Introduction
Data analysis is a cornerstone of modern financial forecasting. Businesses rely on it to make strategic decisions and plan for the future. With the ever-changing market dynamics and technological advancements, financial forecasting has transformed dramatically. Organizations are now more aware than ever that accurate forecasting can mean the difference between thriving and merely surviving. Technological developments like artificial intelligence (AI) and machine learning (ML) are reshaping how financial data is analyzed and utilized. These innovations enable companies to process vast amounts of data more efficiently, drawing insights that were once difficult to obtain. As a result, organizations can anticipate market trends and adapt to changes more swiftly. In this article, we’ll explore key trends that are influencing data analysis for financial forecasting in 2024. These trends include advancements in predictive modeling, the rise of data democratization, and the integration of real-time analytics. Staying updated with these trends is vital for businesses aiming for sustainable growth. Ignoring these developments could leave organizations vulnerable in an increasingly competitive landscape. As we dive into the specifics, remember that leveraging these insights effectively can empower your business to make informed decisions and ultimately achieve financial success. To enhance your understanding of data science principles, consider picking up a copy of “Data Science for Business” by Foster Provost and Tom Fawcett. It’s a great read to get you started on your data journey!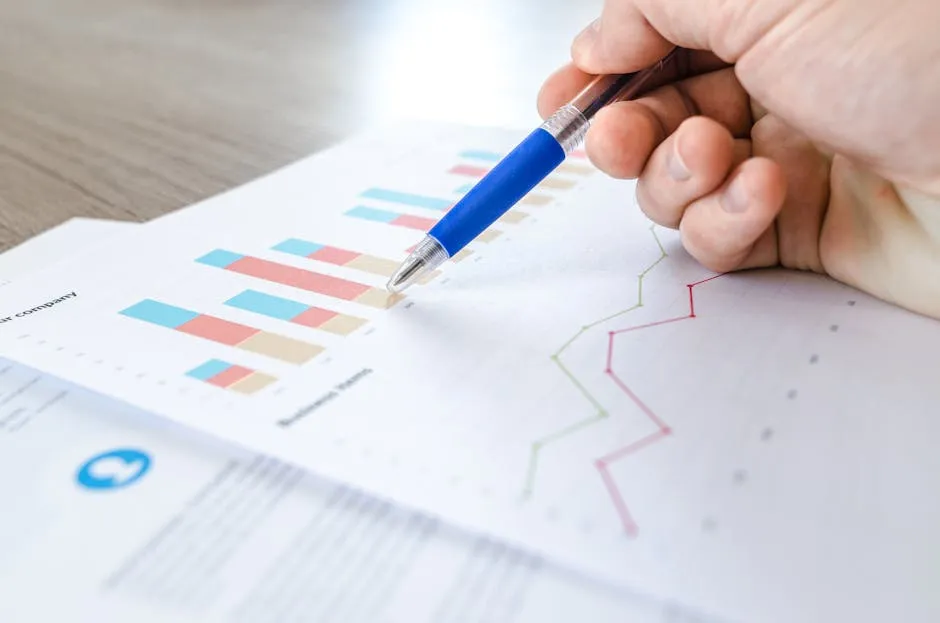
The Evolution of Financial Forecasting
Historical Context
Financial forecasting has evolved significantly over the years. Traditionally, businesses relied on manual methods and static models, often leading to inaccuracies. These methods were limited by the volume of data that could be processed and the inability to react quickly to market changes. This reliance on guesswork and assumptions limited many companies’ strategic planning abilities. With the rise of data analytics, organizations began to embrace more sophisticated approaches. Data-driven forecasting enables companies to harness historical data, market trends, and economic indicators to inform their predictions. This shift has led to a more reliable and accurate forecasting process, allowing businesses to make more informed decisions.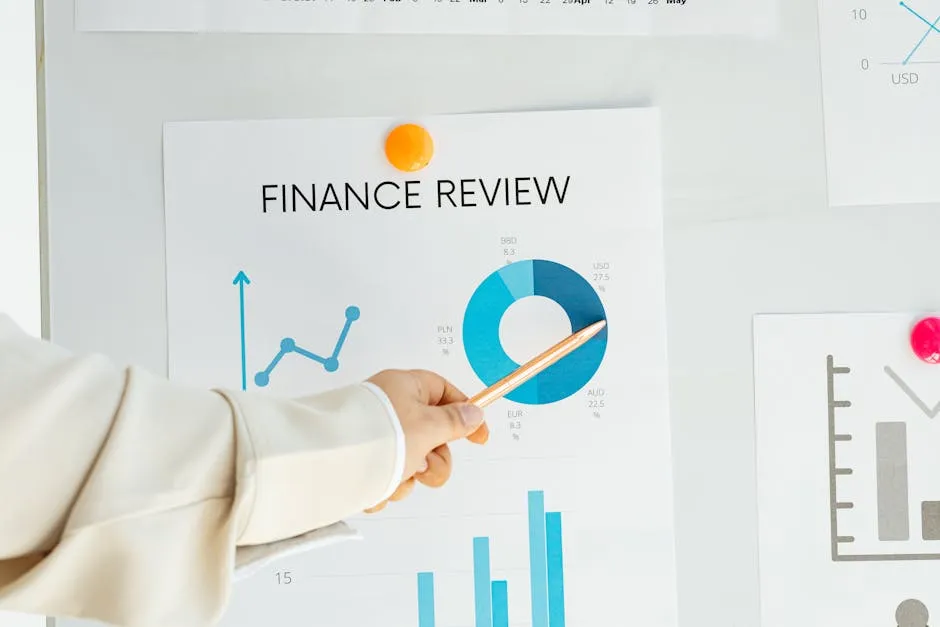
The Shift to Data-Driven Approaches
The transition to data-driven approaches marks a significant turning point in financial forecasting. Big data and analytics are now at the forefront, transforming how companies approach their financial predictions. Organizations can leverage analytics to uncover insights that were previously hidden, enabling them to make strategic decisions based on real-time data. Accurate data collection and preparation are essential for this transformation. Companies must invest in data management systems that ensure the integrity and quality of their data. By doing so, they can create a solid foundation for their forecasting models, enhancing their accuracy and reliability. As we continue to explore the latest trends in data analysis for financial forecasting, it becomes clear that the evolution from traditional methods to data-driven approaches is crucial. Businesses that embrace these changes are better positioned to navigate the complexities of the financial landscape and drive sustainable growth. And while you’re at it, why not check out “Predictive Analytics” by Eric Siegel to dive deeper into the predictive aspects of data science.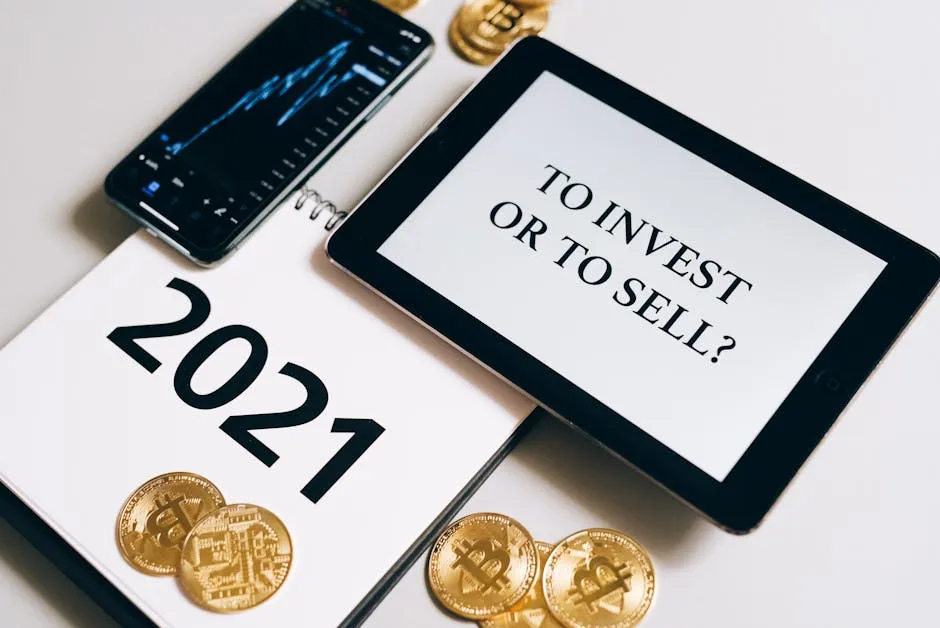
Key Trends in Data Analysis for Financial Forecasting
1. Increased Use of AI and Machine Learning
Artificial Intelligence (AI) and Machine Learning (ML) are making waves in financial forecasting. These technologies analyze vast datasets with unprecedented speed and accuracy. By processing historical data and identifying patterns, AI can predict future financial outcomes with remarkable precision. Predictive analytics is one key application. For instance, financial institutions use AI algorithms to assess credit risks. This not only enhances accuracy but also speeds up decision-making processes. Take the case of Upstart, an AI-powered lending platform. It evaluates creditworthiness beyond traditional metrics, leading to fairer lending practices. If you want to harness the power of machine learning for your investments, you might find “Machine Learning for Asset Managers” by Marcos López de Prado quite enlightening. Real-time insights are another benefit. Companies can monitor market trends and adjust forecasts instantly. For example, Delta Airlines employs AI to track baggage handling, significantly improving operational efficiency. This capability allows businesses to react to market changes swiftly, keeping them competitive. Incorporating AI into forecasting isn’t just a trend; it’s a necessity. It reduces errors, enhances productivity, and mitigates risks. Companies that adopt these technologies see a marked improvement in their forecasting accuracy, often by as much as 30%. As AI continues to evolve, its role in financial forecasting will only become more critical.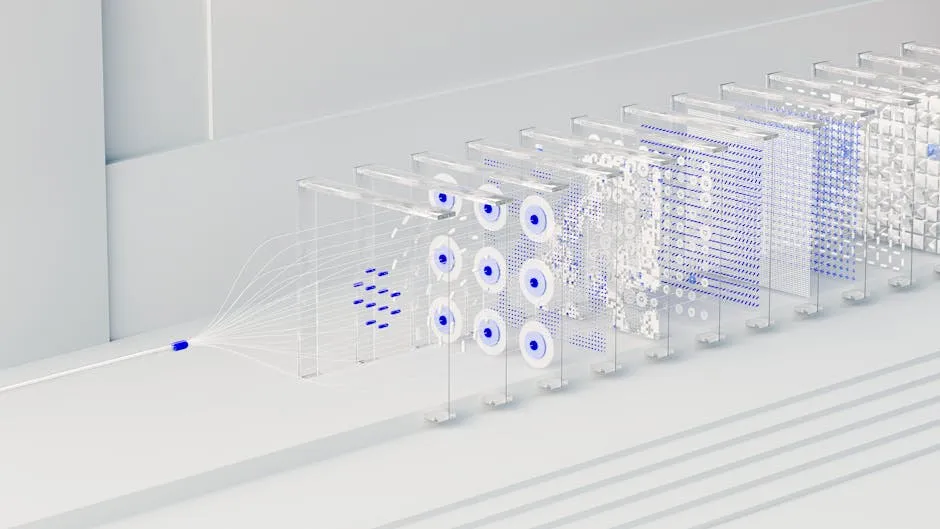
2. The Democratization of Data
Data democratization is a game changer in financial forecasting. This concept refers to making data accessible to all employees, regardless of their technical expertise. The goal? Empower non-technical users to analyze data and derive insights independently. Self-service analytics tools play a key role here. These platforms allow users to create reports and dashboards without needing IT intervention. Coca-Cola, for instance, trains its managers to become “citizen data scientists.” This initiative enables broader data analysis capabilities across the organization. If you want to dive deeper into the world of data science, grab a copy of “Artificial Intelligence: A Guide to Intelligent Systems” by Michael Negnevitsky to understand the underlying technologies better. The benefits of democratization are clear. Research indicates that 80% of business leaders believe access to data enhances decision-making. Yet, only 60% of organizations effectively provide access to necessary tools. This gap presents an opportunity for companies to leverage data more effectively, leading to better financial forecasts and informed decision-making. By democratizing data, organizations can foster a culture of data-driven decision-making. As more employees engage with data, they can identify trends and make predictions that align with the company’s financial goals. This shift not only improves forecasting accuracy but also encourages innovation within teams.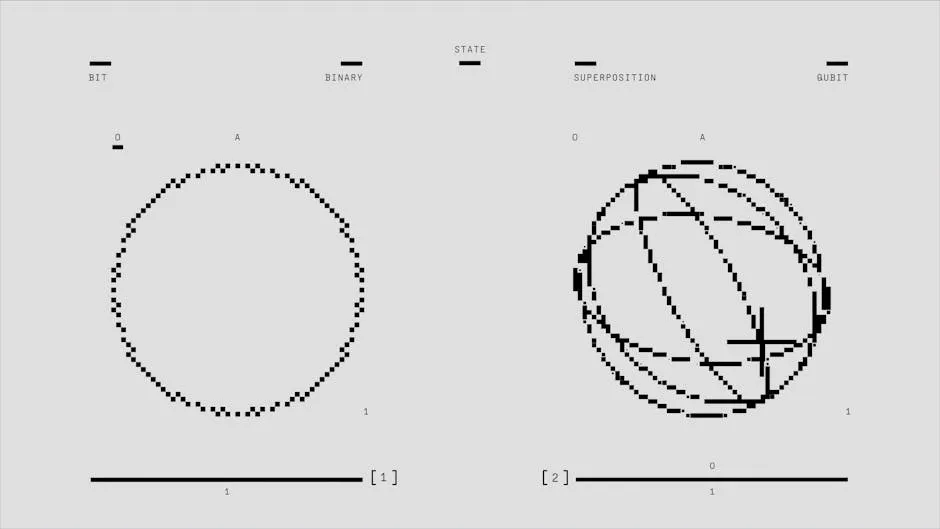
For more insights on effective data analysis, check out this article on tips for effective data analysis in economics and statistics.
3. Edge Computing for Real-Time Analytics
Edge computing is revolutionizing financial data analysis. By processing data closer to its source, organizations can achieve real-time insights that significantly enhance decision-making speed and accuracy. This technology is particularly valuable in industries that require immediate data processing, such as finance. For example, the United States Postal Service (USPS) utilizes edge analytics to process millions of package images daily. This capability reduces the time needed to locate missing packages from days to mere hours. The benefits extend beyond just efficiency; real-time analytics can help financial institutions quickly identify market shifts and respond accordingly. The growing reliance on IoT devices has further fueled the adoption of edge computing. By 2025, Gartner predicts that over 50% of critical data will be generated and processed outside traditional data centers. This shift allows organizations to maintain high data accuracy while minimizing latency. In the financial sector, edge computing enables firms to monitor transactions as they happen. This immediate access to data can help detect anomalies or fraudulent activities in real time. As organizations continue to embrace edge computing, the combination of speed and accuracy will become paramount for effective financial forecasting. In summary, the trends of increased AI utilization, data democratization, and edge computing are reshaping the landscape of financial forecasting. These innovations not only enhance accuracy but also empower organizations to make informed decisions quickly. As we move into 2024, embracing these trends will be crucial for businesses aiming to thrive in a competitive environment.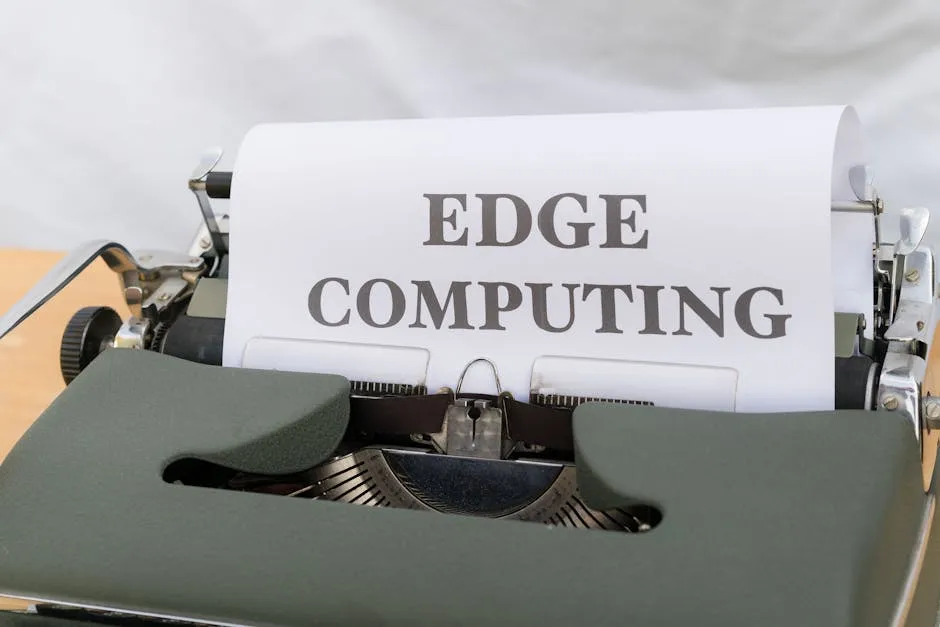
4. Data-as-a-Service (DaaS) Model
Data-as-a-Service (DaaS) is revolutionizing financial forecasting. This model allows companies to access data on-demand through the cloud. Think of it as Netflix for data—streaming valuable insights instead of movies. DaaS provides businesses with the flexibility to obtain data without investing in massive infrastructure. This is especially important for financial forecasting, where timely access to accurate data can make or break a company’s strategy. DaaS enhances accessibility to crucial information. Subscription-based services make it easy for organizations to stay updated with the latest data trends. Imagine being able to pull market data or consumer insights at the click of a button. This accessibility levels the playing field, allowing smaller businesses to compete with larger firms that traditionally had data advantages. According to analysts, the DaaS market is projected to grow at a staggering compound annual growth rate (CAGR) of nearly 40%, reaching $56.85 billion by 2027. This rapid growth reflects the increasing demand for efficient data solutions across industries. Moreover, DaaS allows for better data management. With historical and real-time data at their fingertips, financial professionals can create more accurate forecasts. For instance, companies like Snowflake are leading the charge in this space, offering comprehensive data solutions that empower organizations to leverage data in innovative ways. DaaS simplifies data integration and analytics, making it easier to extract actionable insights. Financial forecasting becomes much more precise, supporting strategic decisions that can drive growth. If you’re looking to enhance your skills in data management, consider “Financial Modeling in Excel For Dummies” by Danielle Stein Fairhurst—it’s a great resource for financial professionals. “Financial Modeling in Excel For Dummies” In essence, the DaaS model is a game-changer for financial forecasting. It democratizes access to essential data, enabling organizations to make informed decisions quickly. As the market continues to expand, businesses that embrace DaaS will likely gain a significant competitive edge. The ability to swiftly adapt to market changes and enhance forecasting accuracy will be invaluable in an increasingly data-driven world.
5. Enhanced Predictive Modeling Techniques
Predictive modeling is evolving rapidly, thanks to advances in data analytics. These enhancements are empowering organizations to make more informed financial forecasts. Techniques such as regression analysis, time series analysis, and machine learning algorithms are at the forefront of this evolution. Each method offers unique advantages, allowing businesses to tailor their forecasting approaches to specific needs. Regression analysis remains a staple for financial forecasting. By examining relationships between variables, it helps organizations predict future financial outcomes. Time series analysis, on the other hand, focuses on historical data to identify trends over time. This technique is crucial for understanding seasonal fluctuations in revenue or expenses. Companies like Amazon leverage time series analysis to optimize inventory management, ensuring they meet consumer demand without overstocking. To deepen your knowledge of statistics, consider “Statistics for Data Science” by James D. Miller—it’s an insightful read for anyone interested in data analytics. “Statistics for Data Science” by James D. Miller Machine learning algorithms are the new kids on the block, but they’re gaining traction quickly. These algorithms analyze vast datasets, uncovering patterns that traditional methods might miss. For example, Upstart, an AI-based lending platform, uses machine learning to assess credit risk more accurately than conventional models. By analyzing alternative data points, Upstart can offer loans to individuals who might have been overlooked by traditional banks. As predictive modeling techniques evolve, so do the tools available to financial professionals. Companies are increasingly investing in advanced analytics platforms that integrate these techniques seamlessly. The global predictive analytics market is expected to reach over $10 billion by 2025. This growth indicates a shift towards more sophisticated forecasting methods that are not only accurate but also agile. In conclusion, enhanced predictive modeling techniques are reshaping financial forecasting. By embracing regression analysis, time series analysis, and machine learning, organizations can make better decisions that drive growth. As these technologies continue to advance, the potential for improved forecasting accuracy and strategic insights will only increase.6. The Role of Synthetic Data
Synthetic data is carving out an essential role in financial forecasting. This artificial data mimics real-world data without revealing sensitive information. In an age where data privacy is paramount, synthetic data provides a solution. Financial institutions can train their models without compromising customer privacy or violating regulations. The benefits of synthetic data are manifold. It allows organizations to create datasets that fill in gaps left by incomplete or biased real-world data. For example, a bank might use synthetic data to simulate various economic scenarios, helping it to stress-test its financial models. This capability is invaluable when evaluating risk and preparing for market fluctuations. Organizations like UC Davis Health are already utilizing synthetic data effectively. They employ it to enhance predictive analytics for patient outcomes, ensuring that their models are robust and reliable. This practice not only preserves patient confidentiality but also helps in creating more accurate forecasts. As regulations around data privacy become more stringent, the demand for synthetic data is expected to grow. Experts predict that by 2025, 60% of data used in AI and analytics will be synthetic. This shift demonstrates the increasing reliance on synthetic data to maintain privacy while maximizing the quality of insights derived from data analytics. In summary, synthetic data is a powerful ally in the realm of financial forecasting. It enables organizations to innovate without jeopardizing privacy and compliance. As the financial landscape evolves, leveraging synthetic data will become essential for businesses looking to enhance their forecasting capabilities while adhering to data protection regulations.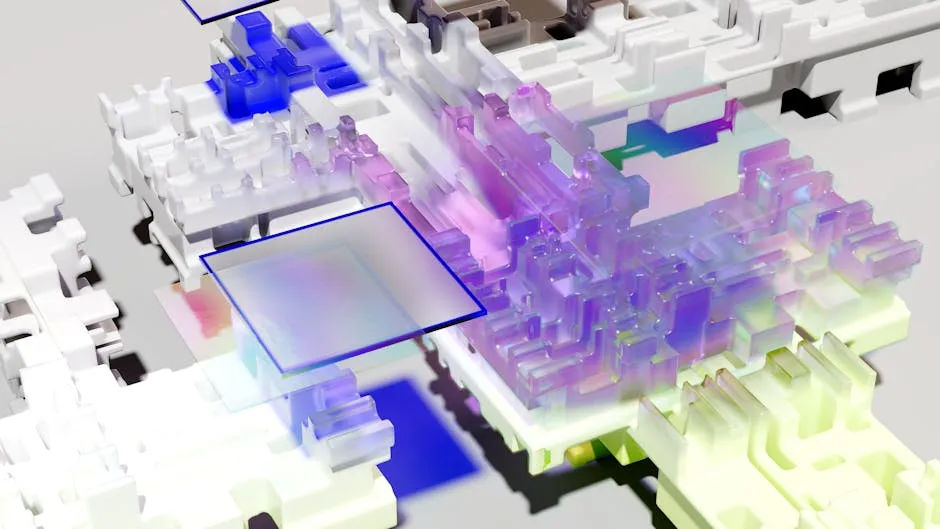
7. Integration of ESG Factors in Financial Forecasting
Environmental, Social, and Governance (ESG) factors are more than just buzzwords; they’re becoming essential elements in financial decision-making. Companies today recognize the need for sustainable practices. Investors want to know how businesses manage their environmental impact and treat their employees. The rise of conscious consumerism has made ESG considerations a vital part of strategic planning. Data analysis is at the heart of this ESG integration. By utilizing advanced analytics, companies can assess ESG metrics effectively. For instance, organizations can analyze carbon footprints, labor practices, and board diversity to gauge their sustainability performance. This data-driven approach enables organizations to create robust ESG strategies that resonate with stakeholders. A recent study revealed that companies focusing on ESG practices often experience enhanced financial performance over time. Let’s take a look at a few case studies that illustrate successful ESG integration into financial forecasts. Consider Unilever, which has made a commitment to sustainability. By analyzing its supply chain data, Unilever identified areas for reducing carbon emissions. This led to a more sustainable business model and a significant reduction in costs. Their efforts not only improved their ESG scores but also boosted investor confidence. Another example is BlackRock, the world’s largest asset manager. BlackRock has started to incorporate ESG factors into its investment strategies, using data analytics to evaluate potential investments. By leveraging ESG data, they can identify risks and opportunities that may not be evident through traditional financial analysis. This shift has allowed BlackRock to attract more investors who prioritize responsible investing. Furthermore, the integration of ESG metrics is not limited to large corporations. Small and medium-sized enterprises (SMEs) are also recognizing the importance of sustainability. A study showed that 70% of SMEs that adopted ESG practices reported improved relationships with customers and suppliers, leading to better financial outcomes. This demonstrates that a commitment to ESG can benefit organizations of all sizes. In conclusion, the integration of ESG factors into financial forecasting is no longer optional. It’s a necessity driven by market demand and societal expectations. By leveraging data analysis to assess ESG metrics, organizations can enhance their decision-making processes. This not only helps in risk management but also positions companies favorably in a competitive landscape. As we move forward, businesses that prioritize ESG considerations will likely see improved financial performance and stronger stakeholder relationships.
8. Regularly Review and Update Forecasting Models
In the fast-paced world of finance, maintaining accuracy in forecasting models is paramount. Regularly reviewing and updating these models ensures they reflect the latest market conditions. Why is this so crucial? Because financial markets are like a game of Whac-A-Mole—just when you think you’ve nailed it, something unexpected pops up. Market dynamics can shift overnight. Economic indicators, consumer behavior, and global events can dramatically alter the landscape. For instance, a sudden change in interest rates or unexpected geopolitical events can have sweeping effects on financial forecasts. Therefore, outdated models may lead to misguided strategies or missed opportunities. Moreover, incorporating real-time data is essential. Businesses that utilize real-time analytics can adjust forecasts as new information emerges. This agility can be the difference between seizing a lucrative opportunity and being left in the dust. Companies like Amazon and Netflix continuously adjust their forecasting models based on customer interactions and market trends. By doing so, they stay one step ahead of the curve. Additionally, it’s not just about the numbers. Engaging key stakeholders during the review process can provide valuable insights. Collaborating with finance teams, data analysts, and even marketing professionals can help uncover hidden patterns or emerging trends that the numbers alone might not reveal. This collaborative approach leads to more comprehensive and reliable forecasting. In conclusion, regularly reviewing and updating forecasting models is not merely a best practice; it’s a necessity. By staying current with market conditions, leveraging real-time data, and engaging stakeholders, businesses can enhance their forecasting accuracy and make informed decisions. Embracing this proactive approach allows companies to navigate uncertainties with confidence, ensuring they remain competitive in the ever-evolving financial landscape.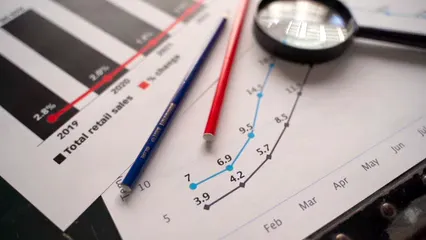
Conclusion
The financial forecasting landscape is evolving rapidly, driven by advancements in data analysis and technology. This article has explored several key trends shaping the future of financial forecasting in 2024. From the increased use of artificial intelligence (AI) and machine learning to the democratization of data, companies must adapt to stay competitive. One of the most significant trends is the integration of AI and machine learning into forecasting processes. These technologies enhance predictive accuracy by analyzing vast data sets and identifying patterns that were previously hidden. Organizations leveraging AI can reduce forecasting errors and improve decision-making efficiency, leading to better financial outcomes. Data democratization is another important trend. By empowering employees at all levels with access to data, businesses foster a culture of data-driven decision-making. This shift enables quicker responses to market changes and enhances overall organizational agility. Companies like Coca-Cola are showcasing the power of self-service analytics tools, allowing non-technical employees to derive insights that drive strategic initiatives. Moreover, the role of real-time analytics cannot be overstated. The ability to adjust forecasts based on current data ensures that organizations remain agile in a rapidly changing environment. Financial institutions utilizing edge computing are already reaping the benefits of real-time data processing, which allows them to make quick, informed decisions. Finally, the integration of Environmental, Social, and Governance (ESG) factors into financial forecasting is becoming increasingly relevant. Investors are prioritizing sustainable practices, and companies that incorporate ESG metrics into their forecasts will likely attract more investment and consumer trust. If you want to learn more about managing your finances effectively, consider “The Lean Startup” by Eric Ries—an essential read for entrepreneurs. “The Lean Startup” As we look ahead to 2024, businesses must embrace these trends to remain competitive. Adopting advanced analytics, fostering a data-driven culture, and ensuring models are regularly updated will be crucial for success. The financial landscape is not static; companies that continuously explore advancements in data analysis will position themselves for sustainable growth and resilience.
FAQs
What is financial forecasting?
Financial forecasting is the process of predicting a company’s future financial performance. It involves analyzing historical data, market trends, and economic indicators to make informed decisions about resource allocation and strategic planning. Accurate forecasting helps businesses anticipate challenges and seize opportunities.
How does AI improve financial forecasting?
AI enhances financial forecasting through predictive analytics and real-time decision-making. By processing large datasets, AI identifies patterns and trends that can inform future performance. This leads to more accurate forecasts and faster responses to market changes, ultimately improving overall financial health.
What is data democratization?
Data democratization refers to the process of making data accessible to all employees, regardless of their technical expertise. This approach empowers non-technical users to analyze data independently, fostering a culture of data-driven decision-making. Companies that successfully implement data democratization can enhance decision-making and improve financial forecasting accuracy.
What are the challenges of implementing data analysis in financial forecasting?
Common challenges include data quality issues, integration difficulties, and resistance to change within organizations. Ensuring data accuracy and completeness is vital for reliable forecasting. Additionally, training employees to effectively use data analysis tools and techniques is necessary for successful implementation.
Why is ESG important in financial forecasting?
Environmental, Social, and Governance (ESG) factors are becoming increasingly important in financial decision-making. Investors are looking for companies that prioritize sustainability and ethical practices. By integrating ESG metrics into financial forecasts, organizations can improve their attractiveness to investors and align with consumer expectations, ultimately enhancing long-term profitability.
Please let us know what you think about our content by leaving a comment down below!
Thank you for reading till here 🙂
All images from Pexels