Introduction
Statistical Process Control (SPC) is a method used to monitor and control processes through statistical analysis. It involves collecting data and using control charts to identify variations in processes. By doing so, SPC helps organizations maintain quality and efficiency in manufacturing and service delivery.
In the world of quality control, SPC is crucial. It allows businesses to detect issues early, preventing defects and minimizing waste. Think of SPC as a watchful guardian, ensuring processes run smoothly and efficiently. If you want to get started with statistical analysis, consider using Minitab Statistical Software. It’s a powerful tool that can help streamline your SPC processes.

What is Statistical Process Control (SPC)?
Statistical Process Control (SPC) is a method used to monitor and control processes through statistical analysis. It involves collecting data and using control charts to identify variations in processes. By doing so, SPC helps organizations maintain quality and efficiency in manufacturing and service delivery.
Why Anomaly Detection Matters
Anomaly detection identifies unusual patterns in data. It’s significant because it helps organizations identify potential problems before they escalate. In the context of SPC, recognizing anomalies is essential for maintaining quality.
When SPC and anomaly detection work together, they create a powerful combination. SPC provides a framework for monitoring processes, while anomaly detection identifies deviations. This synergy allows organizations to act swiftly and effectively when issues arise. To dive deeper into the world of data analysis, check out Data Analysis for Business Decisions by David A. Hensher.
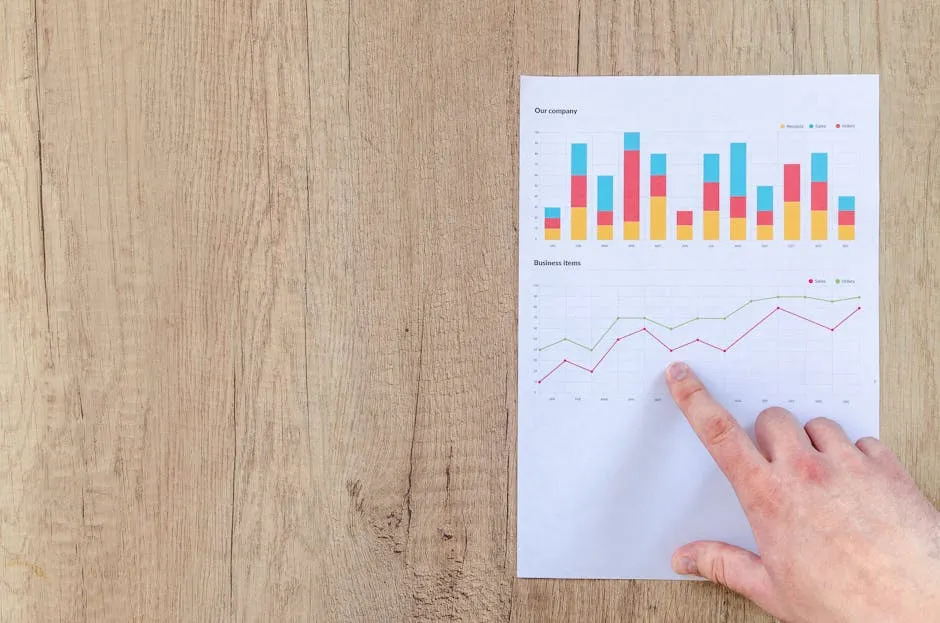
Overview of Reddit Discussions
Reddit offers a vibrant community where professionals and enthusiasts discuss SPC and anomaly detection. These discussions provide valuable insights, real-world examples, and practical advice. Users share experiences, challenges, and solutions, creating a rich repository of knowledge.
By tapping into these conversations, one can gain unique perspectives on SPC. The Reddit community serves as a treasure trove of insights for anyone looking to improve their understanding of anomaly detection and SPC practices. If you want to enhance your knowledge further, consider picking up a copy of Quality Control Handbook by Joseph M. Juran.
Understanding Statistical Process Control (SPC)
What is SPC?
Statistical Process Control dates back to the early 20th century, credited primarily to Walter A. Shewhart. It revolutionized quality control by introducing statistical methods to monitor processes. Today, SPC is widely used across industries, from manufacturing to healthcare.
Key components of SPC include control charts, process capability, and performance metrics. Control charts visually represent data over time, allowing operators to identify trends and variations. Process capability measures how well a process meets specifications, while performance metrics provide insight into overall efficiency. For those looking to deepen their understanding, The Lean Six Sigma Pocket Toolbook by Michael L. George is a fantastic resource.
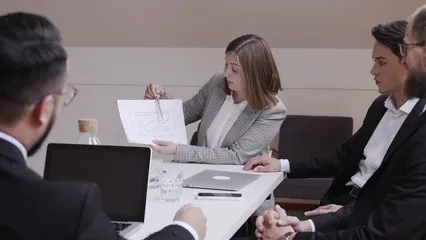
Types of Control Charts
X-bar and R Chart
X-bar and R charts are commonly used in SPC. The X-bar chart tracks the mean of a sample over time, while the R chart monitors the range within that sample. Together, they help identify shifts in the process. For example, in a manufacturing setting, these charts can detect variations in product dimensions.
P Chart and U Chart
P charts are used for monitoring the proportion of defective items in a sample. U charts, on the other hand, track the number of defects per unit. Both charts are essential for quality assurance in processes where defects can occur, such as in food production.
C Chart
C charts monitor the count of defects in a sample. They are particularly useful in settings where the number of opportunities for defects varies. For instance, in software development, tracking the number of bugs can help maintain quality. If you’re looking for a comprehensive guide to quality improvement, consider Statistical Methods for Quality Improvement by Thomas P. Ryan.
XMR Chart
The XMR chart is a unique tool for monitoring individual measurements and ranges. It’s particularly effective in processes with low volume and variability. This chart helps detect anomalies in real-time, making it invaluable for industries like healthcare, where precision is critical.
By understanding SPC and its applications, organizations can enhance their quality control measures. The combination of SPC and anomaly detection creates a robust framework for maintaining process integrity and quality assurance.
Importance of SPC in Anomaly Detection
Statistical Process Control (SPC) is like a trusty sidekick for anomaly detection. It helps organizations spot unusual patterns in their processes. By using data-driven techniques, SPC identifies variations that could signal underlying issues. Think of it as a vigilant watchdog, always on the lookout for something off.
SPC employs control charts to illustrate process behavior over time. These charts help visualize trends and fluctuations. When values stray outside of predetermined control limits, it raises a red flag. This way, companies can react quickly before minor hiccups turn into major disasters.
SPC’s significance spans various industries. In manufacturing, it ensures product quality. A classic example is an automotive assembly line. If a machine starts producing parts that deviate from specifications, SPC catches it early. This prevents defective products from reaching customers and saves on costly recalls. For those looking to understand the broader implications of data science in business, Data Science for Business by Foster Provost is a must-read.

Healthcare also benefits immensely from SPC. Hospitals use it to monitor patient care processes. For instance, tracking patient wait times can reveal inefficiencies. If wait times spike unexpectedly, administrators can investigate and address the problem before it affects patient satisfaction.
In the food industry, SPC is vital for maintaining safety standards. Manufacturers monitor critical control points, like cooking temperatures. If a temperature falls outside the safe range, they can take immediate action to prevent foodborne illness outbreaks.
In summary, SPC is essential for effective anomaly detection across multiple sectors. By identifying variations early, organizations can maintain quality, ensure safety, and enhance overall operational efficiency. So, whether it’s a factory or a hospital, SPC is there, keeping watch and ensuring processes run smoothly. If you’re interested in delving even deeper, consider The Art of Statistics: Learning from Data by David Spiegelhalter.
To learn more about effective data analysis techniques that can complement SPC, check out this article on tips for effective data analysis in economics and statistics.
Case Studies from Reddit Discussions
Reddit is a goldmine for discovering practical anomaly detection methods. Users frequently share their experiences and techniques that have proven effective in real-world scenarios. Here are some standout discussions where professionals exchanged valuable insights.
In one thread, a user named WignerVille suggested an easy anomaly detection method. They recommended calculating confidence intervals based on historical data. If current values fall outside these intervals, they’re flagged as anomalies. This straightforward approach can be adapted for various applications, making it a favorite among data enthusiasts.
Another user, amw5gster, emphasized the importance of searching for “statistical process control” when looking into anomaly detection methods. This term opens the door to a wealth of information and resources, helping users understand how SPC can aid in identifying anomalies effectively. For those who want to explore the technical side of data science, Understanding Machine Learning: From Theory to Algorithms by Shai Shalev-Shwartz is a great resource.

Additionally, a user shared their experience using z-scores to compare current values against the standard deviation of past values. This method allows for a nuanced understanding of how far a current observation strays from typical behavior. Such insights can lead to timely interventions before issues escalate.
These discussions highlight the community’s collective wisdom. By pooling experiences, Reddit users create a valuable resource for those looking to enhance their anomaly detection strategies. And for those who want to understand the broader implications of data management, check out The Data Warehouse Toolkit by Ralph Kimball.
Practical Applications of SPC and Anomaly Detection
Real-World Examples
Statistical Process Control (SPC) and anomaly detection play crucial roles across various industries. For example, in manufacturing, SPC helps maintain product quality. One Reddit user shared their experience with a production line for automotive parts. By implementing SPC, they detected deviations early, preventing costly defects and ensuring customer satisfaction.
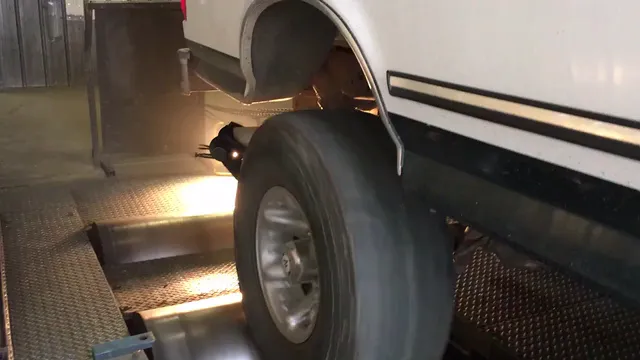
In healthcare, SPC is pivotal. Hospitals utilize it to monitor patient care processes. A user recounted how their facility tracked patient wait times using SPC. When wait times spiked unexpectedly, administrators investigated and resolved underlying issues, enhancing patient satisfaction. If you’re interested in improving your understanding of statistical quality control, consider Introduction to Statistical Quality Control by Douglas C. Montgomery.
The food industry also benefits from SPC. One Redditor described how a food manufacturer employed SPC to monitor cooking temperatures. When temperatures fell outside safe ranges, immediate corrective action was taken, preventing potential foodborne illnesses.
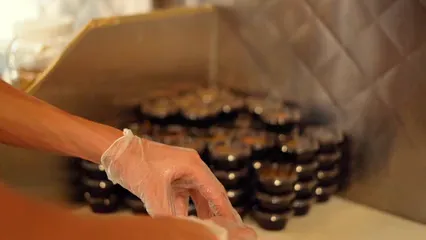
These examples demonstrate SPC’s versatility and effectiveness in real-world applications. The insights gleaned from user experiences on Reddit provide valuable lessons for those looking to implement SPC and anomaly detection in their organizations. If you’re keen on furthering your knowledge in data analysis, Practical Statistics for Data Scientists by Peter Bruce is a great choice.
Tools and Software for SPC
Implementing SPC requires the right tools. Several popular software options stand out in the market. Minitab is a renowned choice for statistical analysis and SPC implementation. It offers a user-friendly interface and robust features for creating control charts and analyzing process data.
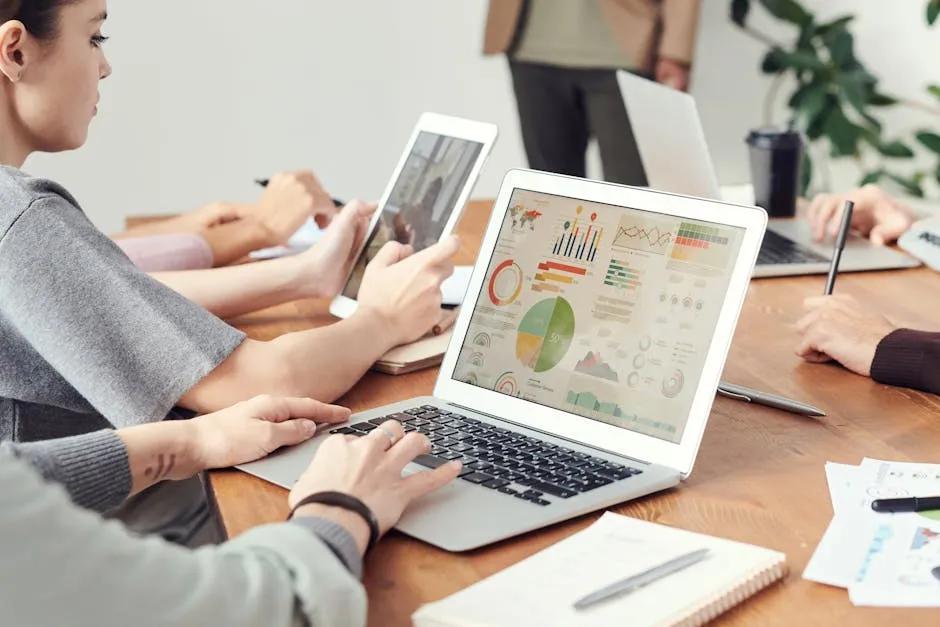
Another strong contender is JMP, which provides dynamic visualizations and interactive analyses. Users appreciate its ability to handle large datasets and its flexibility in creating customized reports. If you’re looking for a comprehensive guide on statistical software, consider Machine Learning for Dummies by John Paul Mueller.
Excel remains a go-to tool for many professionals. While not as specialized, it offers essential functionalities for SPC. Users can create control charts and conduct basic statistical analyses with ease.
Finally, R and Python have emerged as favorites among data scientists. These programming languages offer powerful libraries for statistical analysis and graphical representations. Reddit discussions often highlight users’ success in implementing SPC using these tools, showcasing their adaptability and effectiveness. For those interested in learning Python, check out The Data Science Design Manual by Steven T. Karris.
By evaluating various tools and software, professionals can select the best fit for their SPC needs. Each option provides unique features, making it easier to maintain quality control and enhance anomaly detection processes.
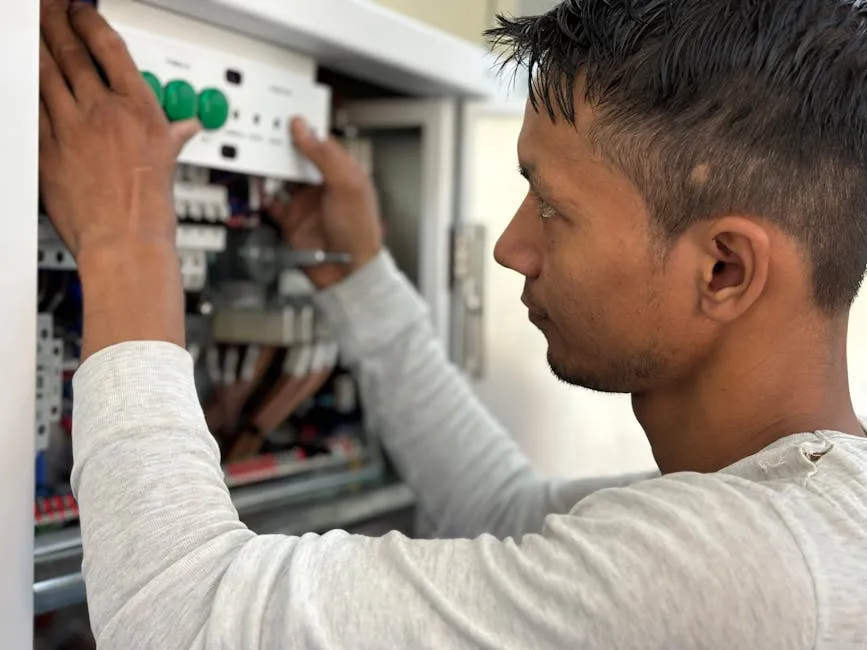
Future of SPC and Anomaly Detection
Trends in Statistical Process Control
Statistical Process Control (SPC) is evolving rapidly. Emerging technologies are reshaping how organizations monitor and control processes. One noteworthy trend is the integration of advanced analytics into SPC practices. Organizations now leverage big data analytics to gain insights from vast amounts of operational data. This approach enhances their ability to identify trends and predict anomalies before they escalate.
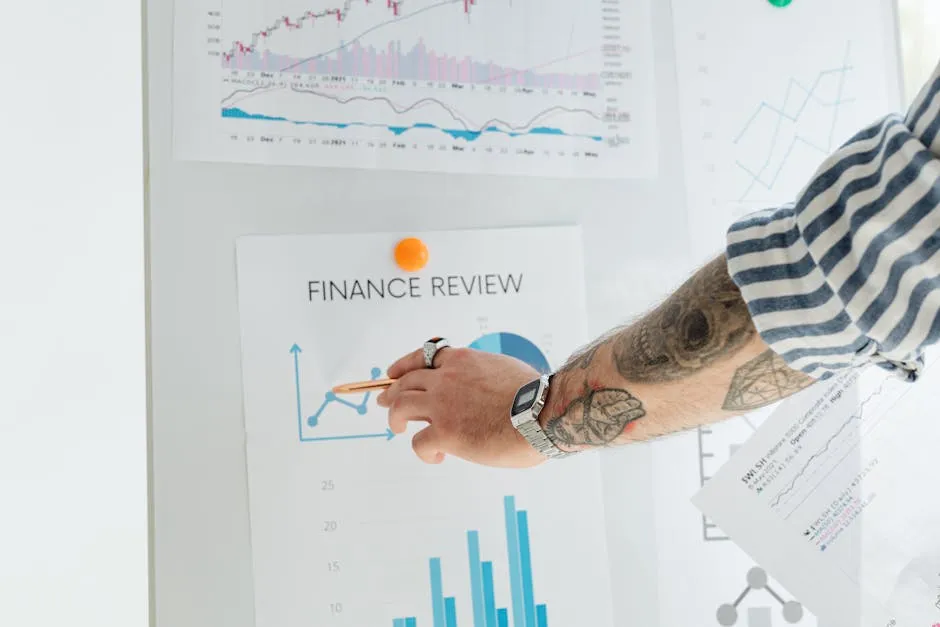
Artificial Intelligence (AI) and machine learning are at the forefront of these changes. These technologies are enhancing anomaly detection capabilities significantly. Machine learning algorithms can analyze historical data and learn from it, enabling them to identify patterns that might not be apparent to the human eye. For instance, a model trained on data from a manufacturing process can detect subtle shifts that indicate potential problems. If you want to delve deeper into machine learning principles, consider The Elements of Statistical Learning by Trevor Hastie.
Furthermore, real-time monitoring is becoming more common. Businesses can now use IoT (Internet of Things) devices to collect data in real time. This allows for immediate feedback and quicker responses to any issues that arise. As a result, companies can maintain higher quality standards and reduce waste, all while keeping costs in check.
Community Insights from Reddit
Reddit discussions provide valuable insights into the evolving landscape of SPC and anomaly detection. Users frequently share their experiences and perspectives on the future of these methodologies. For example, one user emphasized the importance of AI in anomaly detection. They noted that machine learning models can adapt and improve over time, making them more effective at identifying anomalies.

Another Redditor highlighted the significance of community-driven resources. They pointed out that searching for “statistical process control” can yield rich discussions filled with practical advice. Users often share techniques and tools that have proven successful in their environments. These conversations create a collaborative atmosphere where professionals can learn from each other’s successes and failures. If you’re eager to understand the latest trends in data science, consider reading The Big Book of Data Science by Matthew D. Rattner.
Additionally, users have expressed interest in the integration of SPC with other quality management frameworks. As companies seek to enhance their processes, blending SPC with methodologies like Six Sigma becomes increasingly popular. This combination allows organizations to leverage the strengths of both approaches, ultimately leading to more effective quality control strategies.
In summary, the future of SPC and anomaly detection is bright. With the incorporation of emerging technologies and insights from community discussions, organizations can continuously improve their processes and maintain high standards of quality. As professionals engage with each other on platforms like Reddit, they contribute to a growing body of knowledge that drives innovation in the field.
Conclusion
Statistical Process Control (SPC) is like the superhero of quality management. It plays a crucial role in detecting anomalies, ensuring processes run like a well-oiled machine. By applying SPC, organizations can catch deviations before they snowball into larger issues, saving time and resources. Imagine having a trusty sidekick that alerts you every time something goes amiss—this is what SPC does!
The beauty of SPC lies in its data-driven approach. Control charts and statistical methods help businesses visualize variations and identify trends. These tools empower organizations to maintain high-quality standards and enhance operational efficiency. Plus, the importance of anomaly detection in SPC cannot be overstated. It’s like having a magnifying glass that highlights the tiniest inconsistencies in a process. For a deeper dive into quality control methods, don’t miss out on Control Charting Techniques for Quality Control by William D. Wackerly.
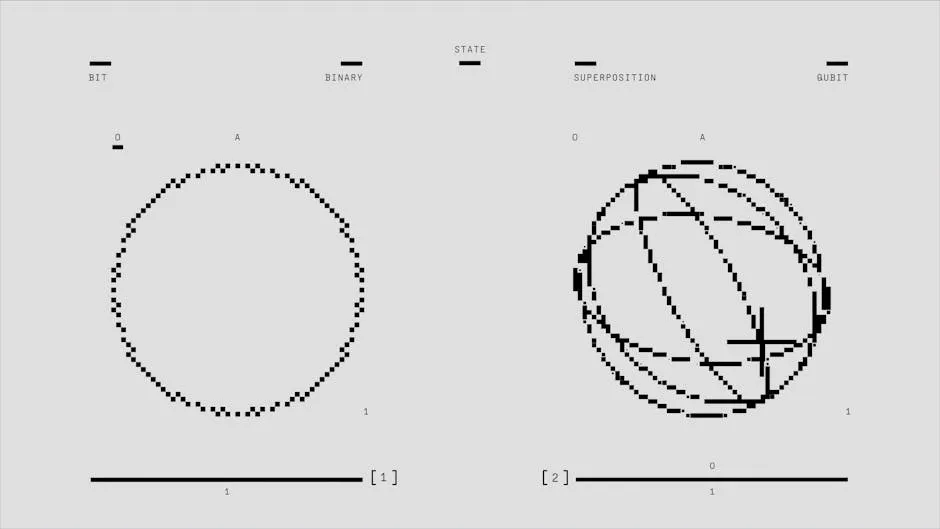
For those eager to learn more about SPC and anomaly detection, Reddit is a treasure trove of insights. Users share their experiences, offering practical advice and innovative techniques to tackle common challenges. Engaging with this community can provide you with fresh perspectives and ideas that might just revolutionize your approach to data analysis.
In conclusion, continuous learning is vital in the field of data analysis. As technology evolves, so should our understanding of methodologies like SPC. Staying updated with the latest trends and community discussions is key to maintaining a competitive edge. So, dive into those Reddit threads, soak up the knowledge, and become a master of SPC and anomaly detection!
FAQs
What is the difference between SPC and traditional quality control?
SPC differs from traditional quality control in its approach. While traditional quality control often relies on inspection after production, SPC emphasizes prevention through continuous monitoring and data analysis. SPC uses control charts to track process variations over time, allowing for real-time adjustments. This proactive approach helps identify issues before they escalate, leading to improved quality and efficiency. Essentially, SPC transforms quality control from a reactive measure into a dynamic, data-driven practice that enhances overall process performance.
How can I get started with implementing SPC in my organization?
Implementing SPC is simpler than you might think! Here’s a step-by-step guide to help you get started: 1. **Identify the Process**: Choose a process that impacts quality and efficiency. 2. **Collect Data**: Gather relevant data points to establish a baseline for your process. 3. **Select Control Charts**: Determine which control charts suit your needs (e.g., X-bar, R chart). 4. **Set Control Limits**: Calculate upper and lower control limits based on historical data. 5. **Monitor the Process**: Regularly analyze data using control charts to detect variations. 6. **Take Action**: If values go beyond control limits, investigate and implement corrective actions. 7. **Train Your Team**: Ensure your team understands SPC principles and how to use the tools effectively. By following these steps, you’ll be on your way to harnessing the power of SPC in no time!
What are some common challenges faced in anomaly detection?
Anomaly detection can be a tricky business! Here are some common challenges and how to overcome them, inspired by discussions on Reddit: 1. **Data Quality**: Poor-quality data can lead to false positives. Ensure you have clean, validated data before analysis. 2. **Threshold Setting**: Deciding where to set thresholds for anomalies can be subjective. Experiment with different methods, like z-scores or confidence intervals, to find what works best. 3. **Model Complexity**: Overly complex models may lead to overfitting. Start with simple models and gradually increase complexity as needed. 4. **Interpreting Results**: Anomalies can sometimes be misclassified. Collaborate with subject-matter experts to validate findings and make informed decisions. By addressing these challenges head-on, you’ll enhance your anomaly detection efforts and drive meaningful results.
Are there specific industries where SPC is especially useful?
Absolutely! SPC shines brightly in several industries, including: – **Manufacturing**: Essential for maintaining product quality and reducing waste. – **Healthcare**: Helps monitor patient care processes, ensuring optimal treatment and safety. – **Food Production**: Vital for adhering to safety standards and preventing foodborne illnesses. – **Finance**: Used in fraud detection and risk management to identify suspicious transactions. These industries benefit immensely from SPC practices, demonstrating its versatility and effectiveness in maintaining high-quality standards.
How do I interpret control charts effectively?
Interpreting control charts doesn’t have to be daunting! Here are some tips to make it easier: 1. **Understand the Basics**: Familiarize yourself with control limits, data points, and trends. 2. **Look for Patterns**: Regularly monitor charts for trends or shifts in data. 3. **Identify Outliers**: Pay attention to points outside control limits; these indicate potential anomalies. 4. **Use Rules of Thumb**: Apply common rules like the Western Electric rules to detect unusual patterns. 5. **Collaborate**: Engage with team members to discuss findings and gather different perspectives. By mastering control chart interpretation, you’ll be well-equipped to identify issues and maintain process quality effectively.
Please let us know what you think about our content by leaving a comment down below!
Thank you for reading till here 🙂
All images from Pexels