thus, numerical data serves as the foundation for all statistical interpretations.
In short, numerical data is crucial. It allows statisticians to transform raw figures into insightful conclusions. This process helps organizations make informed decisions, ensuring they stay ahead of the competition. If you’re looking to improve your data analysis skills, a great resource is The Data Warehouse Toolkit: The Definitive Guide to Dimensional Modeling. This book provides invaluable insights into structuring your data effectively.
Examples of Numerical Data
- Finance: Stock prices, interest rates, and annual revenue figures.
- Healthcare: Patient weights, blood pressure readings, and cholesterol levels.
- Education: Student grades, attendance percentages, and test scores.
- Sports: Player statistics, game scores, and average points per game.
- Demographics: Population counts, age distributions, and income levels.
These examples illustrate how numerical data plays a pivotal role across multiple sectors. Each field uses this data differently, but the aim remains the same: to extract useful insights for better decision-making.
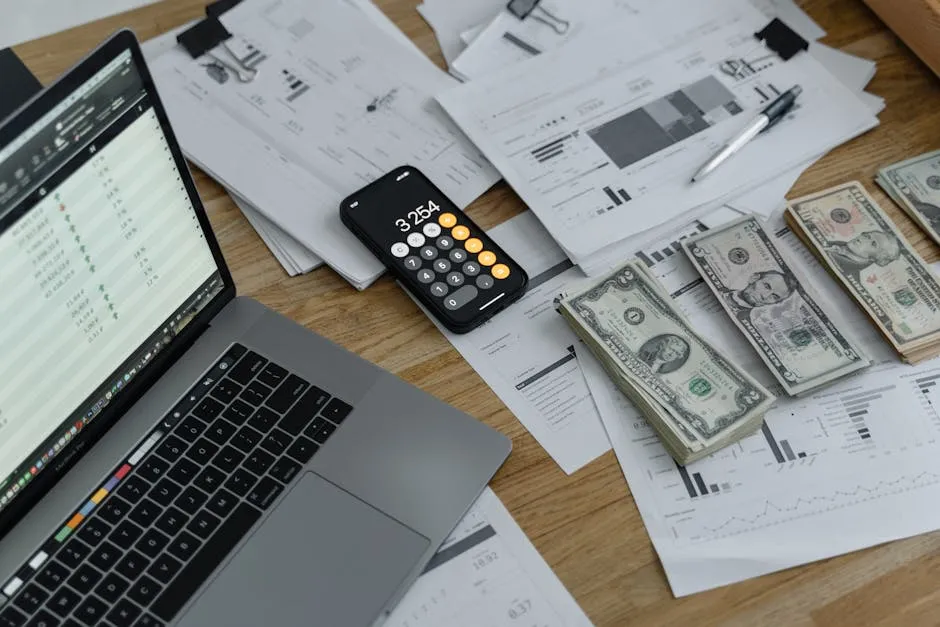
How Statistics Derive from Numerical Data
Processes Involved in Statistical Analysis
Collection
Collecting numerical data is the first step in statistical analysis. Various methods exist for gathering this data, including surveys, experiments, and observational studies.
For instance, surveys might ask people about their spending habits, gathering numerical responses. In a clinical trial, researchers measure patient outcomes, collecting vital data for analysis. Observational studies involve tracking behaviors or events over time, such as recording temperature fluctuations in a city.
Regardless of the method, accuracy is key. Poorly collected data can lead to misleading conclusions, like trying to navigate a maze with a blindfold.
Analysis
Once numerical data is collected, it’s time for the fun part: analysis! Analysts process this raw data to produce statistics, such as mean, median, and mode.
- Mean: The average value. Add up all numbers and divide by their count. This tells us the central tendency.
- Median: The middle value when sorted. It helps show where the center lies, especially when outliers skew the mean. Learn more about median statistics in Poland.
- Mode: The most frequently occurring number. This can reveal trends, such as the most common shoe size sold in a store. Explore statsmodels residuals statistics for deeper insights.
Beyond these measures, analysts employ various statistical techniques to interpret data. They create visualizations like graphs and charts, making the numbers come alive. This transformed data helps researchers draw conclusions and inform decisions.
If you’re interested in how to visualize data effectively, consider checking out The Visual Display of Quantitative Information. This book is a treasure trove of information on presenting data visually and making it understandable.
In summary, the journey from numerical data to statistics is like turning raw ingredients into a delicious dish. Each step requires care, precision, and a sprinkle of creativity!

Interpretation of Statistics
Descriptive Statistics
Descriptive statistics summarize and describe the essential features of a dataset. They provide a clear snapshot of the data at hand. Common measures include the mean, median, and mode.
For example, consider a class of students’ test scores: 85, 90, 78, 92, and 88. The mean score is 86.6, providing a quick overview of performance. The median, 88, indicates the middle score when arranged in order. Meanwhile, the mode is 85, showing the most frequently occurring score. These statistics help us comprehend the overall performance without diving into every single score.
Inferential Statistics
Inferential statistics take a step further. They use a sample of data to make predictions or generalizations about a larger population. This branch of statistics is crucial when it’s impractical to collect data from every member of a population. Understand the problems associated with inferential statistics.
For instance, if a researcher wants to know the average height of adult males in a city, measuring every single adult isn’t feasible. Instead, they might survey a random sample of 100 men. By analyzing this sample, they can infer the average height for the entire population. This approach not only saves time but also provides valuable insights based on statistical principles.
If you’re eager to dive deeper into statistics, consider picking up Naked Statistics: Stripping the Dread from the Data. This book demystifies statistics and makes learning enjoyable!
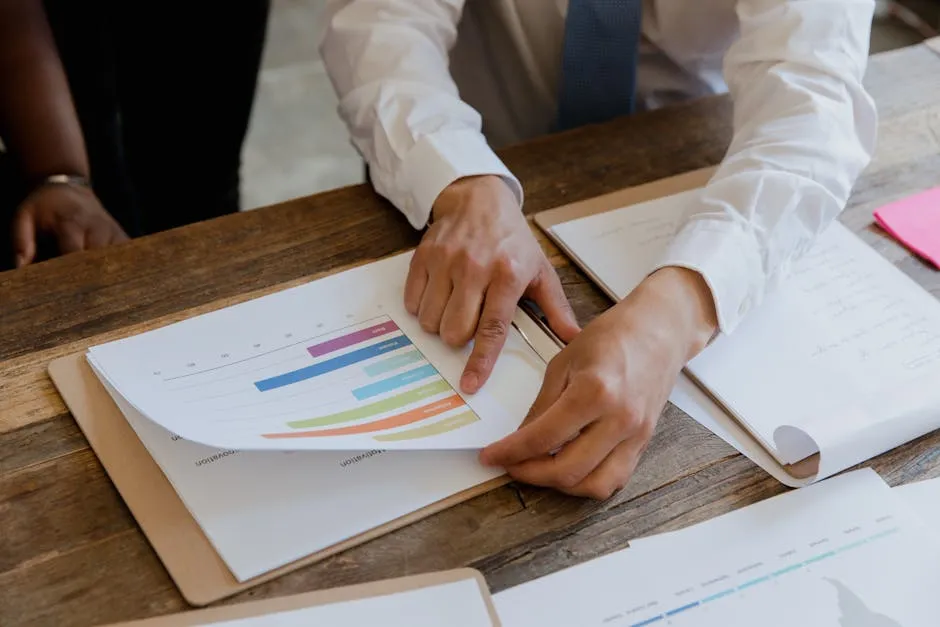
Practical Implications of Understanding the Difference
Importance in Research
Understanding the difference between data and statistics is critical for researchers. Misinterpreting these concepts can lead to flawed conclusions. Data alone, while informative, lacks context. Statisticians transform data into statistics to interpret and summarize it effectively.
For example, if a researcher reports that “50% of participants liked the new product,” this statistic is meaningless without knowing the sample size and demographics. Researchers must differentiate between raw data and the insights statistics provide. Clear interpretations guide sound decision-making.
In research, clarity is key. Researchers should be equipped to distinguish between the two to avoid misrepresentation and ensure accurate conclusions. This understanding fosters informed discussions and better policy-making.
Moreover, in fields like healthcare, economics, and social sciences, the implications can be vast. For instance, policymakers rely on statistics to allocate resources effectively. Misinterpretations could lead to poor funding choices, impacting community welfare.
Thus, differentiating between numerical data and statistics is not just an academic exercise; it’s essential for real-world applications. Researchers, analysts, and decision-makers must grasp this distinction to navigate the complexities of data-driven environments successfully. With this knowledge, they can ensure their analyses are robust and conclusions well-founded.
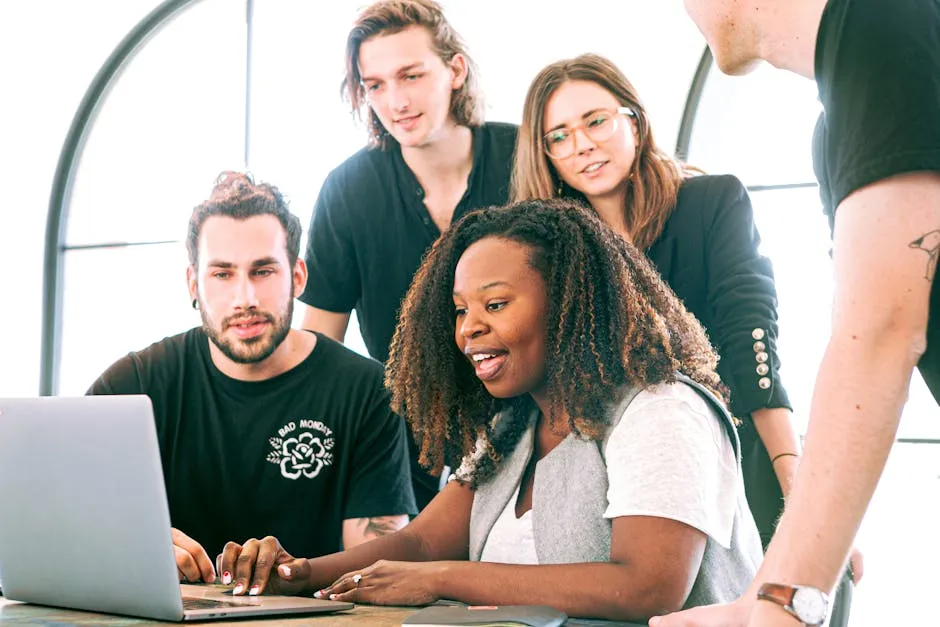
Real-World Applications
Understanding the difference between statistics and numerical data is crucial across various fields. Let’s break down a few key areas where this distinction plays a vital role.
In healthcare, statistics help analyze patient data to improve treatments and outcomes. For instance, hospitals might track recovery times using numerical data. By applying statistical methods, they can identify trends that lead to better patient care. Imagine a hospital trying to figure out if a new medication actually works—statistics from numerical data can provide the answer.
Moving to economics, this distinction helps economists make sense of market trends. They gather numerical data on employment figures, inflation rates, and consumer spending. Through statistical analysis, they can interpret this data to predict economic shifts. Without statistics, economic forecasts would be as reliable as a fortune cookie!
In social sciences, researchers rely heavily on the difference between these two concepts. They collect numerical data through surveys, observations, or experiments. Then, they apply statistical techniques to draw conclusions about societal behaviors and attitudes. This process helps policymakers make informed decisions. Think of a politician wanting to understand voter preferences—statistics from numerical data can guide campaign strategies. Learn more about applied statistics for social science research.
If you’re interested in honing your skills in data science, you might want to check out Data Science for Business: What You Need to Know about Data Mining and Data-Analytic Thinking. It provides a comprehensive overview of how to apply data science concepts in business contexts.
In summary, recognizing the difference between statistics and numerical data is essential for effective decision-making in healthcare, economics, and social sciences.
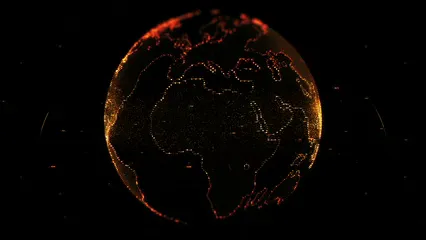
Consequences of Misinterpretation
Misinterpreting statistics can lead to serious consequences. When statistics derived from numerical data are misunderstood, the fallout can affect individual lives and entire communities.
Consider a healthcare scenario where a hospital misreads patient recovery statistics. If they conclude that a treatment is effective based on skewed data, patients might receive ineffective care. This misinterpretation could lead to longer recovery times and increased healthcare costs.
In economics, inaccurate interpretations can lead to misguided policies. If a government misinterprets unemployment statistics, it might implement policies that do more harm than good. For instance, increasing taxes during a recession could exacerbate economic woes.
In the realm of social sciences, misinterpretations can skew public perception. If a study on social behavior is reported incorrectly, it may lead to stereotypes or stigmas. This could adversely impact communities, reinforcing negative biases.
Ultimately, misinterpreting statistics can result in poor decision-making and unintended consequences. It’s vital to understand the distinction between statistics and numerical data to avoid these pitfalls and ensure informed choices.
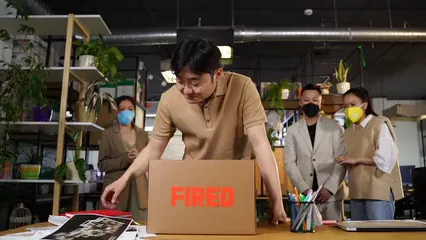
Conclusion
In wrapping up, it’s clear that understanding how a statistic is different from numerical data is essential in various domains. Statistics are the interpretations and insights derived from raw numerical data. They are processed, analyzed, and presented in ways that reveal patterns, trends, and relationships.
To recap, numerical data is the foundation—the raw facts and figures collected through various methods. Statistics take this data and provide meaning, allowing for informed decision-making. This distinction is not merely academic; it has practical implications in healthcare, economics, and social sciences.
If you’re eager to learn more about data science, consider picking up The Data Science Handbook: A Comprehensive Guide to Data Science. This resource is perfect for anyone looking to deepen their understanding of data science principles.
Understanding these concepts is crucial in everyday life and professional practice. In a world overflowing with data, being able to sift through and interpret it accurately is a superpower. It empowers individuals and organizations to make better decisions, ultimately leading to improved outcomes and well-informed policies.
In our data-driven society, knowledge is indeed power. So, the next time you encounter statistics, remember: it’s not just numbers; it’s insight waiting to be understood!
FAQs
What is the difference between qualitative and quantitative data?
Qualitative data describes characteristics and qualities, like colors or opinions. For example, responses to a survey can indicate preferences. Quantitative data, on the other hand, consists of numbers that can be measured. An example would be the number of students in a classroom or their test scores, which can be analyzed mathematically.
Can statistics be misleading?
Yes, statistics can be misleading if presented without context. For instance, cherry-picking data points can skew results, leading to false conclusions. It’s crucial to understand the underlying data and the methods used to analyze it to avoid misinterpretation.
How can I improve my understanding of statistics?
To enhance your understanding, consider taking online courses or reading books on statistics. Websites like Khan Academy and Coursera offer valuable resources. Engaging with practical examples and exercises can also solidify your grasp of statistical concepts.
Why is it important to differentiate between data and statistics?
Differentiating between data and statistics is vital for accurate research and decision-making. It ensures that interpretations are based on sound analysis rather than raw figures. This understanding is essential in avoiding misinterpretations that could lead to poor decisions.
What are some common statistical methods used in analyzing numerical data?
Common statistical methods include regression analysis, which examines relationships between variables, and hypothesis testing, used to determine if observed data differences are significant. Other methods include ANOVA (analysis of variance) and chi-square tests, which help in comparing groups and categorical data. Each method serves a unique purpose in interpreting numerical data effectively.
Please let us know what you think about our content by leaving a comment down below!
Thank you for reading till here 🙂
All images from Pexels