Introduction
Statistics plays a vital role across various fields, including science, social science, and business. It helps us make sense of the vast amounts of data generated daily. Whether you’re analyzing trends in health data, making marketing decisions, or interpreting social research, statistics provides the tools needed to draw meaningful conclusions.
Imagine trying to navigate the complex world of data without a map. That’s where statistics comes in! It acts as a compass, guiding researchers and analysts through the often murky waters of information. By understanding the principles of statistics, you can enhance your decision-making abilities. This article aims to outline the fundamental principles of statistics, their applications, and their relevance in decision-making processes.
So, why should you care about the principles of statistics? Well, mastering these concepts can elevate your analytical skills and improve your ability to interpret data effectively. Moreover, the principles of statistics help you understand the validity of research findings, which can be crucial in today’s data-driven society. If you’re looking to get started, a great companion is Statistics for Dummies, which simplifies the concepts beautifully!
With that in mind, let’s dive into the fundamentals of statistics that will empower you to make informed decisions based on data!
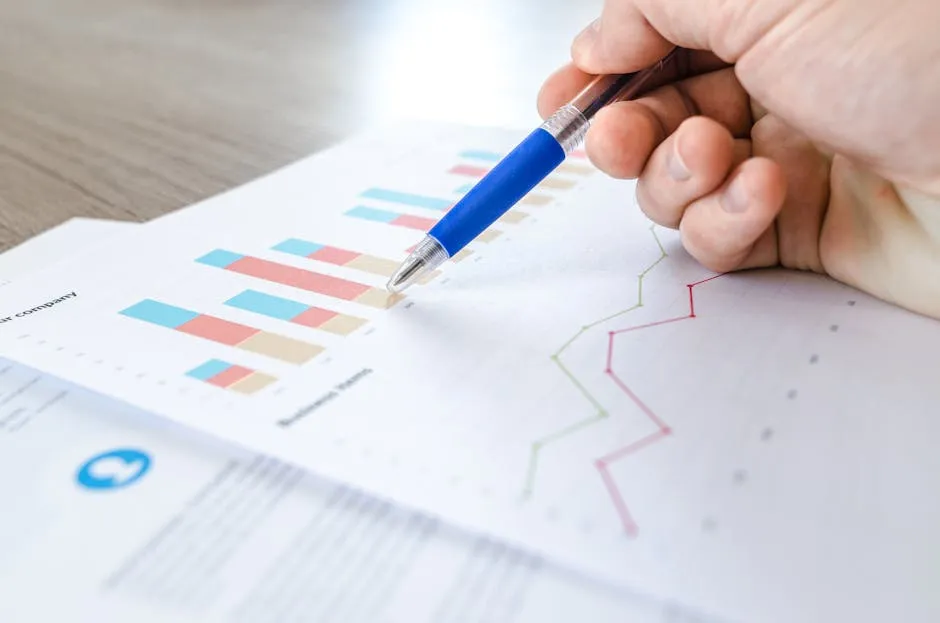
Understanding Statistics
Definition of Statistics
Statistics is the systematic collection and analysis of data. It’s like cooking: you gather ingredients (data), mix them (analyze), and create a dish (conclusion). This process is essential for drawing insights about populations when details about those populations are vague or incomplete.
At its core, statistics involves two main types: descriptive and inferential. Descriptive statistics summarizes data points, providing a snapshot of what the data looks like. Think of it as the highlights of a movie—crucial moments that give you a sense of the entire plot without spoiling the ending. For a deeper understanding of how descriptive statistics can be applied in manufacturing, check out our guide on descriptive statistics in manufacturing plant.
Understanding descriptive statistics is crucial for analyzing data effectively. Learn more about its applications in manufacturing.
On the other hand, inferential statistics takes it a step further. It allows us to make predictions or inferences about a population based on sample data. This is akin to predicting the outcome of a sports game based on previous performances. By understanding these two forms, you can better appreciate how statistics helps us navigate uncertainty and make informed choices.
If you want to dive deeper into inferential statistics, I recommend Naked Statistics: Stripping the Dread from the Data. It breaks down complex ideas into digestible bites—perfect for those who find statistics intimidating!
Types of Statistics
Descriptive Statistics: This type summarizes data points to provide clear insights. It includes measures like mean, median, and mode. For example, if you’re looking at test scores, descriptive statistics can reveal the average score, the middle score, and the most frequently occurring score, helping you understand trends and performance levels.
Inferential Statistics: This is where the magic happens! Inferential statistics enables predictions or generalizations about a larger population based on a smaller sample. It allows researchers to make educated guesses, like predicting election outcomes based on poll data. This method is crucial when it’s impractical or impossible to collect data from an entire population. For insights into the challenges of inferential statistics, refer to our article on the problem with inferential statistics.
Inferential statistics allows researchers to draw conclusions from sample data. Explore the challenges associated with this method.
Understanding these two fundamental types of statistics is key to grasping how data analysis works. They lay the groundwork for exploring deeper statistical methods and principles that can further refine your analytical skills. With these insights, you’re now ready to tackle the core principles of statistics and enhance your data literacy!
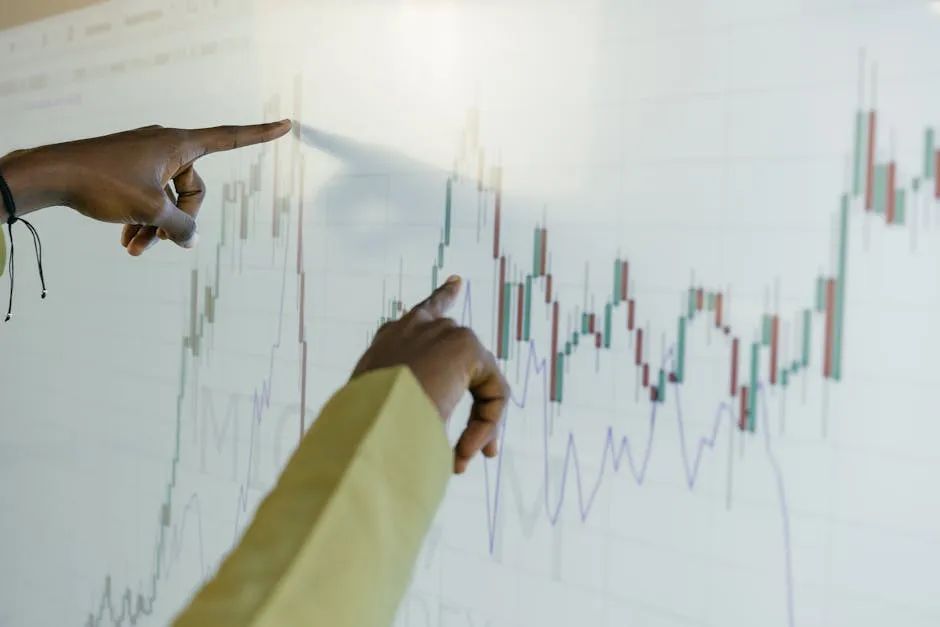
Core Principles of Statistics
Deterministic vs. Stochastic Principles
Statistics can be divided into two fundamental categories: deterministic and stochastic principles. Let’s break these down.
Deterministic Principles refer to specific measurements. Think of them as a precise recipe that guarantees a perfect cake every time. In statistics, these measurements yield exact numerical values. For instance, if you know the height of every student in a class, you can confidently state the average height. These values come from logical reasoning, whether it’s inductive (drawing general conclusions from specific instances) or deductive (applying general principles to specific cases).
On the flip side, we have Stochastic Principles. These are a bit fuzzier—like trying to guess how many jellybeans are in a jar without peeking. Here, the actual outcome of individual measurements isn’t critical. Instead, we focus on identifying meaningful patterns from a range of possibilities. Imagine using historical weather data to predict tomorrow’s weather. You don’t need the exact temperature, but rather the trend that suggests it might rain. Stochastic principles embrace the uncertainty and variability in data, allowing statisticians to make educated guesses.
If you want to dive deeper into statistical modeling, consider checking out The Data Warehouse Toolkit: The Definitive Guide to Dimensional Modeling. It’s a fantastic resource for understanding how to structure data effectively!
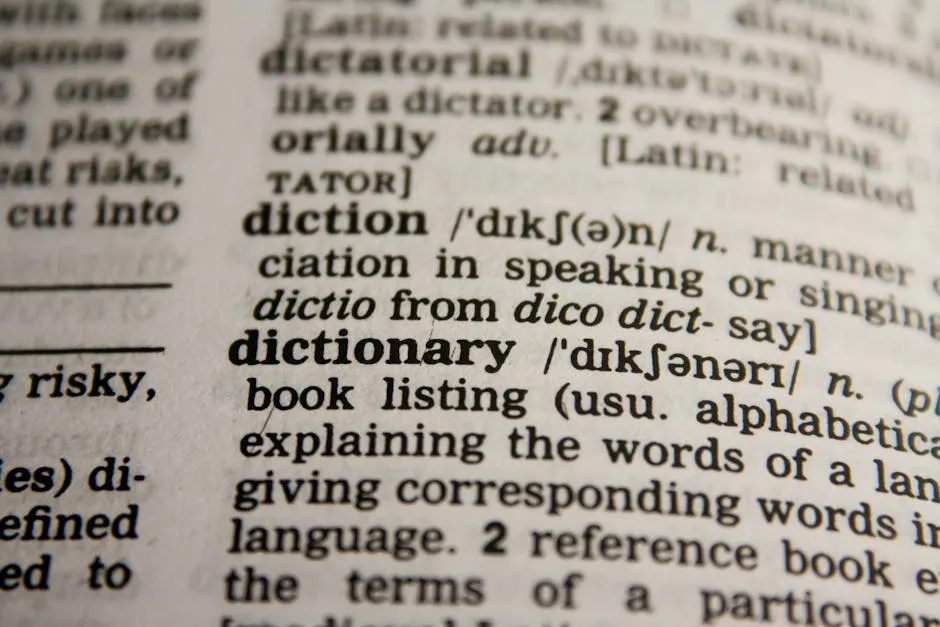
Common Statistical Terms
Mean, Median, Mode, Range: These terms form the backbone of descriptive statistics. The mean is the average of a data set, calculated by adding up all the values and dividing by the count. The median is the middle value when the data is sorted, providing a robust measure that isn’t swayed by outliers. The mode is simply the most frequently occurring value. Finally, the range measures the spread of the data, calculated by subtracting the smallest value from the largest.
Variance and Standard Deviation: Now, variance is like a report card for data spread. It measures how far each number in the set is from the mean. But it’s the standard deviation (SD) that puts things into perspective. Think of SD as a cozy blanket that tells you how tightly your data points are clustered around the mean. A small SD means the data points are close to the mean, while a large SD indicates a wider spread.
Hypothesis Testing: This is where the fun begins! Hypothesis testing is a method for making decisions using data. You start with a null hypothesis, which states there’s no effect or difference. Then, you test this hypothesis. A Type I error occurs when you reject a true null hypothesis—this is a false positive. On the other hand, a Type II error happens when you fail to reject a false null hypothesis—this is a false negative. P-values come into play here, helping to quantify the evidence against the null hypothesis. A low P-value (typically below 0.05) suggests that you can confidently reject the null hypothesis. For a quick reference, check out our statistics hypothesis testing cheat sheet.
Hypothesis testing is a critical aspect of statistics. Review our cheat sheet for a comprehensive overview.
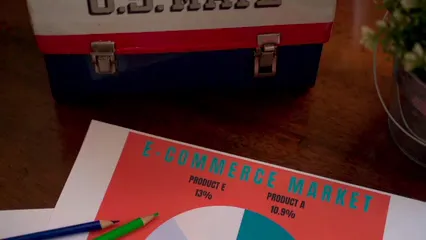
Distribution and Variation
Let’s talk about distribution, specifically the Normal Distribution. Picture a bell curve. Most people’s heights, test scores, or IQs fall around the average—hence the bell shape. This normal distribution is crucial in statistics. It implies that data near the mean are more frequent than data far from the mean. Knowing this helps statisticians make predictions and assess probabilities.
When discussing distribution, we can’t ignore Standard Deviation. It tells us how much variation exists in our data. In a normal distribution, about 68% of the values fall within one standard deviation from the mean, and about 95% fall within two standard deviations. This means that if you know the mean and standard deviation, you can make informed guesses about the likelihood of certain outcomes.
Statistics, in essence, provides a toolbox filled with methods to analyze data. Understanding these core principles will enhance your ability to make informed decisions based on solid evidence. Whether you’re a budding statistician or a curious reader, grasping these concepts opens up a world of analytical possibilities. If you’re keen on enhancing your data skills, consider picking up The Art of Statistics: Learning from Data. It’s a fantastic guide to understanding how to make sense of data!
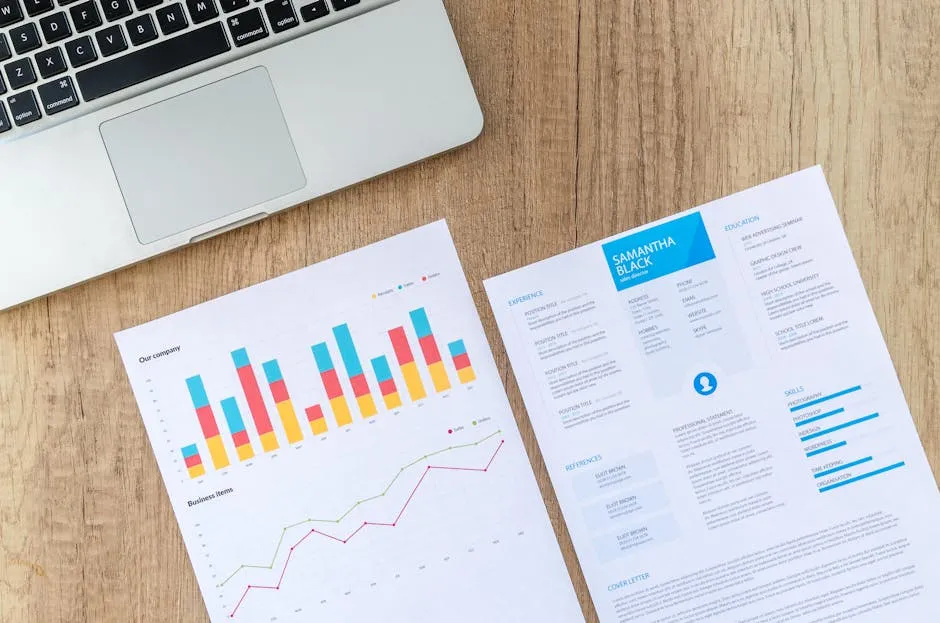
Sampling Techniques
Importance of Sampling
Why Sample? Sampling is like having a bite of a delicious pie before committing to a whole slice. It allows researchers to make statistical generalizations about entire populations without needing to survey every single individual. Imagine trying to ask every student in a university about their favorite pizza toppings—talk about a daunting task! Sampling saves time and resources while still providing valuable insights.
When done correctly, sampling helps researchers predict trends, draw conclusions, and make informed decisions. It reduces the risk of inaccuracies that might arise from surveying everyone, which can lead to overwhelming amounts of data that are hard to analyze. In a nutshell, sampling is essential for making sense of large datasets. It provides a manageable way to gather insights and enables researchers to represent diverse groups effectively.
If you’re curious about the different sampling methods, Data Analytics Made Accessible is a great read that sheds light on various techniques and their applications!

Types of Sampling Methods
Random Sampling: Picture this: you’re at a party, and everyone is wearing a different hat. If you randomly select five people to ask their opinion on the music, you’re practicing random sampling! This method minimizes bias and gives each individual an equal chance of being chosen. Random sampling is critical for ensuring that your sample accurately reflects the population. By eliminating favoritism, researchers can draw more reliable conclusions.
Imagine conducting research on consumer behavior. If you only ask friends or people from the same neighborhood, you might miss out on varied perspectives. Random sampling allows researchers to gather diverse opinions, making their findings more robust and applicable to broader demographics.
Stratified Sampling: Now, let’s turn our attention to stratified sampling. This method is like making a fruit salad. You wouldn’t just toss in any random fruit; you’d want a balanced mix! Stratified sampling involves dividing the population into subgroups, or strata, based on specific characteristics—like age, gender, or income level. Then, researchers randomly sample from each subgroup.
This approach ensures representation across all segments of the population. For example, if you’re researching college students’ study habits, stratifying by year (freshman, sophomore, etc.) ensures all voices are heard. This way, you avoid skewing your results toward any one group, leading to more accurate conclusions.
To further enhance your understanding of data collection methods, consider picking up The Elements of Statistical Learning: Data Mining, Inference, and Prediction. It’s a comprehensive guide that dives deeper into statistical methodologies!
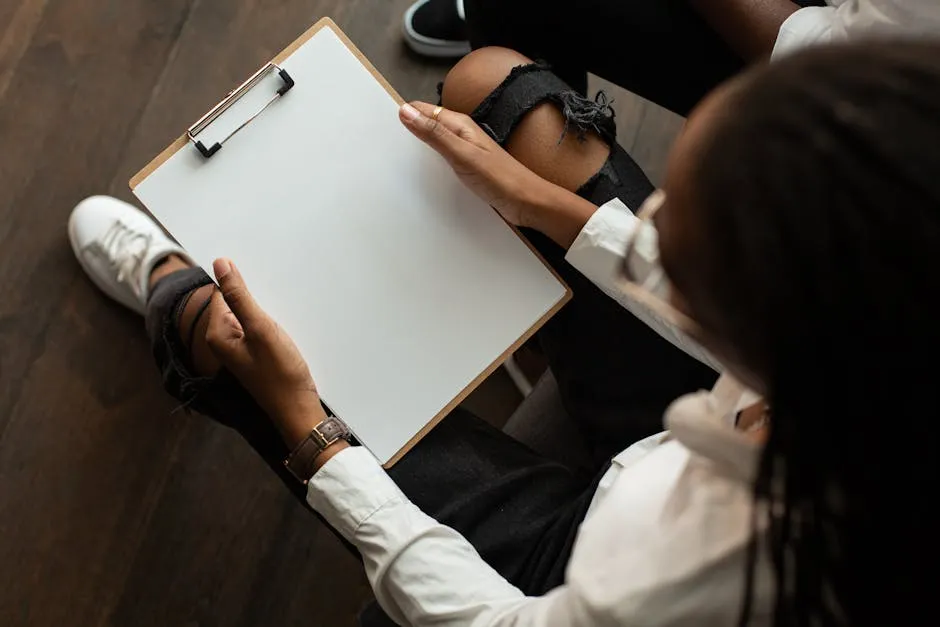
Designing Effective Surveys
Survey Design Principles: Creating a survey is like crafting a recipe. You need the right ingredients for a delicious outcome! Clear objectives and unbiased questions are essential for effective survey design. What are you trying to achieve? Define your goals before even drafting your questions. This clarity will guide you in creating relevant and focused questions.
Moreover, ensure that your questions are straightforward. Ambiguous language can confuse respondents and skew results. Craft questions that are easy to understand, allowing participants to respond honestly. Remember, a well-designed survey is the backbone of reliable data collection.
If you’re looking for practical tools to assist in data collection, a Notebook for Data Analysis can be incredibly helpful for jotting down ideas and observations during your research!
Bias in Surveys: Ah, bias—the sneaky little gremlin that can ruin your survey results! Common pitfalls include leading questions, which can sway respondents toward a particular answer. For example, asking, “Don’t you think our service is the best?” is leading and not objective. Instead, ask open-ended questions, allowing respondents to express their true opinions.
Another issue is the survey’s wording. Jargon or overly complex phrasing can alienate participants. Aim for simplicity. Additionally, be cautious of your sample selection. If you only survey people from a specific demographic, your results may not represent the larger population accurately. Awareness of these biases is crucial for maintaining the integrity of your survey results.
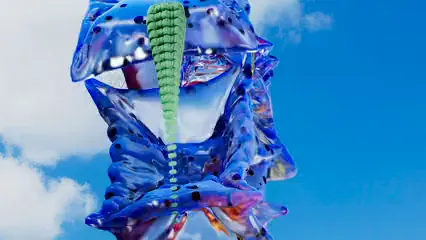
Applications of Statistical Principles
In Research and Trials
Statistics is the backbone of research and clinical trials. It guides decisions in various fields, like public health and medicine. For instance, epidemiological studies rely heavily on statistical methods to assess disease incidence. By analyzing data from diverse populations, researchers can identify risk factors and develop effective interventions. This statistical insight drives public health policies and shapes healthcare practices, ultimately saving lives.
In clinical trials, statistics plays a pivotal role in determining treatment efficacy. Researchers utilize random sampling to assign participants to different treatment groups, ensuring unbiased results. By analyzing outcomes, they can confidently ascertain the effectiveness of new drugs or therapies, providing valuable information for medical professionals and patients alike.
For those interested in the intersection of data science and business, Data Science for Business: What You Need to Know about Data Mining and Data-Analytic Thinking is an essential read!
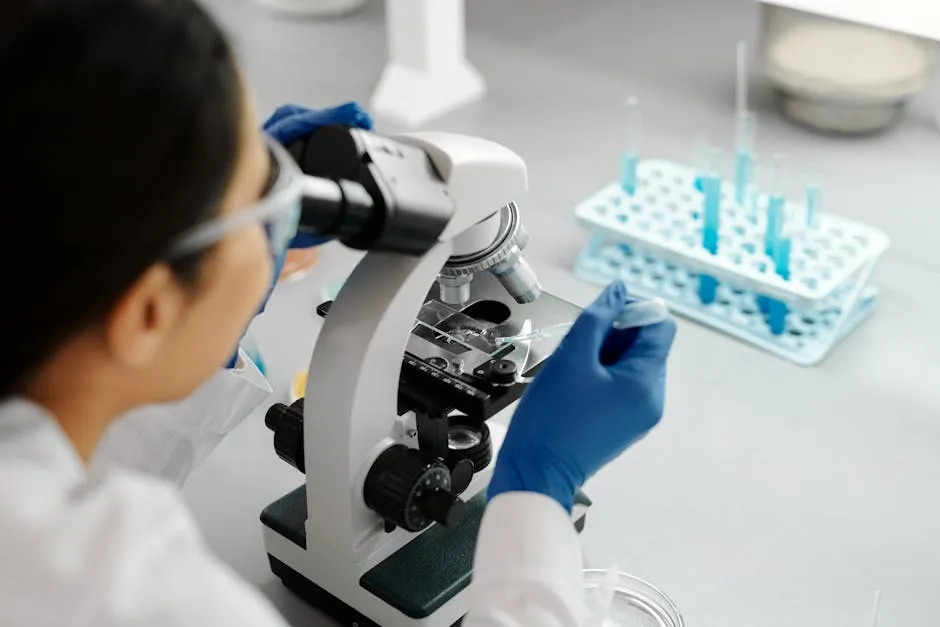
In Business and Economics
In the business world, statistics is a game-changer. Companies utilize market research to understand consumer behavior. By analyzing survey data, businesses can identify trends, preferences, and demographics. This insight informs product development, marketing strategies, and customer engagement, leading to increased sales and customer satisfaction.
Statistics also plays a vital role in financial analysis. Companies rely on data to assess risks and make informed investment decisions. By analyzing historical performance and market trends, businesses can forecast future outcomes and allocate resources effectively. In this data-driven landscape, statistical principles empower organizations to thrive and adapt to changing market dynamics.
A useful tool in this arena is Microsoft Excel 2019 Data Analysis and Business Modeling, which provides powerful tools for data analysis!
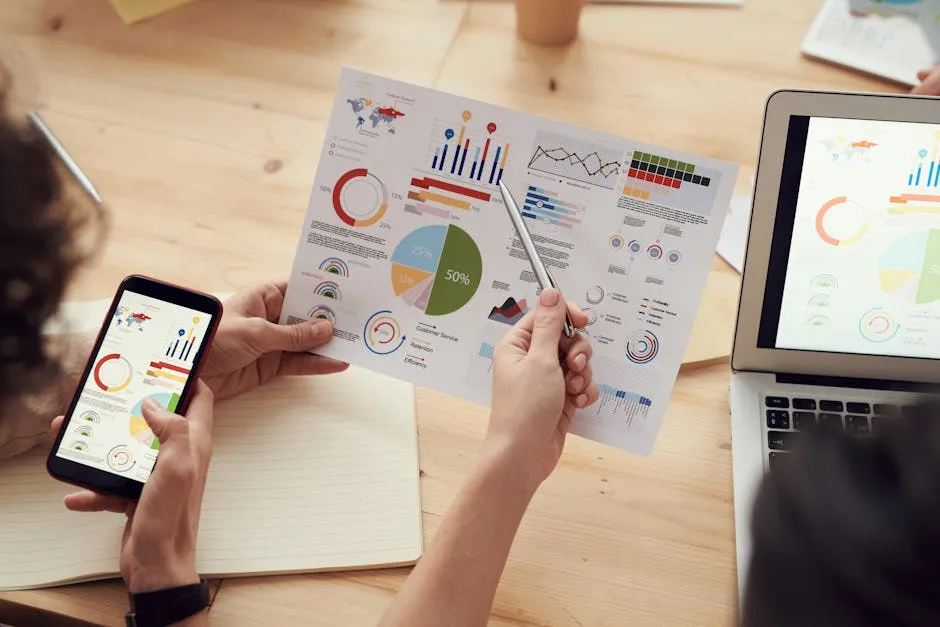
Real-world Examples
Let’s sprinkle in some real-world examples to highlight the applications of statistical principles! Take the renowned study on smoking and lung cancer. Researchers used stratified sampling to ensure diverse representation across age groups and smoking habits. By analyzing the data, they established a strong correlation between smoking and lung cancer, leading to public health campaigns and policy changes.
In another example, a popular online retailer conducted a survey to assess customer satisfaction. They utilized random sampling to gather a representative sample of their customer base. The results revealed key insights into purchasing experiences, prompting the company to enhance customer service and improve product offerings.
These examples illustrate the power of statistical principles in driving meaningful change across various sectors. By applying these methods, researchers and businesses can make data-informed decisions that positively impact society. If you’re interested in visualizing this data, consider picking up Tableau for Dummies—it’s a fantastic way to learn how to present data visually!
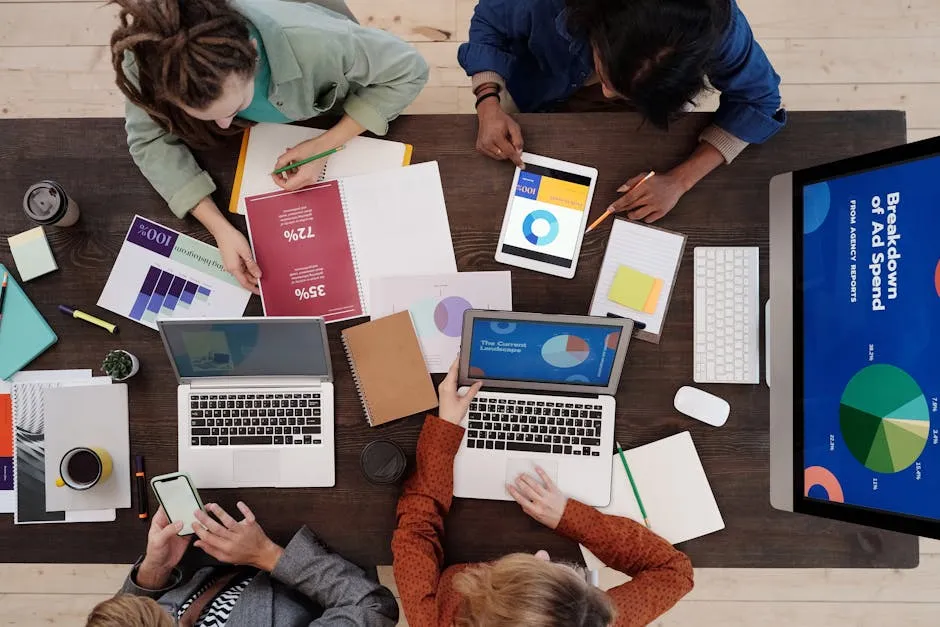
Conclusion
Statistics is a fascinating field that plays a crucial role in many disciplines. Throughout this article, we’ve examined several key principles of statistics, shedding light on their significance. From deterministic and stochastic principles to essential terms like mean, median, and variance, these foundational concepts provide the bedrock for analyzing data intelligently.
Understanding statistics is not just for statisticians. It’s essential for anyone who interacts with data—be it in business, health, or social sciences. The ability to interpret data accurately is vital in today’s information-rich environment. For instance, businesses rely on statistical analysis to make informed decisions, while health professionals use it to understand disease patterns and improve patient outcomes.
Moreover, the principles of statistics empower researchers to draw reliable conclusions from their studies. Whether you’re conducting a clinical trial or a market research survey, a solid grasp of statistical methods can lead to more meaningful results. The process of hypothesis testing, sampling techniques, and understanding distributions enables researchers to navigate uncertainty with confidence.
So, what’s the takeaway? Embrace the power of statistics! By appreciating its principles, you equip yourself with the tools necessary for informed decision-making. Whether you’re analyzing data trends, interpreting survey results, or evaluating research findings, statistics will guide you toward making wiser, evidence-based choices.
In our rapidly evolving world, the ability to discern facts from fiction is invaluable. Statistics not only helps us understand the world better but also enhances our critical thinking skills. So, the next time you encounter data, remember the principles we discussed and consider how they can enhance your understanding and decision-making processes.
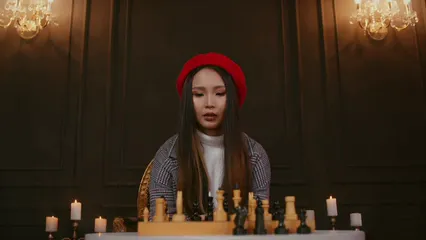
FAQs
What are the basic principles of statistics?
The basic principles of statistics encompass several fundamental concepts that guide data analysis. These include deterministic and stochastic principles, which differentiate between exact measurements and patterns derived from data variability. Understanding key statistical terms such as mean, median, mode, variance, and hypothesis testing is essential for interpreting data accurately. These principles provide a framework for drawing meaningful conclusions and making informed decisions.
How is statistics applied in real life?
Statistics finds its application in numerous fields. In health, researchers analyze data to identify disease trends and evaluate treatment effectiveness. For example, epidemiological studies utilize statistical methods to track the spread of diseases and assess risk factors. In business, companies rely on market research to understand consumer preferences and improve product offerings. Statistical analysis informs financial decisions by assessing risks and forecasting market trends. In social sciences, statistics helps researchers interpret survey data, providing insights into public opinions and behaviors.
What is the difference between descriptive and inferential statistics?
Descriptive statistics summarizes and describes the characteristics of a dataset. It provides measures such as mean, median, and mode to give an overview of the data. Inferential statistics, on the other hand, allows researchers to make predictions or inferences about a larger population based on a sample. For instance, if a survey is conducted among a small group of voters, inferential statistics can help predict election outcomes for the entire population. Essentially, descriptive statistics focuses on presenting data, while inferential statistics enables generalizations and predictions.
Why is sampling important in statistics?
Sampling is crucial because it enables researchers to make generalizations about a population without surveying every individual. By selecting a representative sample, statisticians can draw conclusions that apply to the larger group. Effective sampling reduces bias and enhances the reliability of results. For example, in a health study, randomly selecting participants from diverse demographics ensures that findings reflect the population accurately. Poor sampling can lead to misleading conclusions, emphasizing the importance of using appropriate sampling techniques.
What common mistakes should be avoided in statistical analysis?
Several pitfalls can undermine statistical analysis. One common mistake is misunderstanding the significance of P-values. A low P-value does not imply that results are practically significant. Additionally, researchers often fall into the trap of cherry-picking data to support their hypotheses. This selective reporting can skew findings and mislead audiences. Failing to account for confounding variables, using biased survey questions, and neglecting to check assumptions underlying statistical tests are other common errors. Awareness of these pitfalls will enhance the integrity and accuracy of statistical analyses.
Please let us know what you think about our content by leaving a comment down below!
Thank you for reading till here 🙂
All images from Pexels