Introduction
In the grand theater of research, two main characters often take center stage: statistical significance and practical significance. But what exactly do these terms mean, and why should we care?
Statistical significance refers to the likelihood that an observed effect in a study isn’t just a fluke. It’s all about the numbers. When researchers crunch the data, they often rely on p-values to determine whether their results are statistically significant. A p-value tells us the probability of obtaining the observed results, assuming that the null hypothesis (the idea that there’s no effect) is true. If this p-value falls below a predefined threshold—commonly set at 0.05—voilà! The effect is statistically significant!
On the flip side, we have practical significance. This is where the rubber meets the road, or, in research terms, where the data truly matters. Practical significance evaluates whether the size of the effect is meaningful in real-world scenarios. Just because a result is statistically significant doesn’t mean it has practical implications. For example, imagine a new medication that lowers blood pressure by a statistically significant amount. If the difference is only 0.1 mmHg, does that warrant a prescription? Probably not!
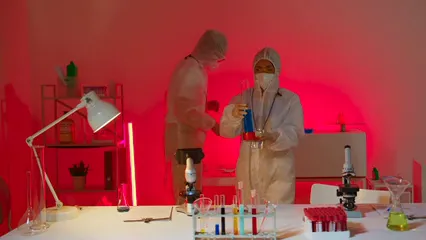
Understanding the difference between practical significance versus statistical significance is crucial for interpreting research findings effectively. Learn more about these key differences.
Understanding the distinction between these two concepts is crucial for anyone who interprets research findings. Misinterpretation can lead to misguided policies, flawed decision-making, and wasted resources. We’ll explore the nitty-gritty of statistical significance, its nuances, and why practical significance shouldn’t be ignored.
So, what can you expect as you read on? We’ll dive deeper into statistical significance, unravel its definition, and discuss the role of p-values and alpha levels. Plus, we’ll shine a light on common misconceptions and the factors that can skew our understanding of significance. By the end of this section, you’ll have a better grasp of these concepts, allowing you to navigate research findings with confidence. Grab your favorite beverage, and let’s get started!
Statistical Significance
What is Statistical Significance?
Statistical significance is a cornerstone of hypothesis testing. It helps researchers determine if an observed effect is likely to be genuine or simply a product of chance. But how does this all work, you ask? The answer lies in the p-value.
The p-value is a number between 0 and 1 that gauges the strength of the evidence against the null hypothesis. If the p-value is low (typically less than 0.05), researchers reject the null hypothesis. This means the results are statistically significant, suggesting an effect likely exists.
Now, let’s talk about alpha levels. The alpha level is a threshold set by researchers, usually at 0.05. It represents the probability of making a Type I error, which occurs when a researcher incorrectly rejects a true null hypothesis. In simpler terms, it’s the risk of declaring a false positive.
But don’t pop the confetti just yet! While statistical significance is crucial, it’s essential to remember its limitations. A significant p-value doesn’t automatically mean the effect is large or important. It merely suggests there’s enough evidence to question the null hypothesis.
The classic example is in clinical trials. Imagine a new drug that shows a statistically significant effect on reducing symptoms of a disease. If the p-value is 0.04, that’s fantastic! But, if the actual difference in symptoms between the treatment and control groups is minimal—say, the treatment group feels only a smidge better—then the practical relevance of that finding may be negligible.
Understanding statistical significance is vital for researchers and practitioners alike. It can lead to informed decisions based on data. However, we must also consider practical significance to ensure the findings have meaningful implications in the real world.
In summary, statistical significance provides a mathematical basis for evaluating hypotheses. But it’s just one piece of the puzzle. As we continue this journey, we’ll explore the misconceptions surrounding statistical significance and how various factors can influence its interpretation. Stay tuned!
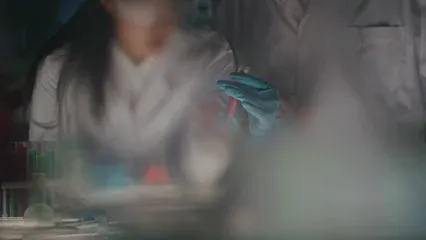
Misconceptions about Statistical Significance
Statistical significance is often misunderstood. Many people believe it equates to real-world relevance. Spoiler alert: it doesn’t! Just because a result is statistically significant doesn’t mean it matters in everyday life.
Take a classic example from the medical field. A study finds that a new drug lowers blood pressure by a statistically significant amount. The p-value is below 0.05, signaling that the results are not likely due to chance. But wait! The actual reduction is a mere 0.1 mmHg. Does that warrant a prescription? Probably not!
Another eye-opener comes from education research. Imagine a study that shows a new teaching method improves test scores by a statistically significant margin. However, the increase is just two points on a 100-point scale. While statistically significant, the change may not be enough to impact student learning meaningfully.
These examples highlight a crucial point: statistical significance can be misleading. It’s essential to assess whether the findings translate to real-world impact.
To better understand these concepts, consider reading “Naked Statistics: Stripping the Dread from the Data” by Charles Wheelan. This book breaks down complex statistical concepts into relatable and digestible nuggets of wisdom.
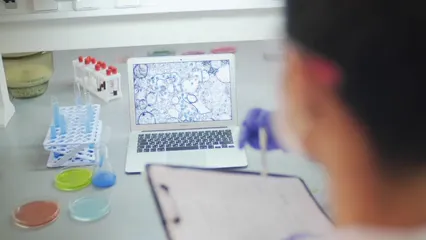
Factors Affecting Statistical Significance
Several factors contribute to statistical significance, and understanding them can prevent confusion. One of the biggest culprits is sample size. Large sample sizes can produce statistically significant results, even if the actual effect is minimal.
Consider this: in a study with 10,000 participants, a tiny difference can yield a statistically significant p-value. Yet, that difference might be practically insignificant. For example, a change in the average height of participants by just 0.2 cm can be deemed statistically significant. But let’s be honest—does that really matter in the grand scheme of things?
Variability in the data also plays a role. When sample data have low variability, researchers can detect smaller effects. This leads to results that sound impressive but lack practical relevance.
In summary, both sample size and variability can inflate the perception of significance. Recognizing these factors is vital for interpreting research findings accurately. It’s not just about being statistically significant; it’s about being meaningful in practice!
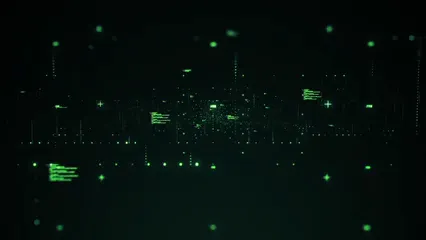
What is Practical Significance?
Practical significance refers to the real-world relevance of research findings. It’s not just about whether an effect exists, but whether that effect matters in everyday situations. While statistical significance tells us that something is likely not due to random chance, practical significance assesses the impact of that finding.
Imagine a company rolling out a new training program. The results show a statistically significant increase in employee productivity. However, if the increase is merely a few seconds per task, is it worth the cost of implementing the program? This is where practical significance comes into play. A tiny gain might not justify the time or resources spent.
Effect size is a key player in determining practical significance. It quantifies the magnitude of a difference or impact, allowing researchers to evaluate how substantial that effect is in real-world terms. For instance, a medication that lowers blood pressure by 0.1 mmHg may be statistically significant, but it hardly qualifies as practical when considering health implications.
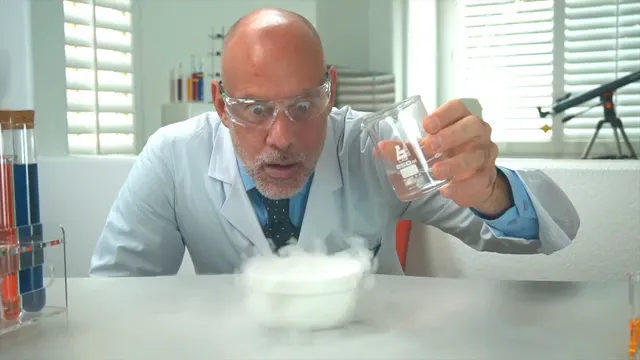
To further enhance your understanding, check out “How to Measure Anything: Finding the Value of ‘Intangibles’ in Business” by Douglas W. Hubbard. This book provides insights into quantifying the unquantifiable, making practical significance more accessible!
In summary, while statistical significance shows that an effect exists, practical significance highlights whether that effect is meaningful in the real world.
Determining Practical Significance
Assessing practical significance isn’t a one-size-fits-all approach; it involves various methods. Confidence intervals (CIs) are one of the most effective tools for this task. A CI provides a range of values around an estimated effect, helping researchers gauge the precision and relevance of their findings.
For example, consider a study assessing a new teaching method. If the CI for test score improvement ranges from 5 to 10 points, and educators deem a 5-point increase as meaningful, then the results are practically significant. Conversely, if the CI includes values less than 5, the effect might not be considered worthwhile.
Effect size analysis is another valuable method. By calculating effect sizes, researchers can quantify how large an impact is in comparison to a baseline or standard. For instance, in evaluating medical treatments, an effect size of 0.8 indicates a large and meaningful effect, while a small effect size of 0.2 might suggest that the treatment is not worth pursuing further.
Practical significance shines in various contexts, such as educational interventions and medical treatments. In education, a new curriculum might show a statistically significant improvement in student scores, but the actual increase must be deemed beneficial to warrant its implementation. In medicine, a drug showing a statistically significant reduction in symptoms must also provide enough symptom relief to make it a viable option for patients.
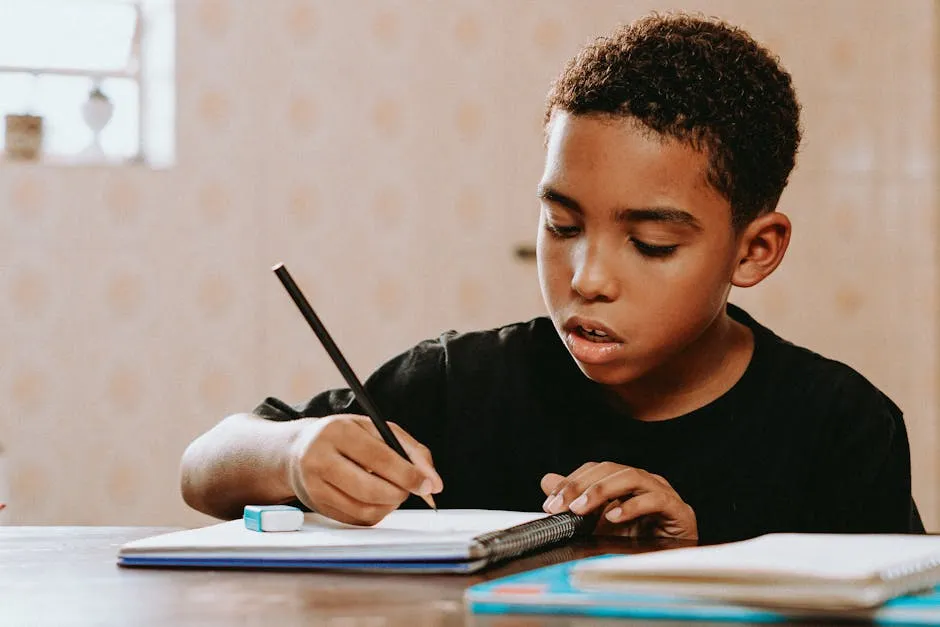
Case Studies
To truly grasp practical significance, examining real-world case studies is invaluable. Take a clinical trial for a new weight-loss drug. Researchers report statistically significant weight loss over six months. However, the average participant lost only 1 pound. While the results are statistically significant, from a practical standpoint, such a minimal impact might lead doctors to question the drug’s viability for patients.
In education, a study evaluates a tutoring program’s effect on student performance. The results indicate a statistically significant rise in scores, but when the effect size is examined, it reveals a mere 2-point increase on a 100-point scale. Teachers may find this improvement insufficient to justify the program’s costs.
These examples underscore the importance of evaluating both statistical and practical significance. Just because a study shows statistically significant results doesn’t guarantee that those results have meaningful implications in the field. Researchers must rely on their expertise and the context of their findings to determine whether an effect is practically significant, ensuring their work translates into valuable real-world applications.
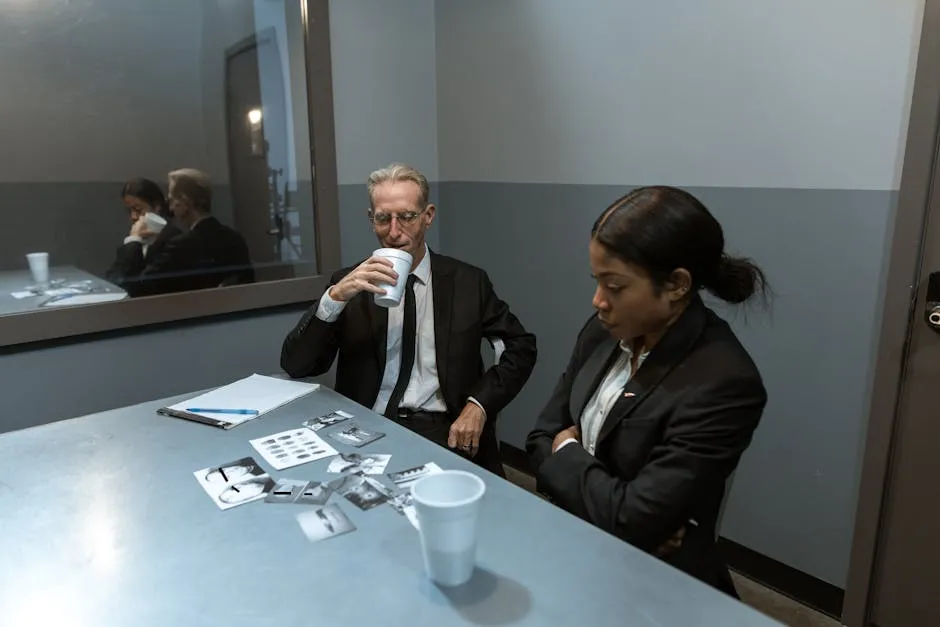
The Relationship Between Statistical and Practical Significance
Highlight the Importance of Subject Area Expertise in Determining Practical Significance
When it comes to research, understanding statistical vs practical significance is like navigating a two-way street. You need to see both directions clearly to make informed decisions. Subject area expertise plays a vital role here, ensuring that findings are not just numbers on a page but actual tools for real-world application.
Imagine a researcher studying a new educational method that shows a statistically significant improvement in test scores. The p-value might scream, “Look at me! I’m significant!” But if the increase is a mere two points on a 100-point scale, does that really warrant a celebration? Probably not. This is where expertise in the field is crucial. A seasoned educator can assess whether that two-point bump translates into meaningful learning improvements or if it’s just statistical fluff.
Consider another example: a clinical trial for a new drug. The results may show statistically significant outcomes in symptom relief. However, if the actual improvement is minimal—say, just a slight reduction in pain levels—healthcare professionals must weigh whether prescribing the drug is genuinely beneficial for patients. Is the minor relief worth the potential side effects? Here, expert judgment helps bridge the gap between numbers and real-life implications.
In many fields, what constitutes a “meaningful” effect varies dramatically. A statistician might focus on p-values and confidence intervals, while a psychologist might look for practical implications in therapy sessions. The same goes for educators, physicians, and even business analysts. Each discipline has its own standards for what matters in practice, and expertise helps navigate these nuanced waters.

Furthermore, the context surrounding the research is key. For instance, an effect size that seems trivial in one study could be groundbreaking in another. A small effect in a high-stakes medical trial can have life-altering implications. Conversely, a similar effect in a less critical setting might be dismissed as irrelevant. Subject area experts can provide insights that statistics alone cannot offer.
In summary, while statistical significance provides a starting point, it’s the practical significance that truly counts. Subject area expertise is essential for interpreting findings correctly and assessing their real-world relevance. This understanding not only enhances the quality of research but also ensures that data-driven decisions lead to meaningful outcomes.
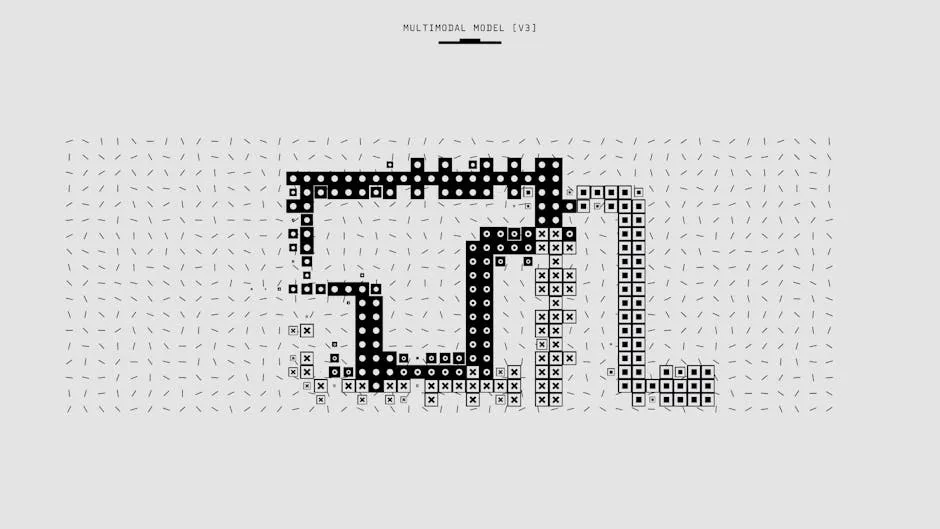
The Importance of Transparency in Reporting Significance
Transparency in research is like a well-lit stage in a theater; it allows the audience to see the entire performance. This clarity is especially crucial when reporting both statistical and practical significance. Researchers must be upfront about their findings to foster trust and credibility.
Statistical significance, often signaled by p-values, tells us whether an observed effect is likely to be real or just a fluke. However, without context, these numbers can mislead. For instance, a p-value can hint at a significant result, yet the actual effect might be negligible. This is where practical significance steps in. It assesses the real-world relevance of those findings. A tiny effect might be statistically significant but can fail to make a meaningful impact in practice.
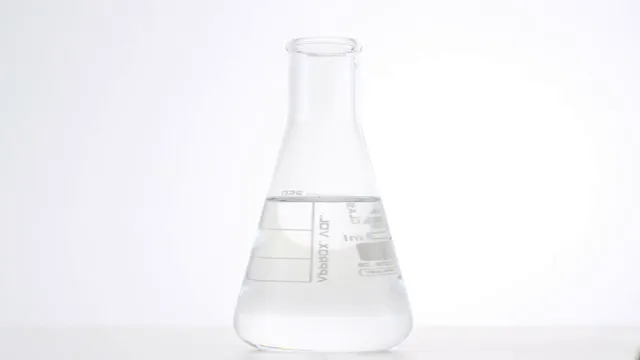
To emphasize transparency, researchers should clearly report both types of significance alongside effect sizes and confidence intervals. This allows readers to grasp not only if a finding is significant but also its importance in practical terms. When both statistical and practical significance are reported transparently, it enables informed decision-making, guiding policymakers and practitioners toward choices that genuinely matter.
Examples of Integrated Analysis
Let’s illustrate the integration of statistical and practical significance with a couple of relatable examples.
Example 1: A New Medication Trial
Imagine a clinical trial for a new medication intended to reduce anxiety. The trial reports a statistically significant reduction in anxiety levels, with a p-value of 0.03. However, the actual reduction measured is only 1 point on a 10-point scale. While the p-value indicates significance, the practical significance is questionable. Patients and doctors may wonder if a 1-point reduction is meaningful enough to warrant this new medication.
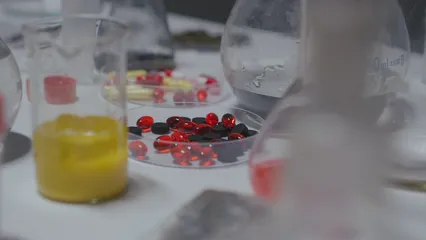
Example 2: Educational Intervention
Consider a study assessing a new teaching method. The results show a statistically significant increase in student test scores, but the average improvement is just 2 points out of 100. While statistically significant, educators need to evaluate whether this small improvement justifies the time and resources required for implementation.
Visual aids can enhance these examples. A graph comparing the p-values and effect sizes of various studies can effectively demonstrate how statistical significance doesn’t always equate to practical relevance. Tables summarizing findings, including both statistical and practical significance, can provide readers with a clear overview of the implications. By integrating these analyses, researchers can present a holistic view of their findings, aiding others in making informed decisions.

Conclusion
In the grand tapestry of research, understanding both statistical and practical significance is essential. While statistical significance highlights whether an effect exists, practical significance determines if that effect matters in real life. Researchers, practitioners, and policymakers must grasp these concepts to avoid misguided decisions based solely on numbers.
When researchers report both types of significance, they empower their audience with the knowledge needed to make informed choices. This transparency fosters trust and integrity in research, ensuring that findings translate into meaningful actions.
To dive deeper into this topic, consider exploring further readings that clarify the nuances of significance in research. For instance, resources on effect size analyses can provide valuable insights into interpreting research findings more effectively.
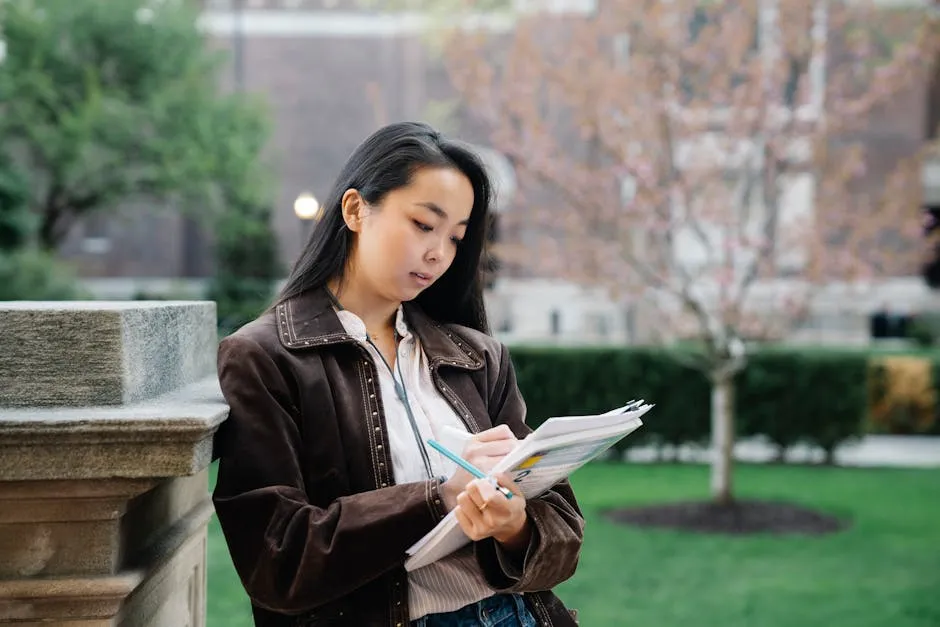
By grasping the importance of both statistical and practical significance, we can ensure that research truly informs and improves practices in various fields, from healthcare to education and beyond.
Before you go, don’t forget to check out some essential tools for your research journey, like a Statistical significance testing kit or an Effect size calculator. They can make your data analysis smoother and more efficient!
Please let us know what you think about our content by leaving a comment down below!
Thank you for reading till here 🙂
All images from Pexels