Introduction
Statistics can often feel like an intimidating subject, but fear not! The Z-test calculator is here to simplify hypothesis testing, making it easier to draw meaningful conclusions from your data. Whether you’re a student grappling with your first statistics class or a seasoned analyst looking to brush up on your skills, this comprehensive guide will demystify the Z-test and its calculator. Get ready to dive into the world of statistical significance, where numbers tell a story and data-driven decisions reign supreme!
Summary
In this in-depth article, we will explore the ins and outs of the Z-test calculator, including its purpose, types, and practical applications. We begin by defining what a Z-test is and when to use it, ensuring you have a solid foundation. Next, we will break down the Z-test formula and how to interpret p-values, critical values, and test statistics. You will learn how to effectively use the Z-test calculator, complete with step-by-step instructions and real-world examples. Additionally, we will address common questions about Z-tests and provide insights into when to choose a Z-test over a t-test. By the end, you will possess not only the knowledge but also the confidence to apply the Z-test calculator in your statistical endeavors.
What is a Z-test?
Definition and Purpose
A Z-test is a statistical method. It helps determine if there’s a significant difference between a sample mean and a known population mean. This test operates under the null hypothesis, which states that there is no difference. It’s particularly useful when the sample size is large (typically n > 30) and the population standard deviation is known.
In essence, the Z-test assesses whether the observed sample differs from what we would expect based on the population. This is crucial in fields like psychology, medicine, and quality control, where making data-driven decisions is vital. For those wanting to delve deeper into the art of statistics, consider picking up Statistics for Dummies. It’s a great starting point for anyone feeling overwhelmed by the numbers!
Types of Z-tests
Z-tests come in various flavors to suit different needs:
- One-sample Z-test: This checks if a sample mean significantly differs from a known population mean. Imagine testing if your new batch of cookies is just as delicious as the last!
- Two-sample Z-test: This compares the means of two independent groups. Picture comparing the average heights of boys and girls in a school. This test determines if the difference in means is statistically significant.
- One-tailed Z-test: This is used when you want to test if the sample mean is either greater than or less than the population mean. It answers the question, “Is it better?” or “Is it worse?”
- Two-tailed Z-test: This tests if the sample mean is significantly different, without specifying a direction. Think of it as asking, “Is it different at all?” It’s like checking if one brand of soda tastes different from another, regardless of which one is better.
Importance in Hypothesis Testing
Z-tests are a cornerstone of hypothesis testing. They allow researchers to make informed decisions based on data. With a Z-test, you can evaluate claims and hypotheses statistically. It helps in determining whether observed differences are real or mere flukes of random sampling.
By understanding Z-tests, analysts gain confidence in interpreting results and making conclusions. This confidence can lead to better decision-making in business strategies, scientific research, and beyond. Ultimately, mastering the Z-test and its calculator empowers users to extract valuable insights from their data, turning raw numbers into actionable knowledge. If you’re looking for a deeper understanding of statistics, consider The Art of Statistics: Learning from Data. It’s a great resource!

When to Use Z-tests
Conditions for Using Z-tests
Z-tests serve as a reliable tool in statistical analysis, but they come with specific conditions. First, ensure your sample size is adequate. Typically, a sample size greater than 30 qualifies for a Z-test. Why? Larger samples tend to follow the normal distribution, thanks to the central limit theorem.
Next, the population variance should be known. If you’re unsure about this, it’s a red flag for using Z-tests. Also, your data should ideally follow a normal distribution. For smaller samples, this assumption is crucial. If your data is skewed, consider other tests or transformations. For those looking to understand these concepts better, Understanding Statistics: A Guide for Beginners is a fantastic read!
Lastly, independence is key. Each data point should not influence another. Think of it as making sure everyone at a party is having their own fun without interrupting others.
Real-world Applications
Now, where do Z-tests shine in real-world scenarios? Picture a quality control manager at a factory. They want to ensure their widgets meet specifications. By applying a one-sample Z-test, they can compare the average size of their products with the known standard. If the mean size significantly deviates, it’s time to investigate!
Another prime example lies in medical studies. Imagine researchers testing a new drug. They may want to see if the average recovery time differs from a known baseline. Here, a two-sample Z-test could compare recovery times between two groups: those receiving the new treatment and those on a placebo. If you want to explore more about the intersection of statistics and health, Practical Statistics for Data Scientists is an excellent choice!
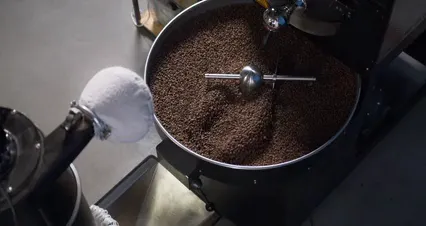
Z-tests also find their place in marketing research. Picture a company launching a new ad campaign. They may want to compare the conversion rates of two different ads. A two-sample Z-test can reveal if one ad significantly outperforms the other.
In summary, Z-tests are invaluable tools in various fields. From manufacturing to healthcare and marketing, they empower analysts to make data-driven decisions. So, when you find yourself pondering whether to use a Z-test, remember these conditions and applications to guide your choice!
Understanding p-values and Z-scores
What is a p-value?
A p-value is a fundamental concept in statistics. It measures the probability of observing test results at least as extreme as those measured, assuming the null hypothesis is true. In hypothesis testing, the null hypothesis typically states that there is no effect or no difference. A lower p-value suggests stronger evidence against the null hypothesis. For instance, a p-value of 0.05 indicates there’s a 5% chance of observing the data if the null hypothesis is accurate. In simpler terms, think of it as your statistical alarm bell—if it rings too loudly (i.e., below your significance level), you might need to reconsider your initial assumptions.
Interpreting p-values
Interpreting p-values can sometimes feel like deciphering a secret code, but it doesn’t have to be complicated! Generally, a p-value below 0.05 is considered statistically significant. This means there is enough evidence to reject the null hypothesis. If your p-value is above this threshold, the evidence isn’t strong enough, and the null hypothesis stands.
However, it’s crucial to remember that a p-value doesn’t tell you how important or meaningful a result is—just how likely you are to see it under the null hypothesis. So, while a p-value of 0.001 may sound impressive, always consider the practical significance in your specific context. If you’re interested in understanding statistical tests better, The Elements of Statistical Learning is a fantastic book!
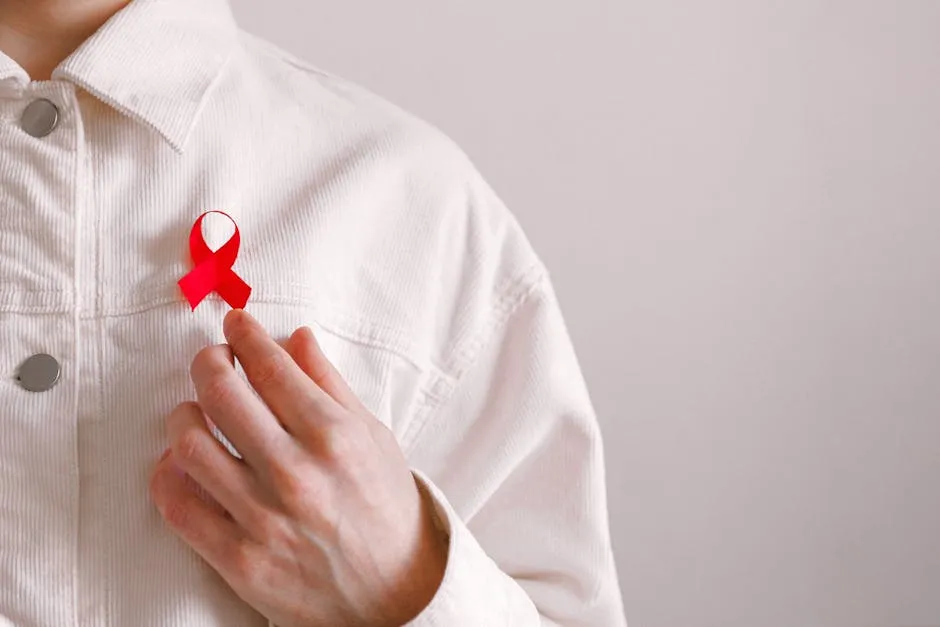
Understanding Z-scores
Z-scores are another essential building block in the realm of statistics. They represent the number of standard deviations a data point is from the mean of a dataset. In other words, a Z-score tells you how unusual a particular observation is. For example, a Z-score of 2 indicates that the data point is two standard deviations above the mean, while a Z-score of -1.5 shows it lies below the mean.
In hypothesis testing, Z-scores play a critical role. They help us determine how extreme our observed sample mean is in relation to the population mean. This is where Z-scores and p-values intersect—the Z-score can be converted into a p-value to assess statistical significance. Higher absolute Z-scores correspond to lower p-values, indicating that our observation is more surprising under the null hypothesis.
Understanding both p-values and Z-scores equips you with the tools to make sound statistical decisions. Whether you’re analyzing data for a school project, conducting research, or making business decisions, these concepts allow you to draw meaningful conclusions. And remember, in the world of statistics, it’s not just about crunching numbers—it’s about piecing together the story they tell! If you’re looking for an engaging introduction to statistics, check out Introduction to Statistics and Data Analysis.
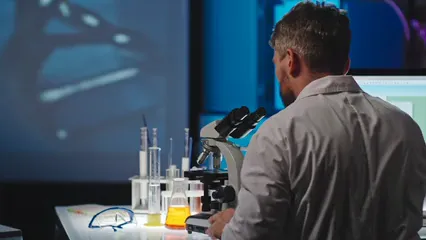
Types of Z-tests: One-tailed vs. Two-tailed
Two-tailed Z-test
A two-tailed Z-test is a statistical procedure used to determine if there’s a significant difference between the sample mean and a known population mean. The null hypothesis (H₀) states that the population mean (μ) equals a specific value (μ₀). The alternative hypothesis (H₁) posits that the population mean is not equal to μ₀.
When to use a two-tailed test? Opt for this approach when you want to check for any difference, regardless of direction. Imagine a factory producing light bulbs. If the average lifespan of these bulbs is claimed to be 1000 hours, a two-tailed test will assess whether the actual mean is either significantly less than or more than 1000 hours.
For example, if we sample 50 bulbs and find a mean lifespan of 980 hours with a population standard deviation of 40 hours, we can calculate the Z-score to see if this difference is significant. A Z-score beyond the critical values (typically ±1.96 for a 95% confidence level) would lead us to reject the null hypothesis.
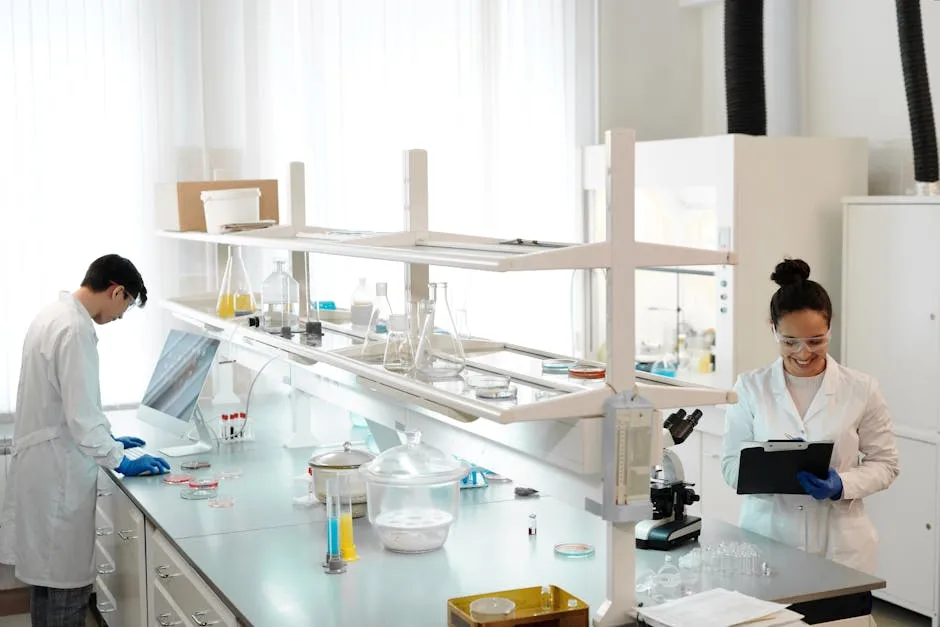
Comparative Analysis
When choosing between one-tailed and two-tailed Z-tests, consider the hypothesis you want to test. A one-tailed Z-test is more powerful when you’re only interested in one direction of the difference. For instance, if a researcher believes that a new diet will lower cholesterol levels, they would use a left-tailed test.
Conversely, a two-tailed Z-test is more conservative. It’s suitable when you’re unsure if the sample mean will be higher or lower than the population mean. One-tailed tests can result in a more significant statistical power, but if you incorrectly assume the direction, you might miss a meaningful result in the opposite direction.
In summary, if you have a specific hypothesis about the direction of the effect, a one-tailed Z-test could be your best bet. If you’re open to finding a difference in either direction, stick with the two-tailed Z-test. It’s all about the context of your research question! And if you want to dive deeper into statistical programming, consider A First Course in Statistical Programming with R.

Interpreting Results
Interpreting results from a Z-test calculator is crucial for drawing meaningful conclusions. After inputting your data, the calculator provides outputs: the Z-score, p-value, and a decision regarding the null hypothesis.
Z-score: This value tells you how many standard deviations your sample mean is from the population mean. A Z-score close to zero indicates that your sample is similar to the population. A larger absolute Z-score suggests a greater difference.
P-value: This number helps assess the strength of the evidence against the null hypothesis. A low p-value (typically less than 0.05) indicates strong evidence to reject the null hypothesis. Conversely, a high p-value suggests insufficient evidence to change your initial assumption.
Decision: Based on the p-value and your significance level (alpha, commonly set at 0.05), you decide whether to reject or fail to reject the null hypothesis. If the p-value is lower than your alpha, reject the null hypothesis. If not, it stands.
Here’s a quick guide:
- Z-score > 1.96 or < -1.96: Significant result (reject null).
- P-value < 0.05: Significant result (reject null).
- P-value > 0.05: Not significant (fail to reject null).
Understanding these outputs allows you to make informed decisions based on your data. Don’t forget to consider the context of your research when interpreting results! If you’re looking for a great overview of statistical inference, check out Statistical Inference.
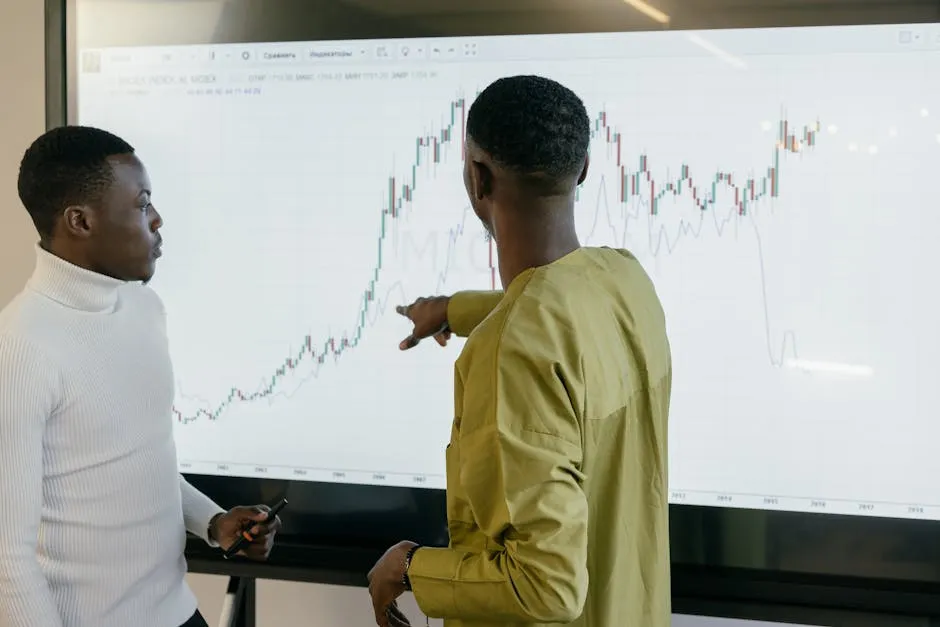
Z-test Examples
Example 1: Quality Control in Manufacturing
Imagine a factory producing light bulbs, where the standard lifespan is claimed to be 1200 hours. The quality control manager tests a sample of 50 bulbs, finding an average lifespan of 1150 hours with a known population standard deviation of 100 hours.
1. Hypotheses:
– Null: H₀: μ = 1200
– Alternative: H₁: μ < 1200
2. Z-test Calculation:
– Sample mean (x̄) = 1150
– Population mean (μ₀) = 1200
– Sample size (n) = 50
– Z = (x̄ – μ₀) / (σ / √n) = (1150 – 1200) / (100 / √50) ≈ -3.54
3. P-value: A Z-score of -3.54 corresponds to a very low p-value (much less than 0.05).
4. Decision: Reject the null hypothesis. The bulbs’ average lifespan is statistically significantly less than 1200 hours.
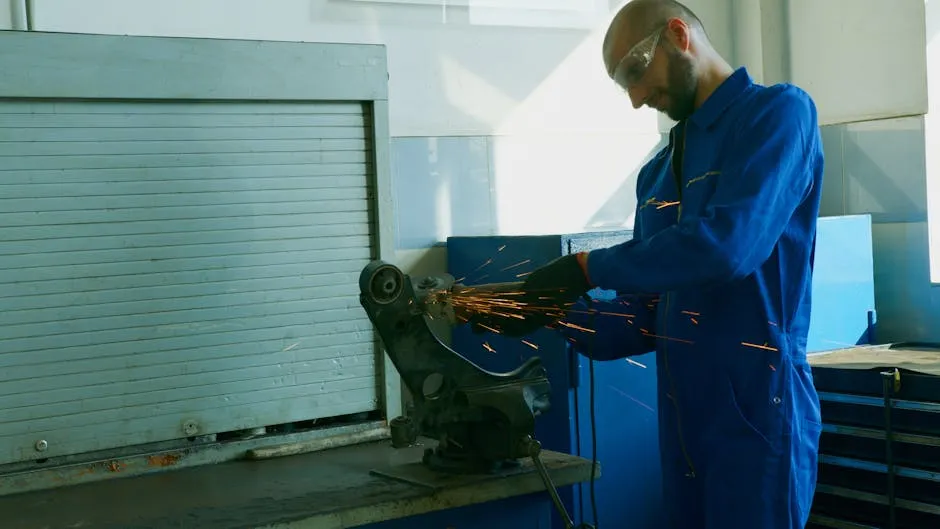
Example 2: Comparing Means in Clinical Trials
Consider a clinical trial comparing the effects of two drugs on reducing blood pressure. Drug A is tested on 40 patients, yielding a mean reduction of 15 mmHg, while Drug B, tested on 60 patients, shows a mean reduction of 12 mmHg. Both drugs have known population standard deviations of 5 mmHg.
1. Hypotheses:
– Null: H₀: μ₁ = μ₂
– Alternative: H₁: μ₁ ≠ μ₂
2. Z-test Calculation:
– Sample means: x̄₁ = 15, x̄₂ = 12
– Sample sizes: n₁ = 40, n₂ = 60
– Z = (x̄₁ – x̄₂) / √((σ₁²/n₁) + (σ₂²/n₂)) = (15 – 12) / √((5²/40) + (5²/60)) ≈ 2.32
3. P-value: A Z-score of 2.32 results in a p-value of approximately 0.02.
4. Decision: Reject the null hypothesis. There’s a significant difference in blood pressure reduction between the two drugs.
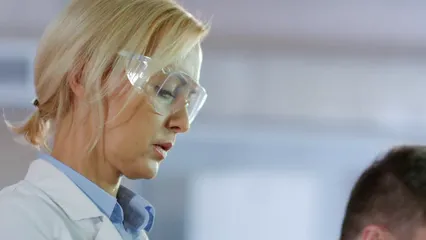
Example 3: Proportions in Marketing Research
A company tests two advertising campaigns. Campaign A reaches 300 people, resulting in 90 purchases (30% conversion), while Campaign B, reaching 400 people, has 120 purchases (30% conversion).
1. Hypotheses:
– Null: H₀: p₁ = p₂
– Alternative: H₁: p₁ ≠ p₂
2. Z-test Calculation:
– Sample proportions: p̂₁ = 0.30, p̂₂ = 0.30
– Sample sizes: n₁ = 300, n₂ = 400
– Pooled proportion: p̂ = (90 + 120) / (300 + 400) = 0.30
– Z = (p̂₁ – p̂₂) / √(p̂(1 – p̂)(1/n₁ + 1/n₂)) = 0 / √(0.30(0.70)(1/300 + 1/400)) = 0
3. P-value: A Z-score of 0 corresponds to a p-value of 1.
4. Decision: Fail to reject the null hypothesis. There’s no significant difference in conversion rates between the two campaigns.
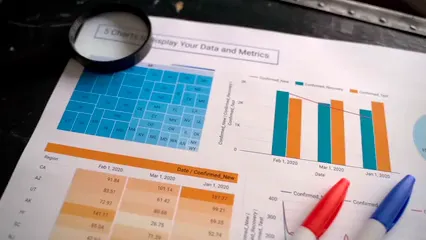
Conclusion
The Z-test calculator is an invaluable tool in statistical analysis. It simplifies hypothesis testing, allowing users to evaluate whether observed data significantly deviates from expected results. From quality control in manufacturing to clinical trials and marketing research, Z-tests provide clarity in decision-making.
Understanding Z-tests enhances your ability to interpret data accurately. Remember, the key outputs—Z-scores and p-values—guide you in determining the significance of your findings. With practice, you’ll confidently apply this knowledge in various real-world scenarios. If you’re interested in a comprehensive overview of data science, The Data Science Handbook is a must-read!
So, whether you’re ensuring the quality of your products or gauging the effectiveness of marketing campaigns, the Z-test calculator equips you with the tools to draw meaningful conclusions from your data. Embrace the power of statistical analysis and let your data-driven decisions shine!
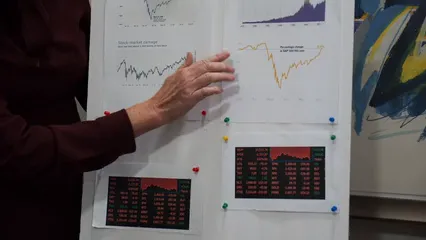
FAQs
What is the difference between a Z-test and a t-test?
A Z-test is used when the sample size is large (usually n > 30) and the population variance is known. In contrast, a t-test is employed for smaller samples or when the population variance is unknown.
When should I use a Z-test?
Use a Z-test when you have a large sample size, know the population standard deviation, and want to compare means or proportions.
How do I calculate the Z-test statistic manually?
To calculate the Z-test statistic, subtract the population mean from the sample mean, divide the result by the standard deviation of the population divided by the square root of the sample size.
What are the assumptions of the Z-test?
The key assumptions include having a simple random sample, knowing the population standard deviation, and ensuring the data follows a normal distribution or is derived from a sufficiently large sample.
Can I use the Z-test for small samples?
While Z-tests are typically used for larger samples, they can be applied to small samples under certain conditions, particularly if the population standard deviation is known and the data is normally distributed. However, the t-test is generally preferred for small sample sizes.
The Z-test is a vital concept in statistical analysis, especially in hypothesis testing. For a comprehensive understanding, refer to the statistics hypothesis testing cheat sheet.
If you’re looking to enhance your cooking skills while diving into statistics, consider checking out the Instant Pot Duo 7-in-1 Electric Pressure Cooker! It’s a great way to whip up meals while you ponder statistical significance!
Please let us know what you think about our content by leaving a comment down below!
Thank you for reading till here 🙂
All images from Pexels