Introduction
Statistical significance and practical significance are two vital concepts in research and data analysis. They help us interpret findings correctly and apply them effectively. But what do they really mean? Statistical significance indicates that a result is unlikely to have occurred by chance. It’s like finding a four-leaf clover in a field of three-leaf ones. You think, “Wow, this might be something special!” Typically, we use p-values to determine statistical significance. If the p-value is below a certain threshold, often set at 0.05, we declare the result statistically significant. However, just because a result is statistically significant doesn’t mean it matters in real life. Enter practical significance, which tells us whether the observed effect is meaningful in a specific context. Imagine discovering that a new diet helps people lose an average of 0.1 kg. While statistically significant, does that weight loss really matter to anyone? For those looking to dive deeper into the fascinating world of statistics, consider picking up “The Art of Statistics: Learning from Data” by David Spiegelhalter. This book is a witty and insightful guide that will transform your understanding of data and its significance in everyday life. Misunderstandings abound when it comes to these terms. Many believe that statistical significance equates to practical importance, leading to confusion. We need clarity to make informed decisions based on research findings. In this article, we’ll break down the definitions of statistical and practical significance, provide examples, and discuss their implications across various fields. Understanding these concepts is crucial for responsible research interpretation. So, buckle up! We’re headed into the exciting world of significance testing, where numbers come alive and lead to real-world applications!
The Process of Hypothesis Testing
Null and Alternative Hypotheses At the heart of hypothesis testing lie two key concepts: the null hypothesis and the alternative hypothesis. The null hypothesis, often symbolized as H0, suggests that there is no effect or no difference in a given context. Think of it as the default position—the skeptic in the room saying, “Prove it!” On the other hand, the alternative hypothesis, represented as H1, posits that an effect or a difference does exist. It’s like the enthusiastic friend at a party, convinced that something exciting is happening. Researchers set out to gather evidence through data collection. They then analyze this data to see if they can reject the null hypothesis in favor of the alternative. If they find enough evidence, they celebrate with a statistical “high five.” If not, well, back to the drawing board! Understanding these hypotheses is crucial for interpreting the results of any statistical test.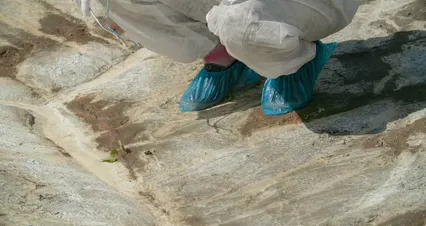
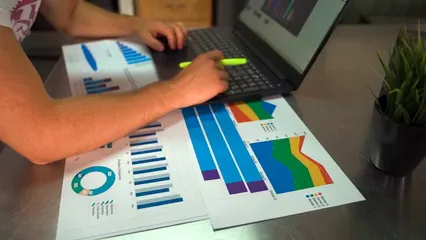
Key Points and Misconceptions
Common Misunderstandings A common pitfall is equating statistical significance with importance. Just because a study reports a statistically significant result doesn’t mean it’s meaningful in a practical sense. For instance, a study might find that a new training program improves performance by a statistically significant 0.5%. Sure, the p-value is below 0.05, but does that tiny improvement warrant the investment? Not always! It’s essential to assess the real-world relevance of the findings before jumping to conclusions. Understanding the differences between practical and statistical significance can enhance research quality. For more insights, refer to the article on practical significance versus statistical significance.Understanding the distinctions between practical and statistical significance can greatly impact research outcomes. practical significance versus statistical significance
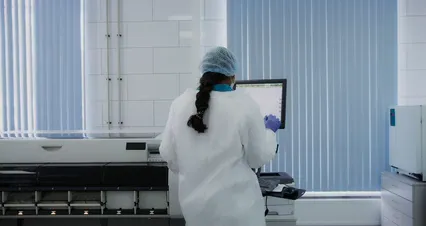

Measuring Practical Significance
Methods Assessing practical significance requires more than just statistical tests. Here are some reliable methods to gauge it effectively:- Cohen’s d: This metric calculates the effect size, indicating how large a difference is relative to variability in the data. For instance, a Cohen’s d of 0.8 suggests a large effect, while a value of 0.2 indicates a small effect. This helps researchers understand if the observed differences are meaningful.
- Confidence Intervals: These provide a range of values likely to contain the true population effect. For example, if a study finds that a new treatment improves outcomes with a 95% confidence interval of [2, 5], we can confidently say that the treatment has a meaningful positive impact.
- Qualitative Assessments: Sometimes numbers don’t tell the whole story. Qualitative insights from interviews or focus groups can reveal whether changes resonate with individuals involved. For example, in educational interventions, teachers’ opinions on new teaching methods can highlight their practical significance beyond mere data.
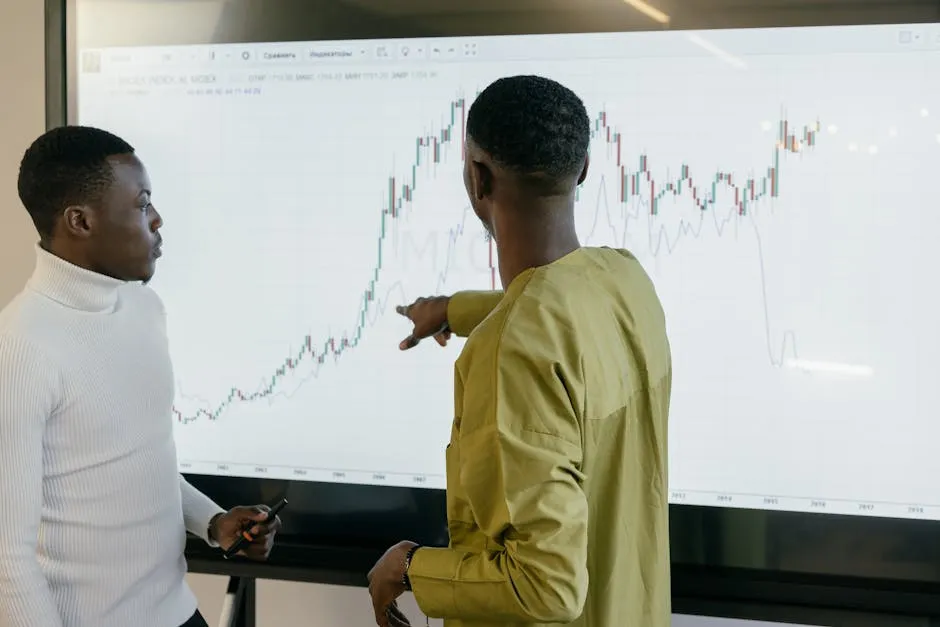
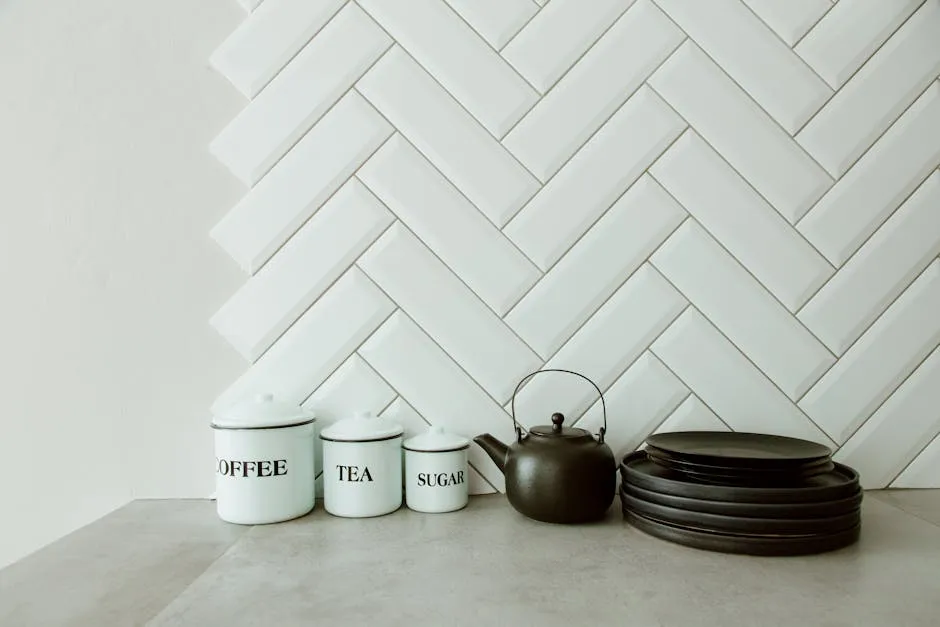
The Importance of Context
Field-Specific Relevance Practical significance varies greatly across fields. In healthcare, a small improvement in patient outcomes can be crucial. A 2% increase in survival rates from a new medication might be life-altering. Conversely, in marketing, a similar percentage change in consumer preferences might barely move the needle. Here, context matters immensely in assessing whether findings have practical implications. If you’re interested in understanding how data influences business decisions, check out “Data Science for Business: What You Need to Know about Data Mining and Data-Analytic Thinking” by Foster Provost. This book offers insights into how data analysis can drive business success.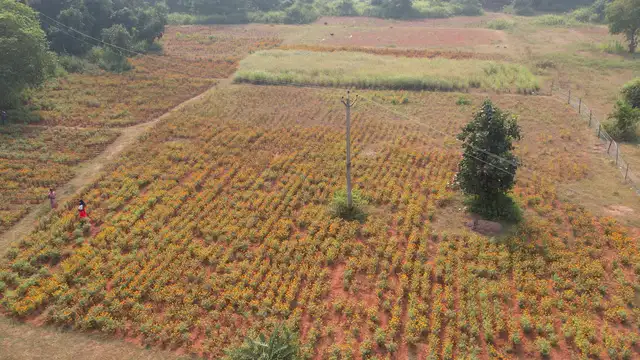
- Healthcare: A clinical trial for a new cancer drug shows a statistically significant 5% increase in survival rates. For oncologists, this is practically significant, potentially saving lives and reshaping treatment protocols.
- Marketing: A campaign shows a 3% increase in customer engagement. While statistically significant, this change may not justify the marketing budget spent. If the increase doesn’t translate to sales, its practical significance diminishes.
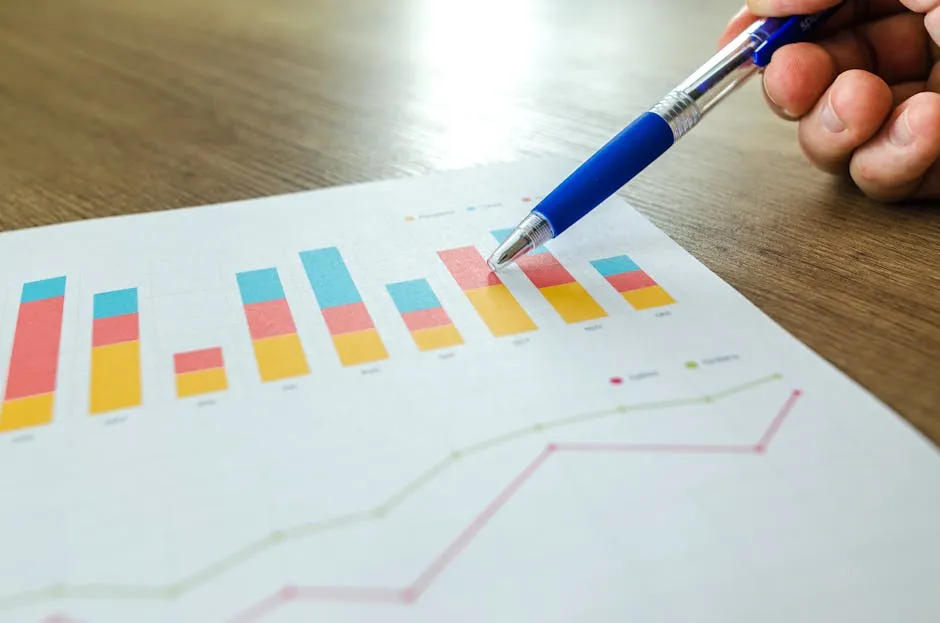
Decision-Making Implications
Understanding both statistical significance and practical significance can greatly influence decision-making in various fields, including policy, healthcare, and business. Imagine a government deciding to implement a new educational program based solely on a statistically significant finding. If the program shows a slight improvement in test scores but lacks practical significance, the investment might not yield meaningful benefits for students. In healthcare, a new treatment could demonstrate statistically significant results in clinical trials. However, if the actual health benefits are minimal, healthcare professionals must weigh the treatment’s practicality against its risks and costs. A 1% increase in recovery rates may sound promising statistically, but it might not justify the expense or side effects for patients. Businesses face similar dilemmas. A marketing campaign may achieve statistically significant engagement increases. However, if those increases translate to negligible sales growth, the campaign’s effectiveness is questionable. Businesses must ensure that their strategies yield not just significant data but also practical outcomes that resonate with their bottom line. In essence, decision-makers must look beyond the numbers. They need to ask, “Is this result not just statistically significant, but also practically meaningful?” By considering both aspects, organizations can make more informed, effective decisions that truly benefit stakeholders. For those looking to enhance their decision-making with the right tools, a Data Analysis Software can be a game-changer. It provides the means to analyze data effectively and make informed decisions based on solid evidence.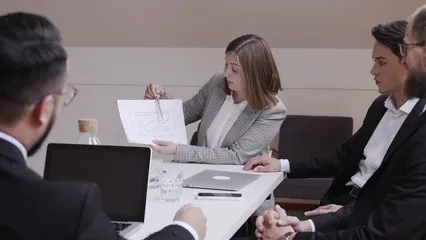
Common Pitfalls
Overreliance on Statistical Significance A common pitfall in research is the overemphasis on statistical significance. Researchers may celebrate a statistically significant finding without considering its practical implications. This can lead to misguided policies or investments. For example, a study might report a significant improvement in employee productivity due to a new management technique. But if the productivity increase is negligible in real terms, does it warrant the change? This overreliance can create a false sense of confidence. Just because a result passes the p-value threshold doesn’t mean it will translate into actionable insights. Organizations must remember that significance alone doesn’t guarantee relevance.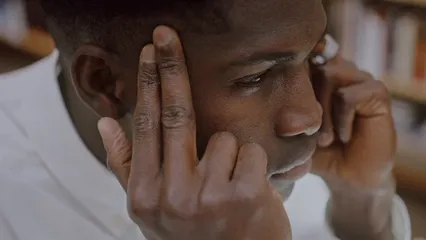

Reporting Standards
When it comes to reporting both statistical and practical significance in research publications, clarity is key. You don’t want your readers scratching their heads, wondering what the numbers mean. Here are some best practices to ensure your findings are communicated effectively. 1. Clearly Define Terms First and foremost, every study should define what statistical and practical significance mean in its context. Avoid jargon! Use clear, accessible language that a broad audience can understand. Readers should know what a p-value is, why it matters, and how it relates to practical implications.
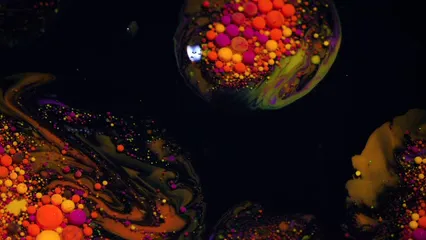

Conclusion
Understanding the difference between statistical significance and practical significance is crucial for effective research interpretation. Statistical significance tells us whether an effect exists, while practical significance reveals whether that effect matters in the real world. To recap, statistical significance focuses on p-values and hypothesis testing. It answers the question, “Is this result likely due to chance?” In contrast, practical significance assesses effect sizes and real-world implications. It asks, “Does this result matter?” Both concepts are essential for responsible research and effective decision-making. Relying solely on statistical significance can lead to misguided conclusions. For example, a study might find a statistically significant result that has no real-world applicability. Conversely, practical significance ensures that researchers consider the actual impact of their findings. In today’s data-driven environment, we need to bridge the gap between numbers and real-world implications. This means researchers must prioritize both statistical and practical significance in their work. Clear reporting and thoughtful interpretation can enhance the impact of research findings, guiding informed decisions across various fields. In conclusion, as you embark on your research adventures, remember the importance of both statistical and practical significance. They are not just academic concepts; they are vital tools for making meaningful contributions to knowledge and practice. So, let’s celebrate the numbers, but let’s also understand what they truly mean for the world around us.FAQs
What is the difference between statistical significance and practical significance?
Statistical significance indicates that a result is unlikely to have occurred by chance, typically measured by a p-value. Practical significance, on the other hand, assesses whether the result has real-world implications or is meaningful in a specific context.
Why is it important to consider practical significance?
Considering practical significance helps researchers and practitioners understand the real-world impact of their findings. A result may be statistically significant but lacks practical importance if its effect size is trivial.
How can I determine if a result is practically significant?
To assess practical significance, look at effect sizes and confidence intervals. If the effect size is large enough to make a difference in real-world applications, the result is practically significant.
What role does sample size play in statistical significance?
Sample size can greatly influence statistical significance. Larger samples increase the likelihood of detecting statistically significant results, even if the effect size is small. This can lead to significant findings that lack practical relevance.
Can a result be statistically significant but not practically significant? Provide an example.
Yes, it can. For instance, a study might find that a new drug lowers blood pressure by a statistically significant amount of 1 mmHg. However, if this reduction doesn’t lead to meaningful health improvements, it’s not practically significant.
Please let us know what you think about our content by leaving a comment down below!
Thank you for reading till here 🙂
All images from Pexels