Introduction
Inferential statistics play a crucial role in healthcare research. They allow researchers to draw conclusions about a population based on a sample. However, biases can creep in, skewing results and leading to potentially dangerous misinterpretations.
Biases in inferential statistics are like uninvited guests at a party—no one wants them, yet they always seem to show up. Overcoming these biases is vital for ensuring accurate and reliable results. After all, healthcare decisions based on flawed data can have serious consequences, affecting patient outcomes and public health policies.
This article will focus on several key types of biases, including selection bias, information bias, and confounding bias. We’ll also provide strategies for identifying and mitigating these biases in healthcare studies. By understanding how biases affect research, we can work towards a more accurate and equitable healthcare system.
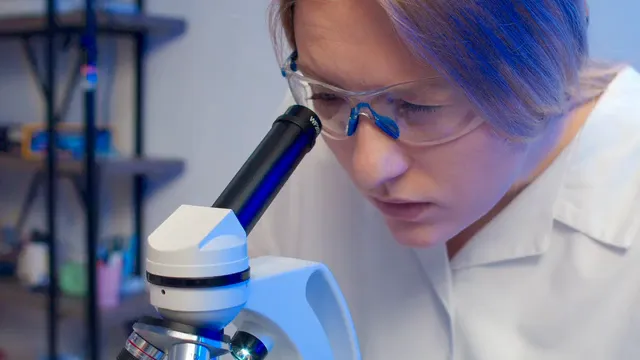
Understanding Bias in Healthcare Studies
Definition of Bias
Bias refers to systematic errors that lead to incorrect conclusions. In the context of statistical analysis, bias can misrepresent the true relationship between variables. This can have severe implications in healthcare, where decisions are often based on research findings. The wrong conclusions can lead to ineffective treatments, misallocation of resources, and even harm to patients.
To help you navigate the complexities of healthcare research and improve your understanding of statistical methods, consider investing in a comprehensive guide on Statistical Methods for Healthcare Research. Such resources can provide invaluable insights into avoiding and correcting biases.

Types of Bias
Selection Bias
Selection bias occurs when the participants included in a study do not represent the overall population. This can happen if certain groups are overrepresented or underrepresented. For example, a study on heart disease that primarily includes older, affluent white males may overlook vital data from younger women or people of different ethnic backgrounds. A real-world case illustrates this: a clinical trial for a new medication that excludes women led to findings that were not applicable to half the population.
This bias can significantly affect the validity of study outcomes, highlighting the need for diverse and representative samples. To combat selection bias, researchers should aim for random sampling, ensuring that every individual has an equal chance of being chosen for the study.
In summary, biases can distort the findings of healthcare studies, leading to misguided conclusions. Recognizing and addressing these biases is crucial for researchers to produce reliable and valid results. In the following sections, we will further explore specific biases and provide effective strategies to overcome them.
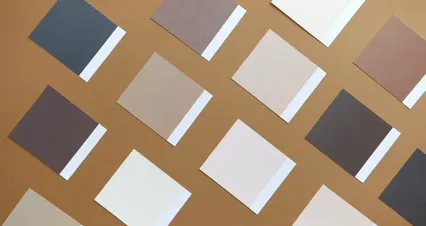
Information Bias
Information bias occurs when there are systematic errors in the collection of data. This can mislead researchers about the true relationship between health exposures and outcomes. Two major types of information bias are recall bias and measurement error.
Recall bias happens when participants do not accurately remember past events or exposures. For instance, in a study assessing the link between diet and heart disease, participants may forget their exact food intake, leading to inaccurate data. An example of this is a clinical trial where older patients often misremember their history of medication use, skewing results.
Measurement error refers to inaccuracies in how data is collected. This can occur in various ways, such as using faulty equipment or poorly designed questionnaires. Imagine a blood pressure study that relies on a device that’s not calibrated correctly. This can lead to misleading conclusions about the effectiveness of a new treatment.
Both recall bias and measurement error can significantly undermine the validity of healthcare studies. Without precise data, it’s like trying to complete a puzzle with missing pieces—certainly frustrating and not quite accurate!
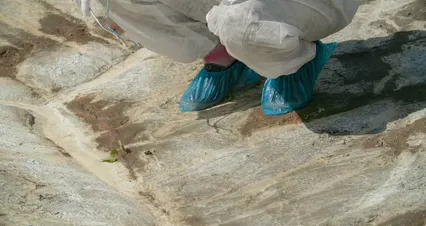
To further ensure your research is accurate, consider using a Data Collection Tool. These can help streamline your data gathering process and reduce errors.
Confounding Bias
Confounding bias arises when an outside factor influences both the exposure and the outcome, creating a false association. This can be particularly problematic in healthcare research, where numerous variables interact.
For example, consider a study investigating the relationship between coffee consumption and heart disease. Age is a confounder here; older individuals might both drink more coffee and have a higher incidence of heart disease. If researchers fail to account for age, they might mistakenly conclude that coffee consumption is a significant risk factor for heart disease.
Other common confounders include socioeconomic status, education level, and lifestyle factors like smoking or exercise. These variables can cloud the true relationship between the primary exposure and outcome, leading to inaccurate interpretations. Addressing confounding requires careful study design and statistical adjustments to clarify the effects of the actual variable of interest.
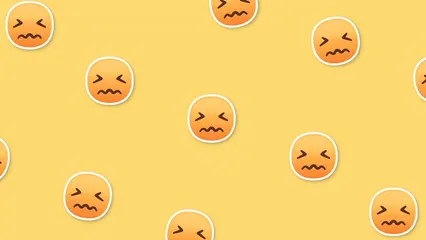
Reporting Bias
Reporting bias occurs when the outcomes of a study are selectively reported based on the nature of the results. This bias skews the interpretation of healthcare findings. Positive results are often more likely to be published than negative or inconclusive ones, creating a misleading representation of evidence.
For instance, in clinical trials, researchers may only publish studies that show a drug is effective while neglecting those that demonstrate ineffectiveness. This selective reporting can lead to an overestimation of the drug’s effectiveness in the literature.
The consequences of reporting bias are far-reaching, potentially leading to poor clinical decision-making and ineffective health policies. To combat this, researchers are encouraged to adhere to reporting guidelines, like CONSORT, ensuring that all outcomes, regardless of their significance, are disclosed. This transparency is vital for fostering trust and advancing healthcare research.
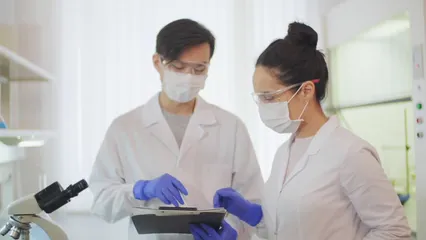
Statistical Techniques for Bias Detection
Detecting bias in healthcare studies is like spotting a hidden monster under your bed. You know it’s there, but finding it can be tricky. Fortunately, several statistical techniques can help shine a light on these lurking biases, ensuring our research conclusions are based on solid ground. Let’s explore three effective methods: sensitivity analysis, directed acyclic graphs (DAGs), and propensity score matching.
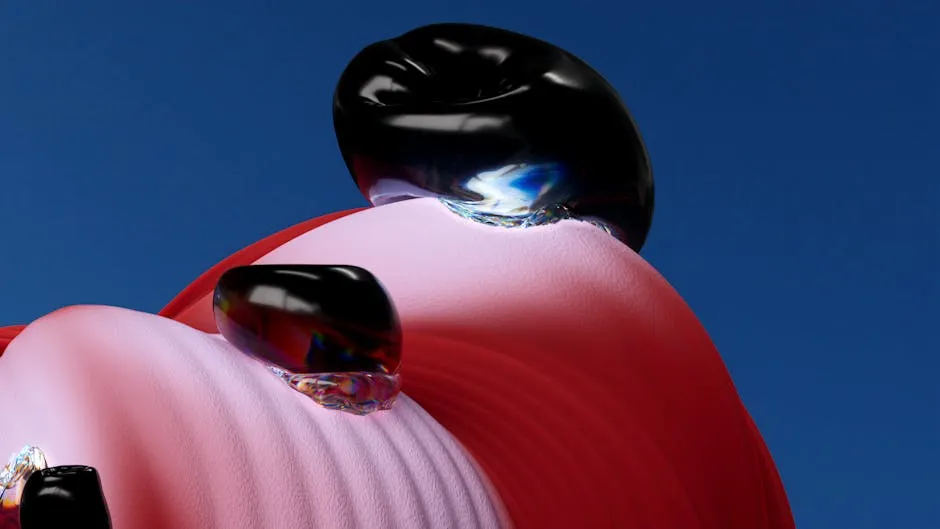
Sensitivity Analysis
Sensitivity analysis allows researchers to assess how sensitive their results are to changes in key assumptions. Think of it as a litmus test for your findings. It helps determine whether different scenarios or variations in data input lead to drastically different conclusions. For instance, if a study claims a new medication reduces symptoms but only under specific conditions, sensitivity analysis can reveal how robust that conclusion is across various scenarios. This technique is particularly useful in healthcare, where small changes can impact patient outcomes significantly.
Directed Acyclic Graphs (DAGs)
DAGs are like flowcharts for biases. They visually represent relationships among variables, making it easier to identify potential confounders and biases in a study’s design. By mapping out these relationships, researchers can pinpoint where biases may sneak into their analyses—like uninvited guests at a party! With DAGs, the causal pathways become clearer, allowing researchers to spot and mitigate biases that could distort their findings. This approach enhances study transparency and improves the quality of healthcare research.
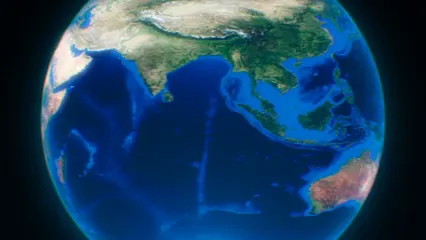
Propensity Score Matching
Propensity score matching is a sophisticated technique that helps control for confounding variables. Imagine you’re comparing two groups: those who received a new treatment and those who didn’t. However, the groups differ in many ways—age, health status, socioeconomic background, and so on. Propensity score matching helps create comparable groups by matching individuals with similar characteristics based on their likelihood of receiving the treatment. This balancing act ensures that any observed effects of the treatment are more likely due to the treatment itself, rather than underlying differences between groups.
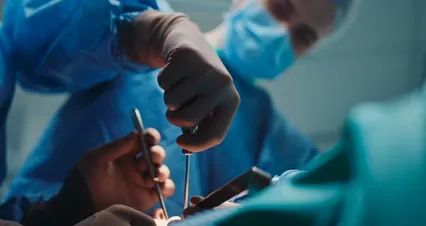
Case Studies
Real-world examples provide valuable insights into how biases can be identified and addressed in healthcare studies. Consider a study exploring the effectiveness of a new diabetes medication. Researchers initially found that the medication led to significant improvements in blood sugar levels. However, further analysis revealed that the participants were predominantly older adults with well-managed diabetes. By recognizing this bias, the researchers adjusted their findings to reflect a broader population, ultimately leading to more accurate conclusions about the medication’s effectiveness across different age groups.
Another notable case involved a study on the correlation between vaccination rates and hospitalization rates during flu season. Initial findings suggested a strong protective effect of vaccinations. However, upon conducting a thorough sensitivity analysis, researchers discovered that the data was skewed due to underreporting of hospitalizations among vaccinated individuals. By addressing this bias, the study provided a clearer picture of the true benefits of vaccination, leading to better public health recommendations.

These examples highlight the importance of bias detection methods in research. By identifying and addressing biases, researchers can improve the accuracy and reliability of their findings, ultimately benefiting patient care and health policies.
Statistical Methods to Adjust for Bias
Propensity Score Methods
Propensity score methods are like the expert bouncers of a research party, controlling who gets in and ensuring a balanced mix of guests. These methods help control confounding bias by matching individuals with similar characteristics. Think of it as pairing a wine lover with a similar palate—this way, the actual effects of the treatment can be tasted without interference from other flavors.
To implement propensity score matching, follow these steps:
- Define the Treatment and Control Groups: Identify who received the treatment and who didn’t. This is your starting point.
- Calculate Propensity Scores: Use logistic regression to estimate the probability of receiving the treatment based on observed characteristics. This score reflects the likelihood of each participant being in the treatment group.
- Match Participants: Pair individuals from the treatment and control groups based on their propensity scores. You can use methods such as nearest neighbor matching or caliper matching for this step.
- Assess Balance: After matching, check whether the groups are balanced in terms of covariates. This ensures that any observed differences in outcomes are likely due to the treatment.
- Analyze Outcomes: Finally, compare the outcomes between the matched groups. This analysis provides a clearer picture of the treatment effect, free from confounding bias.

Propensity score methods increase the validity of study findings, making them more reliable and applicable to real-world scenarios. For a comprehensive understanding of statistical techniques for finance professionals, you can refer to statistical methods for finance professionals 2024.
Understanding statistical methods is essential for researchers in healthcare to ensure accurate results. statistical methods for finance professionals 2024
Weighting Techniques
Weighting techniques adjust for selection bias by giving different weights to participants based on their likelihood of being selected. This approach ensures that the results reflect the overall population more accurately. Imagine a weighted lottery where each ticket’s chance of winning depends on how representative it is of the community.
In healthcare research, weights can be applied in various ways:
- Inverse Probability Weighting: Assign weights inversely proportional to the probability of selection. For example, if older adults are underrepresented in a study, you give them more weight in the analysis.
- Stabilized Weights: These weights stabilize variance estimates and help reduce the impact of extreme weights on the final results.
- Post-stratification: Use weights to adjust for known characteristics, ensuring that your sample reflects the population demographics, such as age, gender, and socioeconomic status.
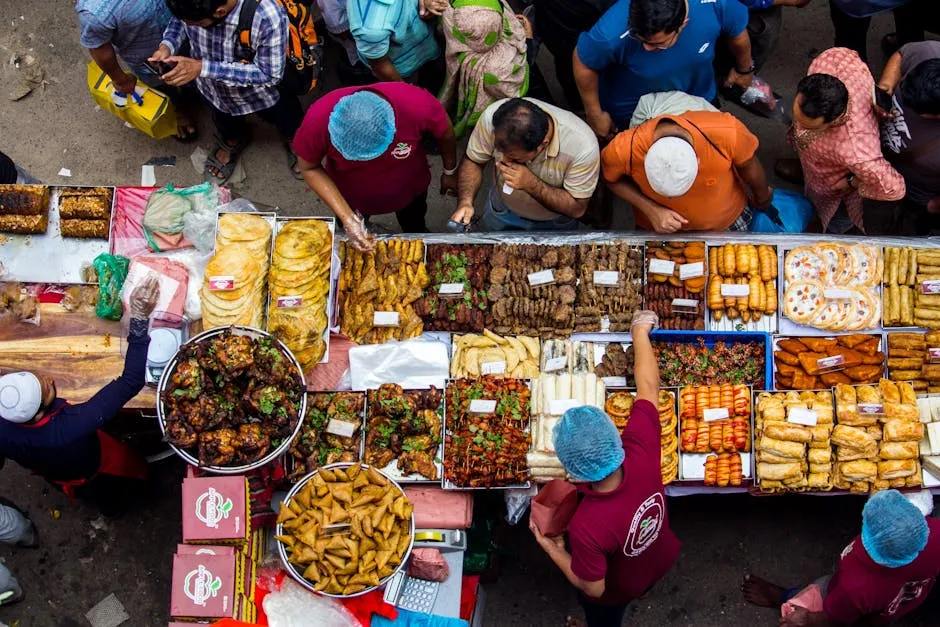
For instance, in a study on flu vaccination, researchers might find that younger individuals are overrepresented. By applying a weighting technique, they can balance the data to ensure that the analysis accurately reflects the vaccination’s effectiveness across all age groups.
To help you implement these techniques effectively, consider using Statistical Analysis Software. Such tools can streamline your analysis and help you apply these methods correctly.
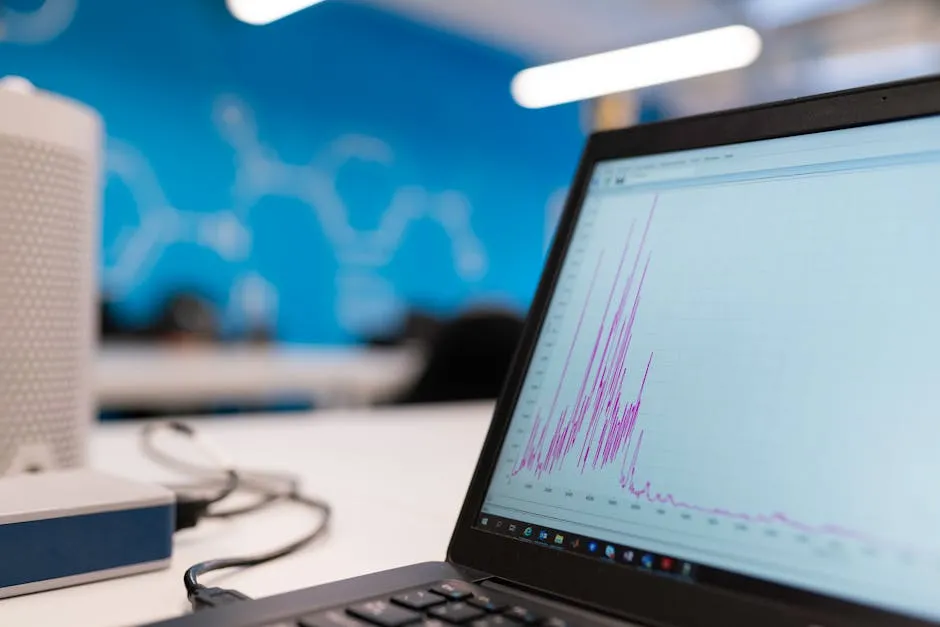
Transparency and Reporting Standards
Transparent reporting is like a good recipe; it ensures everyone knows what ingredients went into the dish. This practice is crucial in minimizing reporting bias. When researchers share their methodologies and results transparently, it reduces the chances of selective reporting, where only favorable outcomes are disclosed.
Several guidelines and standards can help researchers maintain transparency in their studies:
- CONSORT: The Consolidated Standards of Reporting Trials provide a checklist for reporting randomized controlled trials, promoting comprehensive disclosures.
- PRISMA: The Preferred Reporting Items for Systematic Reviews and Meta-Analyses encourages transparency in systematic reviews, ensuring that all relevant findings are presented.
- STROBE: The Strengthening the Reporting of Observational Studies in Epidemiology guidelines emphasize transparency in reporting observational studies.
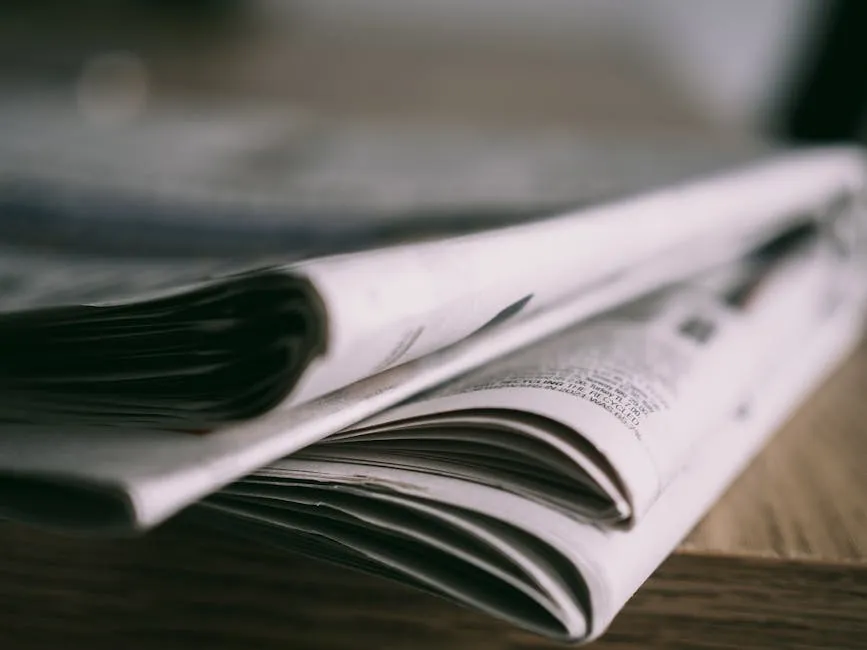
By adhering to these standards, researchers can significantly minimize reporting bias. This commitment not only enhances the trustworthiness of their findings but also contributes to a more robust and credible body of healthcare research.
Conclusion
Recognizing and overcoming biases in inferential statistics is vital for healthcare studies. Biases can distort findings and lead to misleading conclusions, impacting patient care and health policies. By implementing statistical methods such as propensity score matching and weighting techniques, researchers can control for confounding and selection biases, ensuring the reliability of their results.
Transparency in reporting is equally important. Following established guidelines helps minimize reporting bias and fosters trust in healthcare research. Ultimately, addressing these biases not only improves the quality of studies but also enhances their applicability to real-world scenarios. By embracing best practices, researchers can contribute to a more accurate and equitable healthcare system, ultimately benefiting patient outcomes and public health.
FAQs
What are the common types of bias in healthcare studies?
Bias can sneak into healthcare studies like a cat on a hot tin roof. Here are some popular culprits: Selection Bias: When the sample population isn’t representative of the larger group. Think of it as inviting only your closest friends to a party and claiming it was a success based on their rave reviews. Information Bias: This occurs when data collection methods lead to inaccurate information. It’s like using a broken compass; you might think you’re heading north, but you’re actually lost. Confounding Bias: Happens when an outside factor influences both the exposure and outcome. Imagine trying to figure out if eating ice cream causes sunburns, while forgetting that people eat ice cream more often during sunny weather. Reporting Bias: This is when studies selectively report positive results, leaving out the less favorable outcomes. It’s like bragging about your A’s but hiding your D’s from your report card.
How can biases affect healthcare outcomes?
Biases are like the sneaky gremlins of research. They can twist data and lead to misleading conclusions. If researchers overlook biases, they might recommend ineffective treatments or conclude that a drug works when it doesn’t. This can directly affect clinical decision-making, ultimately harming patients. Trust us; nobody wants to end up with a prescription that’s about as useful as a chocolate teapot!
What statistical methods can help in identifying bias?
Researchers have some nifty tools to spot biases in their data: Sensitivity Analysis: This technique tests how sensitive outcomes are to changes in assumptions. It’s like checking how sturdy your chair is by bouncing on it a few times. Directed Acyclic Graphs (DAGs): These visual aids help map relationships among variables, highlighting potential confounders. Picture a family tree where you can see who’s related to whom, helping you avoid awkward dinner conversations. Propensity Score Matching: This method creates comparable groups in observational studies, helping to control for confounding variables. Think of it as matching socks before doing laundry—everything looks better when it’s paired up!
Why is transparency important in healthcare research?
Transparency in healthcare research is like a clear window on a sunny day. It fosters trust and credibility in findings. When researchers disclose methodologies and potential biases, it allows others to scrutinize and replicate studies. This openness can lead to more robust healthcare practices and policies. After all, would you trust a recipe that didn’t show you how much sugar it used?
How can researchers ensure diverse representation in studies?
Ensuring diverse representation is crucial for equitable healthcare outcomes. Here are some actionable steps researchers can take: Define Inclusion Criteria: Clearly outline who can participate and actively seek participants from various demographics. Utilize Community Engagement: Collaborate with community leaders to reach underrepresented populations. It’s like going where the party is—if you want to have a good time, you gotta invite everyone! Adapt Recruitment Strategies: Use multiple channels to recruit participants, such as social media, local events, and community organizations. A wider net catches more fish! Monitor Demographics: Regularly assess participant demographics throughout the study to ensure diversity is being maintained. If you notice a lack of representation, adjust your recruitment efforts mid-study.
Please let us know what you think about our content by leaving a comment down below!
Thank you for reading till here 🙂
All images from Pexels