Introduction
In the cute little world of statistics, beta (β) is a critical player. It’s the sneaky probability of making a Type II error during hypothesis testing. You know, the kind of error where you fail to reject a false null hypothesis. Imagine rejecting a dinner invitation, only to find out your friends were serving your favorite lasagna. Ouch!
Let’s break it down. We have two types of errors in hypothesis testing. The first is the Type I error, represented by alpha (α), where you mistakenly reject a true null hypothesis. Think of it like declaring a movie terrible only to find everyone else adored it. The second is the Type II error (β), where you miss rejecting a false null hypothesis. Here’s the kicker: the probability of a Type II error is what we call beta!
Calculating beta is essential in research and data analysis. It helps researchers determine the power of their tests. A higher beta means a greater likelihood of missing a significant effect. But don’t worry; we’ll tackle that confusion together. This article will guide you through understanding beta statistics, how to calculate them, and why they matter.
Let’s dive into the nitty-gritty of beta statistics, exploring definitions, roles, and the significance of these calculations. Get your calculator ready! And speaking of calculators, if you need a reliable one, check out the Texas Instruments TI-84 Plus Graphing Calculator. It’s perfect for your statistical adventures!
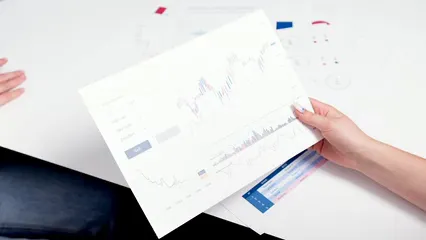
Understanding Beta Statistics
Definition of Beta (β)
Beta (β) is the probability of making a Type II error. It shows the chances of failing to reject a null hypothesis when the alternative hypothesis is true. If your test says everything is fine when it isn’t, that’s a Type II error in action. Ah, the drama of statistics!
Now, what’s the difference between beta (β) and alpha (α)? While alpha represents the probability of a Type I error, beta represents the probability of a Type II error. They’re like the yin and yang of hypothesis testing—opposing forces that keep researchers on their toes!
The Role of Beta in Statistical Tests
Beta plays a crucial role in statistical tests. It’s directly related to the power of a test, defined as 1 – β. Essentially, power measures the likelihood of correctly rejecting a false null hypothesis. If your test has high power, it means you’re good at spotting significant effects. A high beta, on the other hand, means you might be missing out on important findings.
Understanding the concepts of beta and its relationship with power is crucial for effective research. For more insights, check out this post hoc statistical power.
Understanding beta’s role in hypothesis testing and decision-making is vital. Researchers aim for a low beta to ensure they don’t miss significant differences. So, if you want to feel like a statistical superhero, keep an eye on your beta values! And if you’re looking to supercharge your statistics knowledge, grab a copy of Statistical Methods for the Behavioral Sciences. It’s perfect for those who want to delve deeper into the subject!
In the next sections, we’ll break down how to calculate beta step-by-step. Buckle up, and let’s unravel the mysteries of beta calculations together!
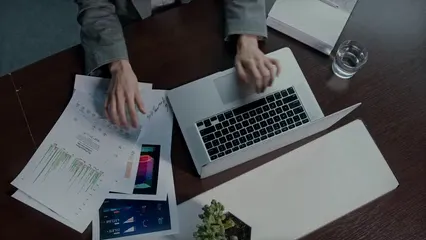
Calculating Beta: Step-by-Step Guide
Required Parameters for Beta Calculation
To calculate beta (β), you’ll need a few key parameters. Let’s break them down into bite-sized pieces.
- Alpha (α): This is the probability of making a Type I error. Essentially, it’s your safeguard against false positives. Commonly, researchers set α at 0.05, meaning there’s a 5% chance of incorrectly rejecting a true null hypothesis. Think of it as the threshold you’re willing to accept before shouting, “Eureka!”
- Sample Size (n): This parameter refers to the number of observations in your study. Larger sample sizes generally lead to more reliable results. So, if you’re planning to conduct a study, remember that the more, the merrier! A sample size of 30 or more is often considered adequate for statistical analysis, especially when using the central limit theorem.
- Effect Size: This measures the strength of the relationship you’re investigating. Effect size can come from previous studies or be estimated based on the context. A larger effect size indicates a more substantial difference or relationship, which can help reduce beta. If you think of effect size as the “wow factor,” then a larger effect size means more reasons for your audience to say, “Wow!”
Each of these parameters plays a crucial role in calculating beta. By understanding their functions, you can better grasp how beta informs the power of your tests and the likelihood of Type II errors. And if you want to get a great resource on effect size, check out Naked Statistics: Stripping the Dread from the Data. It’s a fun way to learn more!
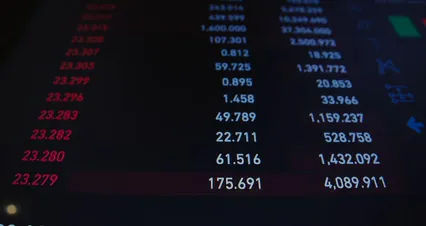
General Formula for Beta
Now that we have our parameters lined up, let’s introduce the formula for calculating beta. The general formula is as follows:
β = P(Fail to reject H0 | H1 is true)
In simpler terms, beta represents the probability of failing to reject the null hypothesis when the alternative hypothesis is true. To derive this formula, you need to understand the context of your test.
First, you define your null and alternative hypotheses. Then, you establish the rejection region based on your chosen alpha level. The area not covered by this rejection region will give you the beta value.
To make it clearer, visualize your sampling distribution. The rejection wall is where you’d reject the null hypothesis. Any sample means that fall to the left of this wall (for a left-tailed test) are where you would fail to reject the null hypothesis when the alternative hypothesis is actually true.
Step-by-Step Calculation
- Gather Data: Obtain paired observations for X and Y.
- Calculate Means: Find the mean of X and the mean of Y.
- Compute Covariance:
Cov(X, Y) = ∑(Xi – X̄)(Yi – Ȳ) / (n – 1)
- Calculate Variance:
Var(X) = ∑(Xi – X̄)2 / (n – 1)
- Find Beta: Plug the values into the beta formula.
Beta Coefficients and Hypothesis Testing
Once we have our beta coefficients, we can perform hypothesis testing. This usually involves setting up null (H0: β = 0) and alternative hypotheses (H1: β ≠ 0). If we reject H0, we conclude that the independent variable significantly predicts the dependent variable.
In regression analysis, a low beta value implies a weak relationship. Conversely, a high beta suggests a strong predictive capability. This makes understanding beta coefficients crucial for interpreting statistical models. If you want a deeper understanding of statistics, consider reading The Art of Statistics: Learning from Data. It’s a fantastic resource!
With these examples, we’ve navigated through various calculations of beta in different contexts. Each situation highlights the importance of sample size, variance, and effect size, which play pivotal roles in determining our beta values. So, whether you’re testing differences between means or just trying to make sense of data, beta has got your back!

Relationship Between Beta, Power, and Sample Size
Understanding Statistical Power
Statistical power measures a test’s ability to detect a true effect. You can think of it as the superhero cape of hypothesis testing! The formula for power is simple: Power = 1 – β. Here, β (beta) represents the probability of making a Type II error.
Increasing the power of a test lowers beta. Why? Because as you enhance your ability to detect differences, you decrease the chances of missing a significant effect. Picture this: you’re at a concert, and the band is playing your favorite song. If the volume is cranked up (higher power), you won’t miss it when the guitar solo kicks in. Conversely, if the sound is low, you might miss those sweet riffs entirely (higher beta).
To boost power, researchers often increase sample size or effect size. A larger sample provides more data points, improving estimates and reducing variability. So, if you’re aiming for a powerful test, remember: more data equals more reliable results! If you’re looking to improve your data analysis skills, grab a copy of Data Science for Business. It’s a great guide for applying data science concepts!
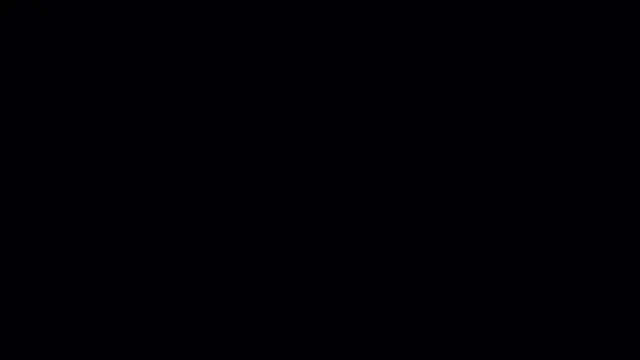
Practical Applications
Researchers can use beta and power calculations to design effective studies. First, they determine the minimum sample size required to achieve desired power levels. This ensures the study is capable of detecting effects if they exist.
Balancing Type I and Type II errors is crucial in practice. Type I error, represented by alpha (α), is the risk of rejecting a true null hypothesis. Reducing alpha can increase beta, making it harder to detect real effects. Researchers must carefully choose these thresholds based on the context of their study.
Imagine a pharmaceutical trial. If the alpha is too low, you risk missing potential benefits of a new medication (high beta). However, if the alpha is set too high, you might falsely conclude the drug is effective when it isn’t. Striking the right balance can be the difference between a successful treatment and a disappointing outcome.
In summary, understanding the relationship between beta, power, and sample size helps researchers craft studies that avoid pitfalls while maximizing their chances of success. Remember, a well-designed study is like a well-prepared meal: it requires the right ingredients and balance!
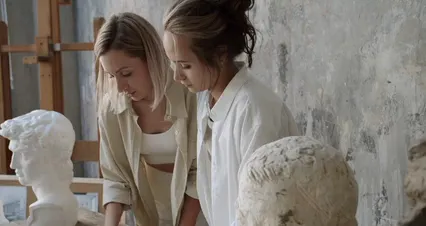
Conclusion
Calculating beta (β) is essential in research and data analysis. It helps researchers make informed decisions by estimating the probability of Type II errors. In simpler terms, beta tells us the chances of missing a significant effect when it exists. This is crucial for ensuring the reliability of study findings.
Understanding beta, alongside alpha (α) and statistical power, provides a comprehensive view of hypothesis testing. Alpha reflects the risk of making a Type I error, while power indicates the likelihood of correctly rejecting a false null hypothesis. The relationship between these statistics is fundamental. A decrease in alpha often leads to an increase in beta, thus impacting power. Researchers must strike a balance to minimize both types of errors.
By applying beta calculations in practice, researchers can enhance their studies’ credibility. A well-calibrated beta value can guide decisions about sample sizes and significance levels. It encourages a thoughtful approach to study design, ultimately leading to more robust conclusions. If you’re interested in practical applications, consider picking up The Complete Guide to Data Analysis. It’s a great resource for hands-on approaches!
So, whether you’re crunching numbers for a groundbreaking study or just trying to make sense of data, remember: calculating beta is your trusty sidekick! Apply this knowledge to your analyses, and watch your research prowess soar.
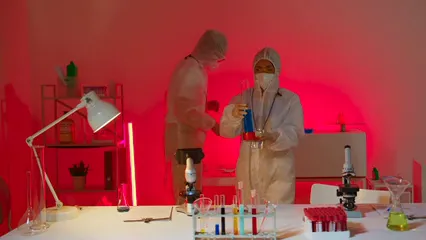
FAQs
What is the difference between beta and alpha?
Beta (β) and alpha (α) are two key concepts in hypothesis testing. Alpha represents the probability of making a Type I error, which is rejecting a true null hypothesis. In simpler terms, it’s the risk of a false positive. For example, declaring a movie a flop when it’s actually a hit is an alpha error. Beta indicates the probability of making a Type II error. This occurs when we fail to reject a false null hypothesis. It’s like missing out on a great dinner because you thought your friends were serving broccoli when they were actually serving your favorite lasagna. While alpha is often set at 0.05, beta values can vary depending on the study’s design and sample size. Together, these statistics help researchers navigate the tricky waters of hypothesis testing. Understanding their roles is crucial for accurate data interpretation.
How can I reduce beta in my study?
Reducing beta in your study is essential for improving the chances of detecting significant effects. Here are some effective strategies: 1. Increase Sample Size: A larger sample size typically leads to lower beta. More data points provide a clearer picture, reducing variability and enhancing the power of your test. 2. Enhance Effect Size: If you can increase the effect size—the magnitude of the change or difference you’re studying—you can lower beta. This can be achieved by modifying your experimental design or interventions. 3. Adjust Alpha Level: While lowering alpha can increase beta, consider optimizing it to balance both. A strategic approach to setting alpha can enhance your study’s power without compromising the risk of Type I errors. 4. Use More Powerful Tests: Some statistical tests have inherently higher power than others. For instance, using a paired t-test instead of an independent t-test can provide better detection of effects when applicable. By employing these strategies, you can effectively reduce beta, enhancing the reliability of your study’s findings.
Why is beta difficult to calculate?
Calculating beta (β) can be challenging for several reasons. One primary factor is the need for knowledge about the true population parameters, particularly the population mean. This information is often unknown, making it tough to determine the probability of a Type II error accurately. Additionally, beta is influenced by various study design elements, including sample size, effect size, and the chosen alpha level. These factors can complicate the calculation. For example, if sample sizes are small, variability increases, making it difficult to pinpoint true effects. Statistical software can assist in calculating beta, but understanding the underlying concepts remains crucial. Researchers must navigate these complexities to ensure their analyses are robust and meaningful.
What is an acceptable value for beta?
An acceptable value for beta (β) often falls between 0.05 and 0.20. A β value of 0.20 indicates a 20% chance of making a Type II error, which is generally considered acceptable in many fields. This means there’s an 80% chance of correctly rejecting a false null hypothesis, indicating a robust test. However, the acceptable beta value can vary depending on the study’s context and objectives. In high-stakes research, such as clinical trials, researchers may aim for lower beta values (e.g., 0.10) to minimize the risk of missing significant effects. Ultimately, the choice of beta value should align with the research goals and the potential consequences of Type II errors. Balancing this with alpha is essential for effective hypothesis testing.
How does beta affect research conclusions?
Beta (β) plays a critical role in shaping research conclusions. A high beta value indicates a greater likelihood of failing to detect a significant effect when one truly exists. This can lead to erroneous conclusions, suggesting that a treatment or intervention is ineffective when, in reality, it may have had a substantial impact. Conversely, a low beta value suggests that the research is more likely to detect significant effects. This enhances the credibility of study findings and supports informed decision-making. Researchers must carefully consider beta when interpreting results, as high beta can result in missed opportunities for discovery. In essence, the implications of beta on research conclusions cannot be overstated. It directly influences the reliability of findings, making it crucial for researchers to understand and optimize beta in their analyses.
Please let us know what you think about our content by leaving a comment down below!
Thank you for reading till here 🙂
All images from Pexels
So, to compute beta, you’ll need:
- The mean of your null distribution.
- The standard error.
- The critical value associated with your alpha level.
With these components, you can calculate the probability of falling into the non-rejection zone, thus yielding your beta value.
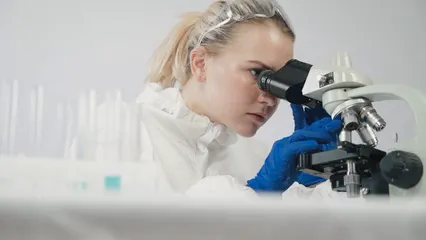
Example Calculations
Example 1: One-Sample Z-Test
Let’s put theory into practice with a step-by-step example of calculating beta using a one-sample Z-test.
- Parameters Defined:
- α (Alpha): 0.10
- n (Sample Size): 50
- σ (Population Standard Deviation): 40
- H0 (Null Hypothesis): μ = 300
- Actual Population Mean: 310
Step 1: Identify the Null Distribution
The null distribution assumes that H0 is true. Since n = 50 (which is greater than 30), the distribution can be approximated as normal with:
- Mean: 300
- Standard Error: Standard Error = 40 / √50 ≈ 5.657
Step 2: Identify the Rejection Wall
Next, we find the sample mean that leads to rejecting H0 using the Z-score. Using a Z-table, the critical value for α = 0.10 in a right-tailed test is approximately 1.28. Thus, we calculate:
Rejection Wall = 300 + 1.28 × 5.657 ≈ 307.25
Step 3: Identify the Actual Distribution
Now, the actual distribution is centered on the true population mean:
- Mean: 310
- Standard Error: 5.657 (same as above)
Step 4: Compute the Proportion of Sample Means in the “Do Not Reject H0” Region
Now we need to calculate the probability that a sample mean is below 307.25 when the true mean is 310. This can be done using the Z-score:
Z = (307.25 – 310) / 5.657 ≈ -0.49
Looking this up in a Z-table gives us a probability of about 0.312. Thus, β for this scenario is approximately 0.312.
Expected Outcomes and Interpretation of Results
A beta value of 0.312 indicates a 31.2% chance of failing to detect a significant difference when there actually is one. In practical terms, this means that in a study, if the population mean is indeed 310, there’s a good chance that the test will incorrectly conclude that it is not significantly different from 300.
This example illustrates how beta calculations can directly impact research conclusions. By understanding these calculations, you can make more informed decisions in your studies. And if you’re looking to enhance your statistics knowledge, consider picking up a copy of Statistics for Dummies. It’s a fantastic resource!
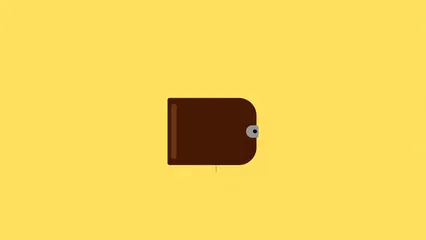
Example 2: Two-Sample T-Test
Let’s tackle another example, shall we? This time, we’ll use a two-sample t-test to calculate beta. The beauty of this test is that it compares the means of two independent groups. Think of it as a friendly competition—who’s got the better average?
- Parameters Defined:
- α (Alpha): 0.05
- n1 (Sample Size 1): 40
- n2 (Sample Size 2): 50
- σ1 (Standard Deviation 1): 20
- σ2 (Standard Deviation 2): 25
- H0 (Null Hypothesis): μ1 = μ2
- Actual Population Means: μ1 = 100, μ2 = 110
Step 1: Identify the Null Distribution
Under the assumption that the null hypothesis holds true, we find the means of both groups:
- Mean1 (group 1): 100
- Mean2 (group 2): 110
The pooled standard deviation (SP) becomes essential here. It’s calculated as:
SP = √((n1 – 1)·σ12 + (n2 – 1)·σ22) / (n1 + n2 – 2)
Plugging in the numbers, we get:
SP = √((40 – 1)·202 + (50 – 1)·252) / (40 + 50 – 2) ≈ 22.91
Step 2: Identify the Rejection Wall
Now, let’s calculate the critical t-value using the t-distribution table for a two-tailed test:
With df = n1 + n2 – 2 = 88 and α = 0.05, the critical t-value is approximately 1.986. The rejection wall becomes:
Rejection Wall = Mean Difference + t · SP · √(1/n1 + 1/n2)
Thus, for our means:
Rejection Wall = (100 – 110) + 1.986 · 22.91 · √(1/40 + 1/50) ≈ -2.47
Step 3: Identify the Actual Distribution
The actual distribution is centered around our true means:
- Mean1 = 100
- Mean2 = 110
Using the same standard error as before, we can calculate the probability of not rejecting the null hypothesis.
Step 4: Compute the Proportion of Sample Means in the “Do Not Reject H0” Region
Using the t-distribution:
P(T < -2.47) ≈ 0.007
Thus, the beta value is approximately:
β = 1 – 0.007 = 0.993
Comparison with the First Example
In our first example, the beta was about 0.312. Here, we see a significant difference—0.993 suggests a much stronger ability to detect differences between groups when the actual means differ. The larger sample sizes and varying standard deviations play a crucial role in this outcome. If you’re interested in learning more about data science, I recommend checking out The Data Science Handbook. It’s a great resource to expand your knowledge!

Example 3: Regression Analysis
Now, let’s shift gears and look at how to calculate beta in the context of regression analysis.
Calculating Beta Coefficients
In regression, beta coefficients represent the relationship between independent variables and the dependent variable. The formula to find the beta coefficient (β) is:
β = Cov(X, Y) / Var(X)
Where:
- Cov(X, Y) is the covariance between the independent variable X and dependent variable Y.
- Var(X) is the variance of the independent variable.
Step-by-Step Calculation
- Gather Data: Obtain paired observations for X and Y.
- Calculate Means: Find the mean of X and the mean of Y.
- Compute Covariance:
Cov(X, Y) = ∑(Xi – X̄)(Yi – Ȳ) / (n – 1)
- Calculate Variance:
Var(X) = ∑(Xi – X̄)2 / (n – 1)
- Find Beta: Plug the values into the beta formula.
Beta Coefficients and Hypothesis Testing
Once we have our beta coefficients, we can perform hypothesis testing. This usually involves setting up null (H0: β = 0) and alternative hypotheses (H1: β ≠ 0). If we reject H0, we conclude that the independent variable significantly predicts the dependent variable.
In regression analysis, a low beta value implies a weak relationship. Conversely, a high beta suggests a strong predictive capability. This makes understanding beta coefficients crucial for interpreting statistical models. If you want a deeper understanding of statistics, consider reading The Art of Statistics: Learning from Data. It’s a fantastic resource!
With these examples, we’ve navigated through various calculations of beta in different contexts. Each situation highlights the importance of sample size, variance, and effect size, which play pivotal roles in determining our beta values. So, whether you’re testing differences between means or just trying to make sense of data, beta has got your back!

Relationship Between Beta, Power, and Sample Size
Understanding Statistical Power
Statistical power measures a test’s ability to detect a true effect. You can think of it as the superhero cape of hypothesis testing! The formula for power is simple: Power = 1 – β. Here, β (beta) represents the probability of making a Type II error.
Increasing the power of a test lowers beta. Why? Because as you enhance your ability to detect differences, you decrease the chances of missing a significant effect. Picture this: you’re at a concert, and the band is playing your favorite song. If the volume is cranked up (higher power), you won’t miss it when the guitar solo kicks in. Conversely, if the sound is low, you might miss those sweet riffs entirely (higher beta).
To boost power, researchers often increase sample size or effect size. A larger sample provides more data points, improving estimates and reducing variability. So, if you’re aiming for a powerful test, remember: more data equals more reliable results! If you’re looking to improve your data analysis skills, grab a copy of Data Science for Business. It’s a great guide for applying data science concepts!
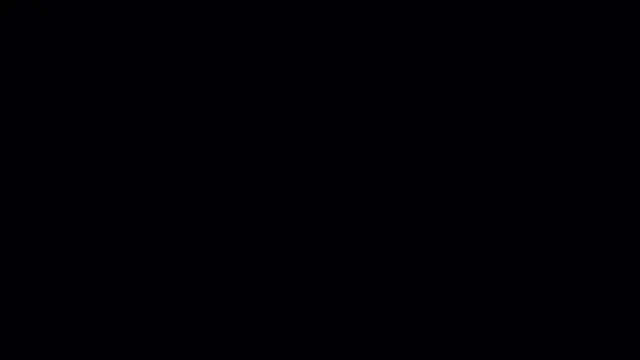
Practical Applications
Researchers can use beta and power calculations to design effective studies. First, they determine the minimum sample size required to achieve desired power levels. This ensures the study is capable of detecting effects if they exist.
Balancing Type I and Type II errors is crucial in practice. Type I error, represented by alpha (α), is the risk of rejecting a true null hypothesis. Reducing alpha can increase beta, making it harder to detect real effects. Researchers must carefully choose these thresholds based on the context of their study.
Imagine a pharmaceutical trial. If the alpha is too low, you risk missing potential benefits of a new medication (high beta). However, if the alpha is set too high, you might falsely conclude the drug is effective when it isn’t. Striking the right balance can be the difference between a successful treatment and a disappointing outcome.
In summary, understanding the relationship between beta, power, and sample size helps researchers craft studies that avoid pitfalls while maximizing their chances of success. Remember, a well-designed study is like a well-prepared meal: it requires the right ingredients and balance!
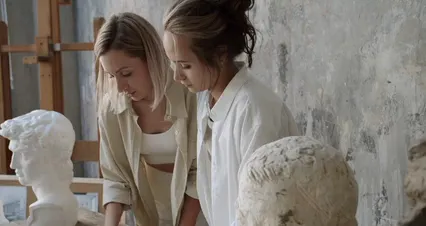
Conclusion
Calculating beta (β) is essential in research and data analysis. It helps researchers make informed decisions by estimating the probability of Type II errors. In simpler terms, beta tells us the chances of missing a significant effect when it exists. This is crucial for ensuring the reliability of study findings.
Understanding beta, alongside alpha (α) and statistical power, provides a comprehensive view of hypothesis testing. Alpha reflects the risk of making a Type I error, while power indicates the likelihood of correctly rejecting a false null hypothesis. The relationship between these statistics is fundamental. A decrease in alpha often leads to an increase in beta, thus impacting power. Researchers must strike a balance to minimize both types of errors.
By applying beta calculations in practice, researchers can enhance their studies’ credibility. A well-calibrated beta value can guide decisions about sample sizes and significance levels. It encourages a thoughtful approach to study design, ultimately leading to more robust conclusions. If you’re interested in practical applications, consider picking up The Complete Guide to Data Analysis. It’s a great resource for hands-on approaches!
So, whether you’re crunching numbers for a groundbreaking study or just trying to make sense of data, remember: calculating beta is your trusty sidekick! Apply this knowledge to your analyses, and watch your research prowess soar.
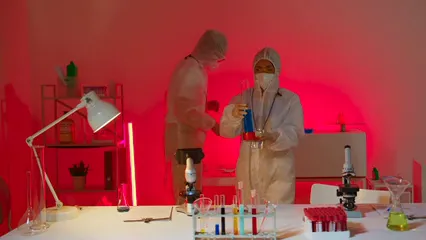
FAQs
Please let us know what you think about our content by leaving a comment down below!
Thank you for reading till here 🙂
All images from Pexels