Introduction
In the world of research, two terms often steal the spotlight: statistical significance and practical significance. While they may sound similar, they serve different purposes and can lead to vastly different interpretations of data. Statistical significance refers to the likelihood that a result or relationship observed in data is not due to random chance. It’s like saying, “Hey, something’s happening here!” But just because we find a statistically significant result doesn’t mean it carries any real-world weight. This is where practical significance comes into play.
Understanding both concepts is crucial for anyone involved in research or data analysis. Researchers need to grasp the nuances to make informed decisions, interpret findings correctly, and avoid the pitfalls that come with misinterpretation. A classic misconception is that if a result is statistically significant, it automatically implies that the effect is substantial or meaningful. Spoiler alert: it doesn’t!
Imagine a medical study that finds a new drug reduces symptoms of a disease with a stunning p-value of 0.01. Hooray, right? But wait! If the actual symptom reduction is only 0.01%, is it worth the hype? This is the essence of the difference between statistical and practical significance.
The purpose of this article is to clarify these differences, provide relatable examples, and offer guidance on how to assess both types of significance. By the end, you’ll be armed with the knowledge to distinguish between the two and to understand the implications of your findings better. Let’s dive into the numbers and make sense of what they truly mean!
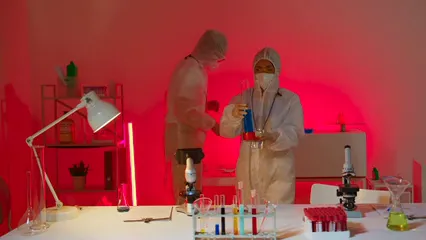
Understanding Statistical Significance
Definition and Importance
Statistical significance is a term that pops up whenever researchers run hypothesis tests. It revolves around p-values, which tell us the probability of observing our results, assuming the null hypothesis (the idea that nothing is happening) is true. If this p-value falls below a predetermined threshold—often set at 0.05—we declare the results statistically significant. Essentially, we’re saying, “The evidence is strong enough to reject the null hypothesis!”
But hold your horses! Just because we have a low p-value doesn’t automatically mean our findings are practically significant. For instance, consider a large clinical trial that finds a statistically significant difference in recovery times between two treatments. If the difference is merely a few hours, it may not be meaningful in the real world, especially if the side effects of the treatment are severe.
On the other hand, practical significance steps in like a wise old sage, asking, “Is this finding meaningful in our everyday lives?” It emphasizes the importance of the effect size and its implications. A statistically significant result with a minuscule effect size might lead to decisions that are more about numbers than about making a difference.
If you want to explore the broader context of data science, consider “The Data Science Bible”. This comprehensive guide covers a wide range of topics in data science.
Recognizing the difference between these two concepts is crucial for researchers and decision-makers alike. Misunderstanding can lead to misguided conclusions. For instance, a medical study might find a statistically significant reduction in symptoms, but if the actual improvement is negligible, should we really be celebrating?
In research and data analysis, it’s essential to evaluate both statistical and practical significance. This ensures that our findings not only have a mathematical basis but also a meaningful impact on real-world applications. As you move forward in your work, remember to apply this understanding. Strike a balance between the numbers that make you look good and the implications that truly matter.
With this knowledge in your toolkit, you can improve the relevance and impact of your findings, making sure that your research isn’t just a statistical exercise but a valuable contribution to your field. Let’s make our findings count—beyond the p-value! tips for effective data analysis in economics and statistics
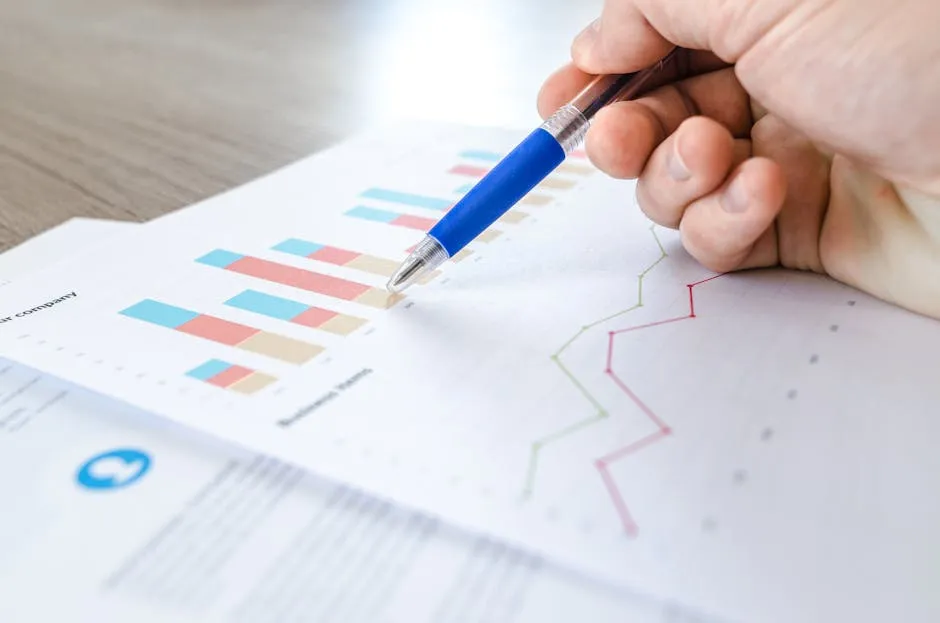
FAQs
What is the key difference between statistical significance and practical significance?
Statistical significance focuses on whether a result is likely due to chance, often measured by a p-value. Practical significance assesses whether the effect size is meaningful in real-world contexts. Simply put, statistical significance answers “Is there an effect?” while practical significance asks “Does this effect matter?”
Why is statistical significance not enough?
Focusing solely on statistical significance can lead to misleading conclusions. A result may be statistically significant due to a large sample size yet have a trivial effect size. This can result in decisions that lack real-world relevance. For instance, a statistically significant finding in a clinical trial might not translate to meaningful improvements for patients.
How can I determine if my findings are practically significant?
To assess practical significance, consider the effect size and its relevance in your field. Tools like Cohen’s d can help quantify effect sizes. Discuss with peers or experts in your field to understand what constitutes a meaningful effect. Contextualize your findings to ensure they resonate with real-world implications.
What role do p-values play in understanding significance?
P-values help determine statistical significance by indicating the likelihood that the observed results could occur by chance if the null hypothesis were true. However, they do not convey information on effect size or practical relevance. It’s essential to look beyond p-values and consider effect sizes and confidence intervals for a complete picture.
Can a result be statistically significant but not practically significant?
Absolutely! For example, a study might find a statistically significant increase in test scores due to a new educational technique, but if the average improvement is only 0.1 point, it’s unlikely to affect teaching practices. Thus, while the result is statistically significant, it may lack practical significance in real-world applications.
Please let us know what you think about our content by leaving a comment down below!
Thank you for reading till here 🙂
Understanding statistical significance and practical significance is essential for informed decision-making in research. tips for effective data analysis in economics and statistics
All images from Pexels
Use visual aids like graphs to present results. They can effectively illustrate the differences between statistical and practical significance. For instance, a bar graph with error bars can show the CI, helping your audience visualize the reliability of the results.
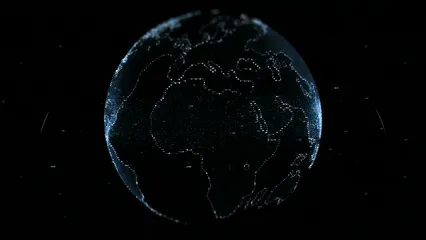
To further enhance your understanding of data presentation, consider “Graphs and Charts for Presenting Data”. This book is an excellent resource for anyone looking to improve their data visualization skills.
Lastly, engage in discussions about your findings. Sometimes, a statistically significant result may not resonate with practitioners in the field. By opening channels of communication, you can better align your research with real-world applications and ensure that your findings have a meaningful impact.
In summary, accounting for both statistical and practical significance in research design and reporting is essential. Clear definitions, proper sample sizes, and effective communication will pave the way for research that truly informs practice and decision-making.

Conclusion
In this article, we’ve unraveled the complex dance between statistical significance and practical significance. On one hand, we’ve got statistical significance, that flashy p-value which shouts, “Look at me! I’m statistically significant!” But hold your applause! Just because something is statistically significant doesn’t mean it matters in the real world. Think of it as the glittering trophy you win for a game where the score doesn’t count.
On the other hand, practical significance steps in like a wise old sage, asking, “Is this finding meaningful in our everyday lives?” It emphasizes the importance of the effect size and its implications. A statistically significant result with a minuscule effect size might lead to decisions that are more about numbers than about making a difference.
If you want to explore the broader context of data science, consider “The Data Science Bible”. This comprehensive guide covers a wide range of topics in data science.
Recognizing the difference between these two concepts is crucial for researchers and decision-makers alike. Misunderstanding can lead to misguided conclusions. For instance, a medical study might find a statistically significant reduction in symptoms, but if the actual improvement is negligible, should we really be celebrating?
In research and data analysis, it’s essential to evaluate both statistical and practical significance. This ensures that our findings not only have a mathematical basis but also a meaningful impact on real-world applications. As you move forward in your work, remember to apply this understanding. Strike a balance between the numbers that make you look good and the implications that truly matter.
With this knowledge in your toolkit, you can improve the relevance and impact of your findings, making sure that your research isn’t just a statistical exercise but a valuable contribution to your field. Let’s make our findings count—beyond the p-value! tips for effective data analysis in economics and statistics
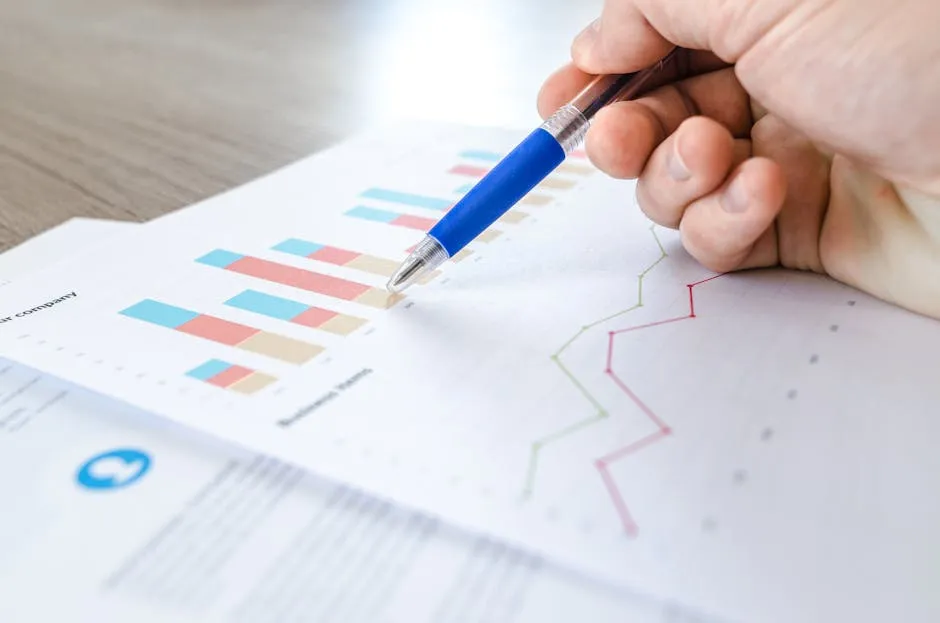
FAQs
Please let us know what you think about our content by leaving a comment down below!
Thank you for reading till here 🙂
Understanding statistical significance and practical significance is essential for informed decision-making in research. tips for effective data analysis in economics and statistics
All images from Pexels
Next, consider your sample size carefully. Large samples can lead to statistically significant results for trivial effects. A small, meaningful effect might require a larger sample to detect, so plan accordingly. This upfront thinking can save you from the heartbreak of statistical significance that lacks practical relevance.
For those interested in business research methods, I recommend “Business Research Methods”. It provides a solid foundation for conducting research that truly matters.
Reporting results is another area where clarity is paramount. Researchers should communicate findings with emphasis on both statistical and practical significance. For example, instead of simply stating a p-value, include effect sizes and CIs in discussions. This provides readers with a fuller picture of the findings’ implications.
Use visual aids like graphs to present results. They can effectively illustrate the differences between statistical and practical significance. For instance, a bar graph with error bars can show the CI, helping your audience visualize the reliability of the results.
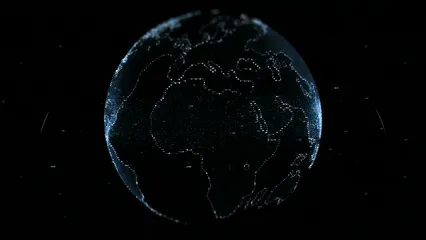
To further enhance your understanding of data presentation, consider “Graphs and Charts for Presenting Data”. This book is an excellent resource for anyone looking to improve their data visualization skills.
Lastly, engage in discussions about your findings. Sometimes, a statistically significant result may not resonate with practitioners in the field. By opening channels of communication, you can better align your research with real-world applications and ensure that your findings have a meaningful impact.
In summary, accounting for both statistical and practical significance in research design and reporting is essential. Clear definitions, proper sample sizes, and effective communication will pave the way for research that truly informs practice and decision-making.

Conclusion
In this article, we’ve unraveled the complex dance between statistical significance and practical significance. On one hand, we’ve got statistical significance, that flashy p-value which shouts, “Look at me! I’m statistically significant!” But hold your applause! Just because something is statistically significant doesn’t mean it matters in the real world. Think of it as the glittering trophy you win for a game where the score doesn’t count.
On the other hand, practical significance steps in like a wise old sage, asking, “Is this finding meaningful in our everyday lives?” It emphasizes the importance of the effect size and its implications. A statistically significant result with a minuscule effect size might lead to decisions that are more about numbers than about making a difference.
If you want to explore the broader context of data science, consider “The Data Science Bible”. This comprehensive guide covers a wide range of topics in data science.
Recognizing the difference between these two concepts is crucial for researchers and decision-makers alike. Misunderstanding can lead to misguided conclusions. For instance, a medical study might find a statistically significant reduction in symptoms, but if the actual improvement is negligible, should we really be celebrating?
In research and data analysis, it’s essential to evaluate both statistical and practical significance. This ensures that our findings not only have a mathematical basis but also a meaningful impact on real-world applications. As you move forward in your work, remember to apply this understanding. Strike a balance between the numbers that make you look good and the implications that truly matter.
With this knowledge in your toolkit, you can improve the relevance and impact of your findings, making sure that your research isn’t just a statistical exercise but a valuable contribution to your field. Let’s make our findings count—beyond the p-value! tips for effective data analysis in economics and statistics
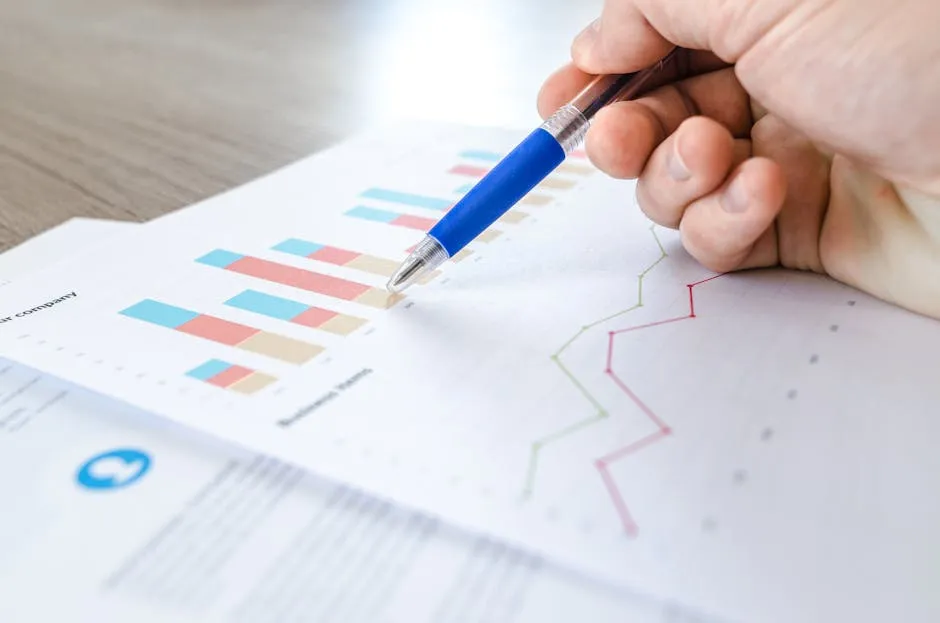
FAQs
Please let us know what you think about our content by leaving a comment down below!
Thank you for reading till here 🙂
Understanding statistical significance and practical significance is essential for informed decision-making in research. tips for effective data analysis in economics and statistics
All images from Pexels
Now, let’s consider another study examining a different treatment. It reported a statistically significant reduction in symptoms, but the CI spanned from 0.1 to 1 mmHg. While the effect is statistically significant, the lower end of the CI suggests that the actual effect could be negligible in real-world terms. Thus, the first study’s findings are not just statistically significant; they also indicate practical relevance.
If you’re eager to learn more about data visualization, consider picking up “Data Visualization: A Practical Introduction”. This book is perfect for anyone wanting to communicate data insights effectively.
When interpreting CIs, context is key. For instance, a CI that includes zero suggests a lack of practical significance, even if the results are statistically significant. Researchers must consider whether the entire CI reflects an effect that is meaningful in practice.
Best Practices for Researchers
Designing studies that account for both statistical and practical significance can be a game changer. Begin by defining a meaningful effect size before you even collect data. This helps ensure that your study is designed to detect effects that matter, rather than just those that are statistically significant.
Next, consider your sample size carefully. Large samples can lead to statistically significant results for trivial effects. A small, meaningful effect might require a larger sample to detect, so plan accordingly. This upfront thinking can save you from the heartbreak of statistical significance that lacks practical relevance.
For those interested in business research methods, I recommend “Business Research Methods”. It provides a solid foundation for conducting research that truly matters.
Reporting results is another area where clarity is paramount. Researchers should communicate findings with emphasis on both statistical and practical significance. For example, instead of simply stating a p-value, include effect sizes and CIs in discussions. This provides readers with a fuller picture of the findings’ implications.
Use visual aids like graphs to present results. They can effectively illustrate the differences between statistical and practical significance. For instance, a bar graph with error bars can show the CI, helping your audience visualize the reliability of the results.
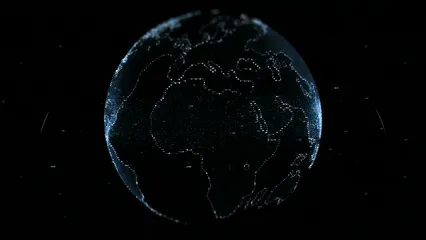
To further enhance your understanding of data presentation, consider “Graphs and Charts for Presenting Data”. This book is an excellent resource for anyone looking to improve their data visualization skills.
Lastly, engage in discussions about your findings. Sometimes, a statistically significant result may not resonate with practitioners in the field. By opening channels of communication, you can better align your research with real-world applications and ensure that your findings have a meaningful impact.
In summary, accounting for both statistical and practical significance in research design and reporting is essential. Clear definitions, proper sample sizes, and effective communication will pave the way for research that truly informs practice and decision-making.

Conclusion
In this article, we’ve unraveled the complex dance between statistical significance and practical significance. On one hand, we’ve got statistical significance, that flashy p-value which shouts, “Look at me! I’m statistically significant!” But hold your applause! Just because something is statistically significant doesn’t mean it matters in the real world. Think of it as the glittering trophy you win for a game where the score doesn’t count.
On the other hand, practical significance steps in like a wise old sage, asking, “Is this finding meaningful in our everyday lives?” It emphasizes the importance of the effect size and its implications. A statistically significant result with a minuscule effect size might lead to decisions that are more about numbers than about making a difference.
If you want to explore the broader context of data science, consider “The Data Science Bible”. This comprehensive guide covers a wide range of topics in data science.
Recognizing the difference between these two concepts is crucial for researchers and decision-makers alike. Misunderstanding can lead to misguided conclusions. For instance, a medical study might find a statistically significant reduction in symptoms, but if the actual improvement is negligible, should we really be celebrating?
In research and data analysis, it’s essential to evaluate both statistical and practical significance. This ensures that our findings not only have a mathematical basis but also a meaningful impact on real-world applications. As you move forward in your work, remember to apply this understanding. Strike a balance between the numbers that make you look good and the implications that truly matter.
With this knowledge in your toolkit, you can improve the relevance and impact of your findings, making sure that your research isn’t just a statistical exercise but a valuable contribution to your field. Let’s make our findings count—beyond the p-value! tips for effective data analysis in economics and statistics
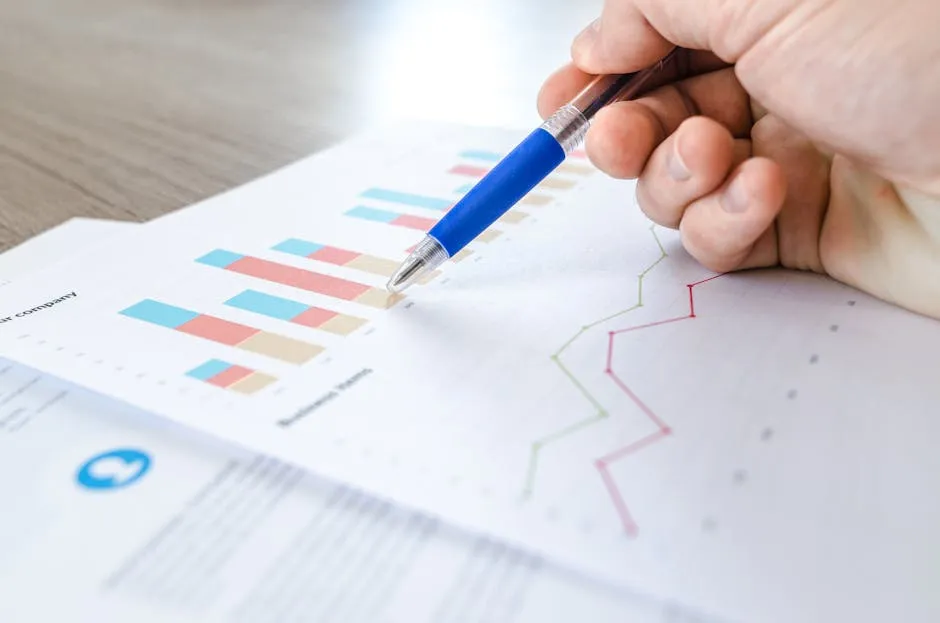
FAQs
Please let us know what you think about our content by leaving a comment down below!
Thank you for reading till here 🙂
Understanding statistical significance and practical significance is essential for informed decision-making in research. tips for effective data analysis in economics and statistics
All images from Pexels
In contrast, a different study might find a new exercise regimen that only shows a 15% reduction in heart disease risk, but it translates to a real-world effect of 3%. Here, both statistical significance and practical significance align, providing valuable insights for public health recommendations.
For those looking to dive deeper into understanding significance in research, you might want to check out “The Data Science Handbook”. This comprehensive guide will enhance your understanding of data analysis in various contexts.
Both types of significance have their place. Statistical significance tells us if an effect exists, while practical significance helps us gauge its real-world impact. Using both together leads to a more nuanced interpretation of research findings.
In the end, it’s about balancing the numbers and understanding their implications. So, the next time you see a shiny p-value, don’t forget to ask if it really matters in the grand scheme of things!

Examples of CIs in Research
Confidence intervals (CIs) provide a window into the reliability of study findings. They are crucial in understanding both statistical and practical significance. In one study on a new medication for high blood pressure, researchers found a statistically significant reduction in readings. The 95% CI ranged from 2 to 5 mmHg. This range tells us that if we repeated the study, we can be 95% confident that the true effect lies within those bounds.
Now, let’s consider another study examining a different treatment. It reported a statistically significant reduction in symptoms, but the CI spanned from 0.1 to 1 mmHg. While the effect is statistically significant, the lower end of the CI suggests that the actual effect could be negligible in real-world terms. Thus, the first study’s findings are not just statistically significant; they also indicate practical relevance.
If you’re eager to learn more about data visualization, consider picking up “Data Visualization: A Practical Introduction”. This book is perfect for anyone wanting to communicate data insights effectively.
When interpreting CIs, context is key. For instance, a CI that includes zero suggests a lack of practical significance, even if the results are statistically significant. Researchers must consider whether the entire CI reflects an effect that is meaningful in practice.
Best Practices for Researchers
Designing studies that account for both statistical and practical significance can be a game changer. Begin by defining a meaningful effect size before you even collect data. This helps ensure that your study is designed to detect effects that matter, rather than just those that are statistically significant.
Next, consider your sample size carefully. Large samples can lead to statistically significant results for trivial effects. A small, meaningful effect might require a larger sample to detect, so plan accordingly. This upfront thinking can save you from the heartbreak of statistical significance that lacks practical relevance.
For those interested in business research methods, I recommend “Business Research Methods”. It provides a solid foundation for conducting research that truly matters.
Reporting results is another area where clarity is paramount. Researchers should communicate findings with emphasis on both statistical and practical significance. For example, instead of simply stating a p-value, include effect sizes and CIs in discussions. This provides readers with a fuller picture of the findings’ implications.
Use visual aids like graphs to present results. They can effectively illustrate the differences between statistical and practical significance. For instance, a bar graph with error bars can show the CI, helping your audience visualize the reliability of the results.
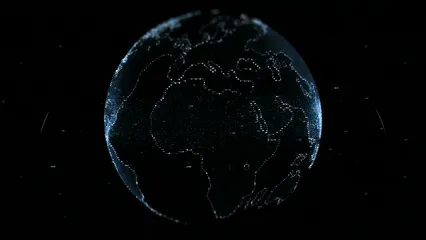
To further enhance your understanding of data presentation, consider “Graphs and Charts for Presenting Data”. This book is an excellent resource for anyone looking to improve their data visualization skills.
Lastly, engage in discussions about your findings. Sometimes, a statistically significant result may not resonate with practitioners in the field. By opening channels of communication, you can better align your research with real-world applications and ensure that your findings have a meaningful impact.
In summary, accounting for both statistical and practical significance in research design and reporting is essential. Clear definitions, proper sample sizes, and effective communication will pave the way for research that truly informs practice and decision-making.

Conclusion
In this article, we’ve unraveled the complex dance between statistical significance and practical significance. On one hand, we’ve got statistical significance, that flashy p-value which shouts, “Look at me! I’m statistically significant!” But hold your applause! Just because something is statistically significant doesn’t mean it matters in the real world. Think of it as the glittering trophy you win for a game where the score doesn’t count.
On the other hand, practical significance steps in like a wise old sage, asking, “Is this finding meaningful in our everyday lives?” It emphasizes the importance of the effect size and its implications. A statistically significant result with a minuscule effect size might lead to decisions that are more about numbers than about making a difference.
If you want to explore the broader context of data science, consider “The Data Science Bible”. This comprehensive guide covers a wide range of topics in data science.
Recognizing the difference between these two concepts is crucial for researchers and decision-makers alike. Misunderstanding can lead to misguided conclusions. For instance, a medical study might find a statistically significant reduction in symptoms, but if the actual improvement is negligible, should we really be celebrating?
In research and data analysis, it’s essential to evaluate both statistical and practical significance. This ensures that our findings not only have a mathematical basis but also a meaningful impact on real-world applications. As you move forward in your work, remember to apply this understanding. Strike a balance between the numbers that make you look good and the implications that truly matter.
With this knowledge in your toolkit, you can improve the relevance and impact of your findings, making sure that your research isn’t just a statistical exercise but a valuable contribution to your field. Let’s make our findings count—beyond the p-value! tips for effective data analysis in economics and statistics
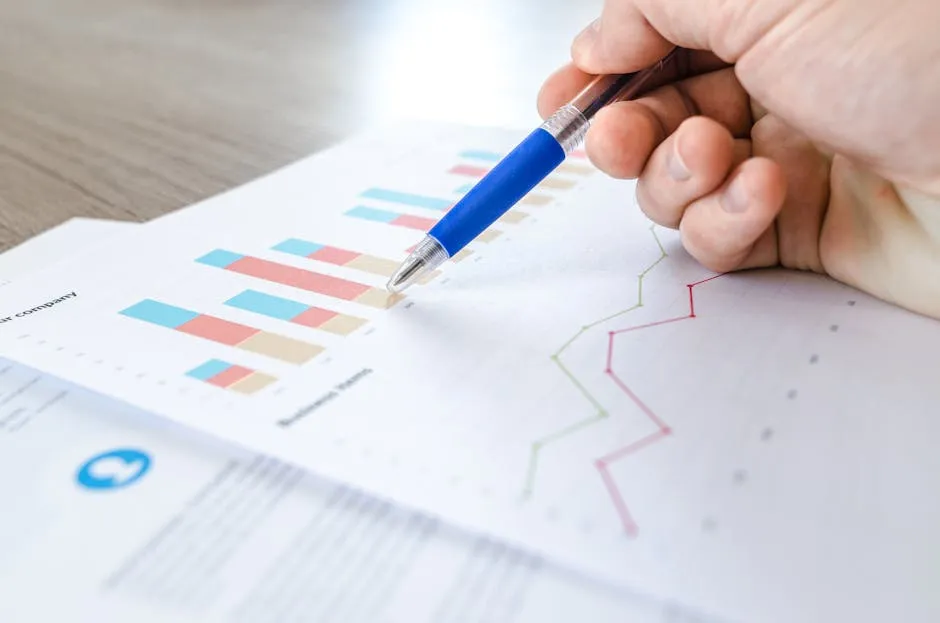
FAQs
Please let us know what you think about our content by leaving a comment down below!
Thank you for reading till here 🙂
Understanding statistical significance and practical significance is essential for informed decision-making in research. tips for effective data analysis in economics and statistics
All images from Pexels
Let’s say you’re in the medical field. Imagine a new drug that lowers blood pressure. A study shows a Cohen’s d of 0.25. Statistically significant? Yes! Practically significant? Not so much! A reduction that small might not move the needle for most patients.
If you’re curious about practical significance in various fields, I recommend “Data Science for Business: What You Need to Know About Data Mining and Data-Analytic Thinking”. This book is perfect for understanding how to apply data analysis in real-world scenarios.
In education, effect sizes can also be revealing. Picture a new teaching method that raises test scores with a Cohen’s d of 0.1. Statistically significant? Sure! But are we really going to overhaul the curriculum for that? Probably not!
In social sciences, odds ratios come into play. They help us understand the odds of an event occurring in one group compared to another. If a new policy reduces crime rates, the odds ratio might show a significant change. But how meaningful is that change in the context of community safety? This is where practical significance shines—it’s all about asking, “Is this change worth celebrating?”

Comparing Statistical and Practical Significance
Let’s illustrate the difference between statistical and practical significance with a medical study. Suppose researchers find that a new medication reduces the risk of heart disease by 30%, with a p-value of 0.03. Statistically significant? Absolutely! But wait! The actual risk reduction translates to a mere 0.05% in real terms. Practically significant? Not a chance!
In contrast, a different study might find a new exercise regimen that only shows a 15% reduction in heart disease risk, but it translates to a real-world effect of 3%. Here, both statistical significance and practical significance align, providing valuable insights for public health recommendations.
For those looking to dive deeper into understanding significance in research, you might want to check out “The Data Science Handbook”. This comprehensive guide will enhance your understanding of data analysis in various contexts.
Both types of significance have their place. Statistical significance tells us if an effect exists, while practical significance helps us gauge its real-world impact. Using both together leads to a more nuanced interpretation of research findings.
In the end, it’s about balancing the numbers and understanding their implications. So, the next time you see a shiny p-value, don’t forget to ask if it really matters in the grand scheme of things!

Examples of CIs in Research
Confidence intervals (CIs) provide a window into the reliability of study findings. They are crucial in understanding both statistical and practical significance. In one study on a new medication for high blood pressure, researchers found a statistically significant reduction in readings. The 95% CI ranged from 2 to 5 mmHg. This range tells us that if we repeated the study, we can be 95% confident that the true effect lies within those bounds.
Now, let’s consider another study examining a different treatment. It reported a statistically significant reduction in symptoms, but the CI spanned from 0.1 to 1 mmHg. While the effect is statistically significant, the lower end of the CI suggests that the actual effect could be negligible in real-world terms. Thus, the first study’s findings are not just statistically significant; they also indicate practical relevance.
If you’re eager to learn more about data visualization, consider picking up “Data Visualization: A Practical Introduction”. This book is perfect for anyone wanting to communicate data insights effectively.
When interpreting CIs, context is key. For instance, a CI that includes zero suggests a lack of practical significance, even if the results are statistically significant. Researchers must consider whether the entire CI reflects an effect that is meaningful in practice.
Best Practices for Researchers
Designing studies that account for both statistical and practical significance can be a game changer. Begin by defining a meaningful effect size before you even collect data. This helps ensure that your study is designed to detect effects that matter, rather than just those that are statistically significant.
Next, consider your sample size carefully. Large samples can lead to statistically significant results for trivial effects. A small, meaningful effect might require a larger sample to detect, so plan accordingly. This upfront thinking can save you from the heartbreak of statistical significance that lacks practical relevance.
For those interested in business research methods, I recommend “Business Research Methods”. It provides a solid foundation for conducting research that truly matters.
Reporting results is another area where clarity is paramount. Researchers should communicate findings with emphasis on both statistical and practical significance. For example, instead of simply stating a p-value, include effect sizes and CIs in discussions. This provides readers with a fuller picture of the findings’ implications.
Use visual aids like graphs to present results. They can effectively illustrate the differences between statistical and practical significance. For instance, a bar graph with error bars can show the CI, helping your audience visualize the reliability of the results.
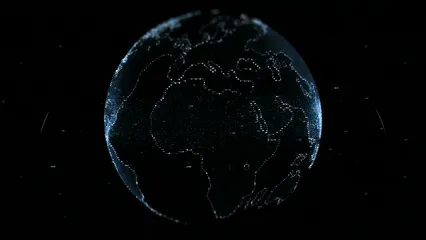
To further enhance your understanding of data presentation, consider “Graphs and Charts for Presenting Data”. This book is an excellent resource for anyone looking to improve their data visualization skills.
Lastly, engage in discussions about your findings. Sometimes, a statistically significant result may not resonate with practitioners in the field. By opening channels of communication, you can better align your research with real-world applications and ensure that your findings have a meaningful impact.
In summary, accounting for both statistical and practical significance in research design and reporting is essential. Clear definitions, proper sample sizes, and effective communication will pave the way for research that truly informs practice and decision-making.

Conclusion
In this article, we’ve unraveled the complex dance between statistical significance and practical significance. On one hand, we’ve got statistical significance, that flashy p-value which shouts, “Look at me! I’m statistically significant!” But hold your applause! Just because something is statistically significant doesn’t mean it matters in the real world. Think of it as the glittering trophy you win for a game where the score doesn’t count.
On the other hand, practical significance steps in like a wise old sage, asking, “Is this finding meaningful in our everyday lives?” It emphasizes the importance of the effect size and its implications. A statistically significant result with a minuscule effect size might lead to decisions that are more about numbers than about making a difference.
If you want to explore the broader context of data science, consider “The Data Science Bible”. This comprehensive guide covers a wide range of topics in data science.
Recognizing the difference between these two concepts is crucial for researchers and decision-makers alike. Misunderstanding can lead to misguided conclusions. For instance, a medical study might find a statistically significant reduction in symptoms, but if the actual improvement is negligible, should we really be celebrating?
In research and data analysis, it’s essential to evaluate both statistical and practical significance. This ensures that our findings not only have a mathematical basis but also a meaningful impact on real-world applications. As you move forward in your work, remember to apply this understanding. Strike a balance between the numbers that make you look good and the implications that truly matter.
With this knowledge in your toolkit, you can improve the relevance and impact of your findings, making sure that your research isn’t just a statistical exercise but a valuable contribution to your field. Let’s make our findings count—beyond the p-value! tips for effective data analysis in economics and statistics
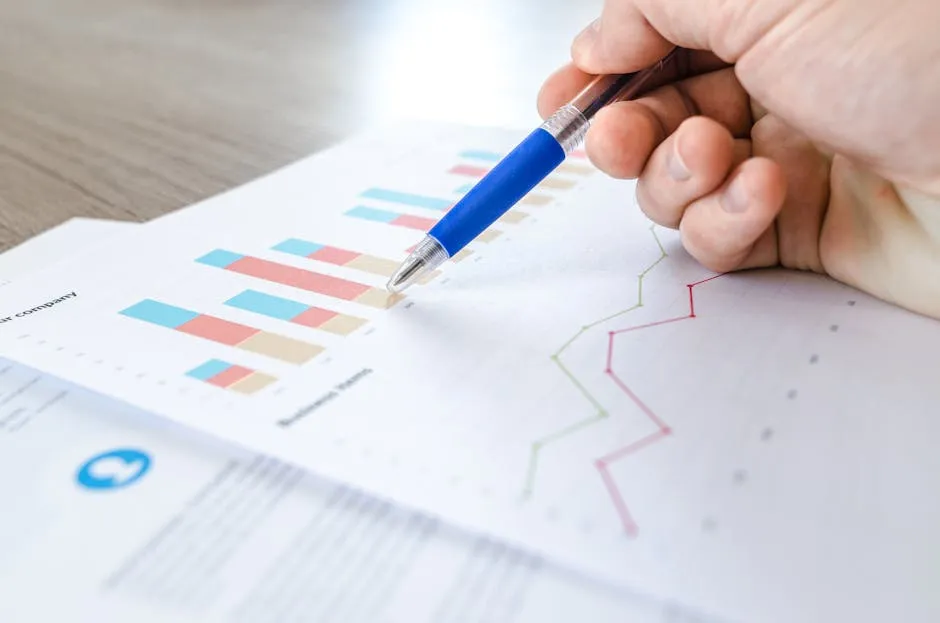
FAQs
Please let us know what you think about our content by leaving a comment down below!
Thank you for reading till here 🙂
Understanding statistical significance and practical significance is essential for informed decision-making in research. tips for effective data analysis in economics and statistics
All images from Pexels
For instance, suppose a study finds that a new treatment reduces symptoms of a condition with a p-value of 0.01. On the surface, that sounds impressive! But if the actual symptom reduction is a minuscule 0.1%, is that something to write home about? Not likely!
If you want to delve into more effective research practices, check out “The Craft of Research”. This book is a must-read for anyone looking to enhance their research skills.
Another example: a survey reveals a statistically significant difference in consumer preferences between two brands. If that difference is a mere 1%, is it worth changing marketing strategies? Probably not! These misinterpretations can result in wasted resources and misguided actions. It’s vital to scrutinize statistical findings closely and assess their practical implications.
Remember, just because something is statistically significant doesn’t mean it’s practically significant. It’s essential to evaluate both dimensions to draw meaningful conclusions from research findings.
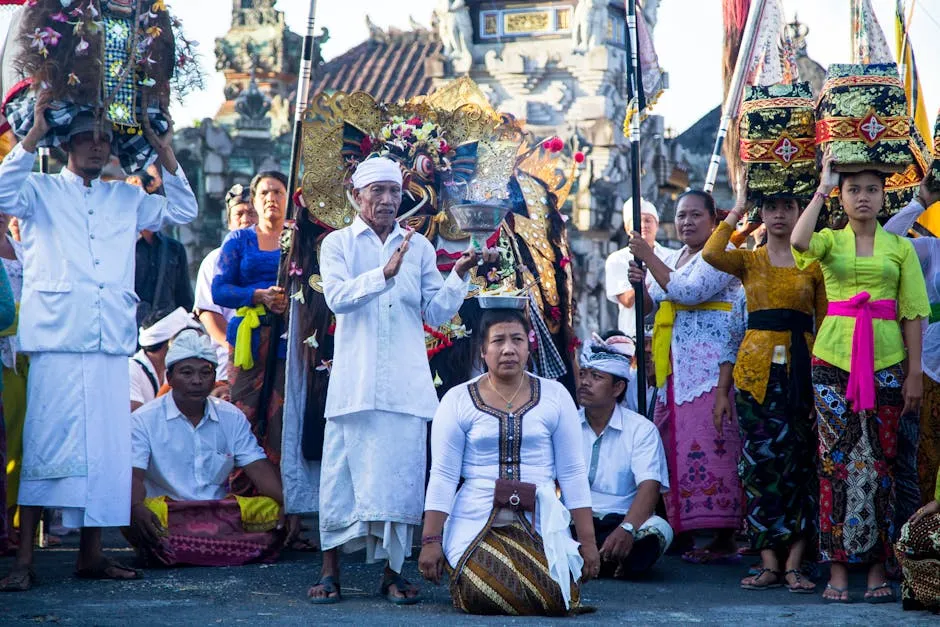
Assessing Practical Significance
When we talk about practical significance, we’re diving into the realm of “Does this actually matter?” Effect size measures help answer that question. One of the most popular measures is Cohen’s d, which quantifies the difference between two groups. A Cohen’s d of 0.2 is considered small, 0.5 medium, and anything above 0.8 is large—think of it as the “Goldilocks” of effect sizes: not too small, not too big, just right!
Let’s say you’re in the medical field. Imagine a new drug that lowers blood pressure. A study shows a Cohen’s d of 0.25. Statistically significant? Yes! Practically significant? Not so much! A reduction that small might not move the needle for most patients.
If you’re curious about practical significance in various fields, I recommend “Data Science for Business: What You Need to Know About Data Mining and Data-Analytic Thinking”. This book is perfect for understanding how to apply data analysis in real-world scenarios.
In education, effect sizes can also be revealing. Picture a new teaching method that raises test scores with a Cohen’s d of 0.1. Statistically significant? Sure! But are we really going to overhaul the curriculum for that? Probably not!
In social sciences, odds ratios come into play. They help us understand the odds of an event occurring in one group compared to another. If a new policy reduces crime rates, the odds ratio might show a significant change. But how meaningful is that change in the context of community safety? This is where practical significance shines—it’s all about asking, “Is this change worth celebrating?”

Comparing Statistical and Practical Significance
Let’s illustrate the difference between statistical and practical significance with a medical study. Suppose researchers find that a new medication reduces the risk of heart disease by 30%, with a p-value of 0.03. Statistically significant? Absolutely! But wait! The actual risk reduction translates to a mere 0.05% in real terms. Practically significant? Not a chance!
In contrast, a different study might find a new exercise regimen that only shows a 15% reduction in heart disease risk, but it translates to a real-world effect of 3%. Here, both statistical significance and practical significance align, providing valuable insights for public health recommendations.
For those looking to dive deeper into understanding significance in research, you might want to check out “The Data Science Handbook”. This comprehensive guide will enhance your understanding of data analysis in various contexts.
Both types of significance have their place. Statistical significance tells us if an effect exists, while practical significance helps us gauge its real-world impact. Using both together leads to a more nuanced interpretation of research findings.
In the end, it’s about balancing the numbers and understanding their implications. So, the next time you see a shiny p-value, don’t forget to ask if it really matters in the grand scheme of things!

Examples of CIs in Research
Confidence intervals (CIs) provide a window into the reliability of study findings. They are crucial in understanding both statistical and practical significance. In one study on a new medication for high blood pressure, researchers found a statistically significant reduction in readings. The 95% CI ranged from 2 to 5 mmHg. This range tells us that if we repeated the study, we can be 95% confident that the true effect lies within those bounds.
Now, let’s consider another study examining a different treatment. It reported a statistically significant reduction in symptoms, but the CI spanned from 0.1 to 1 mmHg. While the effect is statistically significant, the lower end of the CI suggests that the actual effect could be negligible in real-world terms. Thus, the first study’s findings are not just statistically significant; they also indicate practical relevance.
If you’re eager to learn more about data visualization, consider picking up “Data Visualization: A Practical Introduction”. This book is perfect for anyone wanting to communicate data insights effectively.
When interpreting CIs, context is key. For instance, a CI that includes zero suggests a lack of practical significance, even if the results are statistically significant. Researchers must consider whether the entire CI reflects an effect that is meaningful in practice.
Best Practices for Researchers
Designing studies that account for both statistical and practical significance can be a game changer. Begin by defining a meaningful effect size before you even collect data. This helps ensure that your study is designed to detect effects that matter, rather than just those that are statistically significant.
Next, consider your sample size carefully. Large samples can lead to statistically significant results for trivial effects. A small, meaningful effect might require a larger sample to detect, so plan accordingly. This upfront thinking can save you from the heartbreak of statistical significance that lacks practical relevance.
For those interested in business research methods, I recommend “Business Research Methods”. It provides a solid foundation for conducting research that truly matters.
Reporting results is another area where clarity is paramount. Researchers should communicate findings with emphasis on both statistical and practical significance. For example, instead of simply stating a p-value, include effect sizes and CIs in discussions. This provides readers with a fuller picture of the findings’ implications.
Use visual aids like graphs to present results. They can effectively illustrate the differences between statistical and practical significance. For instance, a bar graph with error bars can show the CI, helping your audience visualize the reliability of the results.
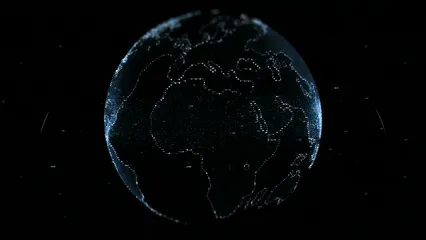
To further enhance your understanding of data presentation, consider “Graphs and Charts for Presenting Data”. This book is an excellent resource for anyone looking to improve their data visualization skills.
Lastly, engage in discussions about your findings. Sometimes, a statistically significant result may not resonate with practitioners in the field. By opening channels of communication, you can better align your research with real-world applications and ensure that your findings have a meaningful impact.
In summary, accounting for both statistical and practical significance in research design and reporting is essential. Clear definitions, proper sample sizes, and effective communication will pave the way for research that truly informs practice and decision-making.

Conclusion
In this article, we’ve unraveled the complex dance between statistical significance and practical significance. On one hand, we’ve got statistical significance, that flashy p-value which shouts, “Look at me! I’m statistically significant!” But hold your applause! Just because something is statistically significant doesn’t mean it matters in the real world. Think of it as the glittering trophy you win for a game where the score doesn’t count.
On the other hand, practical significance steps in like a wise old sage, asking, “Is this finding meaningful in our everyday lives?” It emphasizes the importance of the effect size and its implications. A statistically significant result with a minuscule effect size might lead to decisions that are more about numbers than about making a difference.
If you want to explore the broader context of data science, consider “The Data Science Bible”. This comprehensive guide covers a wide range of topics in data science.
Recognizing the difference between these two concepts is crucial for researchers and decision-makers alike. Misunderstanding can lead to misguided conclusions. For instance, a medical study might find a statistically significant reduction in symptoms, but if the actual improvement is negligible, should we really be celebrating?
In research and data analysis, it’s essential to evaluate both statistical and practical significance. This ensures that our findings not only have a mathematical basis but also a meaningful impact on real-world applications. As you move forward in your work, remember to apply this understanding. Strike a balance between the numbers that make you look good and the implications that truly matter.
With this knowledge in your toolkit, you can improve the relevance and impact of your findings, making sure that your research isn’t just a statistical exercise but a valuable contribution to your field. Let’s make our findings count—beyond the p-value! tips for effective data analysis in economics and statistics
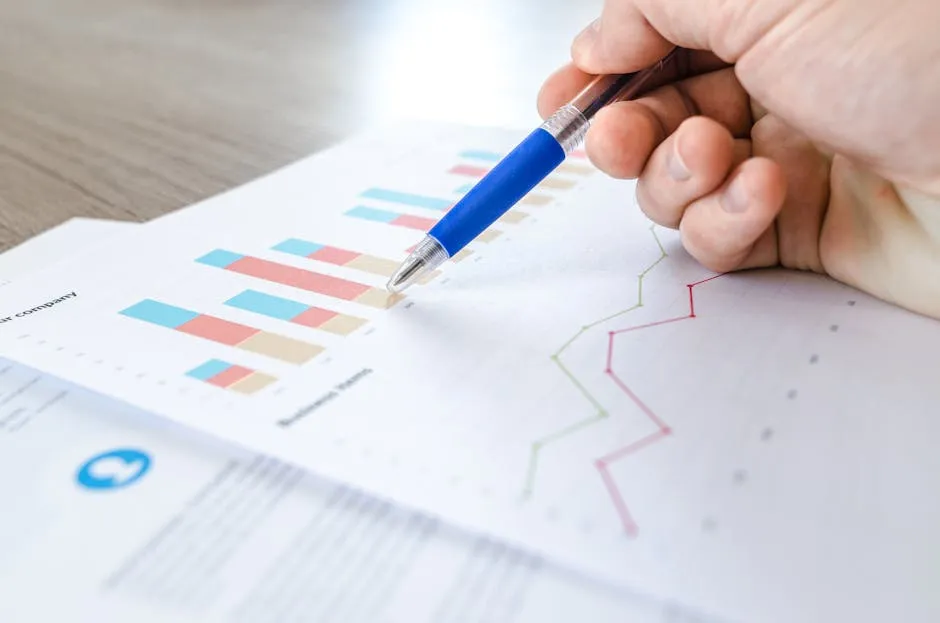
FAQs
Please let us know what you think about our content by leaving a comment down below!
Thank you for reading till here 🙂
Understanding statistical significance and practical significance is essential for informed decision-making in research. tips for effective data analysis in economics and statistics
All images from Pexels
In statistical terms, effect size quantifies the magnitude of the difference between groups. A tiny effect size can still yield a statistically significant p-value if the sample size is large enough. For example, if a new teaching method improves test scores by just one point in a massive study, it might be statistically significant. But does that one-point increase matter? Probably not! Understanding effect size is essential for grasping whether a statistically significant finding has any practical significance.
For a comprehensive understanding of effect size, consider “The Art of Statistics: Learning from Data”. This book dives deep into both statistical concepts and their implications.

Misinterpretations of Statistical Significance
Statistical significance can be misleading. Many researchers mistakenly believe that if a result is statistically significant, it must also be practically important. This assumption can lead to confusion and poor decision-making.
For instance, suppose a study finds that a new treatment reduces symptoms of a condition with a p-value of 0.01. On the surface, that sounds impressive! But if the actual symptom reduction is a minuscule 0.1%, is that something to write home about? Not likely!
If you want to delve into more effective research practices, check out “The Craft of Research”. This book is a must-read for anyone looking to enhance their research skills.
Another example: a survey reveals a statistically significant difference in consumer preferences between two brands. If that difference is a mere 1%, is it worth changing marketing strategies? Probably not! These misinterpretations can result in wasted resources and misguided actions. It’s vital to scrutinize statistical findings closely and assess their practical implications.
Remember, just because something is statistically significant doesn’t mean it’s practically significant. It’s essential to evaluate both dimensions to draw meaningful conclusions from research findings.
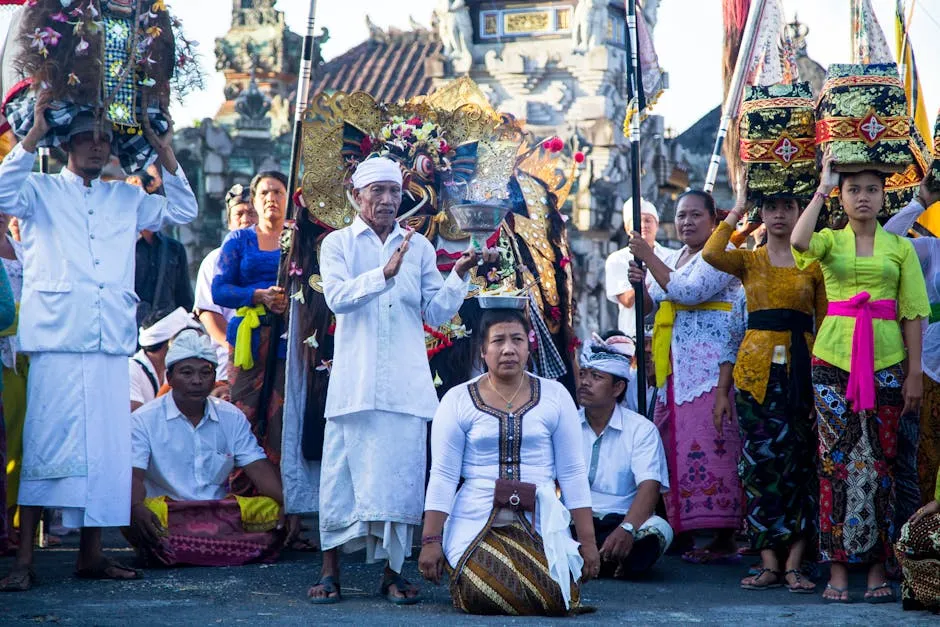
Assessing Practical Significance
When we talk about practical significance, we’re diving into the realm of “Does this actually matter?” Effect size measures help answer that question. One of the most popular measures is Cohen’s d, which quantifies the difference between two groups. A Cohen’s d of 0.2 is considered small, 0.5 medium, and anything above 0.8 is large—think of it as the “Goldilocks” of effect sizes: not too small, not too big, just right!
Let’s say you’re in the medical field. Imagine a new drug that lowers blood pressure. A study shows a Cohen’s d of 0.25. Statistically significant? Yes! Practically significant? Not so much! A reduction that small might not move the needle for most patients.
If you’re curious about practical significance in various fields, I recommend “Data Science for Business: What You Need to Know About Data Mining and Data-Analytic Thinking”. This book is perfect for understanding how to apply data analysis in real-world scenarios.
In education, effect sizes can also be revealing. Picture a new teaching method that raises test scores with a Cohen’s d of 0.1. Statistically significant? Sure! But are we really going to overhaul the curriculum for that? Probably not!
In social sciences, odds ratios come into play. They help us understand the odds of an event occurring in one group compared to another. If a new policy reduces crime rates, the odds ratio might show a significant change. But how meaningful is that change in the context of community safety? This is where practical significance shines—it’s all about asking, “Is this change worth celebrating?”

Comparing Statistical and Practical Significance
Let’s illustrate the difference between statistical and practical significance with a medical study. Suppose researchers find that a new medication reduces the risk of heart disease by 30%, with a p-value of 0.03. Statistically significant? Absolutely! But wait! The actual risk reduction translates to a mere 0.05% in real terms. Practically significant? Not a chance!
In contrast, a different study might find a new exercise regimen that only shows a 15% reduction in heart disease risk, but it translates to a real-world effect of 3%. Here, both statistical significance and practical significance align, providing valuable insights for public health recommendations.
For those looking to dive deeper into understanding significance in research, you might want to check out “The Data Science Handbook”. This comprehensive guide will enhance your understanding of data analysis in various contexts.
Both types of significance have their place. Statistical significance tells us if an effect exists, while practical significance helps us gauge its real-world impact. Using both together leads to a more nuanced interpretation of research findings.
In the end, it’s about balancing the numbers and understanding their implications. So, the next time you see a shiny p-value, don’t forget to ask if it really matters in the grand scheme of things!

Examples of CIs in Research
Confidence intervals (CIs) provide a window into the reliability of study findings. They are crucial in understanding both statistical and practical significance. In one study on a new medication for high blood pressure, researchers found a statistically significant reduction in readings. The 95% CI ranged from 2 to 5 mmHg. This range tells us that if we repeated the study, we can be 95% confident that the true effect lies within those bounds.
Now, let’s consider another study examining a different treatment. It reported a statistically significant reduction in symptoms, but the CI spanned from 0.1 to 1 mmHg. While the effect is statistically significant, the lower end of the CI suggests that the actual effect could be negligible in real-world terms. Thus, the first study’s findings are not just statistically significant; they also indicate practical relevance.
If you’re eager to learn more about data visualization, consider picking up “Data Visualization: A Practical Introduction”. This book is perfect for anyone wanting to communicate data insights effectively.
When interpreting CIs, context is key. For instance, a CI that includes zero suggests a lack of practical significance, even if the results are statistically significant. Researchers must consider whether the entire CI reflects an effect that is meaningful in practice.
Best Practices for Researchers
Designing studies that account for both statistical and practical significance can be a game changer. Begin by defining a meaningful effect size before you even collect data. This helps ensure that your study is designed to detect effects that matter, rather than just those that are statistically significant.
Next, consider your sample size carefully. Large samples can lead to statistically significant results for trivial effects. A small, meaningful effect might require a larger sample to detect, so plan accordingly. This upfront thinking can save you from the heartbreak of statistical significance that lacks practical relevance.
For those interested in business research methods, I recommend “Business Research Methods”. It provides a solid foundation for conducting research that truly matters.
Reporting results is another area where clarity is paramount. Researchers should communicate findings with emphasis on both statistical and practical significance. For example, instead of simply stating a p-value, include effect sizes and CIs in discussions. This provides readers with a fuller picture of the findings’ implications.
Use visual aids like graphs to present results. They can effectively illustrate the differences between statistical and practical significance. For instance, a bar graph with error bars can show the CI, helping your audience visualize the reliability of the results.
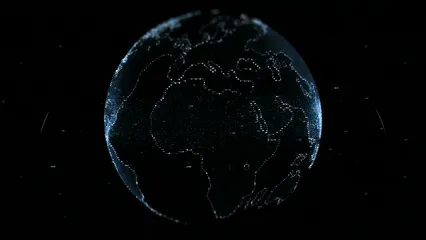
To further enhance your understanding of data presentation, consider “Graphs and Charts for Presenting Data”. This book is an excellent resource for anyone looking to improve their data visualization skills.
Lastly, engage in discussions about your findings. Sometimes, a statistically significant result may not resonate with practitioners in the field. By opening channels of communication, you can better align your research with real-world applications and ensure that your findings have a meaningful impact.
In summary, accounting for both statistical and practical significance in research design and reporting is essential. Clear definitions, proper sample sizes, and effective communication will pave the way for research that truly informs practice and decision-making.

Conclusion
In this article, we’ve unraveled the complex dance between statistical significance and practical significance. On one hand, we’ve got statistical significance, that flashy p-value which shouts, “Look at me! I’m statistically significant!” But hold your applause! Just because something is statistically significant doesn’t mean it matters in the real world. Think of it as the glittering trophy you win for a game where the score doesn’t count.
On the other hand, practical significance steps in like a wise old sage, asking, “Is this finding meaningful in our everyday lives?” It emphasizes the importance of the effect size and its implications. A statistically significant result with a minuscule effect size might lead to decisions that are more about numbers than about making a difference.
If you want to explore the broader context of data science, consider “The Data Science Bible”. This comprehensive guide covers a wide range of topics in data science.
Recognizing the difference between these two concepts is crucial for researchers and decision-makers alike. Misunderstanding can lead to misguided conclusions. For instance, a medical study might find a statistically significant reduction in symptoms, but if the actual improvement is negligible, should we really be celebrating?
In research and data analysis, it’s essential to evaluate both statistical and practical significance. This ensures that our findings not only have a mathematical basis but also a meaningful impact on real-world applications. As you move forward in your work, remember to apply this understanding. Strike a balance between the numbers that make you look good and the implications that truly matter.
With this knowledge in your toolkit, you can improve the relevance and impact of your findings, making sure that your research isn’t just a statistical exercise but a valuable contribution to your field. Let’s make our findings count—beyond the p-value! tips for effective data analysis in economics and statistics
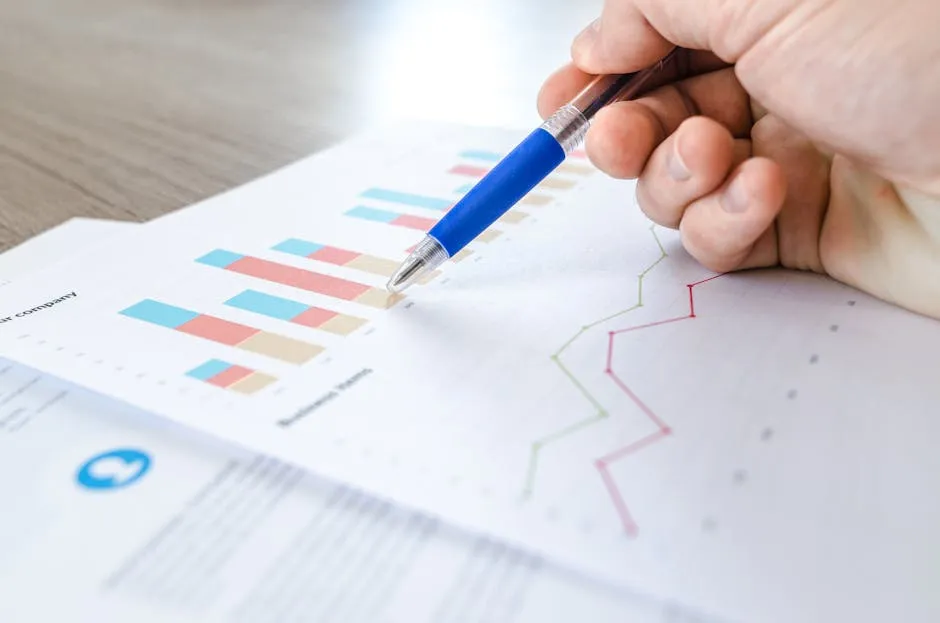
FAQs
Please let us know what you think about our content by leaving a comment down below!
Thank you for reading till here 🙂
Understanding statistical significance and practical significance is essential for informed decision-making in research. tips for effective data analysis in economics and statistics
All images from Pexels
Why? Larger samples increase the test’s power, allowing it to detect even the tiniest effects. This can lead to the proverbial “statistical significance” being bestowed upon findings that lack real-world relevance. Essentially, the bigger the sample, the more likely you are to find something significant—even if it’s not worth celebrating.
If you want to understand sample size better, grab a copy of “Research Methods: A Practical Guide”. It’s a fantastic resource for anyone looking to design studies effectively.

Effect Size
Effect size is another crucial factor in statistical significance. Unlike sample size, which simply reflects how many people you studied, effect size tells you how substantial the effect really is. It’s the difference between a blip on the radar and a full-blown meteor shower.
In statistical terms, effect size quantifies the magnitude of the difference between groups. A tiny effect size can still yield a statistically significant p-value if the sample size is large enough. For example, if a new teaching method improves test scores by just one point in a massive study, it might be statistically significant. But does that one-point increase matter? Probably not! Understanding effect size is essential for grasping whether a statistically significant finding has any practical significance.
For a comprehensive understanding of effect size, consider “The Art of Statistics: Learning from Data”. This book dives deep into both statistical concepts and their implications.

Misinterpretations of Statistical Significance
Statistical significance can be misleading. Many researchers mistakenly believe that if a result is statistically significant, it must also be practically important. This assumption can lead to confusion and poor decision-making.
For instance, suppose a study finds that a new treatment reduces symptoms of a condition with a p-value of 0.01. On the surface, that sounds impressive! But if the actual symptom reduction is a minuscule 0.1%, is that something to write home about? Not likely!
If you want to delve into more effective research practices, check out “The Craft of Research”. This book is a must-read for anyone looking to enhance their research skills.
Another example: a survey reveals a statistically significant difference in consumer preferences between two brands. If that difference is a mere 1%, is it worth changing marketing strategies? Probably not! These misinterpretations can result in wasted resources and misguided actions. It’s vital to scrutinize statistical findings closely and assess their practical implications.
Remember, just because something is statistically significant doesn’t mean it’s practically significant. It’s essential to evaluate both dimensions to draw meaningful conclusions from research findings.
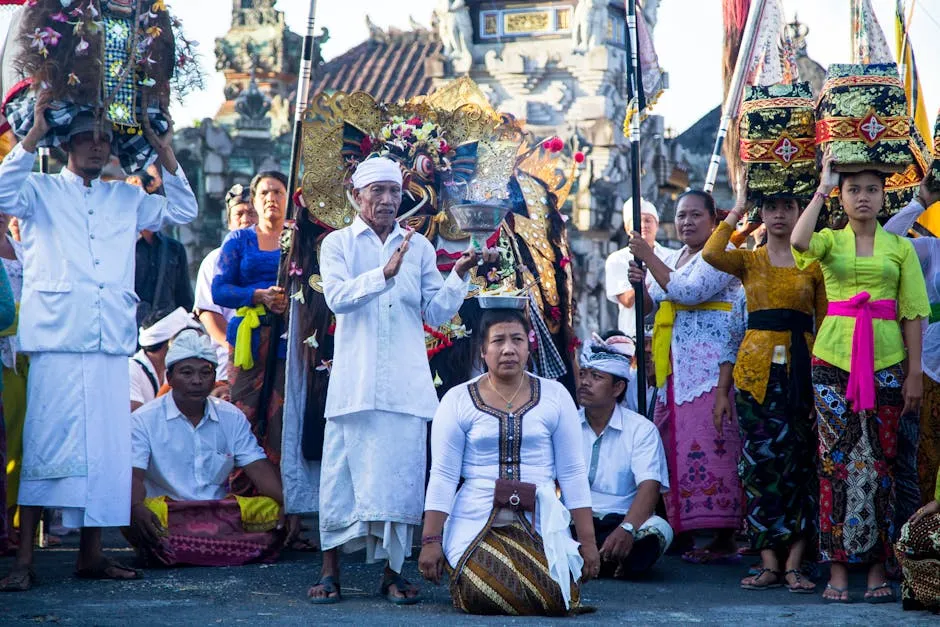
Assessing Practical Significance
When we talk about practical significance, we’re diving into the realm of “Does this actually matter?” Effect size measures help answer that question. One of the most popular measures is Cohen’s d, which quantifies the difference between two groups. A Cohen’s d of 0.2 is considered small, 0.5 medium, and anything above 0.8 is large—think of it as the “Goldilocks” of effect sizes: not too small, not too big, just right!
Let’s say you’re in the medical field. Imagine a new drug that lowers blood pressure. A study shows a Cohen’s d of 0.25. Statistically significant? Yes! Practically significant? Not so much! A reduction that small might not move the needle for most patients.
If you’re curious about practical significance in various fields, I recommend “Data Science for Business: What You Need to Know About Data Mining and Data-Analytic Thinking”. This book is perfect for understanding how to apply data analysis in real-world scenarios.
In education, effect sizes can also be revealing. Picture a new teaching method that raises test scores with a Cohen’s d of 0.1. Statistically significant? Sure! But are we really going to overhaul the curriculum for that? Probably not!
In social sciences, odds ratios come into play. They help us understand the odds of an event occurring in one group compared to another. If a new policy reduces crime rates, the odds ratio might show a significant change. But how meaningful is that change in the context of community safety? This is where practical significance shines—it’s all about asking, “Is this change worth celebrating?”

Comparing Statistical and Practical Significance
Let’s illustrate the difference between statistical and practical significance with a medical study. Suppose researchers find that a new medication reduces the risk of heart disease by 30%, with a p-value of 0.03. Statistically significant? Absolutely! But wait! The actual risk reduction translates to a mere 0.05% in real terms. Practically significant? Not a chance!
In contrast, a different study might find a new exercise regimen that only shows a 15% reduction in heart disease risk, but it translates to a real-world effect of 3%. Here, both statistical significance and practical significance align, providing valuable insights for public health recommendations.
For those looking to dive deeper into understanding significance in research, you might want to check out “The Data Science Handbook”. This comprehensive guide will enhance your understanding of data analysis in various contexts.
Both types of significance have their place. Statistical significance tells us if an effect exists, while practical significance helps us gauge its real-world impact. Using both together leads to a more nuanced interpretation of research findings.
In the end, it’s about balancing the numbers and understanding their implications. So, the next time you see a shiny p-value, don’t forget to ask if it really matters in the grand scheme of things!

Examples of CIs in Research
Confidence intervals (CIs) provide a window into the reliability of study findings. They are crucial in understanding both statistical and practical significance. In one study on a new medication for high blood pressure, researchers found a statistically significant reduction in readings. The 95% CI ranged from 2 to 5 mmHg. This range tells us that if we repeated the study, we can be 95% confident that the true effect lies within those bounds.
Now, let’s consider another study examining a different treatment. It reported a statistically significant reduction in symptoms, but the CI spanned from 0.1 to 1 mmHg. While the effect is statistically significant, the lower end of the CI suggests that the actual effect could be negligible in real-world terms. Thus, the first study’s findings are not just statistically significant; they also indicate practical relevance.
If you’re eager to learn more about data visualization, consider picking up “Data Visualization: A Practical Introduction”. This book is perfect for anyone wanting to communicate data insights effectively.
When interpreting CIs, context is key. For instance, a CI that includes zero suggests a lack of practical significance, even if the results are statistically significant. Researchers must consider whether the entire CI reflects an effect that is meaningful in practice.
Best Practices for Researchers
Designing studies that account for both statistical and practical significance can be a game changer. Begin by defining a meaningful effect size before you even collect data. This helps ensure that your study is designed to detect effects that matter, rather than just those that are statistically significant.
Next, consider your sample size carefully. Large samples can lead to statistically significant results for trivial effects. A small, meaningful effect might require a larger sample to detect, so plan accordingly. This upfront thinking can save you from the heartbreak of statistical significance that lacks practical relevance.
For those interested in business research methods, I recommend “Business Research Methods”. It provides a solid foundation for conducting research that truly matters.
Reporting results is another area where clarity is paramount. Researchers should communicate findings with emphasis on both statistical and practical significance. For example, instead of simply stating a p-value, include effect sizes and CIs in discussions. This provides readers with a fuller picture of the findings’ implications.
Use visual aids like graphs to present results. They can effectively illustrate the differences between statistical and practical significance. For instance, a bar graph with error bars can show the CI, helping your audience visualize the reliability of the results.
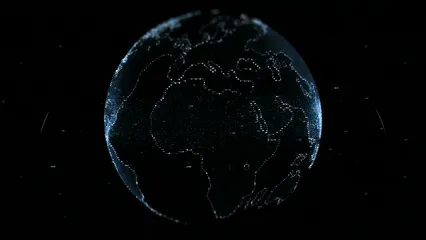
To further enhance your understanding of data presentation, consider “Graphs and Charts for Presenting Data”. This book is an excellent resource for anyone looking to improve their data visualization skills.
Lastly, engage in discussions about your findings. Sometimes, a statistically significant result may not resonate with practitioners in the field. By opening channels of communication, you can better align your research with real-world applications and ensure that your findings have a meaningful impact.
In summary, accounting for both statistical and practical significance in research design and reporting is essential. Clear definitions, proper sample sizes, and effective communication will pave the way for research that truly informs practice and decision-making.

Conclusion
In this article, we’ve unraveled the complex dance between statistical significance and practical significance. On one hand, we’ve got statistical significance, that flashy p-value which shouts, “Look at me! I’m statistically significant!” But hold your applause! Just because something is statistically significant doesn’t mean it matters in the real world. Think of it as the glittering trophy you win for a game where the score doesn’t count.
On the other hand, practical significance steps in like a wise old sage, asking, “Is this finding meaningful in our everyday lives?” It emphasizes the importance of the effect size and its implications. A statistically significant result with a minuscule effect size might lead to decisions that are more about numbers than about making a difference.
If you want to explore the broader context of data science, consider “The Data Science Bible”. This comprehensive guide covers a wide range of topics in data science.
Recognizing the difference between these two concepts is crucial for researchers and decision-makers alike. Misunderstanding can lead to misguided conclusions. For instance, a medical study might find a statistically significant reduction in symptoms, but if the actual improvement is negligible, should we really be celebrating?
In research and data analysis, it’s essential to evaluate both statistical and practical significance. This ensures that our findings not only have a mathematical basis but also a meaningful impact on real-world applications. As you move forward in your work, remember to apply this understanding. Strike a balance between the numbers that make you look good and the implications that truly matter.
With this knowledge in your toolkit, you can improve the relevance and impact of your findings, making sure that your research isn’t just a statistical exercise but a valuable contribution to your field. Let’s make our findings count—beyond the p-value! tips for effective data analysis in economics and statistics
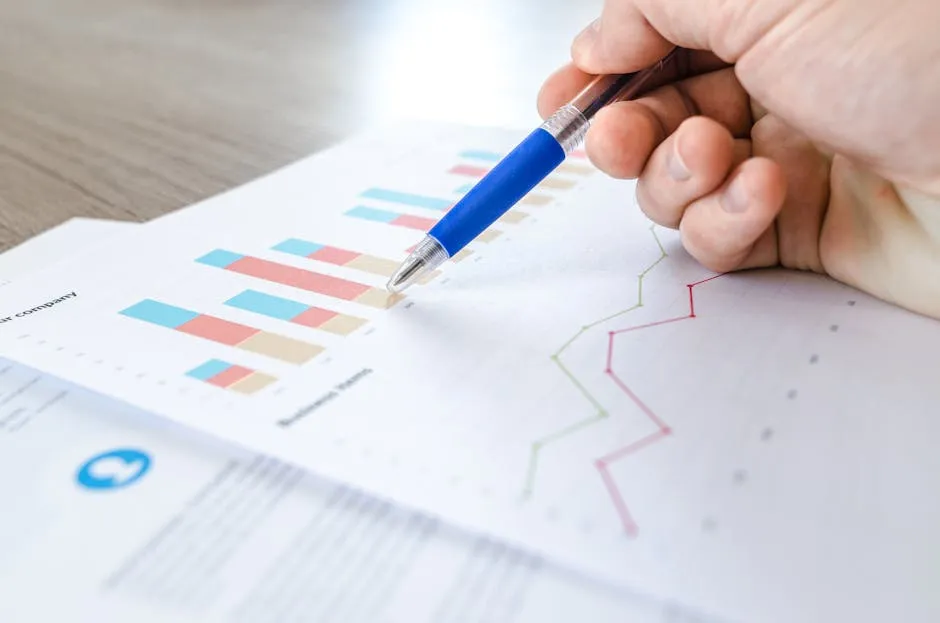
FAQs
Please let us know what you think about our content by leaving a comment down below!
Thank you for reading till here 🙂
Understanding statistical significance and practical significance is essential for informed decision-making in research. tips for effective data analysis in economics and statistics
All images from Pexels
Consider another example: a survey indicates a statistically significant difference in consumer preferences between two products. If the difference is only 1% in favor of one product, is that enough to justify changing marketing strategies? Maybe not!
For those keen on avoiding these pitfalls, “Practical Significance: Understanding the Real World Implications” could be a great read. This book helps bridge the gap between statistical findings and their real-world relevance.
Understanding these nuances is vital in research, as failing to recognize the difference can lead to misguided conclusions and ineffective decisions.
In conclusion, while statistical significance provides valuable insights, it should not be the sole criterion for assessing the relevance of research findings. It’s essential to look beyond the p-value and consider practical implications to make informed decisions.
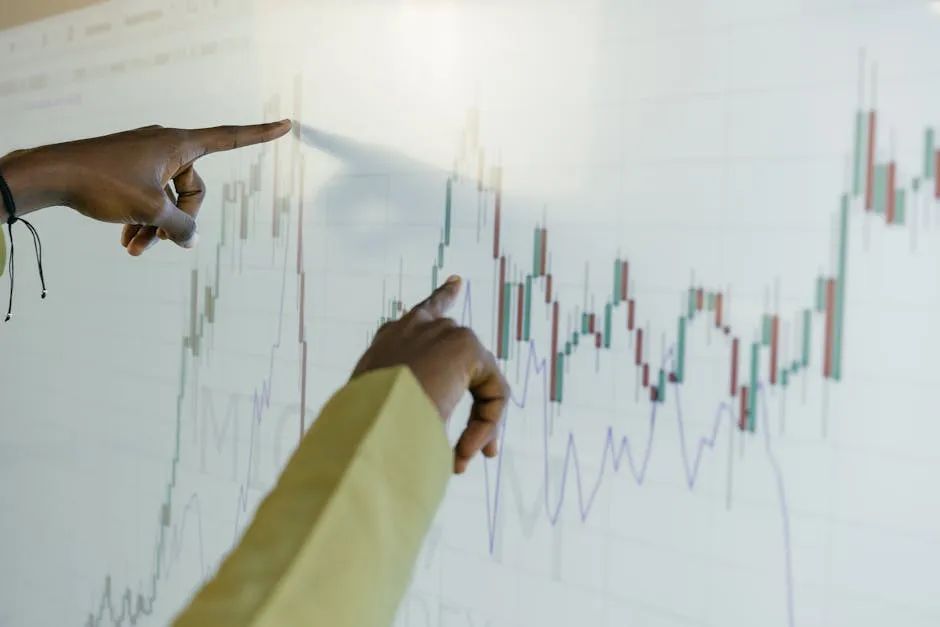
Factors Influencing Statistical Significance
Sample Size
Sample size plays a vital role in determining statistical significance. Picture this: you conduct a study with just ten participants. The chances are high that your results will be inconclusive. Now, crank that number up to a thousand. Voilà! You’re likely to find statistically significant results even for effects that are as trivial as finding a penny on the street.
Why? Larger samples increase the test’s power, allowing it to detect even the tiniest effects. This can lead to the proverbial “statistical significance” being bestowed upon findings that lack real-world relevance. Essentially, the bigger the sample, the more likely you are to find something significant—even if it’s not worth celebrating.
If you want to understand sample size better, grab a copy of “Research Methods: A Practical Guide”. It’s a fantastic resource for anyone looking to design studies effectively.

Effect Size
Effect size is another crucial factor in statistical significance. Unlike sample size, which simply reflects how many people you studied, effect size tells you how substantial the effect really is. It’s the difference between a blip on the radar and a full-blown meteor shower.
In statistical terms, effect size quantifies the magnitude of the difference between groups. A tiny effect size can still yield a statistically significant p-value if the sample size is large enough. For example, if a new teaching method improves test scores by just one point in a massive study, it might be statistically significant. But does that one-point increase matter? Probably not! Understanding effect size is essential for grasping whether a statistically significant finding has any practical significance.
For a comprehensive understanding of effect size, consider “The Art of Statistics: Learning from Data”. This book dives deep into both statistical concepts and their implications.

Misinterpretations of Statistical Significance
Statistical significance can be misleading. Many researchers mistakenly believe that if a result is statistically significant, it must also be practically important. This assumption can lead to confusion and poor decision-making.
For instance, suppose a study finds that a new treatment reduces symptoms of a condition with a p-value of 0.01. On the surface, that sounds impressive! But if the actual symptom reduction is a minuscule 0.1%, is that something to write home about? Not likely!
If you want to delve into more effective research practices, check out “The Craft of Research”. This book is a must-read for anyone looking to enhance their research skills.
Another example: a survey reveals a statistically significant difference in consumer preferences between two brands. If that difference is a mere 1%, is it worth changing marketing strategies? Probably not! These misinterpretations can result in wasted resources and misguided actions. It’s vital to scrutinize statistical findings closely and assess their practical implications.
Remember, just because something is statistically significant doesn’t mean it’s practically significant. It’s essential to evaluate both dimensions to draw meaningful conclusions from research findings.
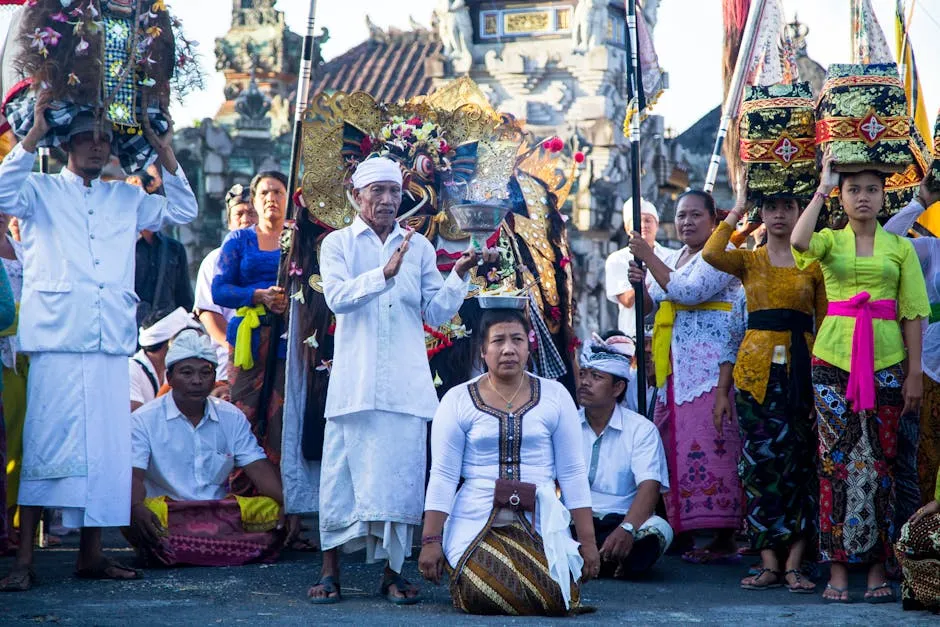
Assessing Practical Significance
When we talk about practical significance, we’re diving into the realm of “Does this actually matter?” Effect size measures help answer that question. One of the most popular measures is Cohen’s d, which quantifies the difference between two groups. A Cohen’s d of 0.2 is considered small, 0.5 medium, and anything above 0.8 is large—think of it as the “Goldilocks” of effect sizes: not too small, not too big, just right!
Let’s say you’re in the medical field. Imagine a new drug that lowers blood pressure. A study shows a Cohen’s d of 0.25. Statistically significant? Yes! Practically significant? Not so much! A reduction that small might not move the needle for most patients.
If you’re curious about practical significance in various fields, I recommend “Data Science for Business: What You Need to Know About Data Mining and Data-Analytic Thinking”. This book is perfect for understanding how to apply data analysis in real-world scenarios.
In education, effect sizes can also be revealing. Picture a new teaching method that raises test scores with a Cohen’s d of 0.1. Statistically significant? Sure! But are we really going to overhaul the curriculum for that? Probably not!
In social sciences, odds ratios come into play. They help us understand the odds of an event occurring in one group compared to another. If a new policy reduces crime rates, the odds ratio might show a significant change. But how meaningful is that change in the context of community safety? This is where practical significance shines—it’s all about asking, “Is this change worth celebrating?”

Comparing Statistical and Practical Significance
Let’s illustrate the difference between statistical and practical significance with a medical study. Suppose researchers find that a new medication reduces the risk of heart disease by 30%, with a p-value of 0.03. Statistically significant? Absolutely! But wait! The actual risk reduction translates to a mere 0.05% in real terms. Practically significant? Not a chance!
In contrast, a different study might find a new exercise regimen that only shows a 15% reduction in heart disease risk, but it translates to a real-world effect of 3%. Here, both statistical significance and practical significance align, providing valuable insights for public health recommendations.
For those looking to dive deeper into understanding significance in research, you might want to check out “The Data Science Handbook”. This comprehensive guide will enhance your understanding of data analysis in various contexts.
Both types of significance have their place. Statistical significance tells us if an effect exists, while practical significance helps us gauge its real-world impact. Using both together leads to a more nuanced interpretation of research findings.
In the end, it’s about balancing the numbers and understanding their implications. So, the next time you see a shiny p-value, don’t forget to ask if it really matters in the grand scheme of things!

Examples of CIs in Research
Confidence intervals (CIs) provide a window into the reliability of study findings. They are crucial in understanding both statistical and practical significance. In one study on a new medication for high blood pressure, researchers found a statistically significant reduction in readings. The 95% CI ranged from 2 to 5 mmHg. This range tells us that if we repeated the study, we can be 95% confident that the true effect lies within those bounds.
Now, let’s consider another study examining a different treatment. It reported a statistically significant reduction in symptoms, but the CI spanned from 0.1 to 1 mmHg. While the effect is statistically significant, the lower end of the CI suggests that the actual effect could be negligible in real-world terms. Thus, the first study’s findings are not just statistically significant; they also indicate practical relevance.
If you’re eager to learn more about data visualization, consider picking up “Data Visualization: A Practical Introduction”. This book is perfect for anyone wanting to communicate data insights effectively.
When interpreting CIs, context is key. For instance, a CI that includes zero suggests a lack of practical significance, even if the results are statistically significant. Researchers must consider whether the entire CI reflects an effect that is meaningful in practice.
Best Practices for Researchers
Designing studies that account for both statistical and practical significance can be a game changer. Begin by defining a meaningful effect size before you even collect data. This helps ensure that your study is designed to detect effects that matter, rather than just those that are statistically significant.
Next, consider your sample size carefully. Large samples can lead to statistically significant results for trivial effects. A small, meaningful effect might require a larger sample to detect, so plan accordingly. This upfront thinking can save you from the heartbreak of statistical significance that lacks practical relevance.
For those interested in business research methods, I recommend “Business Research Methods”. It provides a solid foundation for conducting research that truly matters.
Reporting results is another area where clarity is paramount. Researchers should communicate findings with emphasis on both statistical and practical significance. For example, instead of simply stating a p-value, include effect sizes and CIs in discussions. This provides readers with a fuller picture of the findings’ implications.
Use visual aids like graphs to present results. They can effectively illustrate the differences between statistical and practical significance. For instance, a bar graph with error bars can show the CI, helping your audience visualize the reliability of the results.
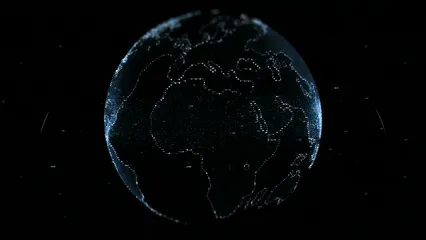
To further enhance your understanding of data presentation, consider “Graphs and Charts for Presenting Data”. This book is an excellent resource for anyone looking to improve their data visualization skills.
Lastly, engage in discussions about your findings. Sometimes, a statistically significant result may not resonate with practitioners in the field. By opening channels of communication, you can better align your research with real-world applications and ensure that your findings have a meaningful impact.
In summary, accounting for both statistical and practical significance in research design and reporting is essential. Clear definitions, proper sample sizes, and effective communication will pave the way for research that truly informs practice and decision-making.

Conclusion
In this article, we’ve unraveled the complex dance between statistical significance and practical significance. On one hand, we’ve got statistical significance, that flashy p-value which shouts, “Look at me! I’m statistically significant!” But hold your applause! Just because something is statistically significant doesn’t mean it matters in the real world. Think of it as the glittering trophy you win for a game where the score doesn’t count.
On the other hand, practical significance steps in like a wise old sage, asking, “Is this finding meaningful in our everyday lives?” It emphasizes the importance of the effect size and its implications. A statistically significant result with a minuscule effect size might lead to decisions that are more about numbers than about making a difference.
If you want to explore the broader context of data science, consider “The Data Science Bible”. This comprehensive guide covers a wide range of topics in data science.
Recognizing the difference between these two concepts is crucial for researchers and decision-makers alike. Misunderstanding can lead to misguided conclusions. For instance, a medical study might find a statistically significant reduction in symptoms, but if the actual improvement is negligible, should we really be celebrating?
In research and data analysis, it’s essential to evaluate both statistical and practical significance. This ensures that our findings not only have a mathematical basis but also a meaningful impact on real-world applications. As you move forward in your work, remember to apply this understanding. Strike a balance between the numbers that make you look good and the implications that truly matter.
With this knowledge in your toolkit, you can improve the relevance and impact of your findings, making sure that your research isn’t just a statistical exercise but a valuable contribution to your field. Let’s make our findings count—beyond the p-value! tips for effective data analysis in economics and statistics
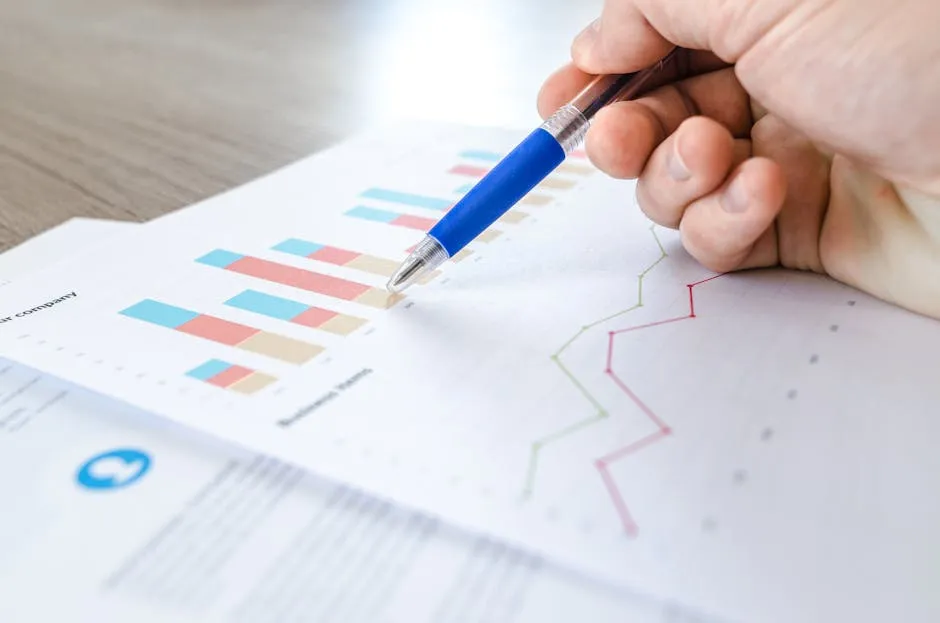
FAQs
Please let us know what you think about our content by leaving a comment down below!
Thank you for reading till here 🙂
Understanding statistical significance and practical significance is essential for informed decision-making in research. tips for effective data analysis in economics and statistics
All images from Pexels
Another factor is effect size. Effect size measures the magnitude of the difference between groups. A statistically significant result can occur with a small effect size, making it crucial to assess both metrics together. Understanding the relationship between statistical significance and effect size is key to interpreting research findings accurately.
If you’re interested in a deeper dive into effect sizes, consider reading “The Effect Size Primer”. This book offers practical insights into evaluating the impact of your findings.
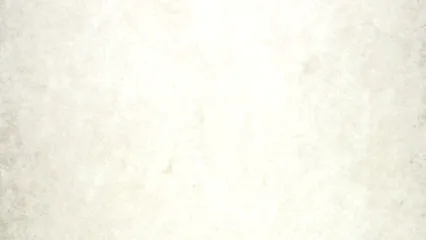
Misinterpretations of Statistical Significance
Misunderstandings about statistical significance abound. One common pitfall is the assumption that statistical significance implies practical importance. Let’s say a study finds a statistically significant effect of a new drug on heart disease risk. If the risk reduction is just 0.1%, healthcare providers might not consider this change substantial enough to alter treatment protocols.
Consider another example: a survey indicates a statistically significant difference in consumer preferences between two products. If the difference is only 1% in favor of one product, is that enough to justify changing marketing strategies? Maybe not!
For those keen on avoiding these pitfalls, “Practical Significance: Understanding the Real World Implications” could be a great read. This book helps bridge the gap between statistical findings and their real-world relevance.
Understanding these nuances is vital in research, as failing to recognize the difference can lead to misguided conclusions and ineffective decisions.
In conclusion, while statistical significance provides valuable insights, it should not be the sole criterion for assessing the relevance of research findings. It’s essential to look beyond the p-value and consider practical implications to make informed decisions.
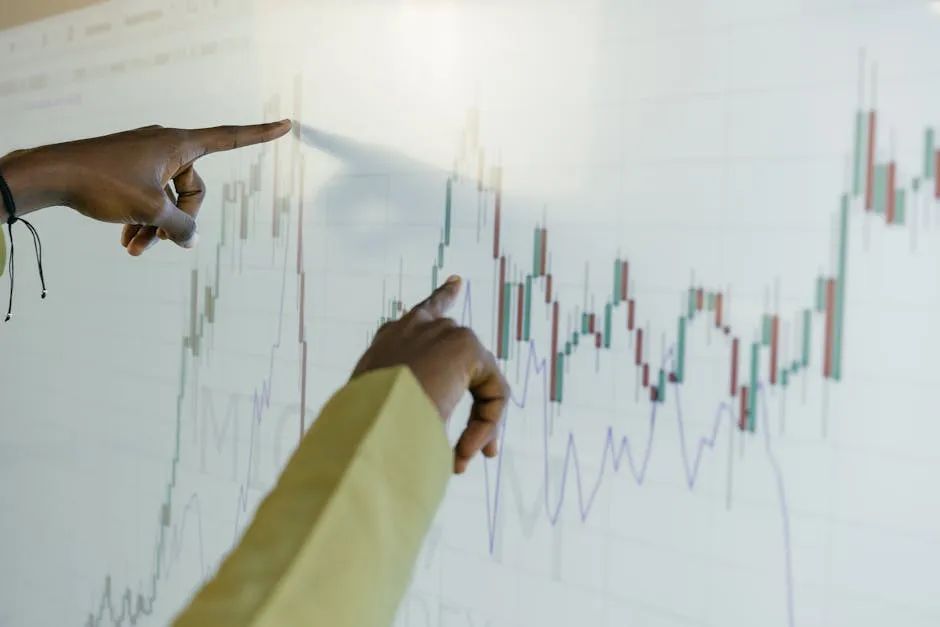
Factors Influencing Statistical Significance
Sample Size
Sample size plays a vital role in determining statistical significance. Picture this: you conduct a study with just ten participants. The chances are high that your results will be inconclusive. Now, crank that number up to a thousand. Voilà! You’re likely to find statistically significant results even for effects that are as trivial as finding a penny on the street.
Why? Larger samples increase the test’s power, allowing it to detect even the tiniest effects. This can lead to the proverbial “statistical significance” being bestowed upon findings that lack real-world relevance. Essentially, the bigger the sample, the more likely you are to find something significant—even if it’s not worth celebrating.
If you want to understand sample size better, grab a copy of “Research Methods: A Practical Guide”. It’s a fantastic resource for anyone looking to design studies effectively.

Effect Size
Effect size is another crucial factor in statistical significance. Unlike sample size, which simply reflects how many people you studied, effect size tells you how substantial the effect really is. It’s the difference between a blip on the radar and a full-blown meteor shower.
In statistical terms, effect size quantifies the magnitude of the difference between groups. A tiny effect size can still yield a statistically significant p-value if the sample size is large enough. For example, if a new teaching method improves test scores by just one point in a massive study, it might be statistically significant. But does that one-point increase matter? Probably not! Understanding effect size is essential for grasping whether a statistically significant finding has any practical significance.
For a comprehensive understanding of effect size, consider “The Art of Statistics: Learning from Data”. This book dives deep into both statistical concepts and their implications.

Misinterpretations of Statistical Significance
Statistical significance can be misleading. Many researchers mistakenly believe that if a result is statistically significant, it must also be practically important. This assumption can lead to confusion and poor decision-making.
For instance, suppose a study finds that a new treatment reduces symptoms of a condition with a p-value of 0.01. On the surface, that sounds impressive! But if the actual symptom reduction is a minuscule 0.1%, is that something to write home about? Not likely!
If you want to delve into more effective research practices, check out “The Craft of Research”. This book is a must-read for anyone looking to enhance their research skills.
Another example: a survey reveals a statistically significant difference in consumer preferences between two brands. If that difference is a mere 1%, is it worth changing marketing strategies? Probably not! These misinterpretations can result in wasted resources and misguided actions. It’s vital to scrutinize statistical findings closely and assess their practical implications.
Remember, just because something is statistically significant doesn’t mean it’s practically significant. It’s essential to evaluate both dimensions to draw meaningful conclusions from research findings.
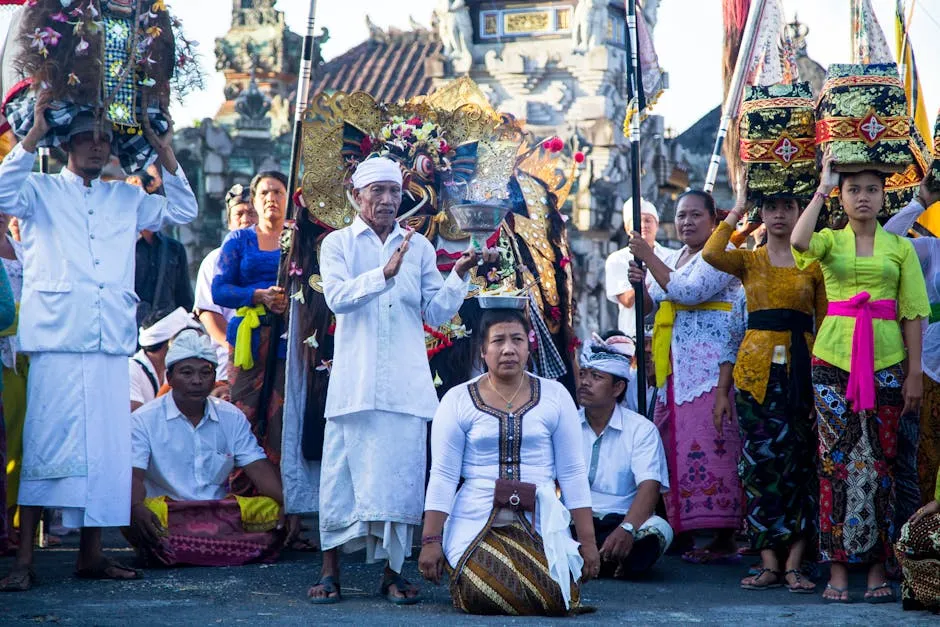
Assessing Practical Significance
When we talk about practical significance, we’re diving into the realm of “Does this actually matter?” Effect size measures help answer that question. One of the most popular measures is Cohen’s d, which quantifies the difference between two groups. A Cohen’s d of 0.2 is considered small, 0.5 medium, and anything above 0.8 is large—think of it as the “Goldilocks” of effect sizes: not too small, not too big, just right!
Let’s say you’re in the medical field. Imagine a new drug that lowers blood pressure. A study shows a Cohen’s d of 0.25. Statistically significant? Yes! Practically significant? Not so much! A reduction that small might not move the needle for most patients.
If you’re curious about practical significance in various fields, I recommend “Data Science for Business: What You Need to Know About Data Mining and Data-Analytic Thinking”. This book is perfect for understanding how to apply data analysis in real-world scenarios.
In education, effect sizes can also be revealing. Picture a new teaching method that raises test scores with a Cohen’s d of 0.1. Statistically significant? Sure! But are we really going to overhaul the curriculum for that? Probably not!
In social sciences, odds ratios come into play. They help us understand the odds of an event occurring in one group compared to another. If a new policy reduces crime rates, the odds ratio might show a significant change. But how meaningful is that change in the context of community safety? This is where practical significance shines—it’s all about asking, “Is this change worth celebrating?”

Comparing Statistical and Practical Significance
Let’s illustrate the difference between statistical and practical significance with a medical study. Suppose researchers find that a new medication reduces the risk of heart disease by 30%, with a p-value of 0.03. Statistically significant? Absolutely! But wait! The actual risk reduction translates to a mere 0.05% in real terms. Practically significant? Not a chance!
In contrast, a different study might find a new exercise regimen that only shows a 15% reduction in heart disease risk, but it translates to a real-world effect of 3%. Here, both statistical significance and practical significance align, providing valuable insights for public health recommendations.
For those looking to dive deeper into understanding significance in research, you might want to check out “The Data Science Handbook”. This comprehensive guide will enhance your understanding of data analysis in various contexts.
Both types of significance have their place. Statistical significance tells us if an effect exists, while practical significance helps us gauge its real-world impact. Using both together leads to a more nuanced interpretation of research findings.
In the end, it’s about balancing the numbers and understanding their implications. So, the next time you see a shiny p-value, don’t forget to ask if it really matters in the grand scheme of things!

Examples of CIs in Research
Confidence intervals (CIs) provide a window into the reliability of study findings. They are crucial in understanding both statistical and practical significance. In one study on a new medication for high blood pressure, researchers found a statistically significant reduction in readings. The 95% CI ranged from 2 to 5 mmHg. This range tells us that if we repeated the study, we can be 95% confident that the true effect lies within those bounds.
Now, let’s consider another study examining a different treatment. It reported a statistically significant reduction in symptoms, but the CI spanned from 0.1 to 1 mmHg. While the effect is statistically significant, the lower end of the CI suggests that the actual effect could be negligible in real-world terms. Thus, the first study’s findings are not just statistically significant; they also indicate practical relevance.
If you’re eager to learn more about data visualization, consider picking up “Data Visualization: A Practical Introduction”. This book is perfect for anyone wanting to communicate data insights effectively.
When interpreting CIs, context is key. For instance, a CI that includes zero suggests a lack of practical significance, even if the results are statistically significant. Researchers must consider whether the entire CI reflects an effect that is meaningful in practice.
Best Practices for Researchers
Designing studies that account for both statistical and practical significance can be a game changer. Begin by defining a meaningful effect size before you even collect data. This helps ensure that your study is designed to detect effects that matter, rather than just those that are statistically significant.
Next, consider your sample size carefully. Large samples can lead to statistically significant results for trivial effects. A small, meaningful effect might require a larger sample to detect, so plan accordingly. This upfront thinking can save you from the heartbreak of statistical significance that lacks practical relevance.
For those interested in business research methods, I recommend “Business Research Methods”. It provides a solid foundation for conducting research that truly matters.
Reporting results is another area where clarity is paramount. Researchers should communicate findings with emphasis on both statistical and practical significance. For example, instead of simply stating a p-value, include effect sizes and CIs in discussions. This provides readers with a fuller picture of the findings’ implications.
Use visual aids like graphs to present results. They can effectively illustrate the differences between statistical and practical significance. For instance, a bar graph with error bars can show the CI, helping your audience visualize the reliability of the results.
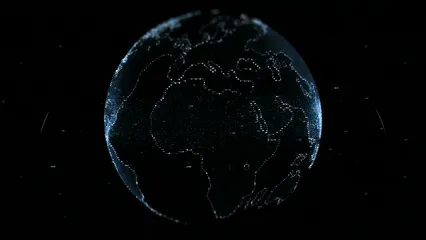
To further enhance your understanding of data presentation, consider “Graphs and Charts for Presenting Data”. This book is an excellent resource for anyone looking to improve their data visualization skills.
Lastly, engage in discussions about your findings. Sometimes, a statistically significant result may not resonate with practitioners in the field. By opening channels of communication, you can better align your research with real-world applications and ensure that your findings have a meaningful impact.
In summary, accounting for both statistical and practical significance in research design and reporting is essential. Clear definitions, proper sample sizes, and effective communication will pave the way for research that truly informs practice and decision-making.

Conclusion
In this article, we’ve unraveled the complex dance between statistical significance and practical significance. On one hand, we’ve got statistical significance, that flashy p-value which shouts, “Look at me! I’m statistically significant!” But hold your applause! Just because something is statistically significant doesn’t mean it matters in the real world. Think of it as the glittering trophy you win for a game where the score doesn’t count.
On the other hand, practical significance steps in like a wise old sage, asking, “Is this finding meaningful in our everyday lives?” It emphasizes the importance of the effect size and its implications. A statistically significant result with a minuscule effect size might lead to decisions that are more about numbers than about making a difference.
If you want to explore the broader context of data science, consider “The Data Science Bible”. This comprehensive guide covers a wide range of topics in data science.
Recognizing the difference between these two concepts is crucial for researchers and decision-makers alike. Misunderstanding can lead to misguided conclusions. For instance, a medical study might find a statistically significant reduction in symptoms, but if the actual improvement is negligible, should we really be celebrating?
In research and data analysis, it’s essential to evaluate both statistical and practical significance. This ensures that our findings not only have a mathematical basis but also a meaningful impact on real-world applications. As you move forward in your work, remember to apply this understanding. Strike a balance between the numbers that make you look good and the implications that truly matter.
With this knowledge in your toolkit, you can improve the relevance and impact of your findings, making sure that your research isn’t just a statistical exercise but a valuable contribution to your field. Let’s make our findings count—beyond the p-value! tips for effective data analysis in economics and statistics
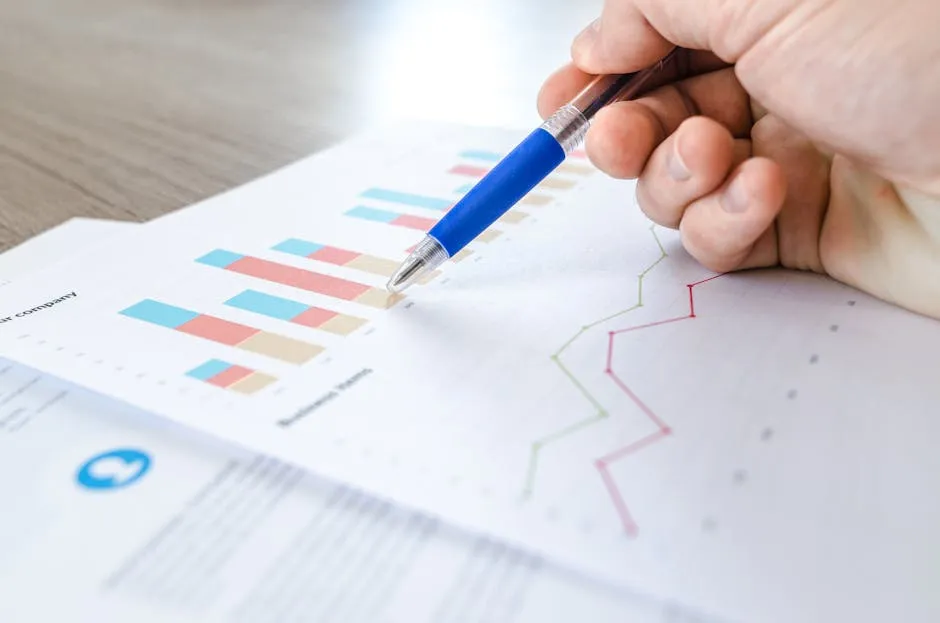
FAQs
Please let us know what you think about our content by leaving a comment down below!
Thank you for reading till here 🙂
Understanding statistical significance and practical significance is essential for informed decision-making in research. tips for effective data analysis in economics and statistics
All images from Pexels
Statistical significance is important because it provides a framework for researchers to evaluate hypotheses and make decisions based on data. It helps to quantify the evidence against the null hypothesis. However, it is essential to remember that statistical significance alone does not address whether the effect size is large enough to matter in practice.
If you’re looking to understand the ins and outs of statistical significance, you might want to check out “Statistical Significance: A Primer”. This book will guide you through the complexities and help you grasp the concepts that are crucial for effective research.
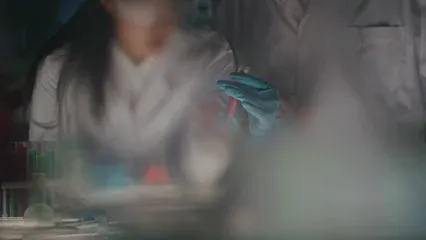
Factors Influencing Statistical Significance
Several factors can influence whether a result is deemed statistically significant. First, consider sample size. A larger sample can lead to statistically significant results, even for trivial effects. Imagine testing a new teaching method on a class of a thousand students. If the method produces a statistically significant improvement in test scores, the result might look impressive. Yet, if the actual increase is just a fraction of a point, it could hardly be considered meaningful.
Another factor is effect size. Effect size measures the magnitude of the difference between groups. A statistically significant result can occur with a small effect size, making it crucial to assess both metrics together. Understanding the relationship between statistical significance and effect size is key to interpreting research findings accurately.
If you’re interested in a deeper dive into effect sizes, consider reading “The Effect Size Primer”. This book offers practical insights into evaluating the impact of your findings.
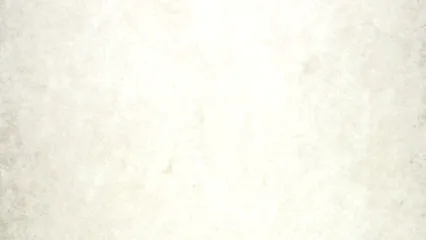
Misinterpretations of Statistical Significance
Misunderstandings about statistical significance abound. One common pitfall is the assumption that statistical significance implies practical importance. Let’s say a study finds a statistically significant effect of a new drug on heart disease risk. If the risk reduction is just 0.1%, healthcare providers might not consider this change substantial enough to alter treatment protocols.
Consider another example: a survey indicates a statistically significant difference in consumer preferences between two products. If the difference is only 1% in favor of one product, is that enough to justify changing marketing strategies? Maybe not!
For those keen on avoiding these pitfalls, “Practical Significance: Understanding the Real World Implications” could be a great read. This book helps bridge the gap between statistical findings and their real-world relevance.
Understanding these nuances is vital in research, as failing to recognize the difference can lead to misguided conclusions and ineffective decisions.
In conclusion, while statistical significance provides valuable insights, it should not be the sole criterion for assessing the relevance of research findings. It’s essential to look beyond the p-value and consider practical implications to make informed decisions.
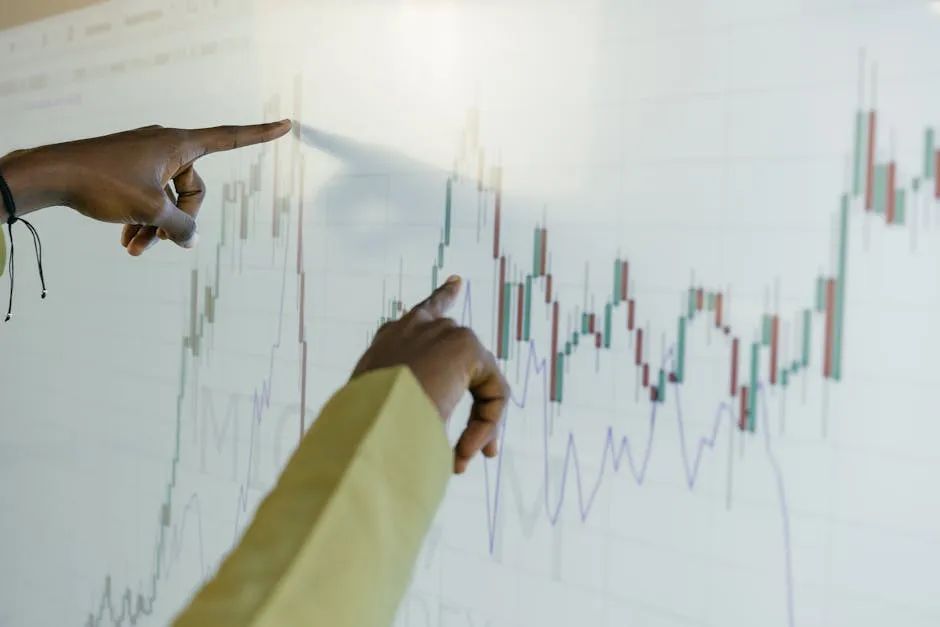
Factors Influencing Statistical Significance
Sample Size
Sample size plays a vital role in determining statistical significance. Picture this: you conduct a study with just ten participants. The chances are high that your results will be inconclusive. Now, crank that number up to a thousand. Voilà! You’re likely to find statistically significant results even for effects that are as trivial as finding a penny on the street.
Why? Larger samples increase the test’s power, allowing it to detect even the tiniest effects. This can lead to the proverbial “statistical significance” being bestowed upon findings that lack real-world relevance. Essentially, the bigger the sample, the more likely you are to find something significant—even if it’s not worth celebrating.
If you want to understand sample size better, grab a copy of “Research Methods: A Practical Guide”. It’s a fantastic resource for anyone looking to design studies effectively.

Effect Size
Effect size is another crucial factor in statistical significance. Unlike sample size, which simply reflects how many people you studied, effect size tells you how substantial the effect really is. It’s the difference between a blip on the radar and a full-blown meteor shower.
In statistical terms, effect size quantifies the magnitude of the difference between groups. A tiny effect size can still yield a statistically significant p-value if the sample size is large enough. For example, if a new teaching method improves test scores by just one point in a massive study, it might be statistically significant. But does that one-point increase matter? Probably not! Understanding effect size is essential for grasping whether a statistically significant finding has any practical significance.
For a comprehensive understanding of effect size, consider “The Art of Statistics: Learning from Data”. This book dives deep into both statistical concepts and their implications.

Misinterpretations of Statistical Significance
Statistical significance can be misleading. Many researchers mistakenly believe that if a result is statistically significant, it must also be practically important. This assumption can lead to confusion and poor decision-making.
For instance, suppose a study finds that a new treatment reduces symptoms of a condition with a p-value of 0.01. On the surface, that sounds impressive! But if the actual symptom reduction is a minuscule 0.1%, is that something to write home about? Not likely!
If you want to delve into more effective research practices, check out “The Craft of Research”. This book is a must-read for anyone looking to enhance their research skills.
Another example: a survey reveals a statistically significant difference in consumer preferences between two brands. If that difference is a mere 1%, is it worth changing marketing strategies? Probably not! These misinterpretations can result in wasted resources and misguided actions. It’s vital to scrutinize statistical findings closely and assess their practical implications.
Remember, just because something is statistically significant doesn’t mean it’s practically significant. It’s essential to evaluate both dimensions to draw meaningful conclusions from research findings.
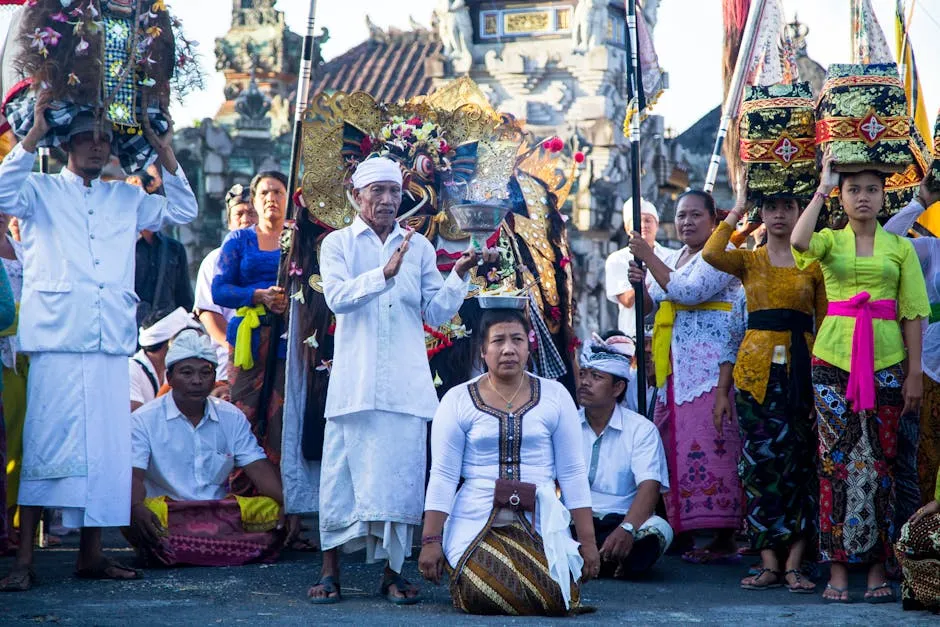
Assessing Practical Significance
When we talk about practical significance, we’re diving into the realm of “Does this actually matter?” Effect size measures help answer that question. One of the most popular measures is Cohen’s d, which quantifies the difference between two groups. A Cohen’s d of 0.2 is considered small, 0.5 medium, and anything above 0.8 is large—think of it as the “Goldilocks” of effect sizes: not too small, not too big, just right!
Let’s say you’re in the medical field. Imagine a new drug that lowers blood pressure. A study shows a Cohen’s d of 0.25. Statistically significant? Yes! Practically significant? Not so much! A reduction that small might not move the needle for most patients.
If you’re curious about practical significance in various fields, I recommend “Data Science for Business: What You Need to Know About Data Mining and Data-Analytic Thinking”. This book is perfect for understanding how to apply data analysis in real-world scenarios.
In education, effect sizes can also be revealing. Picture a new teaching method that raises test scores with a Cohen’s d of 0.1. Statistically significant? Sure! But are we really going to overhaul the curriculum for that? Probably not!
In social sciences, odds ratios come into play. They help us understand the odds of an event occurring in one group compared to another. If a new policy reduces crime rates, the odds ratio might show a significant change. But how meaningful is that change in the context of community safety? This is where practical significance shines—it’s all about asking, “Is this change worth celebrating?”

Comparing Statistical and Practical Significance
Let’s illustrate the difference between statistical and practical significance with a medical study. Suppose researchers find that a new medication reduces the risk of heart disease by 30%, with a p-value of 0.03. Statistically significant? Absolutely! But wait! The actual risk reduction translates to a mere 0.05% in real terms. Practically significant? Not a chance!
In contrast, a different study might find a new exercise regimen that only shows a 15% reduction in heart disease risk, but it translates to a real-world effect of 3%. Here, both statistical significance and practical significance align, providing valuable insights for public health recommendations.
For those looking to dive deeper into understanding significance in research, you might want to check out “The Data Science Handbook”. This comprehensive guide will enhance your understanding of data analysis in various contexts.
Both types of significance have their place. Statistical significance tells us if an effect exists, while practical significance helps us gauge its real-world impact. Using both together leads to a more nuanced interpretation of research findings.
In the end, it’s about balancing the numbers and understanding their implications. So, the next time you see a shiny p-value, don’t forget to ask if it really matters in the grand scheme of things!

Examples of CIs in Research
Confidence intervals (CIs) provide a window into the reliability of study findings. They are crucial in understanding both statistical and practical significance. In one study on a new medication for high blood pressure, researchers found a statistically significant reduction in readings. The 95% CI ranged from 2 to 5 mmHg. This range tells us that if we repeated the study, we can be 95% confident that the true effect lies within those bounds.
Now, let’s consider another study examining a different treatment. It reported a statistically significant reduction in symptoms, but the CI spanned from 0.1 to 1 mmHg. While the effect is statistically significant, the lower end of the CI suggests that the actual effect could be negligible in real-world terms. Thus, the first study’s findings are not just statistically significant; they also indicate practical relevance.
If you’re eager to learn more about data visualization, consider picking up “Data Visualization: A Practical Introduction”. This book is perfect for anyone wanting to communicate data insights effectively.
When interpreting CIs, context is key. For instance, a CI that includes zero suggests a lack of practical significance, even if the results are statistically significant. Researchers must consider whether the entire CI reflects an effect that is meaningful in practice.
Best Practices for Researchers
Designing studies that account for both statistical and practical significance can be a game changer. Begin by defining a meaningful effect size before you even collect data. This helps ensure that your study is designed to detect effects that matter, rather than just those that are statistically significant.
Next, consider your sample size carefully. Large samples can lead to statistically significant results for trivial effects. A small, meaningful effect might require a larger sample to detect, so plan accordingly. This upfront thinking can save you from the heartbreak of statistical significance that lacks practical relevance.
For those interested in business research methods, I recommend “Business Research Methods”. It provides a solid foundation for conducting research that truly matters.
Reporting results is another area where clarity is paramount. Researchers should communicate findings with emphasis on both statistical and practical significance. For example, instead of simply stating a p-value, include effect sizes and CIs in discussions. This provides readers with a fuller picture of the findings’ implications.
Use visual aids like graphs to present results. They can effectively illustrate the differences between statistical and practical significance. For instance, a bar graph with error bars can show the CI, helping your audience visualize the reliability of the results.
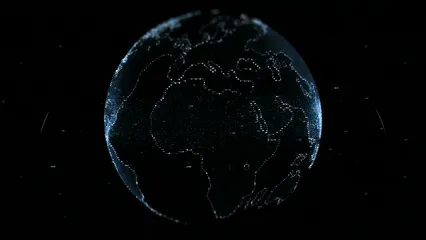
To further enhance your understanding of data presentation, consider “Graphs and Charts for Presenting Data”. This book is an excellent resource for anyone looking to improve their data visualization skills.
Lastly, engage in discussions about your findings. Sometimes, a statistically significant result may not resonate with practitioners in the field. By opening channels of communication, you can better align your research with real-world applications and ensure that your findings have a meaningful impact.
In summary, accounting for both statistical and practical significance in research design and reporting is essential. Clear definitions, proper sample sizes, and effective communication will pave the way for research that truly informs practice and decision-making.

Conclusion
In this article, we’ve unraveled the complex dance between statistical significance and practical significance. On one hand, we’ve got statistical significance, that flashy p-value which shouts, “Look at me! I’m statistically significant!” But hold your applause! Just because something is statistically significant doesn’t mean it matters in the real world. Think of it as the glittering trophy you win for a game where the score doesn’t count.
On the other hand, practical significance steps in like a wise old sage, asking, “Is this finding meaningful in our everyday lives?” It emphasizes the importance of the effect size and its implications. A statistically significant result with a minuscule effect size might lead to decisions that are more about numbers than about making a difference.
If you want to explore the broader context of data science, consider “The Data Science Bible”. This comprehensive guide covers a wide range of topics in data science.
Recognizing the difference between these two concepts is crucial for researchers and decision-makers alike. Misunderstanding can lead to misguided conclusions. For instance, a medical study might find a statistically significant reduction in symptoms, but if the actual improvement is negligible, should we really be celebrating?
In research and data analysis, it’s essential to evaluate both statistical and practical significance. This ensures that our findings not only have a mathematical basis but also a meaningful impact on real-world applications. As you move forward in your work, remember to apply this understanding. Strike a balance between the numbers that make you look good and the implications that truly matter.
With this knowledge in your toolkit, you can improve the relevance and impact of your findings, making sure that your research isn’t just a statistical exercise but a valuable contribution to your field. Let’s make our findings count—beyond the p-value! tips for effective data analysis in economics and statistics
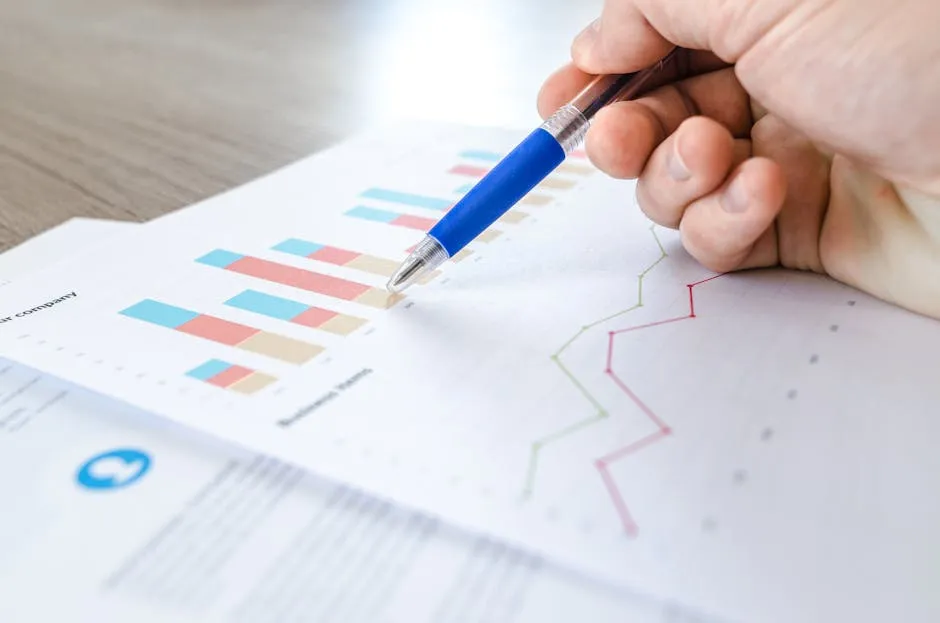
FAQs
Please let us know what you think about our content by leaving a comment down below!
Thank you for reading till here 🙂
Understanding statistical significance and practical significance is essential for informed decision-making in research. tips for effective data analysis in economics and statistics
All images from Pexels