Introduction
Statistics is the unsung hero in countless fields. Whether you’re a clinician assessing patient outcomes, a researcher deciphering data trends, or a business professional analyzing market behaviors, statistics is your best friend. It helps us make sense of the chaos and provides a framework for informed decision-making.
But here’s the catch: Not everyone has a statistics degree. Many professionals, while brilliant in their domains, often find statistics daunting. This article aims to bridge that gap. We will simplify essential statistical concepts, methods, and analyses to empower those who aren’t statisticians.
Our target audience? Clinicians, researchers, and anyone who needs to interpret statistical data without a PhD in statistics hanging on their wall. By the end of this guide, you’ll have a foundational understanding of how statistics can enhance your work and decision-making processes.
So, whether you’re wrestling with a research paper, preparing a business proposal, or just trying to understand that elusive data set, this guide is for you. Let’s unravel the mystery of statistics together.
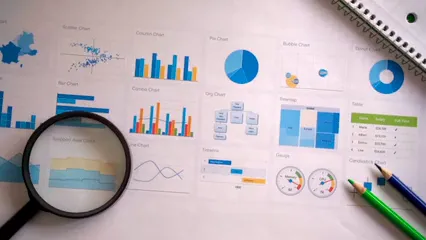
Understanding Statistics
What is Statistics?
At its core, statistics is the science of collecting, analyzing, interpreting, and presenting data. It’s like the detective of the data world, helping to uncover truths hidden beneath the surface. In decision-making, statistics allows us to draw conclusions from data, guiding us toward better choices based on evidence rather than gut feelings.
Statistics can be divided into two main branches: descriptive and inferential statistics. Descriptive statistics summarizes and describes the characteristics of a dataset. Think of it as the tour guide of your data—it highlights the most important features without making assumptions about the larger context.
On the flip side, inferential statistics takes things a step further. It allows us to make predictions or generalizations about a population based on a sample. This is where things get exciting! With inferential statistics, we can estimate population parameters, test hypotheses, and make decisions based on data samples.
If you’re looking to dive deeper into the world of statistics, consider reading “The Art of Statistics: Learning from Data” by David Spiegelhalter. This book is a fantastic resource that demystifies statistical concepts and makes them accessible to anyone!
Descriptive Statistics
Descriptive statistics is all about summarizing data. Three key concepts here are the measures of central tendency: mean, median, and mode.
– Mean: The average value of a dataset. Imagine you’re at a dinner party with 10 friends, and you order 10 pizzas. If everyone eats two slices, the mean consumption is two slices per person.
– Median: This is the middle value when data is sorted. If your pizza party had an odd number of guests, the median would represent the number of slices eaten by the person exactly in the middle.
– Mode: The most frequently occurring value in your dataset. In our pizza scenario, if three friends devoured an extra slice each, then three slices would be the mode.
Next, let’s talk about measures of variability, which tell us how spread out our data is. The range, variance, and standard deviation are key players here.
– Range: The difference between the highest and lowest values.
– Variance: A measure of how far a set of numbers is spread out from the mean.
– Standard Deviation: It’s the square root of the variance, giving us a sense of how much individual data points deviate from the mean.
In real life, descriptive statistics helps us summarize complex data, making it easier to understand trends and patterns. Whether you’re analyzing patient recovery times or tracking sales performance, these statistics bring clarity to the chaos.
With this foundational knowledge, you’re now equipped to tackle more complex statistical concepts. Understanding these basics is like learning to ride a bike; once you get the hang of it, you can explore more challenging terrains. So, let’s pedal forward!
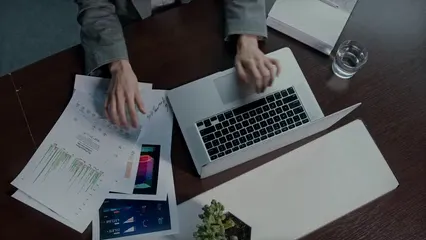
Inferential Statistics
Inferential statistics is the superhero of the statistical world. It swoops in to help us draw conclusions about large populations based on small samples. Imagine trying to guess the favorite pizza topping of an entire city by asking just a few random folks. That’s the essence of inferential stats!
Key concepts here include:
– Population: This refers to the entire group we want to understand. Think of it as the entire pizza-loving city.
– Sample: A smaller group selected from the population. This is our random group of pizza enthusiasts.
– Sampling Methods: Techniques to select the sample. Random sampling, stratified sampling, and systematic sampling are popular choices. They help ensure our sample reflects the population accurately.
With inferential statistics, we can make predictions or generalizations that are statistically valid. This means that even if our sample group isn’t perfect, we can still make educated guesses about the larger crowd.
If you’re interested in mastering the art of statistics, consider picking up “Naked Statistics: Stripping the Dread from the Data” by Charles Wheelan. It’s a witty and engaging read that will make statistics feel less scary!
Types of Data
Data Categories
Understanding the types of data is crucial in statistics. It’s like knowing which tools to use in your toolbox. Let’s break it down into four main categories: nominal, ordinal, interval, and ratio data.
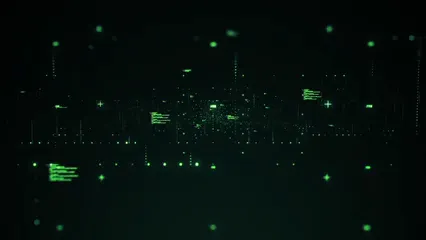
Nominal Data
Nominal data is all about names and categories. This type of data doesn’t have any numerical value. Think labels!
Examples include:
- Colors of cars: red, blue, green.
- Types of cuisine: Italian, Mexican, Indian.
The importance of nominal data lies in its ability to categorize non-numeric information. It’s like sorting your closet by color instead of size!
Ordinal Data
Ordinal data ranks items in a meaningful order, but the intervals between ranks aren’t uniform. It’s like a competitive race where finishing times matter, but the gaps between them can vary.
For instance:
- Satisfaction surveys often use ordinal scales: very dissatisfied, dissatisfied, neutral, satisfied, very satisfied.
- Movie ratings: 1 to 5 stars.
Ordinal data helps in understanding preferences and trends, making it a favorite in surveys and research!
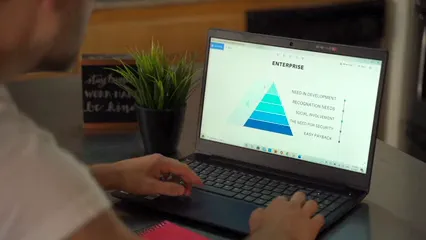
Interval and Ratio Data
Interval and ratio data are the fancy pants of the data world. These types have numerical values and allow for arithmetic operations.
Interval Data: This data type has meaningful intervals but lacks a true zero point. A classic example is temperature measured in Celsius or Fahrenheit. You can say 20 degrees is warmer than 10 degrees, but 0 degrees doesn’t mean ‘no temperature.’
Ratio Data: This type has all the features of interval data, plus a true zero point. Think height, weight, and age. If someone weighs 0 kg, they literally don’t weigh anything!
Both interval and ratio data are essential for advanced statistical analysis. They provide the backbone for calculations and comparisons in research.
By understanding these data types, you can select appropriate statistical methods and accurately interpret results. It’s like choosing the right recipe for your favorite pizza!
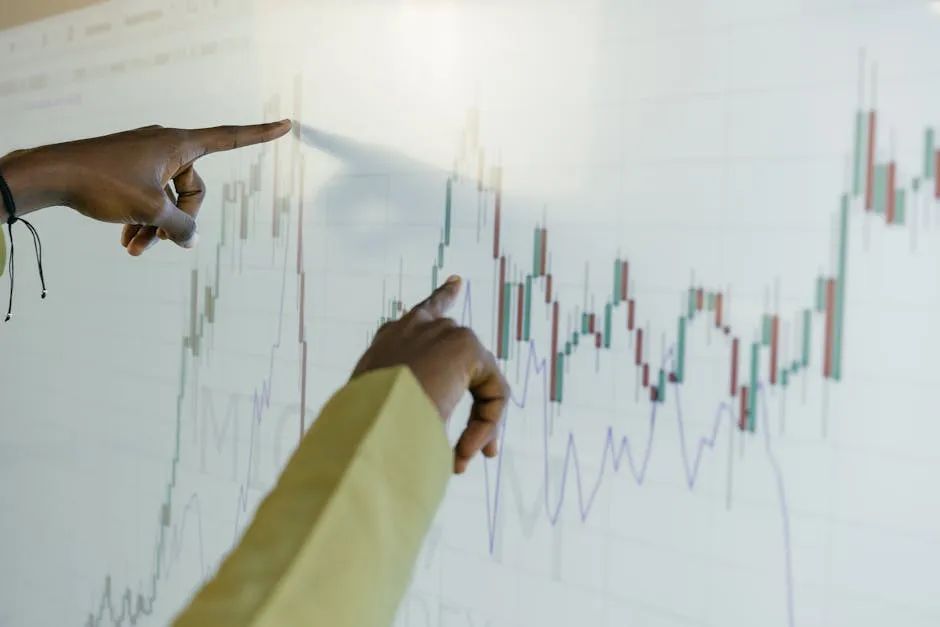
Core Statistical Concepts
Hypothesis Testing
Hypothesis testing is a fundamental concept in statistics. It helps researchers make decisions based on sample data. At the heart of this process are two competing statements: the null hypothesis and the alternative hypothesis. The null hypothesis, often denoted as H0, suggests that there is no effect or no difference. On the other hand, the alternative hypothesis, denoted as H1, proposes that there is an effect or a difference.
Imagine you want to test if a new medication is effective. Your null hypothesis might state, “The new medication has no effect on blood pressure.” The alternative hypothesis would claim, “The new medication lowers blood pressure.”
To determine which hypothesis is more likely true, researchers calculate the significance level (alpha), usually set at 0.05. This level indicates the probability of rejecting the null hypothesis when it’s actually true. In other words, there’s a 5% chance of making an error if the null hypothesis holds.
Next, p-values come into play. A p-value measures the strength of the evidence against the null hypothesis. If the p-value is less than the alpha level, we reject the null hypothesis. So, if our p-value is 0.03, we have enough evidence to suggest the medication is effective. However, if the p-value is 0.08, we fail to reject the null hypothesis. In this case, we don’t have sufficient evidence to support the new medication’s effectiveness.
For a deeper dive into hypothesis testing, check out “How to Lie with Statistics” by Darrell Huff. This book will have you questioning everything you thought you knew about data!
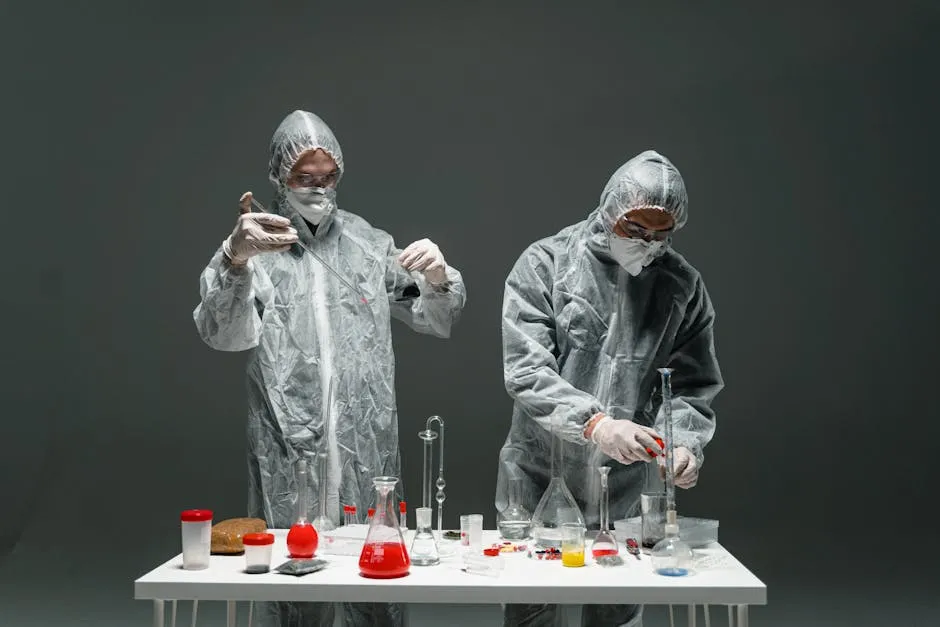
Common Tests
Now, let’s explore some common statistical tests used in hypothesis testing.
- T-tests: These tests compare means between two groups. There are two types:
- Independent T-tests assess means from different groups, like comparing the blood pressure of patients on the new medication versus those on a placebo.
- Paired T-tests look at means from the same group at two different times, such as measuring blood pressure before and after treatment in the same patients.
- ANOVA (Analysis of Variance): ANOVA is useful when comparing means across three or more groups. For instance, if you want to compare the effectiveness of three different diets on weight loss, ANOVA can help determine if at least one diet leads to different outcomes.
- Chi-square tests: These tests evaluate relationships between categorical variables. For example, you could use a chi-square test to see if there’s a relationship between smoking status (smoker vs. non-smoker) and lung cancer incidence.
Each test has its specific use case, and understanding when to apply them is crucial for accurate data analysis.
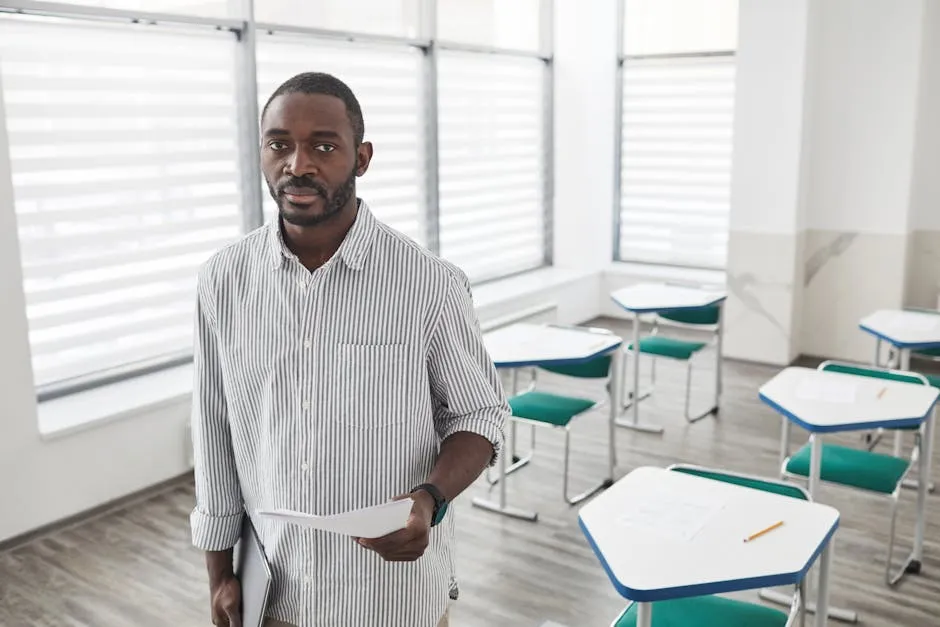
Correlation and Regression
Correlation measures the strength and direction of a relationship between two variables. It provides insights into how they move together. For instance, as ice cream sales increase, so do the number of people wearing sunglasses.
The most commonly used correlation coefficient is Pearson’s correlation coefficient (r). It ranges from -1 to 1. A value of 1 indicates a perfect positive correlation, meaning that as one variable increases, the other does too. A value of -1 signifies a perfect negative correlation, where an increase in one variable results in a decrease in the other. A value of 0 means no correlation exists.
Imagine studying the relationship between hours studied and exam scores. If you find a Pearson’s r of 0.8, it suggests a strong positive correlation: more study hours likely lead to higher exam scores.
Regression analysis goes a step further. It not only shows relationships but also predicts outcomes. Simple linear regression examines the relationship between one independent variable and one dependent variable. For example, predicting a student’s test score based on the number of hours studied.
On the other hand, multiple regression involves two or more independent variables. You might use it to predict a person’s weight based on their height and age.
For a more comprehensive understanding of regression, consider reading “Statistics for Data Science” by James D. Miller. This book is packed with practical examples to help you apply statistical concepts effectively!
Understanding these core statistical concepts equips you with the knowledge to analyze data effectively. Whether you’re testing a new treatment, exploring relationships between variables, or making predictions, these tools are invaluable in the world of statistics.

Statistical Software and Tools
Software Overview
Navigating the world of statistics can be a wild ride, especially with the plethora of software tools at your disposal. Let’s take a closer look at some popular options: SPSS, R, and SAS. Each has its own flair, catering to different users and needs.
SPSS (Statistical Package for the Social Sciences) is the go-to for social scientists and researchers. It boasts an intuitive interface, making it user-friendly for nonstatisticians. With SPSS, you can perform complex statistical analyses with just a few clicks. It excels in descriptive statistics, regression analysis, and hypothesis testing. The best part? You don’t need to write a single line of code!
R, on the other hand, is like the Swiss Army knife of statistical software. It’s open-source and comes packed with packages for nearly every statistical application you can imagine. While R requires a bit of coding know-how, its flexibility makes it a favorite among statisticians and data scientists. Want to create stunning visualizations? R has your back with libraries like ggplot2.
Then we have SAS (Statistical Analysis System), which is a heavyweight champion in the realm of data analytics. It’s widely used in industries such as healthcare and finance. SAS offers powerful data management capabilities and complex statistical analyses. However, it can be a bit on the pricey side, which might make it less appealing for casual users.
In summary, whether you prefer the simplicity of SPSS, the versatility of R, or the power of SAS, there’s a statistical tool out there to fit your needs. No matter which one you choose, understanding how to leverage these tools can enhance your data analysis game significantly.

Practical Applications of Statistics in Research
Real-World Examples
Statistics aren’t just numbers in a textbook; they’re the backbone of decision-making across various fields. Let’s explore how statistics are applied in clinical research, business analytics, and social sciences.
In clinical research, statistics play a crucial role in determining the effectiveness of new medications. For instance, when testing a new drug, researchers use random sampling to select participants. By analyzing the data collected from these samples, they can infer how the drug will perform in the broader population. A classic example is a clinical trial that evaluates the efficacy of a new heart medication. The results guide healthcare professionals in prescribing the right treatment to patients, potentially saving lives.
Moving on to business analytics, companies rely heavily on statistical methods to make informed decisions. Take Netflix, for example. They analyze viewer data to determine which shows to produce next. By examining patterns and preferences, Netflix can predict what will keep subscribers glued to their screens. This data-driven approach has revolutionized the entertainment industry, highlighting how statistics can shape business strategies.
In the social sciences, statistics help researchers understand societal trends. For example, polling data during elections provides insights into voter preferences. By using techniques like regression analysis, researchers can determine the factors influencing voter behavior. This knowledge not only informs political campaigns but also contributes to a better understanding of societal dynamics.
If you want to understand how data mining fits into this picture, check out “Data Science for Business: What You Need to Know about Data Mining and Data-Analytic Thinking” by Foster Provost. It’s a must-read for anyone looking to leverage data in their business decisions!
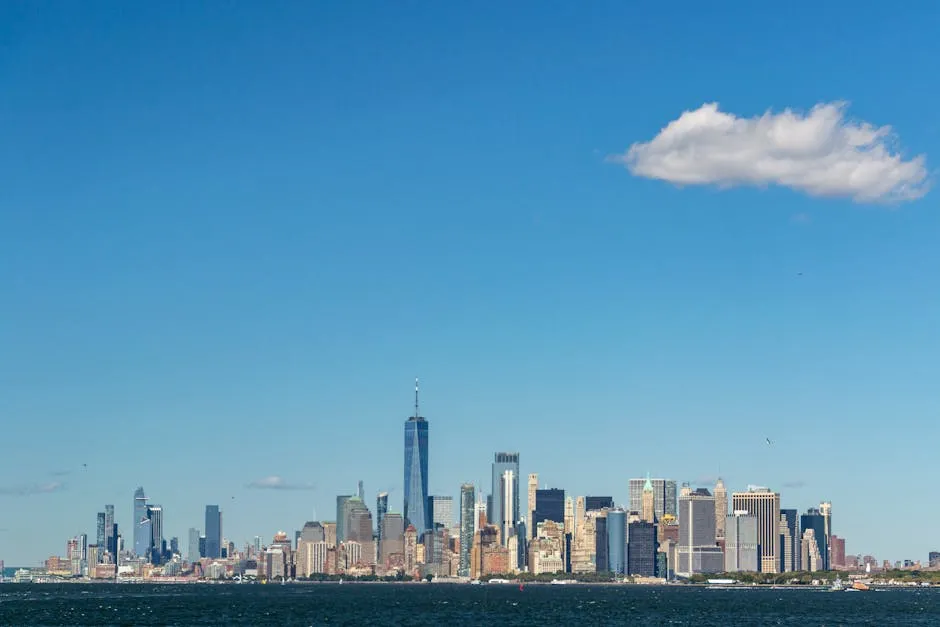
Interpreting Statistical Results
Reading statistical reports can feel like deciphering an ancient language. But fear not! Here are some tips to help you interpret results effectively.
First, always check the sample size. A larger sample typically leads to more reliable results. If the sample size is too small, the findings may not be generalizable to the broader population.
Next, pay attention to the p-values. A p-value less than 0.05 typically indicates statistical significance, suggesting that the observed results are unlikely to have occurred by chance. However, a significant p-value doesn’t necessarily imply practical significance. It’s essential to consider the effect size, which measures the strength of the relationship.
Look out for confidence intervals as well. These provide a range within which we can expect the true population parameter to fall. A narrower interval indicates more precise estimates.
Lastly, avoid common pitfalls. Be wary of overgeneralizing results or falling for the “correlation does not imply causation” trap. Just because two variables move in tandem doesn’t mean one causes the other.
By keeping these tips in mind, you can navigate statistical reports like a pro and make informed decisions based on data. Understanding statistics is not just a skill—it’s an essential tool for success in any field!
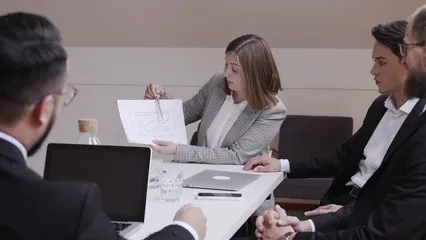
Conclusion
Statistics is more than just numbers and formulas; it’s a crucial tool for effective decision-making across various fields. From healthcare to business, understanding statistical principles empowers professionals to interpret data accurately and make informed choices. In this article, we’ve covered essential aspects of statistics, breaking them down into digestible parts for nonstatisticians.
We began by defining statistics and its two main branches: descriptive and inferential statistics. Descriptive statistics helps summarize data, while inferential statistics enables conclusions about larger populations based on samples. We also explored different types of data, such as nominal and ordinal, highlighting their significance in statistical analysis.
Core concepts like hypothesis testing, correlation, and regression provide the framework for understanding and applying statistical methods. We discussed common statistical tests, such as t-tests and ANOVA, and their roles in analyzing data. These tools help professionals draw meaningful insights from complex datasets.
Moreover, we touched on the importance of statistical software, which can simplify the analysis process. Familiarity with tools like SPSS, R, or SAS can enhance your ability to interpret data effectively. Practical applications of statistics in clinical research and business analytics illustrate its real-world relevance, showcasing how informed decisions can lead to improved outcomes.
Grasping statistical concepts is vital for professional growth. It equips you with the knowledge to critically evaluate research findings and understand the implications of data in your field. As you embark on this statistical journey, we encourage you to seek further resources or training. Online courses, textbooks, and tutorials can deepen your understanding and enhance your skills. Statistics may seem intimidating at first, but with practice and the right tools, you can harness its power to elevate your decision-making capabilities.

FAQs
What are the basic types of statistical analysis?
Statistical analysis can be categorized into three main types: descriptive, inferential, and exploratory statistics. Descriptive statistics summarize data characteristics, inferential statistics make predictions about populations based on samples, and exploratory statistics help identify patterns and relationships within datasets.
How do I choose the right statistical test?
Selecting the appropriate statistical test depends on various factors. Consider the type of data you have (nominal, ordinal, interval, or ratio) and your research question. For example, if you want to compare means between two groups, a t-test is suitable. If you’re comparing three or more groups, ANOVA is the way to go.
What should I do if my data does not meet the assumptions for parametric tests?
If your data violates the assumptions necessary for parametric tests, don’t panic! Non-parametric tests serve as excellent alternatives. For instance, if your data isn’t normally distributed, consider using the Mann-Whitney U test instead of the t-test or the Kruskal-Wallis test in place of ANOVA.
Where can I learn more about statistics?
Numerous resources are available for those eager to learn more about statistics. Consider enrolling in online courses on platforms like Coursera or edX. Textbooks, such as “Statistics for Dummies” or “The Art of Statistics” by David Spiegelhalter, can provide foundational knowledge. Additionally, tutorials for statistical software like R or SPSS can enhance your practical skills.
Please let us know what you think about our content by leaving a comment down below!
Thank you for reading till here 🙂
For a deeper understanding of essential statistics concepts, check out this AP Statistics Formula Sheet.
All images from Pexels