Introduction
Model Output Statistics (MOS) is a superhero in the realm of weather forecasting. It swoops in to save the day by improving the accuracy of predictions. But what exactly is MOS? Think of it as a clever tool that uses statistical techniques to transform complex numerical weather prediction (NWP) outputs into more relatable and understandable weather forecasts.
Weather forecasting has come a long way, yet it still faces challenges. Direct outputs from NWP models can be a bit rough around the edges. They often lack the finesse needed for accurate local predictions. MOS bridges this gap by taking these outputs and refining them, making them more user-friendly. This article aims to shine a light on the methodologies, applications, advantages, and limitations of MOS. It’s time to uncover how this powerful tool enhances our daily weather updates.
Moreover, understanding MOS is crucial for meteorologists, forecasters, and the general public. It allows for the conversion of technical data into information that everyone can grasp. This makes it easier for folks to make plans around the weather—whether it’s a picnic, a road trip, or just figuring out if they need an umbrella.
Speaking of picnics, why not make yours more enjoyable with a stylish outdoor blanket? It’s perfect for lounging on the grass while you enjoy your favorite snacks in the sun!
So, buckle up! We’re about to embark on a journey through the fascinating world of Model Output Statistics. Get ready to learn how it enhances weather forecasting accuracy and helps us decode the weather patterns that affect our lives.

Understanding Model Output Statistics (MOS)
What is MOS?
Model Output Statistics (MOS) is a statistical method used in weather forecasting. Its primary role is to correlate predictands—like temperature, visibility, and wind characteristics—with predictors derived from numerical weather prediction (NWP) models. In simpler terms, MOS helps make sense of the wild and chaotic world of weather predictions.
How does it work? By employing techniques like multiple linear regression, MOS finds the best statistical relationships between the weather outcomes we care about and the model outputs. This means it takes a bunch of data from NWP forecasts and matches it against historical weather data. The result? Enhanced predictions that tell us not just what the weather will be, but how it feels in our specific locale.
The beauty of MOS lies in its ability to relate predictands to predictors effectively. For instance, it might correlate today’s predicted temperature with historical data from the same day. This gives forecasters a more accurate picture of what to expect. By using these statistical techniques, MOS doesn’t just throw numbers at us; it provides insights that are actionable and relevant.
In practice, MOS is a game-changer. It allows forecasters to provide detailed and localized predictions. These forecasts are more relatable and understandable for the public, and they can be crucial for decision-making in sectors like agriculture, aviation, and emergency services. Whether you’re planning a barbecue or flying a plane, MOS has your back, ensuring the weather is no longer a guessing game.
MOS is particularly relevant for sectors like agriculture, where accurate weather forecasts can significantly impact crop planning. statistical distribution relevant for agriculture
And speaking of agriculture, if you’re a gardening enthusiast, you might want to check out this handy Gardening Tools Set. It’s perfect for nurturing your green thumb and ensuring your plants flourish!
In summary, MOS is not just another acronym in the meteorological jargon. It’s a powerful ally in enhancing the accuracy of weather forecasting, transforming complex numerical data into meaningful information for everyone. So next time you check the weather, remember the unsung hero working behind the scenes—Model Output Statistics!
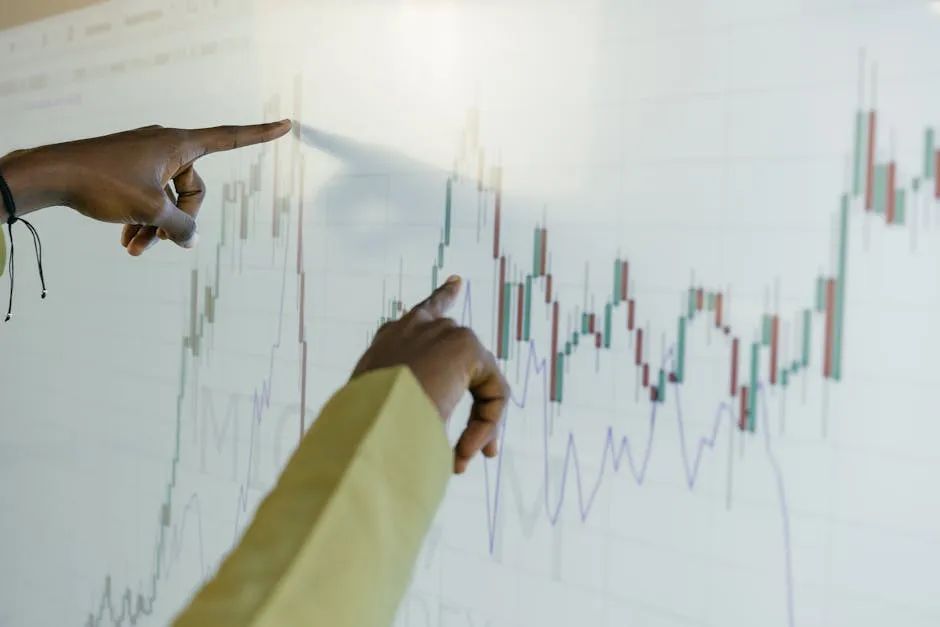
How MOS Works
Model Output Statistics (MOS) utilizes historical weather data to create precise equations that enhance forecasting accuracy. The process begins with gathering a treasure trove of past weather data, which serves as the backbone for developing MOS equations. Think of it as a fine wine aging to perfection; the right data makes all the difference.
To kick off, historical weather data is analyzed alongside outputs from numerical weather prediction (NWP) models. This approach helps forecasters identify valuable predictors—like temperature, humidity, and wind speed—that significantly impact local weather conditions. These predictors are extracted from various data sources, including NWP model outputs, climatic data, and local observations. Each source contributes a unique flavor to the final forecast recipe.
The significance of these predictors cannot be overstated. NWP models, while powerful, often miss the nuances of local weather patterns. MOS steps in to bridge this gap. By statistically correlating local observations with NWP outputs, MOS effectively refines the predictions. It’s like having a local friend who knows the lay of the land when you’re trying to navigate a new city.
But wait, are you prepared for your outdoor adventures? Having a reliable Emergency Survival Kit is essential for any outdoor enthusiast. It ensures you’re ready for anything nature throws your way!
A simple flowchart below illustrates the MOS process:
- Collect Historical Data: Gather past weather data from various stations.
- Analyze NWP Outputs: Look at predictions from numerical models.
- Select Predictors: Identify which weather variables significantly impact local conditions.
- Develop MOS Equations: Use statistical techniques to create relationships between predictors and predicted weather outcomes.
- Generate Forecasts: Produce refined, localized weather predictions.
In short, the MOS process is like crafting a gourmet dish from a blend of quality ingredients. By leveraging historical data and model outputs, MOS produces forecasts that are not just accurate but also meaningful for everyday decisions.
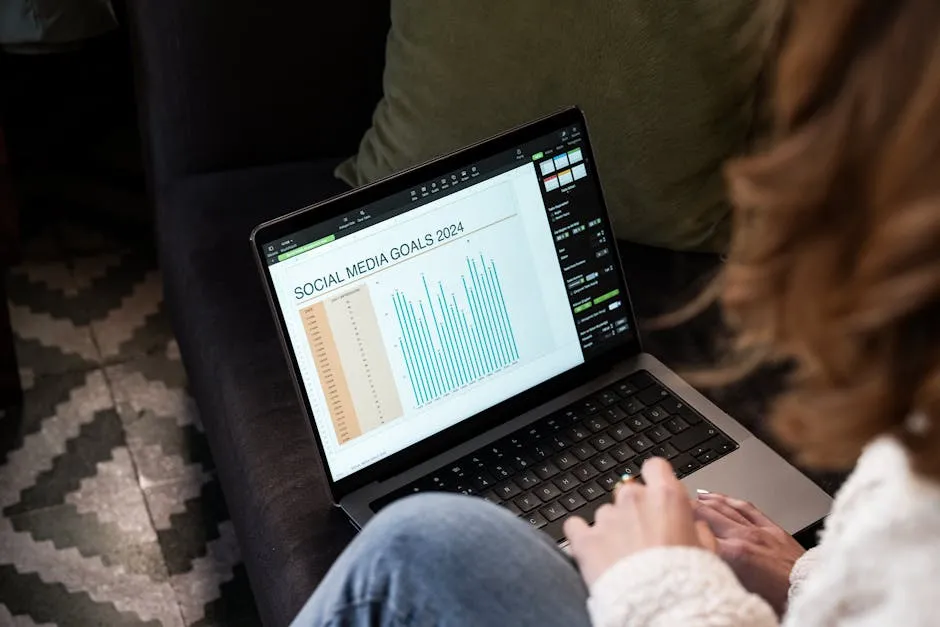
Historical Context of MOS
Development and Evolution
Model Output Statistics (MOS) has an intriguing history that traces back to the 1960s. It all started within the U.S. National Weather Service (NWS), where the quest for improved forecasting began. The Techniques Development Laboratory (TDL) was the birthplace of MOS, conceived in 1965 as a response to the limitations of direct NWP model outputs. By 1968, the first MOS forecasts were issued, marking a pivotal moment in meteorological practices.
Over the years, MOS has undergone remarkable transformations. With the advent of new NWP models and increasing computational power, the MOS equation sets have been continuously refined. This evolution speaks volumes about the commitment of meteorologists to enhance forecasting accuracy.
Key milestones include the transition from experimental applications to operational status, allowing MOS to become a staple in weather forecasting. As technology advanced, so did the sophistication of MOS techniques, incorporating more complex statistical methods that improved predictive accuracy. Today, MOS remains an indispensable tool, with a multi-decadal legacy that continues to shape weather forecasts.

Case Study: MOS in the U.S. National Weather Service
The U.S. National Weather Service employs MOS to produce reliable and localized forecasts. Through various MOS products, such as the Localized Aviation MOS Program (LAMP) and GFS MOS, the NWS provides forecasts for a range of weather events.
LAMP, updated hourly, is particularly beneficial for aviation, ensuring pilots receive timely and accurate weather information. The GFS MOS is another pivotal product, offering forecasts every six hours.
These products exemplify how the NWS utilizes MOS to meet the diverse needs of its user base. By continuously updating and refining MOS methodologies, the NWS ensures that meteorologists have the best tools at their disposal. The commitment to accuracy and reliability in weather forecasting has propelled MOS into a central role in the meteorological toolkit, demonstrating its lasting impact on public safety and weather preparedness.
In conclusion, the historical context of MOS underscores its significance and evolution within weather forecasting. As meteorologists navigate the challenges of accurate predictions, MOS stands as a testament to the innovative spirit of the NWS and its dedication to serving the public.

Applications of MOS
Key Applications in Weather Forecasting
Model Output Statistics (MOS) has become a vital player in the forecasting game. It transforms NWP outputs into usable, relatable weather predictions. Let’s take a closer look at its primary applications.
Temperature Forecasting
MOS excels in predicting temperature. By using historical data, it refines raw model outputs. For instance, MOS can predict daily high and low temperatures with impressive accuracy. This is crucial for agriculture, where crop planning hinges on reliable temperature forecasts.
Wind Speed Predictions
Wind speed is another area where MOS shines. Accurate wind speed forecasts are essential for various sectors. For aviation, knowing wind conditions can determine flight safety and efficiency. Marine navigation also heavily relies on wind predictions to ensure safe passage through waters.
Precipitation Estimates
When it rains, it pours! Well, not always. MOS helps in estimating precipitation amounts, which is vital for flood forecasting and water resource management. By correlating model data with historical events, MOS can provide insights into expected rainfall, helping communities prepare for possible deluges.
Aviation and Marine Contexts
In aviation, MOS forecasts are indispensable. They provide pilots with crucial weather insights during flight planning. For example, the Localized Aviation MOS Program (LAMP) updates forecasts hourly, ensuring pilots have the latest information. In marine contexts, accurate wind and wave predictions are essential for safe navigation. MOS plays a pivotal role in generating these forecasts, allowing vessels to avoid adverse weather.
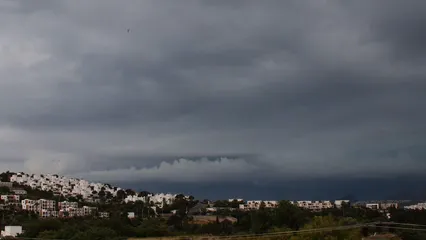
Case Studies of Successful MOS Implementation
Real-world success stories highlight how MOS has revolutionized weather forecasting. One notable example comes from the U.S. National Weather Service (NWS). The NWS employs MOS to improve the accuracy of its forecasts, particularly in predicting local weather events.
In the Midwest, MOS was used to predict severe thunderstorms. By analyzing historical storm data, forecasters could refine their predictions. The result? A significant increase in warning accuracy, allowing residents to prepare in advance. A local resident shared their experience, saying, “Thanks to accurate forecasts, we knew to stay indoors, and it saved us from potential damage.”
Another case involves the use of MOS in predicting winter storms. In the Northeast, MOS forecasts provided timely alerts about expected snow accumulation. As a result, municipalities could mobilize snow removal equipment earlier, minimizing disruptions. City officials noted that the accuracy of MOS predictions led to a reduction in emergency response costs.
Data from various regions also showcases MOS’s effectiveness. For instance, a comparison of MOS forecasts with actual weather data revealed that MOS predictions reduced mean absolute error by 20%. This impressive statistic speaks volumes about the method’s reliability.
In summary, MOS is not just about numbers; it’s about real-world impact. Its applications in temperature forecasting, wind speed predictions, and precipitation estimates have made it an indispensable tool. The success stories from various regions illustrate how MOS enhances forecasting accuracy, ultimately benefiting communities and industries alike.
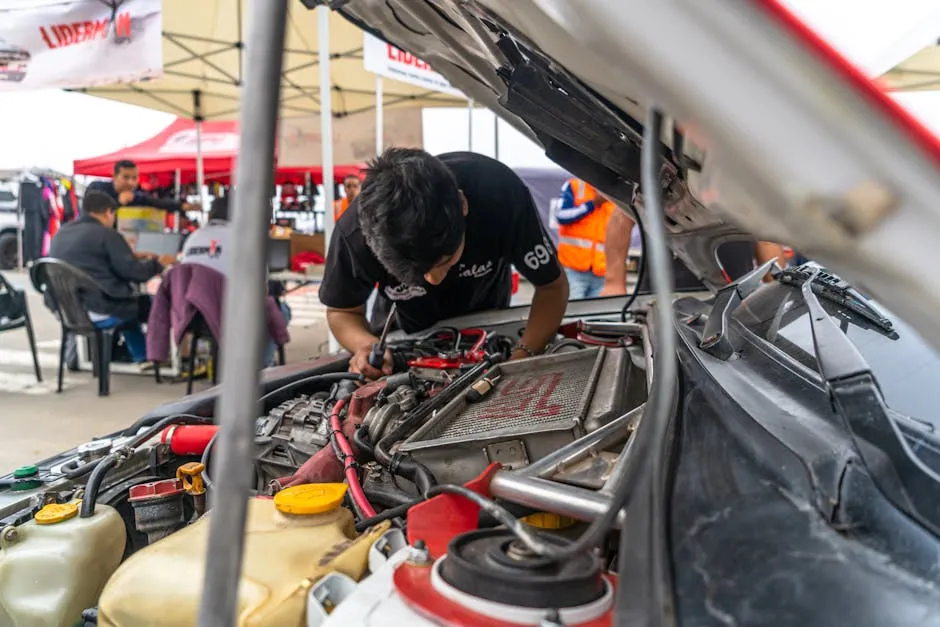
Advantages and Limitations of MOS
Advantages
Model Output Statistics (MOS) brings a range of benefits to the weather forecasting table. First and foremost, it improves accuracy. By correcting biases in numerical weather prediction (NWP) models, MOS provides a more reliable forecast. It’s like having a trusty friend who tells you the weather, but with a PhD in meteorology!
Another significant advantage is the ability to offer probabilities for weather events. Instead of just stating it might rain, MOS can tell you there’s a 70% chance of rain. This gives folks a clearer picture of what to expect. Plus, it helps people make informed decisions. Planning a picnic? MOS can help you decide if you should pack that umbrella or leave it at home.
Further, MOS translates complex meteorological data into forecasts that are relatable to the general public. No more baffling jargon! Instead of “severe thunderstorms expected,” MOS might say, “Expect heavy rain and loud thunder around 5 PM.” This friendly approach makes the weather more accessible and understandable.
And if you’re planning to enjoy the outdoors, don’t forget to check out a Portable Water Filter. Staying hydrated is key, especially on those sunny days!
In short, MOS enhances forecasting by improving accuracy, correcting biases, offering probabilities, and providing forecasts that everyone can grasp. It’s a win-win for meteorologists and the public alike!

Limitations
Despite its many advantages, MOS isn’t without its challenges. One major limitation is the need for extensive historical data. Developing robust MOS equations requires a treasure trove of past observations. Without this historical data, the accuracy of forecasts can take a hit.
Moreover, MOS can be sensitive to changes in NWP models. If a new model emerges or existing models are updated, the MOS equations may need reconfiguration. This can lead to a temporary dip in forecast quality until the new equations are perfected. Think of it as having to learn a new recipe every time you want to whip up your favorite dish!
The complexity of developing these equations is another hurdle. Creating effective MOS equations is a time-consuming process that requires skill and expertise. Meteorologists must analyze vast amounts of data and identify the best predictors for specific weather outcomes. This isn’t a task for the faint of heart!
Local climatic conditions also impact the reliability of MOS forecasts. For example, a region with unique weather patterns may not fit neatly into the statistical models used in MOS. This can lead to inaccuracies in the forecast. It’s like trying to fit a square peg in a round hole—sometimes it just doesn’t work!
In conclusion, while MOS significantly enhances weather forecasting, it comes with its set of challenges. Extensive historical data is crucial, and changes in models can impact forecast quality. The process of developing effective equations is complex, and local climatic conditions can influence reliability. Understanding these limitations helps forecasters and users alike navigate the world of weather predictions.
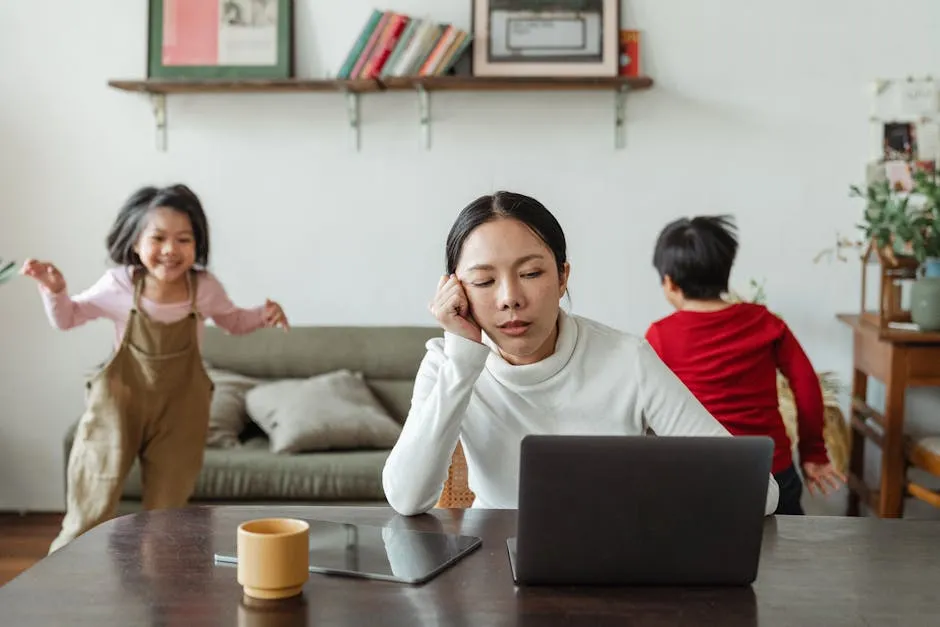
Future of Model Output Statistics
Emerging Trends and Technologies
The future of Model Output Statistics (MOS) looks bright, thanks to emerging trends and technologies. One of the most exciting advancements is the integration of machine learning and artificial intelligence into MOS methodologies. These technologies can analyze vast datasets more efficiently than traditional methods, uncovering patterns that might be missed otherwise.
Machine learning algorithms can enhance the accuracy of MOS by continuously learning from new data. This means that as more weather events occur, the system gets smarter and more precise. Imagine a weather assistant that learns your local patterns and becomes your go-to source for forecasts!
Additionally, the integration of real-time data feeds can revolutionize how MOS operates. By incorporating live observations into the forecasting process, MOS can make adjustments on the fly. This would allow for more immediate and relevant forecasts, especially during rapidly changing weather conditions.
Furthermore, as computational power increases, the complexity of MOS equations can grow. This opens the door for more sophisticated models that account for a wider range of variables. The result could be forecasts that are even more tailored to local conditions and events.

And for those stargazing nights, consider getting an Astronomy Book for Stargazing. It’ll help you make the most of those clear nights!
In summary, the future of MOS is poised for remarkable growth. With machine learning, real-time data integration, and increased computational capabilities, Model Output Statistics will continue to evolve, ultimately improving the accuracy and reliability of weather forecasting for everyone.

The Potential for Integrating MOS with Real-Time Data Feeds and Complex Forecasting Models
Imagine waking up to a weather forecast that adjusts as quickly as the weather itself! Integrating Model Output Statistics (MOS) with real-time data feeds could make this a reality. Currently, MOS processes historical data to improve predictions. But what if it could also utilize live data? That’s where the magic happens!
By feeding real-time observations into MOS, the system could adapt forecasts on the fly. Picture a sunny day turning into stormy chaos. With instant updates from weather stations, MOS can quickly adjust predictions, giving you a more accurate picture of what’s brewing outside. This integration could revolutionize how we prepare for the weather, making it smarter and more responsive.
Now, let’s talk about the potential for more complex forecasting models. As technology advances, the models used in MOS can incorporate machine learning and artificial intelligence. These modern tools can sift through massive datasets faster than a cheetah on roller skates. By analyzing patterns in real-time data, they can refine MOS equations continuously.
This means that MOS wouldn’t just rely on past weather; it would learn from current conditions. Think of it as upgrading from a flip phone to the latest smartphone. The level of detail and accuracy would skyrocket, allowing for precise local forecasts that adapt to changing conditions.
In addition, coupling MOS with advanced models could enhance the understanding of microclimates. These localized weather phenomena often get lost in broader forecasts. By honing in on specific areas, MOS could provide tailored predictions that account for unique geographical features. Is that a mountain range nearby? A sprawling city? MOS would factor it all in!
Furthermore, integrating MOS with real-time data and complex models could lead to improved risk assessments. For instance, during severe weather events, accurate forecasts could inform emergency services and the public. This would allow for timely evacuations or preparations, potentially saving lives and minimizing damage.
In summary, the future of MOS looks promising. By embracing real-time data and advanced forecasting models, it can transform weather predictions into a dynamic, responsive tool. Get ready for the days when your weather app feels like a personal meteorologist, adjusting forecasts as quickly as the clouds roll in!

Please let us know what you think about our content by leaving a comment down below!
Thank you for reading till here 🙂
All images from Pexels