Introduction
Industrial statistics is like a secret sauce for businesses. It blends data and analytical methods to drive decisions that boost efficiency and effectiveness. In today’s fast-paced world, industries can’t just guess their way to success. They need solid data to back their strategies. Imagine a factory where machines hum in harmony. Each part of the process is optimized through statistical methods. From product design to operational improvements, statistics plays a crucial role in shaping outcomes. It helps identify bottlenecks, forecast demand, and ensure quality. With the right data, companies can make informed decisions that lead to better performance. Whether it’s manufacturing, healthcare, or logistics, the relevance of industrial statistics is undeniable. As we venture deeper into this topic, we’ll uncover how statistical methods enhance performance across various sectors. Let’s set the stage for a comprehensive exploration of industrial statistics.
Understanding Industrial Statistics
What is Industrial Statistics?
Industrial statistics is the application of statistical techniques to analyze and improve operations in manufacturing and service industries. It facilitates data-driven decision-making, ensuring that organizations can respond to changing market demands and enhance overall performance. By harnessing the power of data, companies can identify trends, reduce waste, and improve quality.Historical Context
The journey of industrial statistics is fascinating. It began in the early 20th century alongside the rise of manufacturing processes. Pioneers like W. Edwards Deming and Joseph Juran laid the groundwork for quality control and process improvement. Their work transformed industries by emphasizing the importance of statistical methods for managing quality and efficiency. Over the decades, the field has evolved, integrating advanced techniques and technologies that enhance data analysis capabilities. Today, industrial statistics encompasses a broad range of methodologies, from basic descriptive statistics to complex predictive analytics.Importance in Industry
In today’s competitive landscape, the importance of industrial statistics is paramount. Companies that leverage statistical insights gain a distinct advantage. For instance, in manufacturing, quality control charts help monitor production processes, ensuring that products meet standards. In healthcare, statistical analysis contributes to better patient outcomes by identifying effective treatment protocols. The automotive industry uses data to optimize supply chains and reduce costs, while the technology sector relies on statistical models for product development. Across all sectors, industrial statistics is crucial for making informed decisions. Organizations that embrace data-driven practices can respond swiftly to market shifts and enhance their operational strategies. In summary, industrial statistics is not just a niche field; it is a vital component of modern business success.
Key Concepts in Industrial Statistics
Basic Statistical Methods
Descriptive Statistics
Descriptive statistics is the art of summarizing data. It includes methods to present data in a meaningful way. Common examples are mean, median, mode, and standard deviation. For instance, if a factory produces 1,000 widgets, descriptive statistics help summarize metrics like average production time or defect rate. Why is this important? Well, summarizing data allows managers to quickly grasp performance trends. Instead of sifting through endless spreadsheets, they can see key metrics at a glance. Think of it as the difference between reading a novel and checking out a concise summary. In industrial settings, concise summaries aid in monitoring productivity, quality, and operational efficiency. And speaking of useful resources, if you’re looking to dive deeper into statistical analysis, grab a copy of R Programming for Data Science by Hadley Wickham. This book is an excellent introduction to using R for data analysis, making it a must-have for any aspiring data scientist.
Inferential Statistics
Inferential statistics goes a step further. It helps make predictions or generalizations about a population based on a sample. Key concepts include hypothesis testing and confidence intervals. Hypothesis testing helps determine if a specific claim about a population is valid. For instance, a manufacturer might want to test if a new production method reduces defect rates. A confidence interval, on the other hand, provides a range within which we expect a population parameter to fall. Consider a real-world application. A healthcare company uses inferential statistics to analyze patient recovery times. By collecting data from a sample of patients, they can infer recovery expectations for all patients. This helps them improve treatment protocols and allocate resources effectively. For more insights on this topic, check out the problem with inferential statistics. And if you’re interested in exploring statistical software, MINITAB Statistical Software is a fantastic tool to help you perform statistical analysis with ease and efficiency.
Advanced Statistical Techniques
Regression Analysis
Regression analysis is a powerful tool in industrial statistics. It helps predict outcomes based on relationships between variables. For example, a company might analyze how changes in temperature affect product quality. By identifying these relationships, they can forecast future trends and make data-driven decisions. A case study illustrates this well. A beverage company used regression analysis to optimize its production process. By analyzing historical data, they discovered that slight temperature adjustments led to significant improvements in flavor consistency. This not only enhanced customer satisfaction but also reduced waste. Such predictive power is invaluable in today’s data-driven landscape. For those looking to deepen their understanding of data science concepts, I highly recommend Data Science for Dummies by Anil Maheshwari. It’s a fantastic resource for anyone looking to start their journey in data science with practical insights.
Design of Experiments (DOE)
Design of Experiments (DOE) is all about structured testing. It allows companies to examine multiple factors simultaneously. This technique is crucial for improving processes and products. By systematically varying different parameters, organizations can identify what works best. For example, a tech company might use DOE in product development. They could test different materials and designs to find the optimal combination for a new gadget. By analyzing the results, they can streamline their development process and reduce costs. This structured approach ensures that decisions are data-backed, ultimately enhancing product quality and performance. And if you’re keen on mastering the art of data analysis, check out The Art of Statistics: Learning from Data by David Spiegelhalter. This book will elevate your understanding of statistical reasoning and its practical applications.
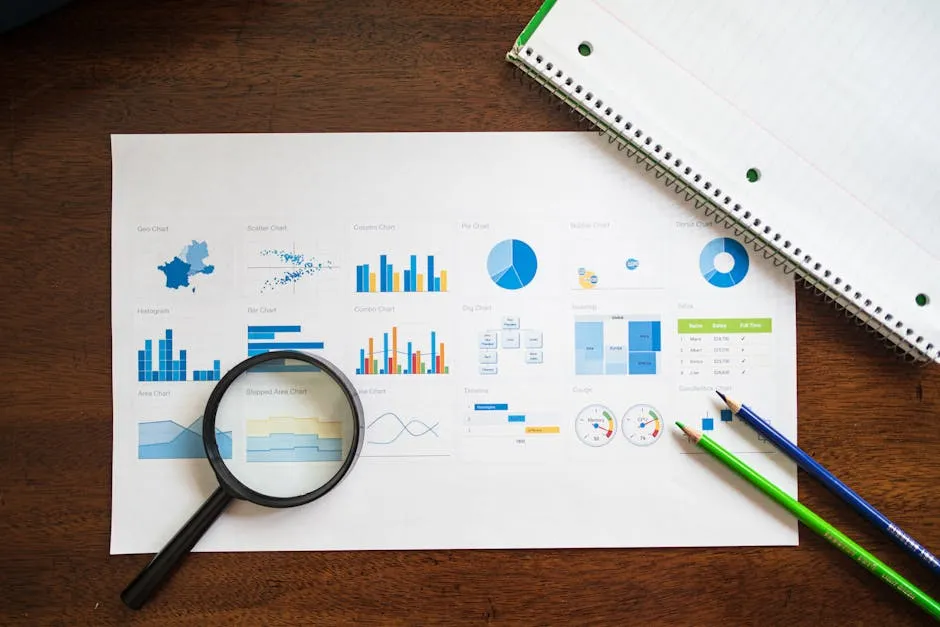
Practical Applications of Industrial Statistics
Quality Control in Manufacturing
Statistical quality control (SQC) is the superhero of manufacturing. It swoops in to save the day by ensuring products meet quality standards. How does it work? Well, SQC employs various statistical methods to monitor and control production processes. Control charts are the main tools here. These charts help track process variation over time. Picture them as the pulse monitor for your production line. If the pulse (or process) goes haywire, it signals to the operators that something is off. This allows for quick adjustments, preventing defects and ensuring consistent quality. Process capability analysis is another critical method. It assesses how well a process performs against set specifications. By examining process data, manufacturers can determine if their processes are capable of producing products within desired limits. If not, it’s time for some process tweaking! This analysis can reveal whether adjustments are necessary or if a complete overhaul is in order.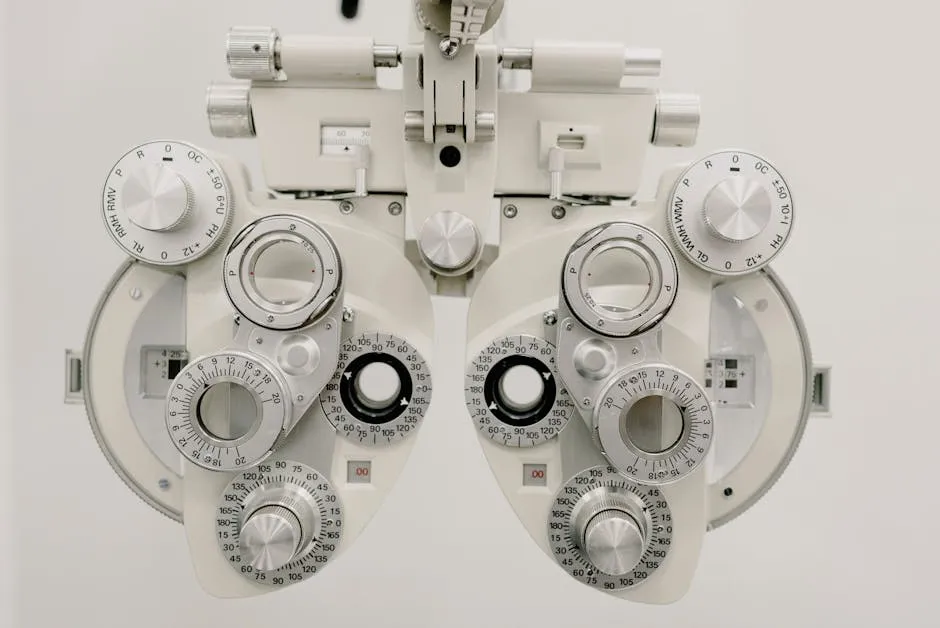

Process Improvement
Process improvement methodologies such as Six Sigma and Lean Manufacturing are like the dynamic duo of operational excellence. They work hand in hand to enhance efficiency and reduce waste, turning factories into well-oiled machines. Six Sigma is all about minimizing defects through data-driven decision-making. It employs DMAIC—Define, Measure, Analyze, Improve, and Control—to tackle process issues. For example, a leading electronics manufacturer faced high defect rates in its production line. By implementing Six Sigma, they reduced defects by 50% in just six months, saving millions in rework costs. Lean Manufacturing, on the other hand, focuses on value creation while minimizing waste. It encourages a streamlined approach, ensuring every step in the production process adds value. Take a car manufacturer that adopted Lean principles. By eliminating unnecessary steps in assembly, they cut production time by 30% and improved customer satisfaction. Both methodologies rely heavily on statistical analysis for informed decision-making. By deploying these strategies, businesses can achieve remarkable improvements, leading to increased profitability and sustainability. If you’re looking for a comprehensive guide on business analytics, check out The Complete Guide to Business Analytics by William J. McCarthy. It’s a great resource for anyone looking to implement analytics in their business strategy.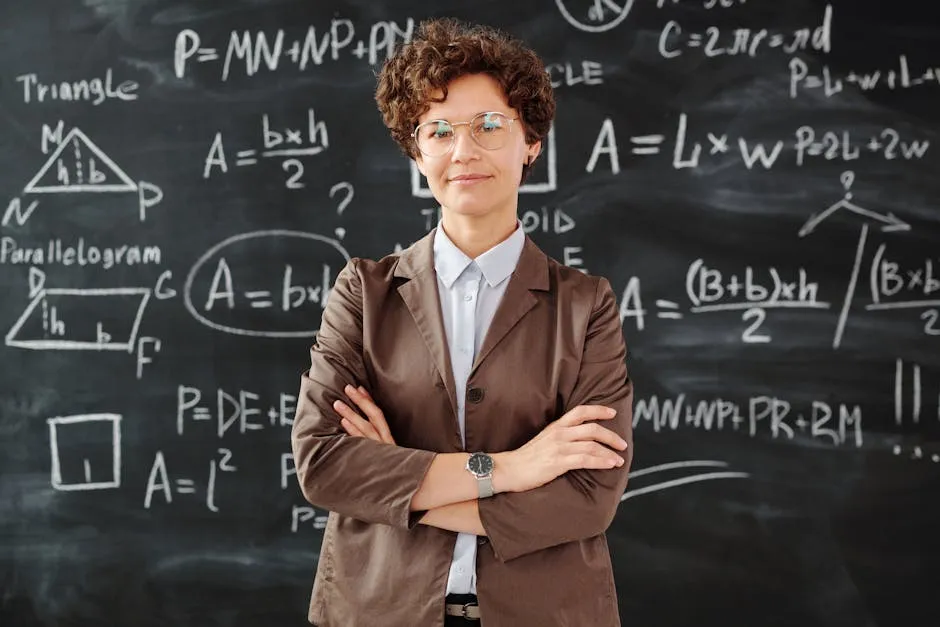
Supply Chain Management
Statistics play a pivotal role in optimizing supply chain management. Think of it as the brain behind the logistics operations. By leveraging data, companies can refine inventory management and demand forecasting. Accurate inventory management is crucial for minimizing costs and meeting customer demands. Statistical methods help predict stock levels, preventing both overstock and stockouts. For instance, a major retail chain used statistical models to analyze sales data. As a result, they improved their inventory turnover rate by 25%, reducing holding costs and enhancing cash flow. Demand forecasting is another area where statistics shine. By analyzing historical sales data and market trends, businesses can predict future demand with greater precision. A beverage company, for example, utilized advanced statistical techniques to forecast seasonal demand fluctuations. This allowed them to optimize production schedules and reduce waste, ultimately boosting profitability. In essence, utilizing statistics in supply chain management transforms chaotic logistics into a harmonious flow of goods, ensuring that the right products reach the right places at the right times. For those who want to dig deeper into data mining techniques, Data Mining: Concepts and Techniques by Jiawei Han is an excellent read.
Tools and Software for Industrial Statistics
Popular Tools
In the realm of industrial statistics, the right tools can make all the difference. Various software options streamline the analysis process, empowering organizations to harness data effectively. R is a popular choice among statisticians. It offers extensive packages for statistical analysis and data visualization. With its flexibility and open-source nature, R enables users to conduct sophisticated analyses without breaking the bank. MINITAB is another go-to software, especially in quality management. It provides user-friendly interfaces and powerful statistical tools, making it ideal for beginners and experts alike. From control charts to regression analysis, MINITAB covers all the essentials for industrial statistics. JMP, developed by SAS, is a favorite for its interactive data visualizations. It allows users to explore data dynamically, uncovering insights that might be missed in static reports. JMP’s capabilities extend to advanced analytics, making it a valuable asset for industries seeking to optimize processes. These tools, among others, form the backbone of industrial statistics. They not only simplify data analysis but also enhance decision-making capabilities, ensuring that businesses stay ahead in the competitive landscape. If you’re interested in a comprehensive approach to business research methods, The Essentials of Business Research Methods by Joe F. Hair Jr. is a great addition to your library.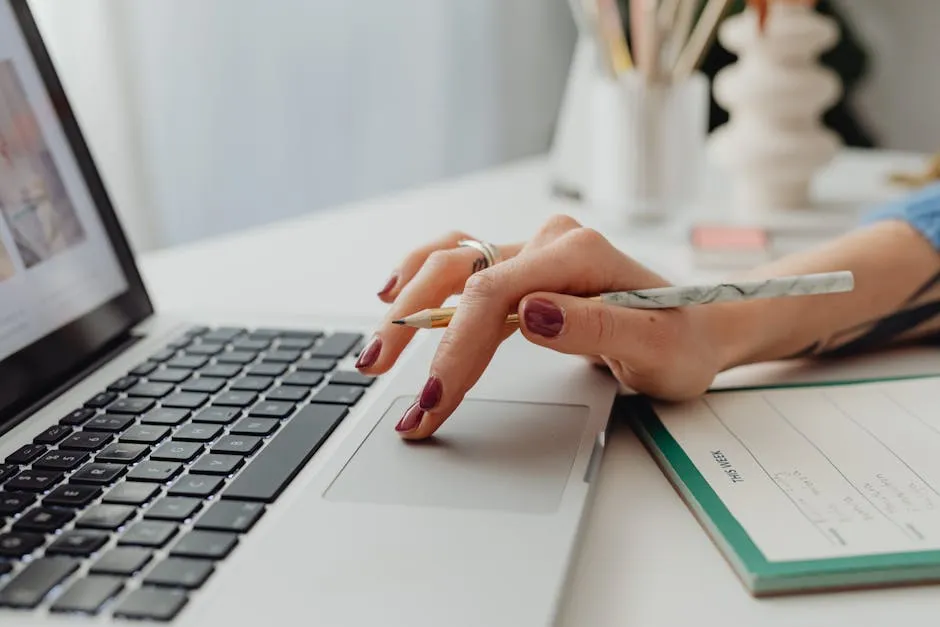
Challenges in Industrial Statistics
Data Quality and Integrity
Data is the lifeblood of industrial statistics. However, it often suffers from issues related to collection and accuracy. Common problems include incomplete datasets, human errors during data entry, and inconsistencies in measurement processes. These issues can lead to misguided decisions and costly mistakes. To enhance data reliability, organizations can implement robust data governance practices. First, standardizing data collection procedures ensures consistency across different teams and processes. Regular training for employees on the importance of accurate data entry can significantly reduce errors. Additionally, employing automated data collection tools minimizes human intervention, leading to more reliable data. Another effective solution is to conduct periodic audits of data quality. By analyzing datasets for anomalies and discrepancies, companies can identify and rectify issues before they escalate. Implementing a feedback loop can also help in continuous improvement, ensuring that data collection methods evolve with technological advancements.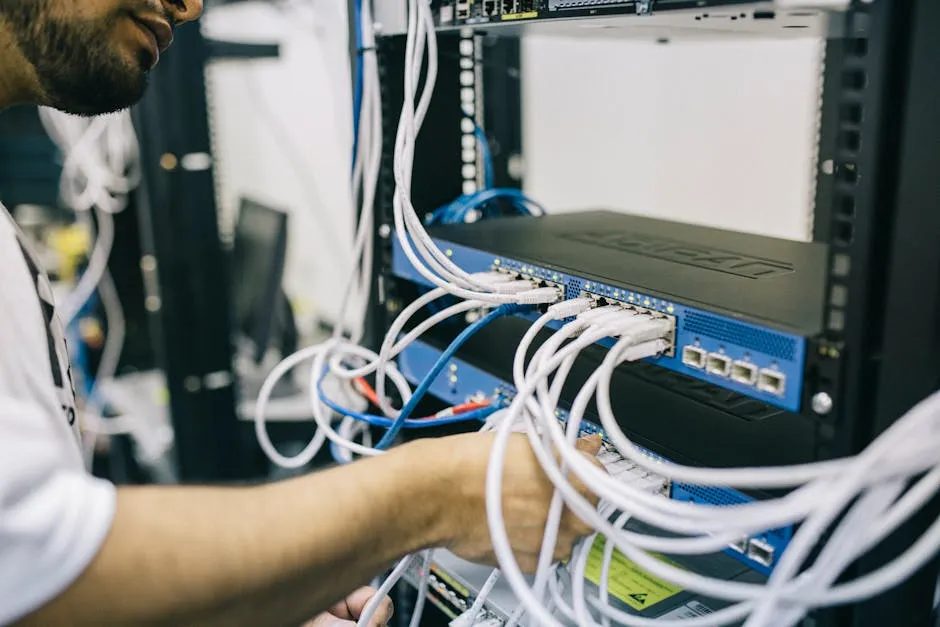
Resistance to Change
Organizations often face cultural challenges when adopting statistical methods. Employees may resist new methodologies due to fear of job loss or discomfort with unfamiliar processes. This resistance can hinder the successful implementation of data-driven strategies. To overcome this challenge, communication is key. Companies should foster an open dialogue about the benefits of statistical methods. Showcasing success stories from other organizations can help employees visualize the positive impact of these changes. Encouraging a culture of learning and growth will make staff more receptive to new ideas. Involve employees in the process of implementing statistical methods. By soliciting their input and feedback, they will feel more invested in the change. Providing training sessions can also build confidence and competence, turning skepticism into support. Ultimately, embracing change is essential for leveraging the full potential of industrial statistics.
Future Trends in Industrial Statistics
Big Data and Analytics
Big data has transformed the landscape of industrial statistics. The sheer volume, velocity, and variety of data available today enable companies to make more informed decisions. With advanced analytics, organizations can uncover hidden patterns and insights that were previously unattainable. Emerging technologies such as artificial intelligence (AI) and machine learning are now pivotal in enhancing statistical analysis. These technologies automate data processing and help identify trends faster than ever. For instance, predictive analytics can forecast equipment failures, allowing for proactive maintenance and reducing downtime. As industries continue to harness big data, the focus will shift towards integrating real-time analytics into operations. This evolution will empower organizations to respond swiftly to changes in demand and optimize processes accordingly. The future of industrial statistics lies in leveraging these technological advancements to drive efficiency and innovation.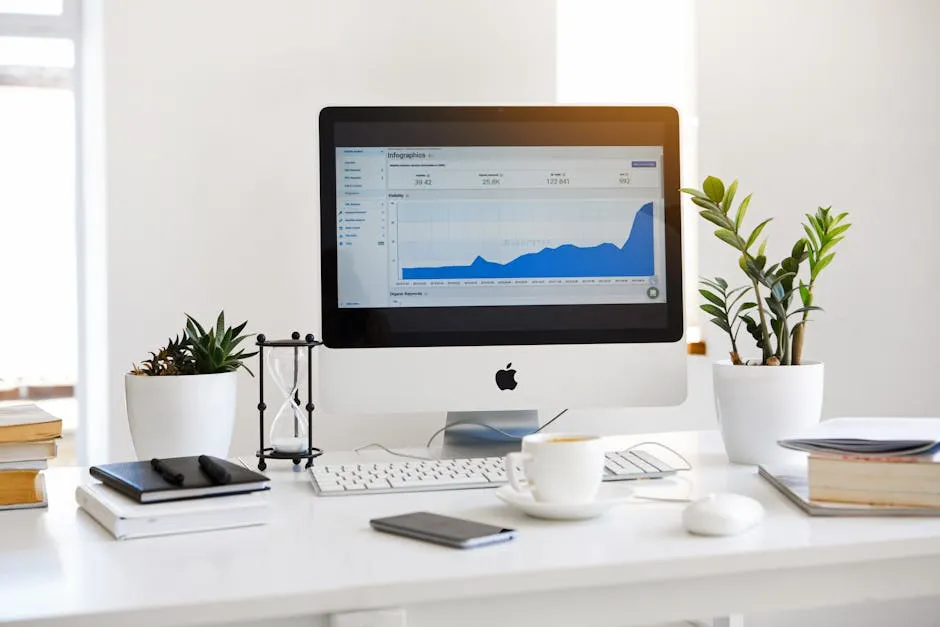
Sustainability and Environmental Metrics
In today’s world, businesses can’t afford to ignore sustainability. The pressure for eco-friendly practices is real. Statistically speaking, industries are turning to data to gauge their environmental impact. This trend is reshaping how companies operate. Using statistics helps organizations track resource usage and waste production. Metrics such as carbon footprints and energy consumption are now crucial. These figures guide decisions aimed at reducing environmental impact. For example, a major automotive manufacturer employs data analytics to optimize production processes. They monitor emissions and adjust operations accordingly, leading to significant reductions in pollution. Another example is the food industry. Companies are analyzing supply chain data to minimize food waste. By understanding consumer demand through statistical models, they can adjust production levels. This not only reduces waste but also saves costs. It’s a win-win situation! Additionally, retail giants are leveraging statistics to enhance sustainability. They analyze customer purchasing behavior to promote eco-friendly products. By presenting these items as appealing options, they encourage consumers to make greener choices. As industries adapt, statistical methods play a pivotal role in sustainability efforts. The integration of data-driven practices not only benefits the environment but also enhances corporate reputation. Companies that embrace these changes are likely to thrive in the long run. If you’re interested in more insights on how to leverage statistics for business decision-making, check out The Business of Data: A Guide to Data-Driven Decision Making by Ian O’Neill.FAQs
What are the most common statistical methods used in industry?
Industrial statistics utilize various methods to enhance decision-making. Regression analysis predicts outcomes based on historical data. Hypothesis testing assesses the validity of claims. Quality control charts monitor production processes for consistency. These methods form the backbone of data-driven strategies across sectors.
How can I learn more about industrial statistics?
To delve deeper into industrial statistics, consider exploring various resources. Books like *Modern Industrial Statistics* provide comprehensive insights. Online courses can also enhance your knowledge. Additionally, training in software such as R or MINITAB can be invaluable. These tools are widely used in the industry for data analysis.
What industries benefit the most from industrial statistics?
Several industries extensively utilize industrial statistics. Manufacturing relies on statistical methods for quality control and process optimization. Healthcare benefits from data analysis to improve patient outcomes. Logistics uses statistics for inventory management and demand forecasting. Finance also leverages these methods for risk assessment and decision-making. Each sector harnesses the power of statistics to drive success.
All images from Pexels