Introduction
Statistics play a pivotal role in the manufacturing landscape today. They are not just numbers; they are the backbone of informed decision-making. In an era filled with data, manufacturers harness statistical tools to streamline operations and enhance productivity. From predicting equipment failures to optimizing production processes, statistics are essential.
Modern manufacturing environments thrive on data-driven decisions. Gone are the days when intuition alone guided choices. Companies now rely on concrete data to minimize risks and maximize efficiency. The importance of statistical analysis cannot be overstated. It allows manufacturers to identify trends, forecast demands, and maintain quality control.
This article will explore the basics of statistics in manufacturing, emphasizing their significance in various aspects of production. We’ll discuss the fundamentals of statistics, the role of data collection methods, and their impact on operational efficiency. By the end, you’ll appreciate how statistics can transform manufacturing processes, paving the way for continuous improvement.

Understanding the Basics of Statistics in Manufacturing
What Are Statistics?
Statistics is the science of collecting, analyzing, and interpreting data. It provides tools to summarize complex information and draw meaningful conclusions. In manufacturing, statistics help in quality control, process optimization, and decision-making.
Two key branches of statistics are descriptive and inferential statistics. Descriptive statistics summarize and present data clearly, allowing manufacturers to visualize trends and patterns. Inferential statistics enable manufacturers to make predictions and generalizations about a larger population based on sample data. This distinction is crucial for making informed operational choices.
If you’re eager to dive deeper into the world of statistics, consider picking up R Programming Language Book. It’s a fantastic resource for anyone looking to harness the power of statistics in their analysis!
The Role of Data in Manufacturing
Data is the lifeblood of modern manufacturing. It is gathered from various sources, including sensors, IoT devices, and machinery. This data collection process is essential for monitoring and improving operational efficiency. Real-time data allows manufacturers to make immediate adjustments, enhancing overall productivity.
Collecting data isn’t just about numbers; it’s about understanding what those numbers mean. For instance, analyzing machine performance data can reveal inefficiencies or potential failures. This proactive approach leads to timely interventions, minimizing downtime and saving costs.
Incorporating real-time data into manufacturing processes empowers companies to respond swiftly to changes. With accurate data collection methods, manufacturers can track production metrics, optimize inventory levels, and maintain quality standards. The ability to harness data effectively is what sets successful manufacturing plants apart in today’s competitive landscape.
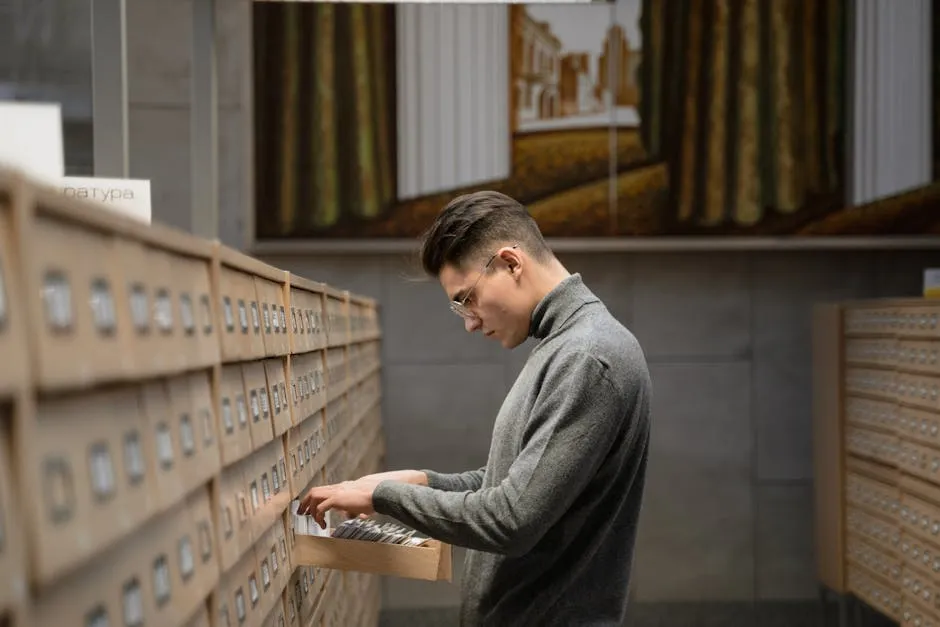
Key Applications of Statistics in Manufacturing
1. Quality Control and Improvement
Statistical Process Control (SPC)
Statistical Process Control (SPC) is a method that uses statistical techniques to monitor and control manufacturing processes. The main goal is to ensure that the process operates at its full potential. When the process is stable, it produces products that meet specifications consistently, minimizing waste and maximizing efficiency.
Control charts are among the key tools used in SPC. These charts visually display process data over time, indicating variations and trends. They help identify when a process is going out of control, allowing manufacturers to take corrective action before defects occur. For instance, a car manufacturer might use a control chart to track the dimensions of parts produced. If the measurements start to drift outside the control limits, engineers can investigate the cause before producing faulty components.
Process capability analysis is another essential tool in SPC. It assesses how well a process can produce output within specified limits. By calculating capability indices, manufacturers can quantify process performance. This metric helps determine if the existing process meets quality standards or if adjustments are necessary. For example, a food processing plant could calculate its process capability to ensure that its production consistently meets safety and quality standards.
Real-world examples of SPC in action illustrate its effectiveness. A global electronics manufacturer implemented SPC to monitor defect rates on an assembly line. By utilizing control charts, the company quickly identified a rise in defects related to a specific machine. After investigation, they discovered that a worn-out part was causing the issue. Replacing the part led to a significant reduction in defects, saving the company both time and money.
In another case, a textile manufacturer used process capability analysis to evaluate the dyeing process. They found that their process capability index was below industry standards, indicating a need for improvement. By adjusting the dyeing parameters and training staff on best practices, they increased their process capability, resulting in higher quality products and fewer customer complaints.
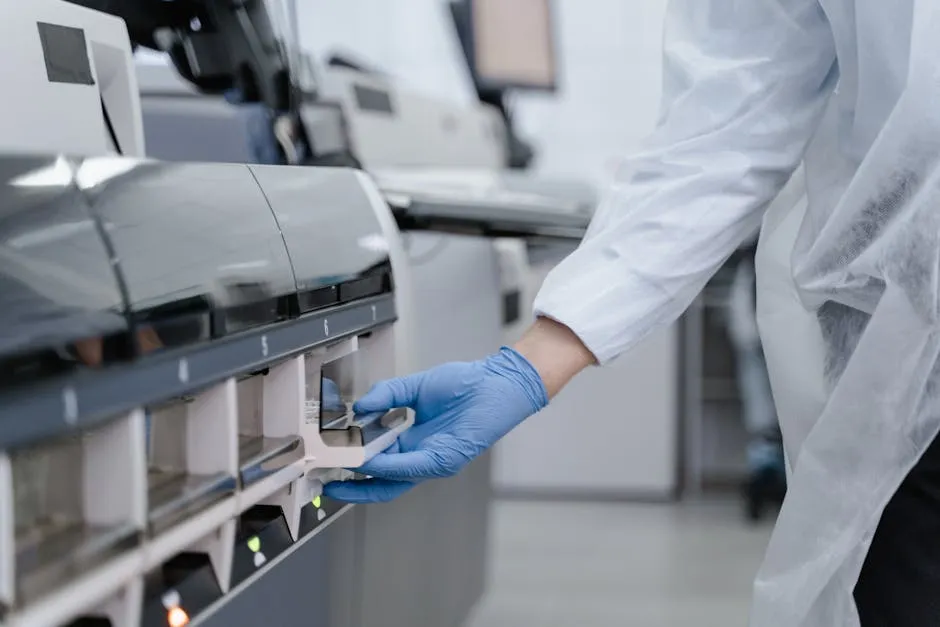
For those looking to enhance their understanding of quality control, grab a copy of Statistical Process Control: A Practical Guide to Improving Your Manufacturing Process. It’s an essential read for anyone serious about enhancing their operational quality!
2. Production Optimization
Designing Experiments (DOE)
Designing Experiments (DOE) is a powerful statistical tool used in manufacturing to test and optimize processes. DOE allows manufacturers to systematically evaluate how various factors influence specific outcomes. By carefully structuring experiments, organizations can gain significant insights that lead to improved efficiency and product quality.
The significance of DOE lies in its ability to explore multiple variables simultaneously. Unlike traditional experimentation, which may change one factor at a time, DOE examines interactions between factors. This approach can reveal how different variables affect the process and lead to more informed decisions. For example, a beverage manufacturer could use DOE to study how temperature, pressure, and ingredient proportions impact the taste and quality of a new drink.
Case studies illustrate successful implementations of DOE in manufacturing. A pharmaceutical company faced challenges in optimizing the formulation of a new medication. By employing DOE, they analyzed how different excipients and mixing times affected the tablet’s dissolution rate. The results led to an optimized formulation that improved the drug’s effectiveness while minimizing production costs.
In another instance, an automotive parts manufacturer sought to enhance the durability of its components. Using DOE, they tested various materials and production methods to determine the optimal combination for strength and weight. The insights gained from these experiments allowed them to produce lighter yet stronger parts, enhancing vehicle performance and fuel efficiency.

To further explore the world of production optimization, consider Lean Six Sigma for Manufacturing: A Practical Guide. It’s packed with strategies to optimize your operations!
3. Demand Forecasting
Accurate demand forecasting is crucial in manufacturing as it directly impacts production planning, inventory management, and overall operational efficiency. Understanding future demand allows manufacturers to align their production schedules with market needs, reducing excess inventory and minimizing stockouts.
Statistical methods, such as regression analysis, are widely used in demand forecasting. By analyzing historical sales data and identifying trends, manufacturers can make informed predictions about future demand. For instance, a consumer electronics manufacturer might use regression analysis to assess how seasonal trends and marketing campaigns influence sales of its latest gadgets.
Companies that invest in better forecasting methods often see significant benefits. A clothing retailer, for example, implemented advanced forecasting techniques to predict demand for its seasonal collections. By analyzing past sales data and considering factors like weather patterns and fashion trends, they optimized their inventory levels. As a result, they reduced markdowns on unsold items and increased overall profitability.
Another notable example is a food manufacturer that utilized statistical forecasting to predict demand for perishable products. By incorporating factors such as shelf life and consumer purchasing patterns, they managed to align production with actual market demand, minimizing waste and maximizing freshness.
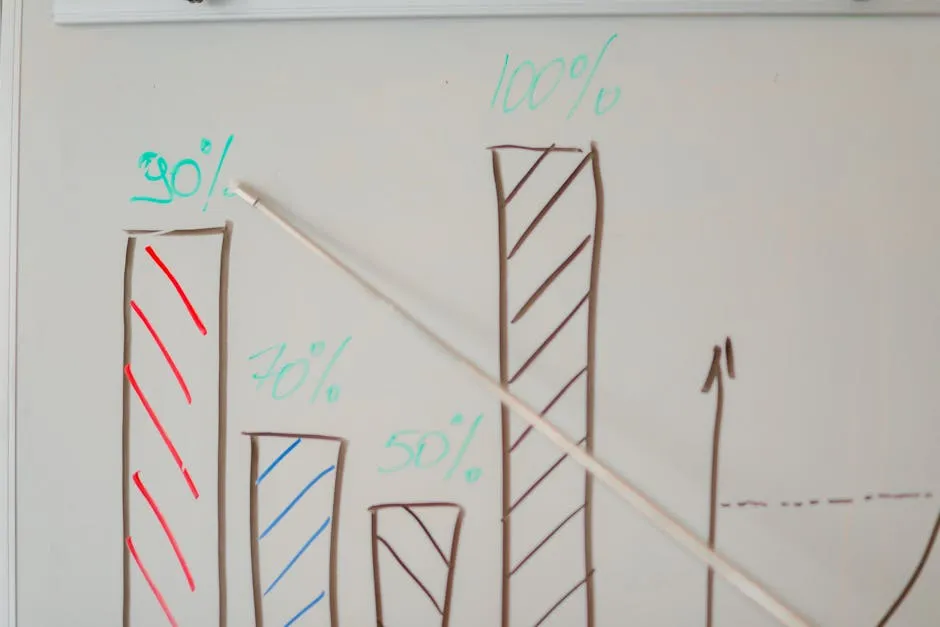
For further insights into demand forecasting, consider checking out Business Statistics: A First Course. It’s a fantastic resource for honing your analytical skills!
In summary, statistics play a vital role in manufacturing by enhancing quality control, optimizing production processes, and improving demand forecasting. By leveraging statistical tools and techniques, manufacturers can make data-driven decisions that lead to greater efficiency, reduced costs, and improved product quality. As data continues to grow in importance, embracing statistical methods will be essential for staying competitive in the manufacturing landscape.

4. Inventory Management
Statistics play a crucial role in inventory management within manufacturing plants. They help companies maintain optimal stock levels, minimize waste, and respond effectively to demand fluctuations. The application of statistical analysis allows for smarter decision-making regarding inventory purchases, storage, and utilization.
One primary technique in managing inventory is the use of statistical models for demand forecasting. By analyzing historical sales data, manufacturers can identify patterns and trends that inform future demand. For example, a manufacturer of home appliances might observe that sales peak during holiday seasons. This insight prompts them to increase stock levels in anticipation of higher demand, ensuring they meet customer needs without overproducing.
Another effective technique is the Economic Order Quantity (EOQ) model. This statistical formula determines the optimal order quantity that minimizes total inventory costs, including holding and ordering expenses. By employing the EOQ model, a company can strategically time its purchases, ensuring that they are neither overstocked nor understocked. This balance significantly reduces waste and storage costs, leading to improved profitability.
Real-time data analytics also enhance inventory management. By integrating IoT sensors and software, manufacturers can collect and analyze live data on stock levels, production rates, and sales patterns. This capability allows for swift adjustments to inventory levels based on actual usage and demand. For instance, a food manufacturer can track the freshness of ingredients and adjust orders accordingly, reducing spoilage and waste.
Case studies highlight the effectiveness of statistical analysis in inventory management. A leading electronics manufacturer implemented an advanced forecasting system that utilized regression analysis to predict product demand accurately. As a result, they reduced excess inventory by 30% and improved cash flow through better stock turnover rates.

Another example involves a textile company that utilized statistical process control (SPC) methods to monitor fabric production. By analyzing production data, they identified the optimal time to reorder raw materials, ensuring that they never faced shortages that could halt production. This proactive approach improved operational efficiency and reduced costs associated with expedited shipping.
For those looking to sharpen their inventory management skills, consider reading Inventory Management: Principles, Concepts and Techniques. It’s a must-read for anyone involved in supply chain management!
In summary, the role of statistics in inventory management is vital for modern manufacturing plants. By employing techniques such as demand forecasting, EOQ models, and real-time data analytics, companies can optimize their inventory levels, reduce waste, and enhance overall operational efficiency. The integration of these statistical methods not only supports better decision-making but also contributes to a healthier bottom line.

5. Predictive Maintenance
Predictive maintenance is a proactive approach that uses statistical methods to anticipate equipment failures before they occur. This strategy has gained traction in manufacturing, as it allows companies to reduce downtime, save on repair costs, and extend the lifespan of their machinery.
The concept of predictive maintenance revolves around collecting and analyzing data from various sources, such as sensors embedded within equipment. By monitoring variables like temperature, vibration, and operating hours, manufacturers can build statistical models that predict when a machine is likely to fail. This approach enables timely interventions, preventing costly breakdowns.
One of the main advantages of predictive maintenance is its cost-effectiveness. By addressing potential issues before they lead to equipment failure, manufacturers can avoid the high costs associated with unplanned downtime. For instance, a packaging company that utilizes predictive maintenance can schedule repairs during off-peak hours, minimizing the impact on production schedules.
Data analysis techniques, including regression analysis and machine learning algorithms, are integral to successful predictive maintenance strategies. These methods allow manufacturers to identify patterns in equipment performance and pinpoint the factors that contribute to failures. By leveraging these insights, companies can develop maintenance schedules that align with actual equipment needs rather than relying on arbitrary timelines.
Real-life examples underscore the effectiveness of predictive maintenance. A global automotive manufacturer implemented a predictive maintenance program that monitored engine assembly robots. By collecting data on their operational parameters, the company identified early signs of wear and tear. As a result, they reduced maintenance costs by 25% and improved overall equipment effectiveness.
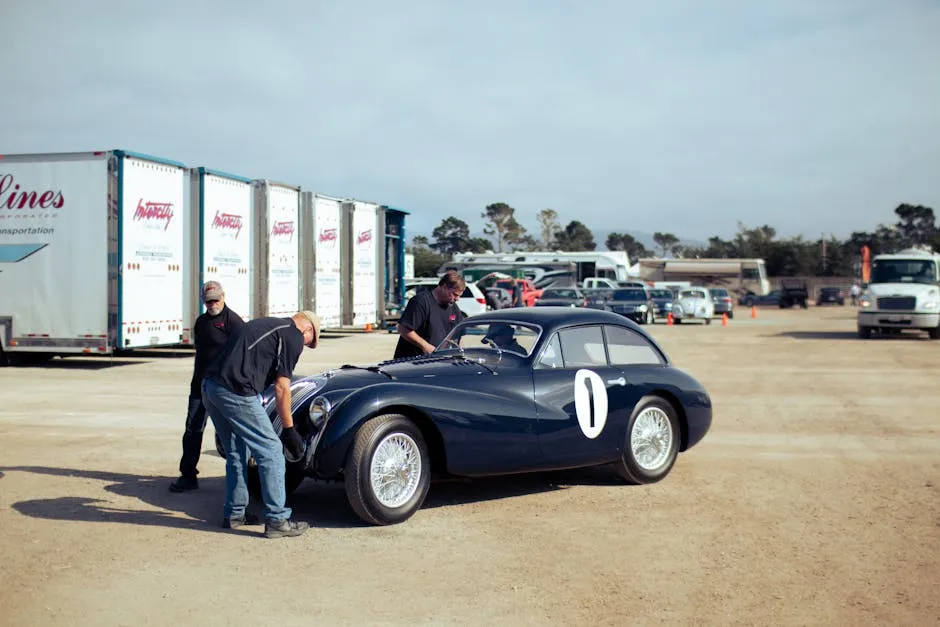
In another instance, a food processing plant used predictive analytics to monitor refrigeration units essential for product preservation. By analyzing operational data, they detected anomalies that indicated potential refrigerant leaks. Addressing these issues promptly not only safeguarded product quality but also complied with environmental regulations.
For those interested in a comprehensive look at predictive maintenance, check out Predictive Maintenance: A Practical Approach to Reducing Costs. It’s a valuable resource for enhancing your maintenance strategy!
In conclusion, predictive maintenance represents a significant advancement in manufacturing practices. By applying statistical analysis to equipment data, manufacturers can anticipate failures, optimize maintenance schedules, and ultimately enhance operational efficiency. This data-driven approach not only saves costs but also ensures that production processes run smoothly, contributing to overall business success.
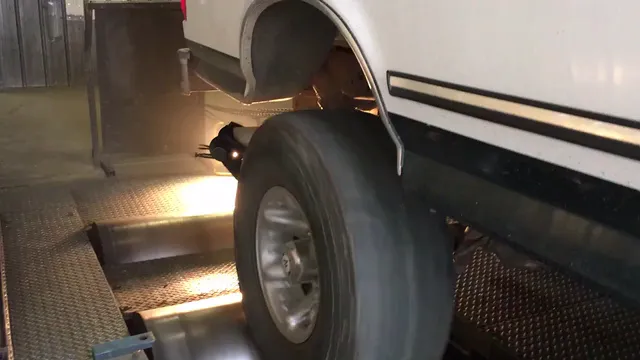
Cost Reduction
Statistical analysis is a powerful ally in the quest for cost reduction in manufacturing. Manufacturers often find themselves in a tug-of-war between maintaining quality and minimizing costs. With the right statistical methods, they can achieve both.
Take, for instance, a beverage company that analyzed production data and discovered excessive waste during bottling. By applying statistical techniques to identify the root causes, they fine-tuned their machinery settings. This simple tweak reduced waste by 20%, resulting in substantial cost savings without compromising on quality.
Moreover, statistical analysis can spotlight inefficiencies in production workflows. A textile manufacturer, for example, used data analysis to optimize fabric cutting patterns. By maximizing material usage, they cut costs on raw materials significantly. Every little bit counts, right?
Waste reduction goes hand in hand with efficiency improvements. Statistics help manufacturers gather insights into production cycles, revealing bottlenecks and redundancies. By analyzing cycle times, companies can streamline operations, cutting unnecessary steps and saving valuable time and resources.

To explore more about cost reduction strategies, consider reading Quality Control for Dummies. It’s a great introduction to the world of quality and cost management!
Improved Product Quality
When it comes to product quality, statistics are the unsung heroes. By employing statistical methods, manufacturers can maintain and even elevate their quality standards.
One common approach is Statistical Process Control (SPC). This technique uses control charts to monitor production processes in real time. For example, a car manufacturer might track measurements like the thickness of paint on vehicles. If the thickness starts to deviate from acceptable levels, the data triggers an alert. This proactive approach helps catch issues before they escalate, ensuring high-quality products.
Case studies abound in this realm. A renowned electronics company adopted SPC and, within months, reported a 30% reduction in defects. By analyzing data on their assembly line processes, they pinpointed specific machines that were underperforming. After adjustments, their products not only met quality standards but frequently exceeded customer expectations.
Another stellar example comes from a pharmaceutical company that used statistical analysis to refine their drug formulation process. By experimenting with various ingredients and concentrations through Design of Experiments (DOE), they found an optimal mix that improved efficacy while reducing production costs. The result? A better product and happier patients.

For a deeper dive into improving product quality, check out The Art of Statistics: Learning from Data. It’s a fantastic guide for anyone looking to enhance their analytical skills!
Risk Management
In the unpredictable world of manufacturing, risk management is essential. Statistics play a critical role in identifying and mitigating potential risks. By analyzing historical data, manufacturers can forecast failures and address vulnerabilities before they wreak havoc.
Take predictive maintenance, for instance. Statistical models analyze equipment performance data, helping manufacturers anticipate when machines are likely to fail. A food processing plant, for example, employed sensors to monitor machinery health. When the data indicated abnormal vibrations, maintenance was scheduled proactively, preventing costly downtime and preserving product quality.
Risk assessment techniques, such as Failure Mode and Effects Analysis (FMEA), utilize statistical principles to evaluate potential failure points. By systematically analyzing processes, teams can prioritize risks and implement strategies to mitigate them. This approach not only safeguards production but also enhances overall operational resilience.
Moreover, manufacturers can utilize statistical methods to understand market risks. By analyzing sales and market trends, companies can adjust their strategies to navigate economic fluctuations. For instance, a consumer goods manufacturer that noticed declining sales in a specific segment used statistical forecasting to pivot its production and marketing efforts accordingly. As a result, they minimized losses and maintained profitability.
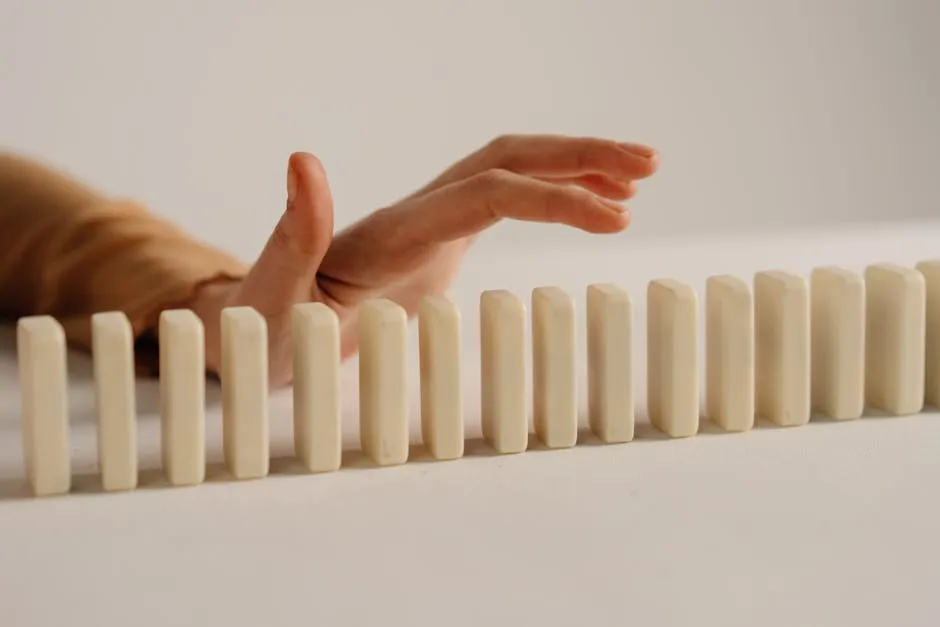
For further insights into risk management strategies, consider reading The Essentials of Business Research Methods. It’s a great resource for understanding how to manage risks effectively!
In summary, incorporating statistical analysis into manufacturing can significantly bolster cost reduction, improve product quality, and strengthen risk management strategies. Embracing these data-driven approaches empowers companies to thrive in a competitive landscape while delivering the best products to their customers.
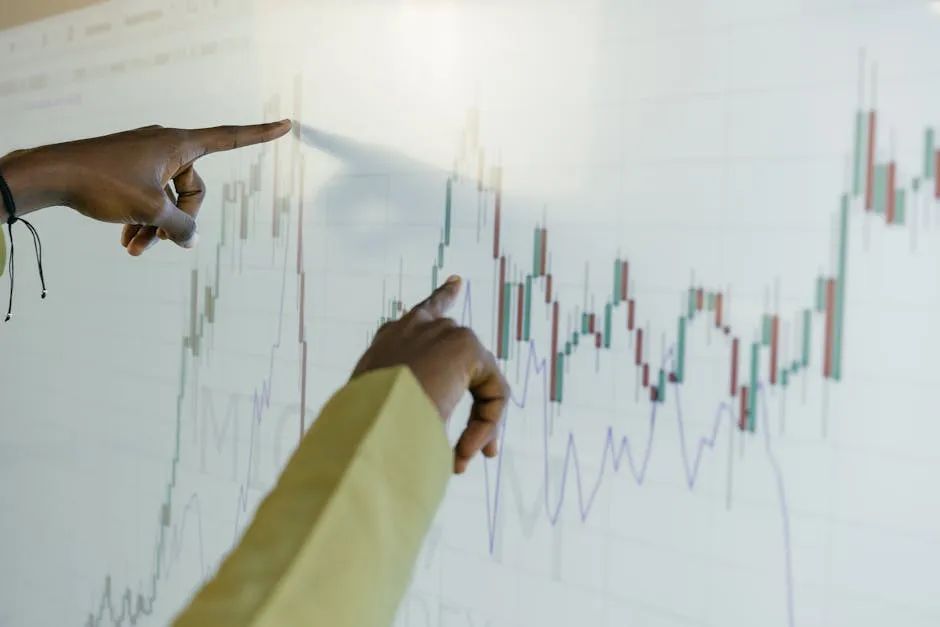
Training and Skill Development
Training employees in statistical methods is essential. It empowers them to make data-driven decisions and enhances overall plant performance. Knowledge of statistics helps staff understand process variations and quality control measures. This understanding translates to improved efficiency and reduced waste, ultimately benefiting the bottom line.
Investing in training programs is a smart move for manufacturers. Workshops, online courses, and hands-on training sessions can equip employees with the necessary tools. For instance, programs focusing on statistical process control (SPC) can provide insights into monitoring production processes effectively.
Additionally, resources like statistical software training can further enhance skill sets. Many organizations offer certification programs that teach employees how to utilize statistical software for data analysis. This knowledge enables operators to interpret data accurately and make informed adjustments to their operations.
If you’re looking for a comprehensive guide to training in statistics, consider The Data Warehouse Toolkit: The Definitive Guide to Dimensional Modeling. It’s a fantastic resource for understanding data management!
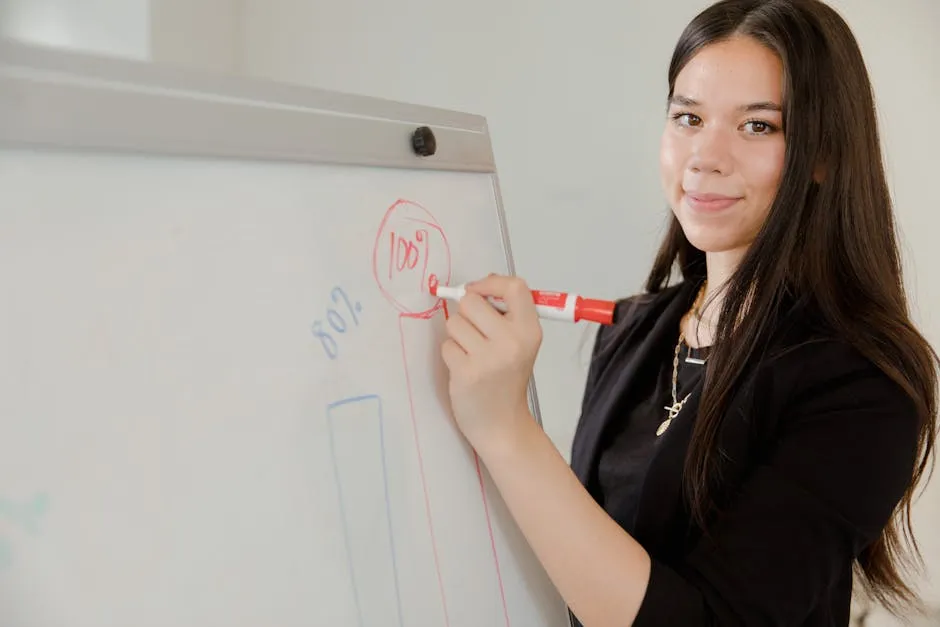
Leveraging Technology
Technology plays a pivotal role in statistical analysis within manufacturing. Modern software packages streamline the process, making it easier to collect and analyze data. Tools like Minitab Statistical Software and R provide powerful functionalities for statistical computations. These applications help manufacturers visualize trends, detect anomalies, and optimize production processes.
As we embrace Industry 4.0, the integration of IoT devices becomes increasingly vital. Sensors on machines collect real-time data, which feeds into statistical software for analysis. This synergy allows manufacturers to monitor equipment performance, track quality metrics, and predict potential failures. By leveraging technology, plants can enhance their statistical practices and achieve greater operational efficiency.
Moreover, the impact of Industry 4.0 on statistical methods is profound. With advanced analytics and machine learning, manufacturers can uncover hidden patterns in their data. These insights lead to proactive decision-making, allowing businesses to stay ahead of the competition. Integrating technology into statistical practices not only simplifies analysis but also empowers manufacturers to innovate continuously.
Conclusion
Statistics is not just about numbers; it’s about making informed decisions that enhance manufacturing efficiency and quality. By understanding and applying statistical methods, manufacturers can significantly improve their operations.
Training employees in statistical analysis is pivotal. It ensures that the workforce is equipped to handle data effectively. Investing in skill development programs and leveraging technology can lead to substantial benefits. Manufacturers must embrace digitization and explore various statistical tools to stay competitive.
Incorporating descriptive statistics in manufacturing plant practices allows companies to glean insights from their data. This proactive approach to data management leads to better decision-making and continuous improvement. As the manufacturing landscape evolves, adopting statistical methods becomes even more crucial for success.
Encouraging a culture of data-driven decision-making can set manufacturers apart. By prioritizing statistics, they can enhance product quality, streamline processes, and reduce costs. It’s clear that the future of manufacturing lies in the hands of those who harness the power of statistics.
FAQs
What is the importance of statistics in manufacturing?
Statistics play a crucial role in manufacturing by enabling data-driven decision-making. They help in quality control, process improvement, and operational efficiency. Accurate data analysis leads to better predictions and reduced waste.
How can statistical analysis improve product quality?
Statistical analysis can identify quality trends and variations in production. By using tools like Statistical Process Control (SPC), manufacturers can monitor processes in real-time, ensuring consistent product quality and minimizing defects.
What are some common statistical tools used in manufacturing?
Common statistical tools include control charts, regression analysis, hypothesis testing, and Design of Experiments (DOE). These tools help analyze data and optimize production processes.
How does predictive maintenance work?
Predictive maintenance uses statistical methods to analyze equipment data. By monitoring variables such as vibration and temperature, manufacturers can predict when equipment is likely to fail, allowing for timely maintenance and reduced downtime.
Can small manufacturers benefit from statistical methods?
Absolutely! Small manufacturers can leverage statistical methods to improve efficiency, reduce costs, and enhance product quality. Even basic statistical tools can provide valuable insights for operational improvements.
Please let us know what you think about our content by leaving a comment down below!
Thank you for reading till here 🙂
All images from Pexels