Introduction
Complex systems are everywhere! Think of a bustling city, a diverse ecosystem, or even your favorite social media platform. Each of these has numerous interacting components, making them intricate and fascinating. But what exactly defines a complex system? At its core, a complex system consists of many elements that interact in non-linear ways, leading to unpredictable outcomes. These systems often display emergent properties, meaning that the whole is greater than the sum of its parts. For instance, the behavior of a flock of birds cannot be understood just by analyzing individual birds. Instead, it emerges from the interactions among them. Statistical laws play a crucial role in unraveling the mysteries of complex systems. They help scientists and researchers understand patterns and regularities that arise within these intricate networks. From predicting earthquake magnitudes with the Gutenberg-Richter law to examining word frequencies through Zipf’s law, statistical laws provide essential insights across various fields, including physics, biology, and social sciences. Their importance cannot be overstated—these laws enable us to make sense of seemingly chaotic phenomena. This article aims to provide a comprehensive overview of statistical laws in complex systems. Readers will find insights into specific laws, such as Zipf’s law and the Gutenberg-Richter law, along with their applications in real-world scenarios. By the end of this read, you’ll not only grasp the significance of these laws but also appreciate their impact on understanding the complexities of our world. So buckle up and get ready to embark on a journey through statistics and complexity!
Understanding Complex Systems
Definition and Characteristics
Let’s dive deeper into what makes complex systems tick! A complex system is defined by its many components and their interactions. Take a look at our weather systems. They consist of countless variables—temperature, humidity, wind speed, and more—all influencing each other. This interconnectedness creates unpredictable weather patterns, illustrating the essence of a complex system. Emergence is another characteristic that sets complex systems apart. This phenomenon occurs when simple interactions among components lead to complex behavior. Consider traffic flow on a highway. Each driver’s decision may seem simple, but collectively, they create traffic jams or smooth sailing. The behavior of the entire system emerges from individual actions. Complex systems can be found in various domains. In biology, ecosystems demonstrate how species interact, compete, and coexist. In social sciences, human networks reveal intricate relationships among individuals. Even the internet, with its myriad connections, serves as a prime example of a complex system. And speaking of complex systems, if you’re looking for a deep dive into this topic, grab a copy of Complexity: A Guided Tour by Melanie Mitchell. It’s a fantastic read that will expand your understanding of these intricate systems!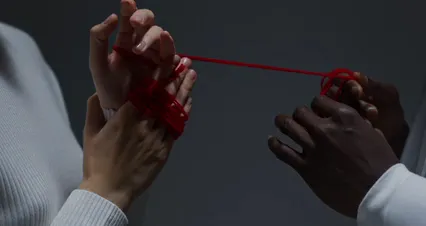
Interconnectedness and Emergence
Interconnectedness is the lifeblood of complex systems. Each component influences others, creating a web of relationships. This dynamic interplay is what gives rise to emergent properties. For instance, in an ecosystem, the decline of a single species can ripple through the food chain, affecting predators and prey alike. Emergence challenges our traditional understanding of cause and effect. Instead of linear relationships, complex systems operate on feedback loops, where outcomes can influence inputs. This non-linear behavior complicates predictions and requires sophisticated analytical methods to understand fully.Historical Context
The study of complex systems has evolved significantly over the decades. Initially, researchers focused on linear systems, believing that simpler models could explain most phenomena. However, as scientists began to uncover the complexities in nature, they started to develop theories that addressed the unique challenges posed by complex systems. Key figures in the development of complex systems theory include pioneers like Norbert Wiener, who introduced cybernetics, and Ilya Prigogine, known for his work on dissipative structures. Their contributions laid the groundwork for modern complexity science, which continues to flourish today. If you’re curious about the intersection of complexity and science, consider checking out The Structure of Scientific Revolutions by Thomas S. Kuhn. This book offers a profound perspective on how scientific paradigms shift, akin to the complexities we explore here. In conclusion, complex systems are characterized by numerous interacting components and emergent properties, making them a fascinating subject of study. Understanding these systems’ interconnectedness and historical context enriches our grasp of the statistical laws governing them. As we move forward, we will explore the specific statistical laws that help illuminate the intricacies of these systems.
Statistical Laws in Complex Systems
Overview of Statistical Laws
Statistical laws are fascinating! They describe regular patterns observable in various scientific domains. These laws provide insights into the behavior of complex systems, which are filled with numerous interacting components. Understanding these patterns is essential for unraveling the complexities of everything from ecosystems to social networks. If you’re interested in a thorough guide to these concepts, consider picking up The Art of Statistics: Learning from Data by David Spiegelhalter. This book is a game-changer for anyone wanting to grasp data analysis in a fun way! Statistical laws matter because they help us predict and understand behaviors in complex systems. For instance, they can forecast the likelihood of events, such as earthquakes, based on historical data. Without these laws, we would be lost in a sea of chaos, struggling to make sense of intricate relationships.
Key Statistical Laws
Zipf’s Law
Zipf’s Law is a gem! It states that in any given dataset, the frequency of any item is inversely proportional to its rank. In simpler terms, if you rank words by usage frequency, the second most common word will appear half as often as the first, the third a third as often, and so on. This law is crucial in linguistics and social sciences. If you want a deeper understanding, check out The Wisdom of Crowds by James Surowiecki. It explores how collective intelligence can often outsmart individual experts! Imagine language! The word “the” reigns supreme, appearing far more often than “zebra.” This distribution is not just a quirk of English; it applies to languages worldwide. Zipf’s Law helps researchers analyze text data, revealing patterns in communication and even shaping natural language processing tools.Understanding Zipf’s Law is essential for grasping the statistical laws in complex systems. statistical laws in complex systems

Gutenberg-Richter Law
Next up, we have the Gutenberg-Richter Law. This law is a cornerstone in seismology. It posits a relationship between the magnitude of earthquakes and their frequency. Simply put, smaller quakes are far more common than massive ones. Understanding this law is vital for predicting seismic activity in regions prone to earthquakes. If you’re interested in a comprehensive guide on the topic, consider reading Data Analysis for Complex Systems by J. D. Hunter. It’s a fantastic resource to enhance your understanding of data within complex frameworks! For instance, if you live in California, this law provides insights into the likelihood of experiencing various magnitudes of earthquakes. It helps scientists and emergency planners gauge risk levels, ensuring communities are prepared for potential disasters.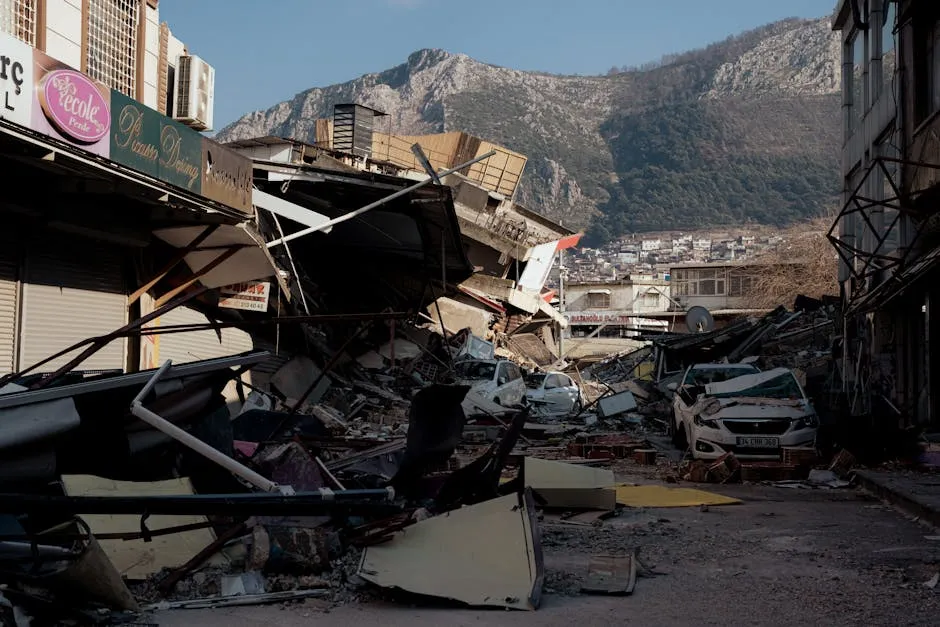
Kleiber’s Law
Kleiber’s Law shifts the focus to biology! This law states that an organism’s metabolic rate scales to the 3/4 power of its mass. In layman’s terms, larger animals typically use energy more efficiently than smaller ones. This principle has profound implications for ecology and evolutionary biology. If you’re intrigued by animal behavior and biology, grab a copy of The Selfish Gene by Richard Dawkins. It’s a thought-provoking read that will change the way you think about evolution! Consider the elephant and the mouse. An elephant’s metabolic processes are slower per unit of mass compared to a mouse. Understanding this scaling law helps ecologists predict how energy flows through ecosystems, allowing for better modeling of species interactions and population dynamics.
Urban Scaling Laws
Urban Scaling Laws are a modern twist on statistical laws! They examine how city productivity scales with size. Larger cities often exhibit unique patterns in social, economic, and infrastructure metrics. The productivity of a city tends to increase at a greater rate than its population. If you’re curious about the future of cities, check out The Fourth Industrial Revolution by Klaus Schwab. It offers insights into how technology is shaping our urban environments! Take New York City, for example. With its bustling economy, cultural institutions, and innovative industries, it outshines smaller towns in many metrics. Urban Scaling Laws help urban planners and policymakers understand growth patterns, improve infrastructure, and enhance the quality of urban life.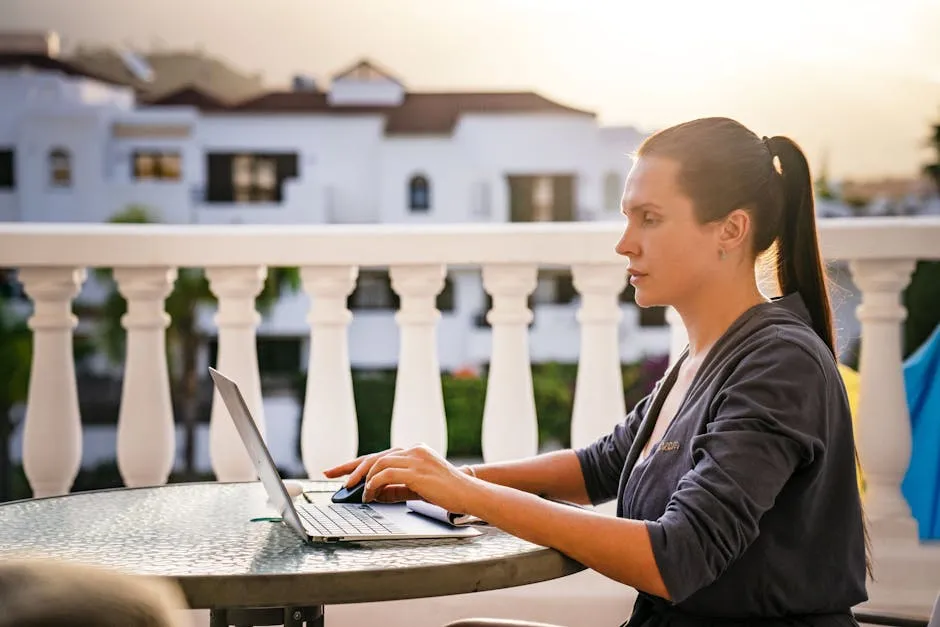
The Role of Data Analysis in Testing Statistical Laws
Understanding statistical laws in complex systems is akin to trying to read a map in a funhouse—everything shifts and bends, making it tricky to find your way. But fear not! Data analysis is here to rescue us from the maze. It helps us test these laws, revealing the underlying patterns that govern our chaotic world. Let’s break it down.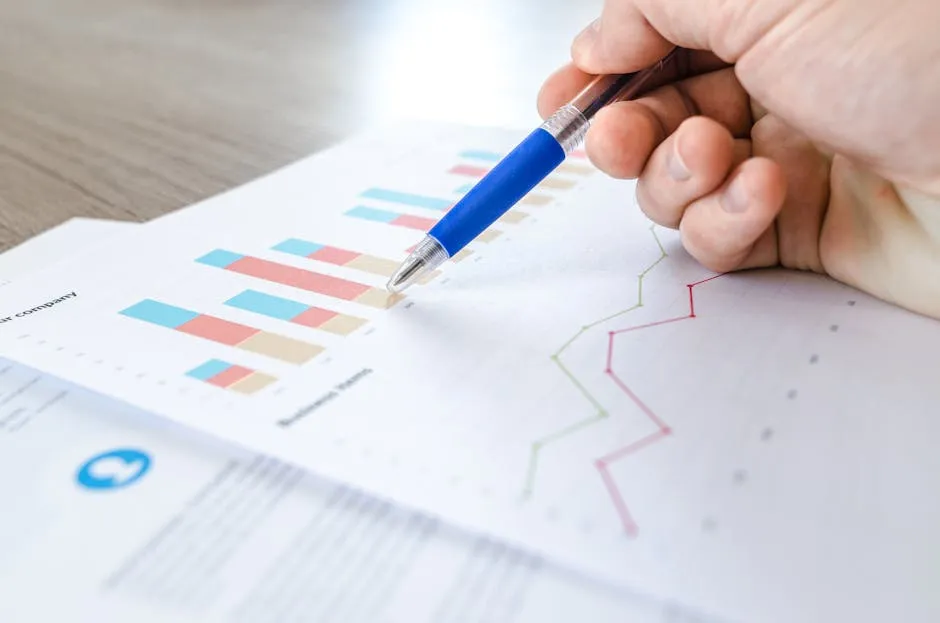
Methods Used
Traditional vs. Modern Methods Traditional statistical methods are like that old-fashioned map—reliable but sometimes limited. They often assume that data points are independent and identically distributed (i.i.d.). However, in complex systems, this assumption frequently falls flat. These systems are interconnected, where one data point can influence another. For instance, if we analyze social networks, a person’s connections can dramatically alter their behavior. Traditional methods might yield misleading results due to overlooked correlations. If you’re interested in learning more about data science methods, consider The Data Science Handbook by Field Cady. It’s a great resource for diving into the world of data analysis! Enter modern statistical methods! These approaches are like upgrading to GPS navigation. They recognize and account for correlations within data. Techniques such as maximum likelihood estimation have evolved, allowing researchers to test statistical laws with greater accuracy. For example, Martin Gerlach and Eduardo Altmann proposed a method that adjusts for correlations by shuffling and under-sampling data. This approach reduces false rejections of statistical laws, providing more reliable results. Moreover, modern methods often incorporate mechanistic models. These models simulate the processes generating the data, offering insights into how statistical patterns emerge. This integration of theory and data analysis creates a more comprehensive understanding of complex systems.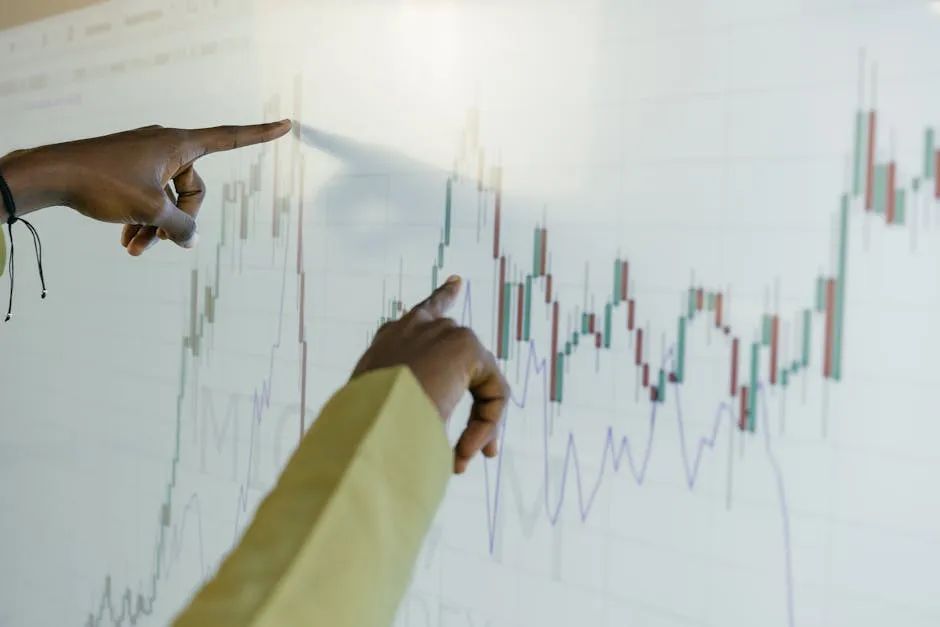
Case Studies
Example Analyses Let’s spice things up with some real-world examples! One notable case study involves the Gutenberg-Richter law, which relates to earthquake magnitudes and their frequencies. Researchers analyzed extensive earthquake datasets from California. Using traditional methods, they faced challenges due to data correlations. They often misidentified the relationship between magnitude and frequency, leading to erroneous conclusions. By applying modern techniques, they discovered that smaller earthquakes are far more common than larger ones. This insight is crucial for disaster preparedness and urban planning. Imagine the difference between a city that knows its risks and one that flies blind! If you’re curious about the data behind such analyses, consider checking out The Data Warehouse Toolkit: The Definitive Guide to Dimensional Modeling by Ralph Kimball. It’s a must-read for those interested in data management! Another intriguing case is Zipf’s law, which examines word frequencies in texts. Researchers analyzed vast corpuses, like the Google Books dataset. Traditional methods struggled with the inherent correlations among words. However, by employing modern statistical techniques, they revealed that the frequency of a word is inversely proportional to its rank. This finding not only enhances our understanding of language but also informs fields like natural language processing. In both examples, the shift from traditional to modern methods made a significant difference in outcomes. The ability to account for correlations led to more accurate interpretations and insights.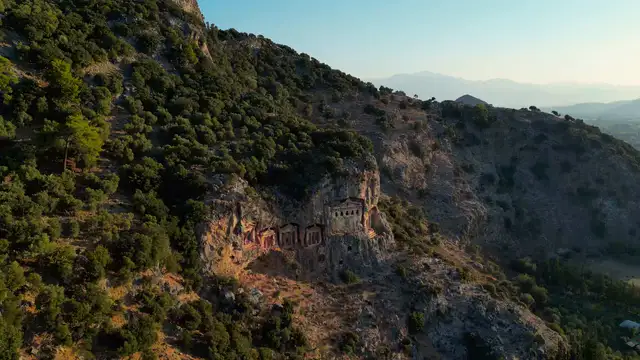
Controversies and Challenges
Validity Issues
Debates in the Scientific Community Despite advancements, debates about the validity of certain statistical laws persist. Critics argue that some laws lack robust empirical support. For instance, while Zipf’s law enjoys widespread acceptance, skeptics question its universality. They point to instances where the law appears to break down, particularly in niche datasets. Additionally, the scientific community often grapples with differing interpretations of statistical laws. Some researchers emphasize empirical validation, while others prioritize theoretical foundations. This friction sparks lively discussions and, at times, heated debates. Nonetheless, these conversations drive the field forward, encouraging deeper exploration.
Data Correlations
Correlations can be a double-edged sword in statistical analyses. On one hand, they provide valuable insights into relationships between variables. On the other hand, they can lead to false conclusions if not properly accounted for. For instance, a strong correlation between ice cream sales and drowning incidents doesn’t imply that one causes the other. Instead, both may be influenced by a third variable—hot weather. In the context of complex systems, overlooking correlations can result in misguided interpretations of statistical laws. Researchers must tread carefully, ensuring that their analyses account for the intricate web of interactions present in real-world data.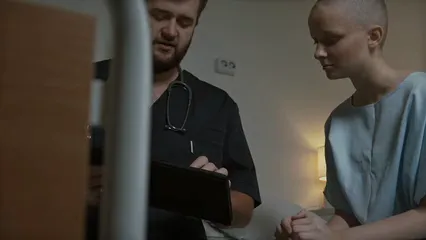
Methodological Challenges
Sampling Biases Sampling biases are another hurdle researchers face when testing statistical laws. When a sample isn’t representative of the larger population, the results can be skewed. For instance, if researchers analyze a dataset comprising only urban areas, they may draw incorrect conclusions about trends applicable to rural regions. To combat this, researchers must adopt robust sampling techniques. Random sampling and stratified sampling can help ensure that the sample reflects the population’s diversity. This practice enhances the reliability of findings and strengthens the overall analysis. Misinterpretation of Results Misinterpretation of results is a common pitfall in statistical analysis. Researchers may misread confidence intervals or p-values, leading to overstated claims. For example, a statistically significant result doesn’t necessarily imply practical significance. A tiny effect size can appear significant with a large sample, but that doesn’t mean it has meaningful implications. To mitigate this, researchers should focus on clear communication of results. Providing context and emphasizing practical relevance can help bridge the gap between statistical significance and real-world impact. In conclusion, data analysis plays a pivotal role in testing statistical laws in complex systems. By embracing modern methods, researchers can navigate the intricacies of data correlations and sampling biases. Although challenges persist, the journey toward understanding these laws is essential for grasping the complexities of our world. With each study, we edge closer to unveiling the hidden patterns that govern our reality!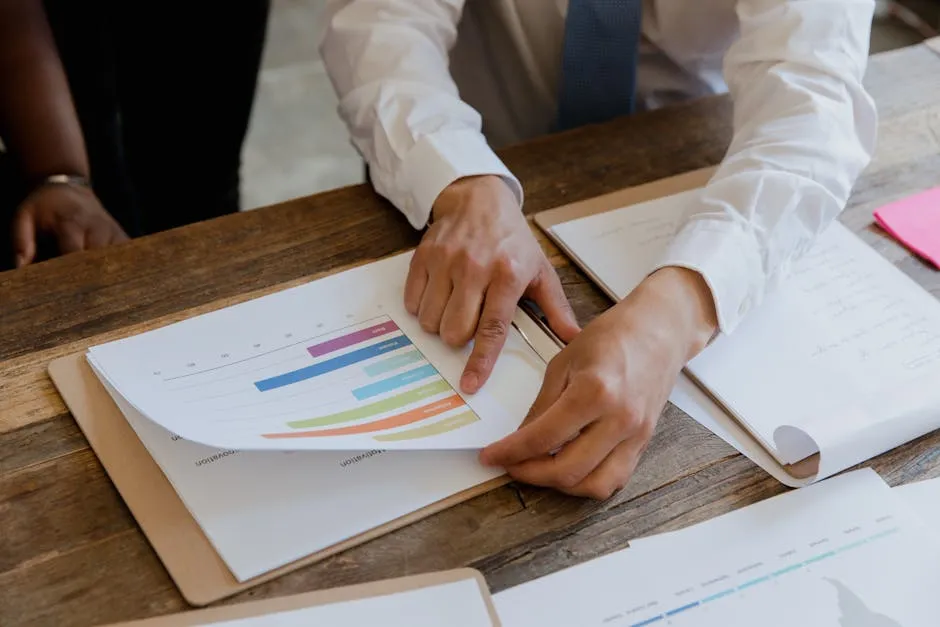
Future Directions in Research
Emerging Trends
The study of statistical laws in complex systems is evolving. Researchers are increasingly recognizing the importance of machine learning and artificial intelligence in analyzing vast datasets. These technologies can uncover patterns that traditional methods might miss. For example, advanced algorithms can detect correlations in data that were once considered noise. This shift enables scientists to refine existing statistical laws, leading to new insights. If you’re interested in the intersection of AI and data, consider picking up The Data Science Book: 50 Essential Concepts by David L. Smith. It provides a fantastic overview of key concepts in data science! Another emerging trend is the focus on real-time data analysis. As technology advances, researchers can now utilize streaming data to observe complex systems as they evolve. This ability allows for more dynamic modeling and better predictions. Imagine predicting traffic patterns in real time! By leveraging statistical laws, urban planners can make informed decisions that improve city infrastructure. Finally, interdisciplinary approaches are gaining traction. Collaboration between fields like biology, physics, and social sciences has never been more crucial. By merging insights from different disciplines, researchers can develop comprehensive models that capture the intricacies of complex systems. This trend not only enhances understanding but also fosters innovation.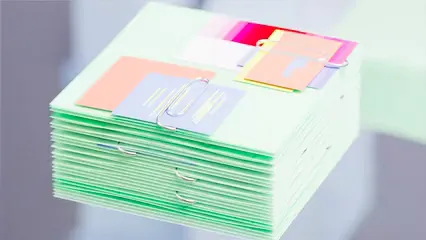
Interdisciplinary Approaches
Interdisciplinary collaboration is essential for advancing research on statistical laws in complex systems. The challenges posed by these systems often exceed the capabilities of any single discipline. For instance, statistical analysis in seismology benefits from insights in physics and geology. Combining these fields leads to a more comprehensive understanding of earthquake patterns through the Gutenberg-Richter law. Moreover, the integration of social sciences enhances our grasp of human behavior in complex networks. Understanding how people interact can illuminate patterns in data, such as social media usage or economic trends. By bridging gaps between disciplines, researchers can tap into a wealth of knowledge that enriches their analyses. Universities and research institutions are beginning to embrace this interdisciplinary approach. They are creating dedicated centers that focus on complex systems. These centers facilitate collaboration and encourage researchers from diverse backgrounds to come together. Such environments foster innovation and spark new ideas, ultimately leading to breakthroughs in understanding statistical laws. In conclusion, the future of research on statistical laws in complex systems is bright. Emerging trends in technology and interdisciplinary collaboration pave the way for deeper insights and innovative solutions to complex problems. As we continue to explore these avenues, we will undoubtedly unlock new understanding that enhances our grasp of the intricate systems that surround us. If you want to explore more about complex systems, consider reading The Emergence of Everything: How the World Became Complex by Harold J. Morowitz. It’s a fascinating exploration of how complexity arises in our world!FAQs
What are complex systems?
Complex systems consist of numerous interconnected components. These elements interact in ways that produce unpredictable outcomes. Think of a bustling city, where traffic, weather, and human behavior all play a role in daily life. Examples include ecosystems, social networks, and the internet. Each of these systems is a web of relationships, where small changes can lead to significant effects.
What are some common statistical laws?
Several statistical laws help us understand complex systems. Here are a few key ones: 1. **Zipf’s Law**: This law reveals that in any dataset, the frequency of an item decreases as its rank increases. For instance, the most common word in a language appears significantly more often than the second most common word. 2. **Gutenberg-Richter Law**: This law in seismology indicates that smaller earthquakes occur more frequently than larger ones. It helps researchers assess earthquake risks in various regions. 3. **Kleiber’s Law**: This law relates an organism’s metabolic rate to its mass, suggesting that larger animals use energy more efficiently than smaller ones. 4. **Urban Scaling Laws**: These laws explore how city productivity varies with size. Larger cities often exhibit increased efficiency and innovation compared to smaller towns.
How do statistical laws apply to real-world phenomena?
Statistical laws have widespread applications in various fields. In urban studies, they help city planners understand growth patterns and infrastructure needs. For example, urban scaling laws can inform policies to enhance public transportation in larger cities. In seismology, the Gutenberg-Richter law aids in earthquake preparedness, guiding construction codes and safety measures. Similarly, in biology, Kleiber’s law is essential for understanding energy dynamics in ecosystems, influencing conservation strategies.
What are the challenges in proving statistical laws?
Proving statistical laws presents several challenges. One major issue is the presence of correlations in data. Traditional methods often assume independence among data points, which can lead to inaccuracies. Researchers must develop new techniques to account for these correlations. Additionally, sampling biases can skew results. If a dataset does not accurately reflect the broader population, conclusions drawn may be misleading. Misinterpretation of statistical results also occurs, emphasizing the need for careful analysis and clear communication of findings.
What is the future of research on statistical laws in complex systems?
The future of research in this area looks promising. Emerging trends include leveraging machine learning to analyze large datasets for hidden patterns. This technology can help refine existing statistical laws and uncover new insights. Moreover, interdisciplinary collaboration is becoming increasingly crucial. By integrating knowledge from various fields, researchers can develop comprehensive models that capture the complexities of different systems. As technology advances and methodologies improve, we can expect a deeper understanding of the statistical laws that govern complex systems.
All images from Pexels