Introduction
Statistical decision making is all about using data to make informed choices. It’s like having a crystal ball, but instead of magic, it relies on numbers. This approach is crucial in many fields, including business, healthcare, and public policy. Imagine making decisions without data—it’s like trying to cook a soufflé without a recipe. You might end up with a flat mess!
In business, companies harness statistical decision making to understand customer behavior, forecast sales, and improve strategies. Picture a marketer analyzing data to determine which ad campaign resonates best with consumers. In healthcare, statistical analysis helps doctors assess treatment effectiveness, ensuring patients receive the best possible care. Public policy also benefits; well-informed decisions can lead to improved community outcomes.
The importance of informed decision making cannot be overstated. Without statistical analysis, decisions can quickly become guesswork, leading to costly mistakes. This guide will cover the fundamentals of statistical decision making, including its definition, the role of statistics, and key concepts like hypothesis testing and decision theory. So, buckle up as we embark on this data-driven journey!
If you’re looking to dive deeper into the world of statistics, consider picking up “Statistics for Dummies” by Deborah J. Rumsey. It’s like having a friendly tutor by your side, guiding you through the sometimes murky waters of data interpretation.
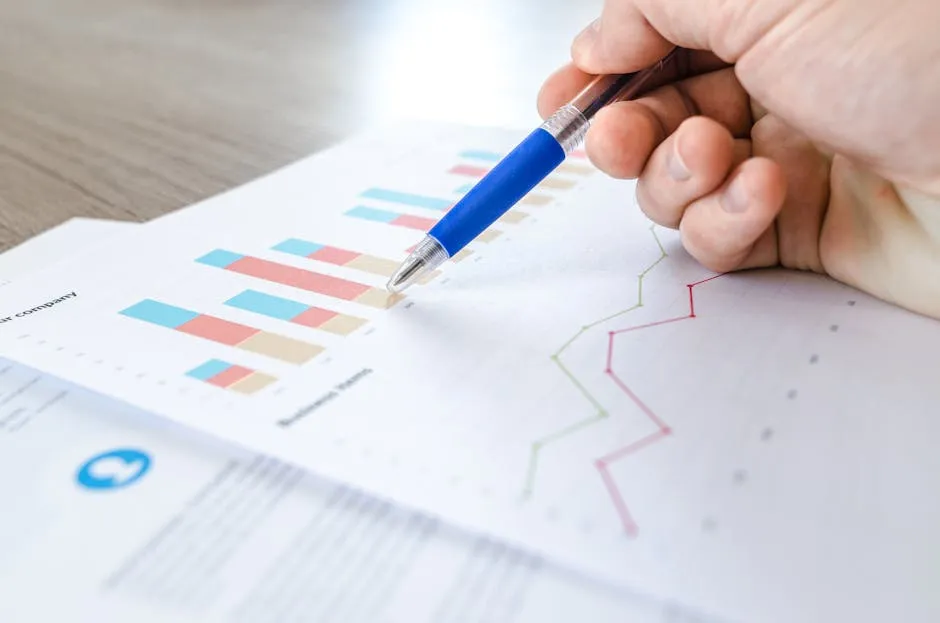
Understanding Statistical Decision Making
What is Statistical Decision Making?
Statistical decision making is the process of using data to inform choices. It involves analyzing data to identify patterns, relationships, and trends. This method is grounded in statistics and decision theory, providing a structured approach to uncertainty.
At its core, statistical decision making helps individuals and organizations determine the best course of action. By leveraging data, the decision-making process becomes more objective and less prone to biases. Think of it as a GPS for your choices—guiding you towards the best route based on available information.
To really understand the backbone of statistical decision making, consider reading “The Art of Statistics: Learning from Data” by David Spiegelhalter. It’s an essential read for anyone wanting to grasp how statistics can shape our decisions.
The Role of Statistics in Decision Making
Statistics plays a vital role in decision making by enabling informed choices. It provides the tools necessary for data collection, analysis, and interpretation. These processes allow decision makers to draw conclusions and make predictions based on empirical evidence.
For instance, in marketing, businesses utilize statistical techniques to analyze consumer behavior and preferences. By examining survey data or sales figures, companies can tailor their strategies to meet customer needs effectively. Similarly, in healthcare, statistical analysis helps assess treatment outcomes, guiding medical professionals in choosing the most effective interventions for patients.
Furthermore, statistics can aid in risk assessment and management. By evaluating potential outcomes and their probabilities, organizations can make calculated decisions that minimize risks. This is particularly important in finance, where investment decisions rely heavily on statistical analysis to forecast market trends and assess potential returns. For those wanting a deeper understanding of finance-related statistics, “How to Measure Anything: Finding the Value of ‘Intangibles’ in Business” by Douglas W. Hubbard is a great resource. Check it out here.
In summary, the integration of statistics into decision making transforms vague intuition into concrete, data-driven insights. As we navigate through the complexities of our data-driven world, understanding how to interpret and utilize statistical information is essential for making sound decisions.
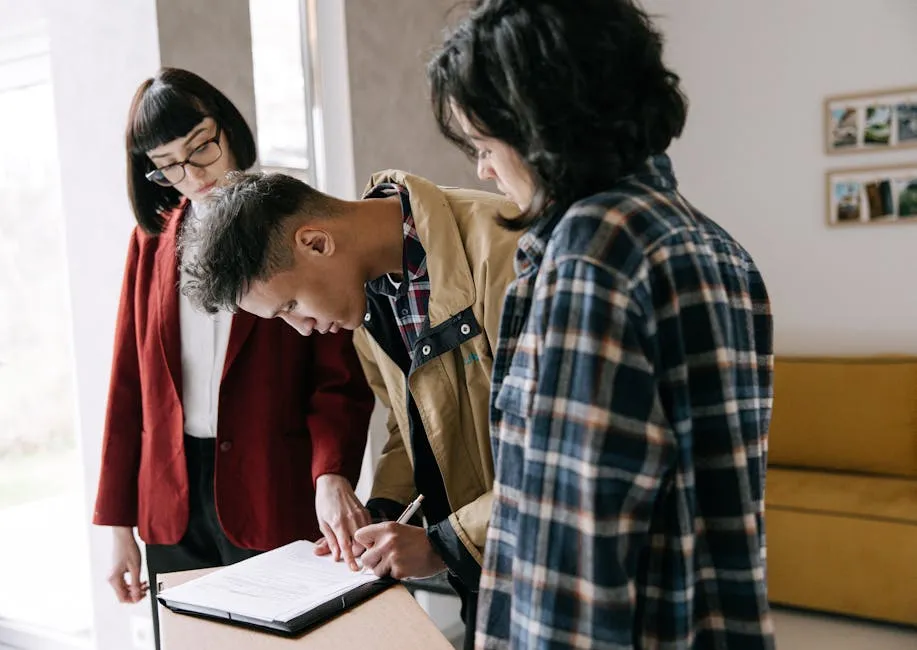
Decision Theory Fundamentals
Decision theory is a fascinating field that combines probability, economics, and philosophy. It’s like a Swiss army knife for making choices under uncertainty. Decision theory comes in three flavors: normative, descriptive, and prescriptive. Each type offers unique insights into how we make decisions.
Normative decision theory focuses on what decisions people should make to maximize their benefits. Think of it as the “ideal” decision maker, calculating choices with perfect accuracy. It’s like having a personal assistant who never makes a mistake—if only we could all be so lucky!
If you’re intrigued by the psychological aspects of decision-making, you might want to check out “Thinking, Fast and Slow” by Daniel Kahneman. It dives deep into the dichotomy of thought processes that influence our decisions.
Descriptive decision theory, on the other hand, takes a peek into how people actually make decisions. It observes real-world behavior and the factors influencing our choices. This approach reveals that humans often stray from the “rational” path, falling prey to biases and heuristics. It’s a bit like watching someone try to assemble IKEA furniture without the instructions—entertaining and often chaotic!
Finally, prescriptive decision theory combines both worlds. It predicts how people should behave based on observed data. By analyzing previous decisions, it aims to improve future choices. Imagine it as a friendly guide, helping you navigate through a maze of options.
In the context of statistical decision making, these theories are essential. They provide a framework for understanding how data influences choices. By applying decision theory, we can make our statistical analyses more robust, ensuring we choose wisely even in the face of uncertainty.
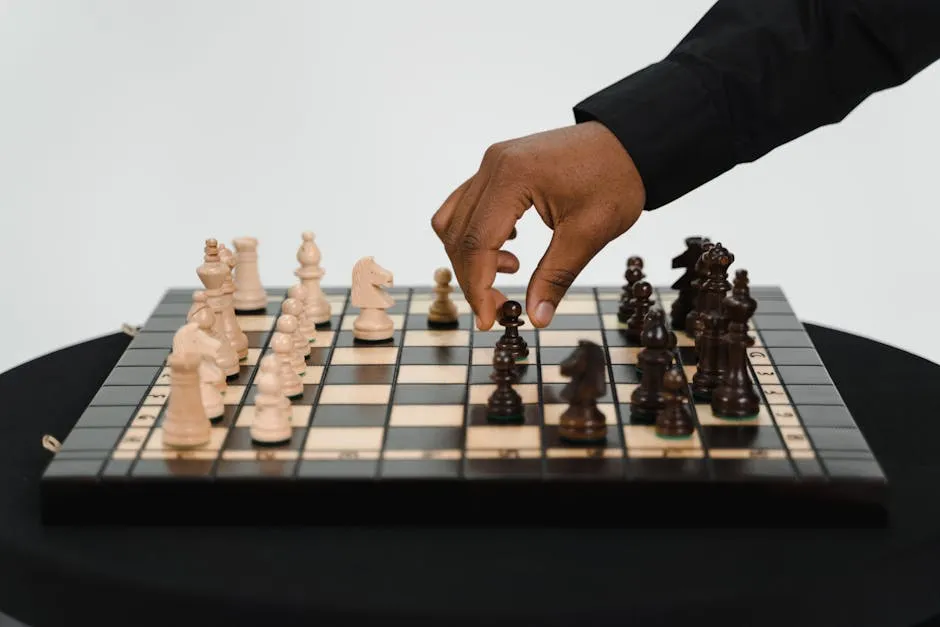
Key Concepts in Statistical Decision Making
Hypothesis Testing
Hypothesis testing is a cornerstone of statistical decision making. It helps us determine if our data supports a specific claim or not. Picture it as a courtroom where the data stands trial. The null hypothesis (H0) is the default position—believing nothing significant is happening. The alternative hypothesis (Ha) challenges that notion, suggesting there is a real effect or relationship.
Why is this important? Well, hypothesis testing allows us to evaluate evidence objectively. If the data is compelling enough, we reject the null hypothesis in favor of the alternative. This process is like a referee making a call in a sports game—clear and decisive.
Enter the p-value, the superstar of hypothesis testing. It represents the probability of observing our data, assuming the null hypothesis is true. A small p-value (typically less than 0.05) indicates our data is unlikely to occur under the null hypothesis. Thus, we feel justified in rejecting H0. It’s like finding a smoking gun that leads us to believe something significant is afoot!
But wait, there’s more! The alpha level is the threshold we set before testing. It’s our line in the sand. If our p-value falls below this level, we reject H0. If not, we retain it. Think of it as the finish line in a race—only the fastest get to pass!
Real-world applications of hypothesis testing are everywhere. For instance, in healthcare, researchers may test a new drug against a placebo. The null hypothesis posits that the drug has no effect. If the data reveals a significant improvement, the null is rejected, paving the way for the drug’s approval. Similarly, businesses may analyze customer satisfaction surveys to evaluate new policies against existing ones.
For a comprehensive understanding of hypothesis testing, check out this statistics hypothesis testing cheat sheet.
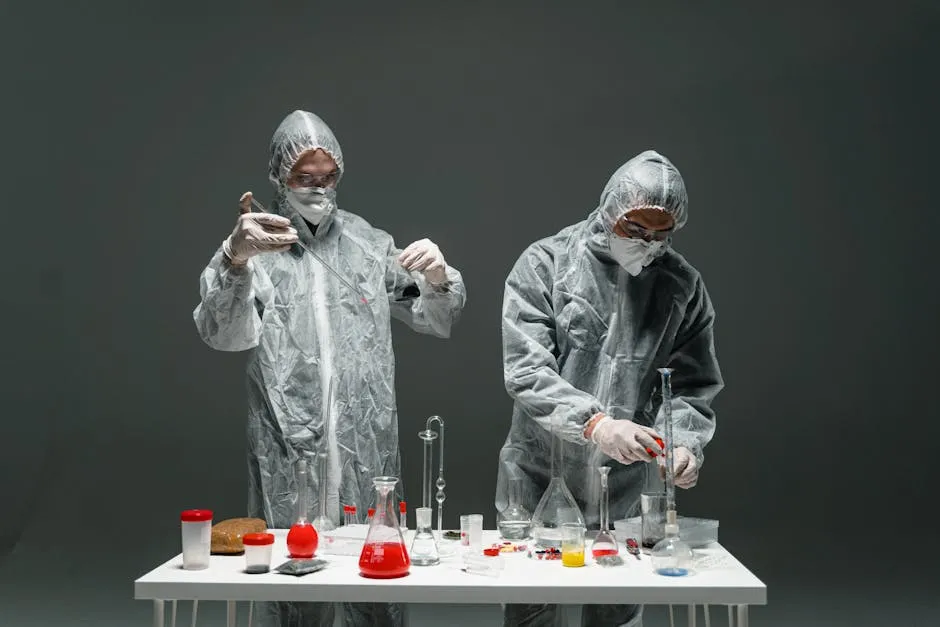
Types of Errors in Decision Making
Now, let’s talk about the not-so-glamorous side of hypothesis testing: errors. In decision making, we face two main culprits: Type I and Type II errors.
Type I error occurs when we reject a true null hypothesis. In simple terms, we believe we’ve found something significant when it’s just a mirage. It’s like calling someone out for cheating in a game when they were actually following the rules. Oops!
On the flip side, we have Type II error. This happens when we fail to reject a false null hypothesis. In this case, we miss a significant effect lurking in the shadows. Imagine a doctor who dismisses a patient’s symptoms, only to discover later that they actually had a serious condition. Yikes!
Both types of errors have implications in decision making. A Type I error could lead to unnecessary actions or interventions, while a Type II error might cause us to overlook critical insights. Knowing the balance between these errors is essential. Adjusting the alpha level can help manage the risk of these errors based on the context.
For example, in medical testing, a lower alpha level might be set to minimize Type I errors, ensuring that only proven treatments are approved. In contrast, a higher alpha may be acceptable in exploratory research, where discovering new relationships is the priority.
In conclusion, understanding hypothesis testing and the types of errors we face empowers us to make informed decisions. By carefully evaluating our data and recognizing potential pitfalls, we can sharpen our decision-making skills and improve outcomes across various fields.

Confidence Intervals
Confidence intervals are like the cozy blankets of statistics. They provide a range of values that likely contain a population parameter. Think of them as a safety net for decision-making. Instead of saying, “I think the average height of my friends is 5’8″,” you say, “I’m pretty sure the average height is between 5’7″ and 5’9″, with 95% confidence.
To calculate a confidence interval, you need three key ingredients: the sample mean, the standard deviation, and the sample size. The formula looks like this:
Confidence Interval = Mean ± ( z × σ/√n )
Here, z is the z-score corresponding to your desired confidence level (like 1.96 for 95%). The standard deviation σ measures the spread of your data, while n is the number of observations.
Let’s say you surveyed 100 people about their coffee consumption, finding an average of 3 cups a day with a standard deviation of 0.5 cups. Plugging these numbers into our formula, you’d find a confidence interval of approximately 2.9 to 3.1 cups. This means you can confidently say, “Most of my friends drink between 2.9 and 3.1 cups of coffee daily!”
Confidence intervals are vital when making decisions based on data. They help us understand the uncertainty surrounding estimates. In healthcare, for example, if a new drug is shown to reduce symptoms with a confidence interval of [10%, 20%], it indicates the treatment could reduce symptoms by as little as 10% or as much as 20%. This range helps doctors assess the drug’s effectiveness and decide whether to prescribe it.
Bayesian Decision Making
Bayesian decision making is like upgrading from a flip phone to a smartphone. It’s a fresh way to approach uncertainty by incorporating prior knowledge into decisions. Unlike traditional methods that rely solely on current data, Bayesian thinking allows you to update your beliefs as new information comes in.
Imagine you’re trying to guess the favorite ice cream flavor of your friends. If you know that last summer, 70% preferred chocolate, that’s your prior information. Now, if you conduct a survey this year and find 60% prefer chocolate, you can adjust your belief. The beauty of Bayesian decision making lies in this dynamic updating process.
In Bayesian decision making, probabilities are not fixed. They evolve as we gather more data. This flexibility makes it particularly useful in fields like medicine and finance, where conditions change rapidly.
For instance, consider a medical diagnosis. A doctor might start with a prior belief based on general statistics about a disease, such as a 10% prevalence rate. As they gather patient-specific information—like symptoms and test results—they update their beliefs. If a test yields a positive result, the Bayesian approach helps calculate the new probability of the disease, providing a clearer picture for treatment decisions.
Bayesian methods shine in various applications. In finance, they help investors adjust their portfolios based on market trends. In marketing, companies can refine their strategies by continuously updating customer preferences. This responsive nature makes Bayesian decision making a powerful tool across many disciplines.
By embracing Bayesian approaches, decision-makers can ensure their choices are informed by both past knowledge and current data, leading to better outcomes in uncertain environments.

Healthcare
Statistical decision making plays a pivotal role in healthcare, particularly in clinical trials and patient care. Think of clinical trials as the Olympics of medicine. Researchers test new treatments against placebos, seeking the gold medal of effective treatment. Here, statistics are the referees, ensuring only valid results win.
In clinical trials, statistical methods assess the effectiveness of treatments. They help determine if a new drug performs better than the standard one or if it’s just a placebo effect. Statistical analysis evaluates data from diverse patient groups, helping researchers understand treatment outcomes across populations. This ensures that findings are applicable to a wider audience, not just a select few.
Statistics also assist in patient care. For instance, doctors use statistical evidence to decide the best treatment options. They analyze data from previous cases, looking for patterns that suggest what works best for similar patients. This data-driven approach minimizes guesswork, ensuring patients receive tailored, effective care.
When assessing treatment effectiveness, statistics are indispensable. They help calculate success rates, side effects, and long-term outcomes. This information empowers healthcare professionals to make informed choices, ultimately leading to improved patient outcomes. In a nutshell, statistics in healthcare transform abstract data into actionable insights, paving the way for better health decisions. If you’re interested in diving deeper into healthcare statistics, consider reading “Statistical Methods for the Social Sciences” by Alan Agresti.
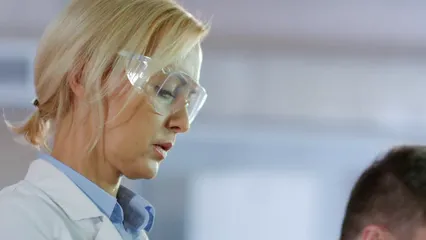
Public Policy
Statistical decision making is crucial in public policy. Governments rely heavily on data to shape policies that affect citizens’ lives. From health initiatives to education reforms, statistics inform nearly every decision made in the public sector.
For example, consider the implementation of a vaccination program. Policymakers gather data on disease prevalence, vaccine effectiveness, and population demographics. By analyzing this information, they can project the potential impact of the program on public health. This data-driven approach helps allocate resources effectively and ensures that policies are based on solid evidence rather than guesswork.
In education, statistics inform decisions about funding, curriculum changes, and student performance assessments. By analyzing test scores and graduation rates, policymakers can identify areas needing improvement. This analysis allows them to target resources where they will have the most significant impact.
Moreover, statistics play a vital role in evaluating the effectiveness of policies. After implementation, data helps assess whether the expected outcomes were achieved. If not, adjustments can be made to improve future initiatives. In summary, statistical decision making is the backbone of effective public policy, guiding leaders to make informed choices that benefit society.

Risk Management
Risk management is another area where statistical decision making shines. Organizations across industries use statistics to identify, assess, and mitigate risks. Whether in finance or insurance, data analysis is key to making informed decisions.
In finance, statistical models help predict market trends. Investors rely on these analyses to make decisions about buying or selling assets. By examining historical data and market behavior, financial analysts can estimate potential risks and returns. This allows them to create portfolios that balance risk and reward.
Insurance companies also heavily depend on statistical decision making. They analyze data to determine policy premiums and assess claims. For instance, actuaries use statistical models to estimate the likelihood of certain events, like accidents or natural disasters. This helps insurers set premiums that reflect the actual risk, ensuring they remain solvent while providing coverage.
Real-world examples abound. Consider how a bank uses statistical analysis to assess loan applications. By evaluating various factors—such as credit scores, income levels, and economic trends—they can determine the likelihood of repayment. This data-driven approach minimizes the risk of defaults.
In summary, statistical decision making is crucial for effective risk management. By harnessing the power of data, organizations can make informed decisions that safeguard their interests and promote stability in their respective industries.
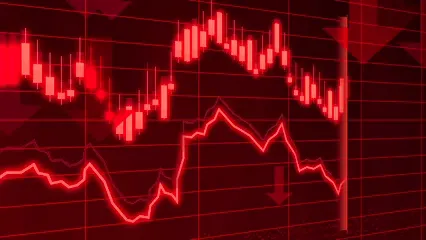
Challenges in Statistical Decision Making
Data Quality and Reliability
Data quality significantly impacts statistical decision making. Poor-quality data can lead to erroneous conclusions, affecting critical decisions. Think of data quality as the fuel for a car; without high-quality fuel, performance suffers. Inaccurate or unreliable data can distort findings and misguide decisions.
Common issues affecting data quality include bias, outliers, and missing data. Bias occurs when the data collection process favors certain outcomes over others. For instance, if a survey only includes responses from one demographic, the results may not represent the entire population. This can lead to skewed interpretations and flawed decisions.
Outliers also pose a challenge. These extreme values can disproportionately influence statistical analyses, potentially leading to misleading results. Imagine a restaurant’s average meal price being skewed by a few exorbitantly priced dishes. Excluding outliers is often necessary to obtain a more accurate picture.
Missing data is another hurdle. Incomplete datasets can compromise the integrity of statistical analyses. For instance, if a clinical trial reports only partial results, drawing conclusions about a treatment’s effectiveness becomes problematic. Researchers must employ various techniques, such as imputation or sensitivity analysis, to address missing data.
Ensuring data quality is essential for sound decision making. Organizations must establish robust data collection processes, regularly audit their datasets, and remain vigilant against bias and outliers. By prioritizing data quality, decision-makers can improve the reliability of their analyses and ultimately enhance outcomes across various fields.
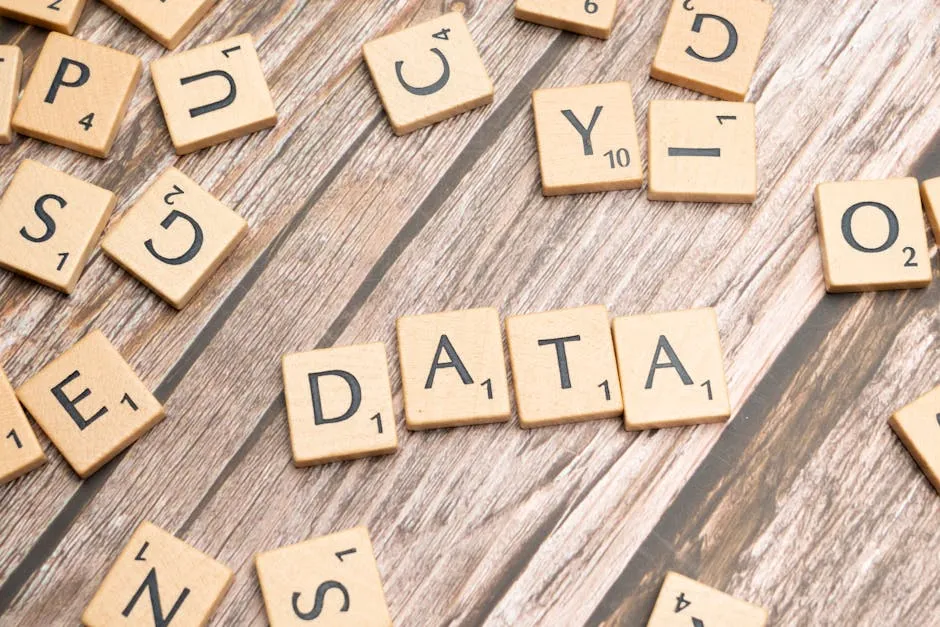
Misinterpretation of Data
Misinterpreting statistical results can lead to disastrous decisions. It’s like reading a recipe and mistaking teaspoons for tablespoons—your dish could end up inedible! One common pitfall is confusing correlation with causation. Just because two events occur simultaneously doesn’t mean one causes the other. For instance, ice cream sales and drowning incidents may rise in summer, but one doesn’t cause the other. To avoid this, always dig deeper into the data.
Another common mistake is neglecting sample size. Small samples can lead to skewed results. If you survey just ten people about their favorite ice cream, you might think chocolate is the clear winner. But what if those ten were all from the same neighborhood? A larger, more diverse sample gives a more reliable picture. Always ensure your sample size is adequate to draw conclusions.
Lastly, be wary of confirmation bias, where you seek data that supports your preconceived notions. This is like wearing rose-colored glasses—everything looks rosy! Instead, take a step back and consider all evidence, even if it contradicts your beliefs.
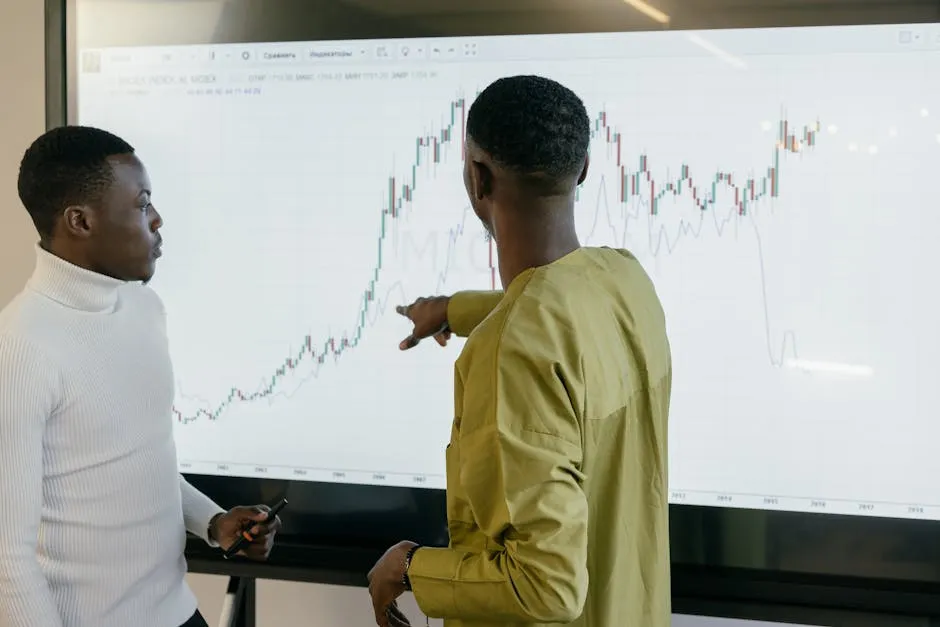
Ethical Considerations
Statistical decision making also carries ethical implications. When using data to inform choices, transparency and accountability are crucial. If a company manipulates data to make its product look better, it risks consumer trust and legal repercussions. Always strive for honesty in presenting statistical findings.
Moreover, consider the impact of your decisions on individuals and communities. For example, if a healthcare provider uses biased data to determine treatment protocols, it could harm marginalized groups. Ethical statistical practices demand that the data is representative and used responsibly.
Another important aspect is data privacy. As organizations collect more data, they must protect individuals’ information. Misusing or failing to safeguard personal data can lead to serious ethical breaches. In summary, ethical considerations in statistical decision making are paramount. Always prioritize transparency, representativeness, and data protection to foster trust and integrity in your analyses.

Future Trends in Statistical Decision Making
In the ever-evolving field of statistical decision making, emerging trends are reshaping the landscape. Big data, machine learning, and artificial intelligence (AI) are at the forefront of this transformation. These technologies enable organizations to analyze vast amounts of data quickly and efficiently, leading to more informed decisions.
Big data refers to the enormous volume of data generated every second. Companies can now harness this data for insights that were previously unattainable. Imagine a retailer analyzing customer purchase history in real-time to optimize inventory. This data-driven approach minimizes waste and maximizes sales.
Machine learning is another game changer. It allows systems to learn from data without explicit programming. For instance, algorithms can predict customer behavior based on past interactions. This predictive capability helps businesses tailor their marketing strategies, improving customer engagement.
AI further enhances statistical decision making by automating complex analyses. It can sift through data patterns that humans might overlook. For example, in healthcare, AI can analyze medical images faster and with greater accuracy than human radiologists. This leads to quicker diagnoses and better patient outcomes.
Moreover, these technologies promote real-time decision making. In industries like finance, rapid data analysis can mean the difference between profit and loss. Traders can react instantly to market changes, leveraging statistical models to make informed trades.
As we look ahead, the integration of ethics in these technological advancements will be crucial. Ensuring that AI and machine learning systems are free from bias is vital. Organizations must invest in training their algorithms with diverse datasets to promote fairness.
In conclusion, the future of statistical decision making lies in the synergy between big data, machine learning, and AI. These technologies will empower organizations to make faster, more accurate decisions. However, ethical considerations must remain at the forefront to ensure that these advancements benefit everyone.

Conclusion
Statistical decision making is essential across numerous fields, from business to healthcare and public policy. It transforms ambiguity into clarity, allowing individuals and organizations to make informed choices rooted in data. By utilizing robust statistical analysis, we can navigate the complexities of our world more effectively.
In business, statistical decision making enables companies to understand customer behavior. In healthcare, it drives evidence-based practices that improve patient outcomes. Public policy relies on statistical insights to shape laws and regulations that affect societal welfare.
However, the importance of ethical practices cannot be overstated. Transparency and accountability in data usage ensure that decisions are fair and just. Moreover, understanding the limitations of statistical analysis, such as potential misinterpretations, is vital for sound decision making.
If you’re keen on understanding how to apply statistical techniques in business, “Data Science for Business: What You Need to Know about Data Mining and Data-Analytic Thinking” by Foster Provost is a must-read. You can find it here.
As we embrace statistical tools and methodologies, we empower ourselves to make choices that enhance our personal and professional lives. Let’s champion data-driven decision making and continue to strive for a world where informed choices lead to positive outcomes for all.
FAQs
What is the difference between descriptive and inferential statistics?
Descriptive statistics summarize data from a sample. They provide insights, like averages or percentages, to help understand the data set’s key characteristics. For example, the average test score of a class is a descriptive statistic. Inferential statistics, on the other hand, allow us to make predictions or inferences about a larger population based on a sample. For instance, if you survey 100 voters to predict an election outcome, you’re using inferential statistics. This method helps estimate broader trends using data from a smaller group. In essence, descriptive statistics describe what’s happening in your data, while inferential statistics help you make predictions or generalizations beyond the sample.
Please let us know what you think about our content by leaving a comment down below!
Thank you for reading till here 🙂
All images from Pexels